A Complete Guide to Editing The Sc 990 Filing Requirements
Below you can get an idea about how to edit and complete a Sc 990 Filing Requirements hasslefree. Get started now.
- Push the“Get Form” Button below . Here you would be introduced into a webpage making it possible for you to make edits on the document.
- Select a tool you need from the toolbar that emerge in the dashboard.
- After editing, double check and press the button Download.
- Don't hesistate to contact us via [email protected] regarding any issue.
The Most Powerful Tool to Edit and Complete The Sc 990 Filing Requirements
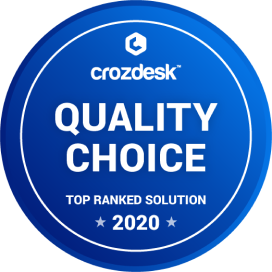
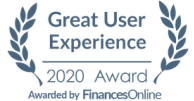
A Simple Manual to Edit Sc 990 Filing Requirements Online
Are you seeking to edit forms online? CocoDoc has got you covered with its detailed PDF toolset. You can make full use of it simply by opening any web brower. The whole process is easy and beginner-friendly. Check below to find out
- go to the PDF Editor Page of CocoDoc.
- Import a document you want to edit by clicking Choose File or simply dragging or dropping.
- Conduct the desired edits on your document with the toolbar on the top of the dashboard.
- Download the file once it is finalized .
Steps in Editing Sc 990 Filing Requirements on Windows
It's to find a default application which is able to help conduct edits to a PDF document. However, CocoDoc has come to your rescue. Examine the Instructions below to know possible methods to edit PDF on your Windows system.
- Begin by acquiring CocoDoc application into your PC.
- Import your PDF in the dashboard and make alterations on it with the toolbar listed above
- After double checking, download or save the document.
- There area also many other methods to edit PDF for free, you can check it here
A Complete Guide in Editing a Sc 990 Filing Requirements on Mac
Thinking about how to edit PDF documents with your Mac? CocoDoc has got you covered.. It allows you to edit documents in multiple ways. Get started now
- Install CocoDoc onto your Mac device or go to the CocoDoc website with a Mac browser. Select PDF file from your Mac device. You can do so by clicking the tab Choose File, or by dropping or dragging. Edit the PDF document in the new dashboard which encampasses a full set of PDF tools. Save the content by downloading.
A Complete Guide in Editing Sc 990 Filing Requirements on G Suite
Intergating G Suite with PDF services is marvellous progess in technology, a blessing for you cut your PDF editing process, making it faster and more time-saving. Make use of CocoDoc's G Suite integration now.
Editing PDF on G Suite is as easy as it can be
- Visit Google WorkPlace Marketplace and search for CocoDoc
- establish the CocoDoc add-on into your Google account. Now you are all set to edit documents.
- Select a file desired by hitting the tab Choose File and start editing.
- After making all necessary edits, download it into your device.
PDF Editor FAQ
Do you know a person that is in prison but innocent?
