A Step-by-Step Guide to Editing The Contribution Letter Mortgage
Below you can get an idea about how to edit and complete a Contribution Letter Mortgage quickly. Get started now.
- Push the“Get Form” Button below . Here you would be introduced into a splasher making it possible for you to make edits on the document.
- Pick a tool you need from the toolbar that pops up in the dashboard.
- After editing, double check and press the button Download.
- Don't hesistate to contact us via [email protected] regarding any issue.
The Most Powerful Tool to Edit and Complete The Contribution Letter Mortgage
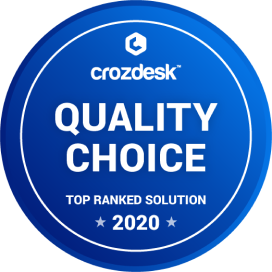
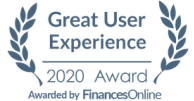
A Simple Manual to Edit Contribution Letter Mortgage Online
Are you seeking to edit forms online? CocoDoc has got you covered with its useful PDF toolset. You can make full use of it simply by opening any web brower. The whole process is easy and quick. Check below to find out
- go to the PDF Editor Page.
- Drag or drop a document you want to edit by clicking Choose File or simply dragging or dropping.
- Conduct the desired edits on your document with the toolbar on the top of the dashboard.
- Download the file once it is finalized .
Steps in Editing Contribution Letter Mortgage on Windows
It's to find a default application which is able to help conduct edits to a PDF document. Fortunately CocoDoc has come to your rescue. Examine the Manual below to form some basic understanding about possible methods to edit PDF on your Windows system.
- Begin by acquiring CocoDoc application into your PC.
- Drag or drop your PDF in the dashboard and make alterations on it with the toolbar listed above
- After double checking, download or save the document.
- There area also many other methods to edit a PDF, you can check this page
A Step-by-Step Guide in Editing a Contribution Letter Mortgage on Mac
Thinking about how to edit PDF documents with your Mac? CocoDoc has got you covered.. It empowers you to edit documents in multiple ways. Get started now
- Install CocoDoc onto your Mac device or go to the CocoDoc website with a Mac browser. Select PDF file from your Mac device. You can do so by pressing the tab Choose File, or by dropping or dragging. Edit the PDF document in the new dashboard which provides a full set of PDF tools. Save the paper by downloading.
A Complete Guide in Editing Contribution Letter Mortgage on G Suite
Intergating G Suite with PDF services is marvellous progess in technology, a blessing for you streamline your PDF editing process, making it faster and more cost-effective. Make use of CocoDoc's G Suite integration now.
Editing PDF on G Suite is as easy as it can be
- Visit Google WorkPlace Marketplace and search for CocoDoc
- set up the CocoDoc add-on into your Google account. Now you are all set to edit documents.
- Select a file desired by hitting the tab Choose File and start editing.
- After making all necessary edits, download it into your device.
PDF Editor FAQ
Do you agree with "publish or perish" in the academic world?
As pointed out by Robert Cameron below, more people are “perishing” rather than publishing. Originally researchers were exchanging letters to each other as a way of communicating what they knew (hence a lot of journals with the name “Letters” in them) and this evolved into the peer review process . These were very formal affairs and they were initially published. In placely like the Royal Society, papers were “read” and then the entire discussion was published (for a very interesting account of this for those metallurgists out there, please find the papers read by TRESCA at the Royal Society). The debate was interesting and polite. I still have some papers by my advisor(where the q and a session was included and it makes for very illuminating reading)This whole process became “turbocharged” as most universities (and whole countries) took leave of their senses by confusing correlation with causationLet me explain:You see, if you did a correlational study before the P or P phenomenon , you would have found that well known professors who have made significant contributions in new areas tend to have a lot of papers with many people, and are likely to have many graduate students: i.e there is a correlation . The first one (significant contributions)was not measurable before the fact, but the second was easily measurable.What happened is that Univ admins, with their (typical) brainlessness, switched cause and effect and thought “hmm so if you publish a lot of papers and have many graduate students you will be well known and make significant contributions” so off we went to the races and we ended up P-ing a lot ( P intended ) :-)). When a Metric becomes a target, it ceases being useful . Your measurement starts influencing the system.Eventually the fever will break and we will find that the emperor has no clothes. Then, (mixing metaphors) the music will end and all the dances will have to find chairs and those left out will perish.But as Chuck Prince (former CEO of Citicorp during the mortgage crisis) so eloquently stated when asked “why didn’t you predict the mortgage crisis?” , “When the music stops, in terms of liquidity, things will be complicated,” Prince said. “But as long as the music is playing, you’ve got to get up and dance.”It is late in the day in academics when we have the morals of wall street, but such is the state today :-((((
How does it feel to get fired from your job suddenly?