As you read this, a man is sitting in a steel and concrete box that is 6 feet wide by 9 feet long. He has been sitting in that prison cell for eleven long, soul-crushing years. In 2009, Garvin Vaughn Duvall was convicted by a jury and sentenced to 30+5+5=40 years in prison for murder, conspiracy, and use of a firearm. He has 27 more years to go before he will even be considered for release. After six months of investigation, I have concluded that Garvin is innocent of the crime he is accused of. In fact, after creating a timeline of all the witnesses' testimony I have determined it was physically impossible for the crime, as charged by the State, to have occurred at all.I was introduced to Garvin and his situation about eight months ago. I was asked by a friend of his to read the transcripts of his trial. I do investigative work, conduct interviews, and do ground level research for criminal cases. Garvin's trial transcripts presented a few mysteries so I decided to dig deeper and FOIAed the investigative files of the Sheriff's Department, went to the vault at the courthouse and looked over all the evidence presented at trial, went and took pictures and looked carefully at every physical location involved in the crime, tracked down and interviewed all of the witnesses from the case again and got my hands on a copy of the discovery materials provided by the prosecution. After reading thousands of pages of the case file (some 3 and 4 times) and creating a timeline of the evidence there was no doubt in my mind Garvin was innocent of the crime for which he was accused. I don't know what did happen; but, I do know what did not happen and that was the State's version of this crime that Garvin was charged and convicted of.In 1999, Paul Stephens was murdered on Baugh Road in Anderson County, S.C. and in 2006 a man came forward (seven years after the fact) claiming Garvin had confessed the killing to him. Garvin and a female co-defendant were charged and despite there being no physical evidence (none, nada, no gun, no blood, no saliva, no fibers, no witnesses, no DNA, no nothing) they were found guilty on the say-so of one man by a very confused jury. My investigation has found there was prosecutorial misconduct during the trial, that Garvin suffered ineffective assistance of counsel from his lawyer and that the Sheriff's Department and prosecution withheld exculpatory evidence (evidence that would help prove his innocence) from the defense. I further found there was collusion between Lt. Garland Major and his star witness and that they were Masonic brothers and knew each other well before this case. This fact was not shared with the Court or the jury even though the fact that one of the witnesses from the country store and the prosecutor were related and that fact was pronounced and made public in open court.Garvin was a quiet, gentle, man that had a 9-5 job working at Ryobi as the melt furnace operator. At fifty he had never been in any kind of trouble his entire life. At the time of the murder in 1999, Garvin had three alibi witnesses that said he was on his in-law's front porch during the time frame the murder occurred. Because there was no physical evidence or leads, the case went into the cold case files at the Anderson County Sheriff's Department. Seven years later, a man came forward who said Garvin confessed the details of the killing to him. The man met and spoke with detectives and investigators three times before filing an "official" statement. Each time he spoke with them, the notes they took of the meetings indicated he told a different story each time he met with them. The story grew and got better and better. The lead detective, Lt. Garland Major, was known for cutting a corner or two to make a case if he had to. The man who came forward claimed to be a Grand Dragon in the Klu Klux Klan, a 32nd degree Mason, a catholic priest, a Protestant minister, and a prison evangelist. Quite the character.Two other witnesses worked at a country store about two miles from the crime scene. They claimed to see while loading an order into a vehicle in the parking lot, a white male and female using the public payphone in the parking lot of the store. One could not identify either Garvin or his co-defendant from a photo lineup, but he claimed to recognize their car as a car he saw down at the crime scene a a few minutes earlier while on his way to work. The other identified the female co-defendant but could not make a positive ID on the male despite standing fifteen feet away from them for fifteen minutes. One claimed to observe the two on the payphone until after 3:15pm after which the other said they left headed towards the crime scene.Here is the State's case. On June 30, 1999, at a few minutes before 3:00pm, three phone calls were made from the country store's payphone to the house of the victim's estranged wife. The State claimed they were calling the victim to entice/lure him to come to the crime scene. The three calls were over (according to phone company records) by 2:59pm. The crime scene was a 12-minute drive from the victim's house. At 3:27pm a call to 911 initiated a fire department alarm for a vehicular fire on Baugh Road and the first firetruck arrived on scene at 3:31pm. The 911 call was made by a man passing by who saw the fire, got out, and tried to open the door of the burning vehicle; but, it was too hot to touch. Let's give him two minutes to do all that so that puts us at 3:25pm that someone showed up at the crime scene after the alleged crime took place. If the white male and female at the country store left after 3:15pm and drove to the crime scene that would put them there at 3:18pm. So according to the State, the crime occurred between 3:18 and 3:25. A seven-minute window. The perpetrators would have had to meet with the victim, convince him to back into a grassy off-road path, walk back 20-30 feet into the underbrush, and then shot him in the back of the head and then again through the heart. They then torched the victim's car and fled the scene. All in seven minutes before the first witness showed to make the 911 call at 3:27pm. That all sounds plausible and was a neatly tied package summarizing the role of the perpetrators in carrying out this murder. There is only one problem...well two.Two of the State's expert witnesses were the medical examiner and the chief fire investigator. The medical examiner testified the victim had been shot in the head and then after that through the heart. He knew this