Going anonymous for obvious reasons.I have worked in the Indian IT industry in India from 1986. In India, as most Indians know, the concept of “hiring & firing” did not exist - at least not in those times till as late the turn of the century. You were fired only if you were found dishonest, cheating, criminal, involved in physical violence - in or out of office, etc. I started out as any fresher and rose steadily. In 2014, I faced the bomb. My employer lost a few contracts and our group faced problems.One day, my manager, who came down from US, called me and said that I (me) was not getting new business. He said that he was not firing me but “I need to pull up my socks”. I felt pretty weird. I was not dealing with the clients’ business end. As a delivery manager sitting in India, I had no access to the CEO organization that generates new business. The clients’ IT organization was constantly looking to cut costs and cancelling new projects was the easiest option. They also demanded more work to be done from lesser people, without increasing billing rates. Anyway, I was held accountable - rightly or wrongly.The next week, the manager called me again. He said that situation with me is an impasse and I would have to be let go. He said that I would be paid full 3-months’ salary, given the experience and release letter, etc., but I must resign. I was asked to meet the HR and they told me that I must submit office assets at once and I need not come to the office anymore except on the last working day to collect my “settlement”.I was really devastated. I had seen people being fired but never thought that I would face the same fate one day. I had worked hard for this company for seven years. I had received (one of the five) “Employee of the Year” award two years ago for achieving outstanding growth. I had brought the practice from a measly 25 persons to 265 in the previous four years and revenues rose from US$ 1.2 million to US$ 48 m during the same period. Of course, I don’t claim that it was only my achievement - it was always team work. From this company, I was being let off. (Later on, I came to know that my manager, who had recently been hired from Infosys, was bringing his own team along and he was getting rid of “old trees” to make way for “new saplings”.)I was 52 at that time and I knew that I am unlikely to get a new job at my age and future looked extremely bleak. It was ironical and little surreal in some respects:I was fired on November 18, 2014.I had just finished paying all my mortgages and I was financially debt free on the same 1-Nov. I had just received my home documents from the bank two weeks ago.My marriage anniversary was a week later.I was in very much elated state till 17-Nov-2014, notwithstanding the scare of the previous meeting a week ago.Anyway, I submitted my laptop, badge, etc., submitted my resignation, filled up all clearance forms, picked my things and left. Mercifully, no security person came to “see me off”. I walked away still in daze without even saying goodbye to anyone. I was distraught to say the least.As I walked to my car, I saw the company gardener tending to the lawn. I felt even more dejected as I thought that “even that man has a job, however low paying but I don’t and probably won’t”.The only relief was that there were no loans to pay and I had invested enough in the primary and secondary markets to take care of my basic needs for life. But my son’s post graduate education (planned in US) was a question mark.I rode home like a zombie completely unaware of what was happening around me. Probably a few irate drivers honked at me - I can’t say. I came home and told my wife what had happened. She was just as much devastated. Being a housewife, she did not understand what was really happening. I spent 15 days in misery. But ultimately, wisdom woke me up. I knew that I had to try my hand at new job.I applied at a few places via headhunters. I received preliminary calls but after the initial round, it was the standard “we will get back to you answer”. I then started to look at my previous employers. At one place, my ex-Manager had risen to the position of Exec VP and he knew about my talent/skills, my previous contributions and put in a word for me. After 4 months of unemployment, I was back at work. I had to take a