because there was an enormous amount of blood trauma and other signs that indicated that although the victim was brain dead from the first shot, that his heart continued to beat and circulate blood throughout the body causing copious amounts of internal and external bleeding. The second shot was through the heart and all pumping and life signs ceased. The medical examiner stated that due to the amount of blood trauma and other indicators that the heart continued to beat up to as much as thirty minutes after the headshot. The chief fire investigator testified the vehicular fire got so hot that it melted the windshield glass, gutted the interior of the car, and burned surrounding shrubs and grass for ten feet. He further testified that the patterns the fire and smoke left were indicators of how the fire progressed and said due to the extreme heat and other factors the fire could have raged for as much as 45 minutes. The severe damage and indications of extreme temperatures in the vehicle showed this was no 3-5 minute fire. So now we have two events that are components of the crime that collectively took one hour and fifteen minutes (give or take a few minutes according to the State's experts) to occur. How do you fit an hour and fifteen minutes of happenings into a seven-minute window? You can't. It is an impossibility. The entire crime as charged was a made-up story. It wasn't based on any facts or evidence other than the one man who claimed Garvin confessed the details of the crime to him. At trial, that man's testimony took another turn from any of his prior assertions and ran lockstep with this scenario the State (they prepped him well), and the lead detective painted describing the crime. So now it is a proven fact that he is a liar as the crime he and the State described could never have occurred.In the seven years between when the crime occurred and the man came forward claiming Garvin confessed to him, two of Garvin's alibi witnesses died. His wife and mother-in-law both died. The third was his father-in-law who at 75 was becoming senile. When Garvin's father-in-law took the stand at trial Garvin's lawyer didn't even bring up the issue of his being Garvin's alibi. Garvin's lawyer failed him in many ways. He should have insisted that his client's trial be separate/severed from the female co-defendants as there was no evidence tying them together. The prosecution said they scheduled them to be tried together for judicial economy (meaning to save the Court time and money). Since when does the government saving money take precedent over an individual's rights to a fair trial and Constitutionally protected due process. When Garvin's lawyer questioned the only witness who claimed Garvin confessed the crime to him, he didn't ask him why every time he met with investigators his story changed or how is it his story just keeps growing and growing or any other number of things he could and should have asked; but, instead he just attacked the man's character and credentials. Mocked him and tried to make him look disingenuous and foolish. Which he may have been; but, it accomplished nothing in getting the facts on the table.A motion under Rule 60(b)(3) fraud, misrepresentation, or other misconduct of an adverse party should be filed regarding the fraud perpetrated on the Court by Lt. Garland Major and his star witness JW Stansell. Although unreported in the trial these two individuals knew each other well. In fact, they were Masonic brothers belonging to the same Masonic lodge or affiliated lodges and had another investigator in this case also belonging to the same group. They had all previously played a role in an application by Garvin Duvall to be a Mason. Upon information and belief, JW Stansell also had elder abuse charges pending against him, which mysteriously disappeared after his testimony in this case. Motivation for him to make these false accusations.JW Stansell had four interviews with investigators prior to trial. His first interview I believe was the truth of the matter as JW Stansell stated he had a passing remark with Garvin Duvall at a birthday party regarding Paul Stephens being a problem and then a week or so after the murder he asked Duvall about it and received "I don't want to talk about it” back as a response." From that point every interview with investigators his story grew and grew into a multi-page story. Multiple allegations by JW Stansell proved untrue or were uncorroborated. ie. That he was shown a weapon, that Duvall melted the gun in the furnace at work (despite the fact that aluminum melts at 1250 degrees, an aluminum furnace only goes to 1400 degrees and weapons grade steel requires 2500 degrees to melt), that Duvall had confessed the crime to him on three occasions, on the one hand in his official statement, Stansell knew nothing about guns and then at trial he recognized by sight a .380 vs. a .38 Special or 9mm merely by looking at its barrel (despite the fact there is no visible difference in the barrel size of all three). None of the information offered by Stansell was anything anyone couldn't have found out by reading the paper or following the case. Stansell testifying to the same facts as the State put forward in the case proves Stansell was lying, as the State's rendition of the charge was impossible to have occurred. An hour and fifteen minutes of events could not have occurred in seven minutes. Simple as that.Please if you have one bone of compassion in your body help me free an innocent man from the hell of prison. I need an attorney and investigator to corroborate my findings and write a petition for a writ of habeas corpus to free Garvin. He has exhausted all of his appeals and post-conviction relief legal actions to no avail. This is his last chance to be exonerated or face 27 more years of hell on earth. Garvin has not had a single institutional infraction in the eleven years he has been in prison and still maintains his innocence. That is exceptional in a prison environment.If you would like to write to Garvin and let him know he has not been forgotten, send him a word of encouragement and tell him there are people on the outside fighting for him his address is.Garvin Vaughn Duvall #334729 F6B-1237-BLee Correctional Institute990 Wisacky HighwayBishopville, SC 29010Join me in freeing an innocent man! Click here to donate to free an innocent man Click here to support Help Free an Innocent Man from prison by Frank Pressly
How is working for a start-up different from a regular job?