salary and designation cut but at least I was working again. I am still here at the same organization.Lessons learnt:In today’s world, past contributions and actions don’t matter; what matters is how much you are worth today.Make your presence felt. You must canvass yourself. This was a mistake on my part - my contributions were unknown beyond a few.Corporate loyalty means nothing; cultivate personal friends and contacts. When I was fired, there was no one to support my case.Have a Plan B and perhaps even a Plan C ready. I cannot tell you what should be your plan B or C but you must have it.If you are fired, it may not be the end of the world. Leave amicably and don’t bad-mouth the ex-employers. The HR of each company have informal connections and they “talk” among each other.Do not assume that your job has a strong cushion. Invest your earnings into instruments that make money:Have a bank balance of at least six months of your gross pay per month.Set aside ₹500,000 per old parent for their medical; most likely they will not be covered by insuranceAlways buy yourself a term insurance of ₹10,000,000 or more and a health insurance of at least ₹500,000 per family member including yourself.Invest in Mutual Funds (if not in stocks). Study good / high ranked MFs in sites such as Valuereasearch.com and invest via SIPs. Follow the equation:Income - Loan Repayments - Savings = ExpensesTry to save at least 50% of your disposable income.Pay off loans as quickly as possible. When you get a bonus, pay in lumpsum.Fortunately, I was following a rigourous savings program from 2005 and I would some cushion had I no job today. Today, I have become even more prudent. I am saving 70% of my take home pay.
What is interesting about normal distribution?
In a sentence: of all continuous distributions over all [math]\mathbb{R}[/math], with a given variance, the normal distribution uniquely maximizes (differential) entropy.And thus, the use of the normal distribution is both incredibly intelligent and incredibly ignorant, which gave humanity many great triumphs — and many abject failures, like this teeny tiny financial shiver that happened about ten years ago[1] :Let’s talk about entropy. Suppose you have a random bit string of about 70% 0s and 30% 1s, that might look something like this:1000000000000001001000001010010001000010 (These could be coin flips, or an instrument readout, or a series of survey “yes/no” responses, or simply any binary signal inside your computer.)This signal is “unfair”, which means we can compress it. Here’s a code that will do the job:“00” -> “a”“01” -> “ba”“10” -> “bba”“11” -> “bbb”(Exercise: explain why this code is unambiguous.) And here’s what we get when we apply the code to the above string:bbaaaaaaabaabbaaabbabbabaabaaabba In this instance, we have compressed 40 characters of input into 33 characters of output.On average, per 2 characters of 0/1 input, we can expect to produce 1.81 characters of a/b output, of which 0.91 will be “a”s and 0.90 will be “b”s (Exercise: why?). This corresponds to a compression of about 10%, and you will see that the output is much “fairer” than the input. We can say that, in a mathematically precise way, the input had less entropy which allowed us to compress it into an output of nearly-maximal entropy. Let us quantify that now.The entropy of a (discrete) probability distribution is just (minus) the expectation value of log probability: that is, given a set of outcomes with probabilities [math]\{p_i\}[/math], the entropy is just[math]H(\{p_i\}) = - \sum_i p_i \log p_i. [/math]Intuitively, the entropy adds together perfectly when you take the joint probabilities of independent events. Suppose event a contributes [math]\log p_a[/math] entropy under distribution A, and event b contributes [math]\log p_b[/math] entropy under distribution B. The probability of joint event ab occurring under joint distribution AB is just [math]p_a p_b[/math] (assuming independence!), and this event contributes entropy [math]\log p_a + \log p_b[/math] just as expected!Calculating the entropy of our earlier bitstring in base 2 gives:[math] H = - 0.7 \log_2 0.7 - 0.3 \log_2 0.3 \approx 0.88 [/math]which is very near the amount of compression we achieved (0.905 compressed symbols per original symbol)*. This is not a coincidence: Shannon’s source coding theorem[2] states that the minimum code rate of a (lossless) compressed signal is just the entropy of the original signal. A bit string of 70% 0s and 30% 1s only “actually” contains 0.88 bits of information per input character, and so we can compress it into at most 0.88 binary characters per input character. A bit string of 90% 0s and 10% 1s, on the other hand, has a (binary) entropy of about 0.46, and so we could compress that data by more than half!As a practical example, consider how to encode the English language. There are 26 letters, so specifying each letter should in theory take between 4 and 5 bits. But English has rules (for example, strings of three consonants are fairly rare), which reduce the actual amount of information each letter carries and thus the actual entropy of the language. According to most estimates, the entropy of the English language is closer to 1 to 2 bits per symbol[3] , and English text is correspondingly quite easy to compress. And we all know this practically: most English speakers can use most (common) seven- or eight-letter words quite easily, and it doesn’t feel like you are picking a word from billions of billions of possibilities — but a seven- or eight-letter password, where the letters don’t follow English rules, is very difficult to remember, and carries a lot more information, enough to uniquely identify you as the holder of a particular computer account.Maximum entropy distributions, therefore, are distributions under which each possible outcome would “carry the most information”. They are therefore the statistically appropriate distributions to use when you have the least information about a phenomenon.But you already knew this. A die rolls 1 to 6; you’ve always assumed each number comes up equally often. You’ve always assumed that because the uniform distribution maximizes entropy over a given range of outcomes. Proving this is an easy application of calculus to the definition of entropy, but the outcome — that the uniform distribution is, in some profound sense, “fair” — is both incredibly trivial and satisfyingly deep.In a similar way, the normal distribution maximizes entropy over a given variance. That is, if you are modelling any process with some variance [math]\mathrm{E}[x^2] = \sigma^2[/math] (where we’ve shifted mean to zero, as we always can), the maximum entropy assumption is that this process obeys the normal distribution[math]Pr(x) = C \exp (-x^2 / 2 \sigma^2). [/math](Yes, we can write out an explicit formula for C, but it’s distracting.) The proof of this[4] is worth going into in some detail if you are at all interested in advanced statistics, and it begins with a related quantity: the Kullback-Leibler divergence, which is (roughly) the relative entropy between two distributions:[math] D_{KL} [f(x) || g(x)] \equiv \int f(x) \log \left( \frac{f(x)}{g(x)} \right) \,\mathrm{d} x = - h[f(x)] - \int f(x) \log g(x) \,\mathrm{d} x \geq 0[/math]and is non-negative, being zero when the two distributions are equal. Now, let’s set [math]g(x)[/math] to the normal distribution, and calculate that second integral term for an arbitrary other distribution [math]f(x)[/math]:[math]\begin{eqnarray*} \int f(x) \log g(x) \,\mathrm{d} x &= \int f(x) \left(\log C - \log(e) \frac{x^2}{2 \sigma^2} \right) \,\mathrm{d} x \\ &= \log C - \frac{1}{2 \sigma^2} \log(e) \int f(x) x^2 \,\mathrm{d} x \\ &= \log C - \frac{1}{2 \sigma^2} \log(e) \sigma^2 \\&= \frac{1}{2} \log C^2/e. \end{eqnarray*}[/math]But wait! That doesn’t depend on [math]f(x)[/math] at all! You see the trick? Because of the functional form of the normal distribution, the second integral calculates the variance no matter what [math]f(x)[/math] is, and that is precisely what we specified! Putting that back into the Kullback-Liebler divergence gives[math] \frac{1}{2} \log C^2/e - h[f(x)] \geq 0[/math]which immediately (1) gives us the entropy of the normal distribution (set [math]f(x) = g(x)[/math] and the RHS is zero), and (2) proves that any other distribution of the same variance has less entropy.So: the normal distribution uniquely maximizes (differential) entropy. Therefore, we use it when we know nothing other than variance about a phenomenon.This is an incredibly interesting and deep perspective on the ubiquity of the normal distribution. We model all kinds of things as normal distributions — heights, weights, intelligences, — and when we do, we profess a kind of intelligent ignorance about the thing we’re modelling. “We don’t know —” we’re saying “ — but we know exactly how to say we don’t know what we don’t know.”I suspect that this links directly to the Central Limit Theorem[5] , which states just that when you add together many independently and identically distributed variables (of specified variance! Where have we heard that before?) you go towards a normal distribution. This in turn has deep links with thermodynamics and statistical mechanics: we’re always modeling things as Boltzmann distributions, with probability proportional to the exponential of minus energy, and our energy expressions are full of quadratic terms — whether the kinetic energy, proportional to velocity squared, or potential energy, where the first approximation to an energy well is quadratic.Where does the Boltzmann distribution, itself, come from? It’s easy to extend the above proof to deduce that, if you look at continuous distributions satisfying some expectation constraint [math]\mathrm{E}[A(x)] = c[/math], the maximum entropy distribution will be [math]g_{max}(x) \propto \exp (-s A(x))[/math] (with some scaling s). But that’s just the good old Boltzmann distribution! How fascinating.But what this also tells us is that, at the end of the day, the normal distribution can represent a kind of intelligent ignorance. To build all our statistics on an untested assumption of normality is, thus, to construct magnificent edifices on surprisingly shaky sand. Which brings us back to this:and the Great Financial Crisis of a decade (!) ago[6] .See, underlying the massive financialization of the world’s economics is the normal distribution, in no small part in the Black-Scholes model[7] which assumes that price movements are normally distributed. We model all kinds of things as normal distributions — such as, say, the expected return on mortgage loans. But we forget that, when we do, we profess a kind of intelligent ignorance about the things we’re modelling. We are saying that mortgage returns have just the one uncertainty, that there is no additional information to them — that there is no entropy minimization to be gotten from scrutinizing incomes, and assets, and approval procedures, and the insidious spectre of correlation, where one home loan going bad means many others in the same neighborhood are also at risk of going bad. And, well, we all know where that got us.In a way, the modern revolutions in statistics and big data are all about trying to push beyond the normal distribution, to chop away at that maximum entropy, to say we can know something more than nothing. It is behind the urge to do nonparametric statistics[8] and machine learning[9] . It is why the sciences are fleeing p-value hypothesis testing and building visualizations that capture complete experimental datasets[10]. Even in statistical physics, there is heated debate about just how far we can push maximum entropy concepts[11] , and how much they really reflect underlying dynamics.But for now, what is interesting about the normal distribution? It is interesting how precisely we can say that we cannot be precise — how much we can infer that we cannot infer — and how powerful such studied stupidity can be, for good or for bad.*I cheated, a little bit: 0.7 is almost the square root of 0.5, and (binary) Huffman coding[12] is particularly efficient when more probable outcomes have probabilities close to inverse powers of two.Footnotes[1] Financial crisis of 2007–2008 - Wikipedia[2] Shannon's source coding theorem - Wikipedia[3] http://citeseerx.ist.psu.edu/viewdoc/download?doi=10.1.1.92.5610&rep=rep1&type=pdf[4] Differential entropy - Wikipedia[5] Central limit theorem - Wikipedia[6] Financial crisis of 2007–2008 - Wikipedia[7] Black–Scholes model - Wikipedia[8] Nonparametric statistics - Wikipedia[9] Machine learning - Wikipedia[10] Moving beyond P values: Everyday data analysis with estimation plots[11] https://iopscience.iop.org/article/10.1088/1751-8113/40/31/N01[12] Huffman coding - Wikipedia
- Home >
- Catalog >
- Finance >
- Loan Form >
- Satisfaction Of Mortgage Form >
- mortgage release letter >
- Contribution Letter Mortgage