The BIG difference:- in a for-profit business, your revenue usually comes from providing services or products to CUSTOMERS who pay you for them.- in a non-profit venture, your revenue usually comes from SOMEONE ELSE besides the people for whom you are providing services (or products, such as food and clothing).For-profit founders and employees can generally assume that their revenue will come from providing the desired services and products to their clients (although they may need some venture capital / startup funding from another source).Non-profit founders and employees (and volunteers - whole 'nother can of worms!) are constantly splitting their time between finding sources of funding and providing services to their clients.We've been working for over 6 years to develop our OSPREY Village project - a "neighborhood with a purpose" to support adults with developmental disabilities and their aging parents in our area (near Hilton Head Island in SC). We have identified a site that we are ready to purchase, have a plan for how to build out the neighborhood, and know what services we need to provide - but are still trying to nail down how to pay for operational expenses without having to charge our future "customers" over $40K per year to provide the residential services that they need!We've even started a thrift store that is now bringing in a nice revenue stream for us - but it's still woefully inadequate when you're working to develop something like this in a state where the only developmentally disabled folks who get funding for residential services (via Medicaid waivers) are those who have nowhere to live or no one to care for them anymore (dead or ill parents). And so there are more people on waiting lists for residential services here than those being served!So "finding the money" is always the biggest challenge for a non-profit - both the startup capital as well as the ongoing operational funding to keep the doors open.The other big difference is that non-profits have to jump through a whole lot more hoops with the IRS and state governments than for-profits do. I started my own S-Corp nine years ago to provide website design and SEO services. No big deal - I just have to report my income to the state DoR and the IRS and pay the anticipated taxes quarterly, then file a corporate return each year. (And pay for a business license from my local municipality annually.)But a non-profit has to be very careful about what "businesses" they engage in that generate revenue - other than thrift stores, any business activities have to be tied to your charitable mission as declared in your Form 1023 (the IRS application for tax exempt status). And you do have to submit a detailed report (Form 990) annually to the IRS and the state as well regarding your funding sources, what you spent it on, your Board members, how many people you've assisted, any conflicts of interest, etc.Run afoul of those rules and you expose your charity to potential Unrelated Business Income Tax (UBIT) payments and penalties or even revocation of your tax-exempt status. And having for-profit business experience, while helpful, doesn't really prepare you for dealing with many of these non-profit-specific issues!So to answer the question: "How is working on a non-profit start-up project different from working for a for-profit start-up project?"It's harder, more complex, and requires more personal investment of time and energy! But it can also be very rewarding when a plan comes together!
How can we compare the difference in the mediation model between two sample sizes?
AbstractAlthough previous research has studied power in mediation models, the extent to which the inclusion of a mediator will increase power has not been investigated. First, a study compared analytical power of the mediated effect to the total effect in a single mediator model to identify the situations in which the inclusion of one mediator increased statistical power. Results from the first study indicated that including a mediator increased statistical power in small samples with large coefficients and in large samples with small coefficients, and when coefficients were non-zero and equal across models. Next, a study identified conditions where power was greater for the test of the total mediated effect compared to the test of the total effect in the parallel two mediator model. Results indicated that including two mediators increased power in small samples with large coefficients and in large samples with small coefficients, the same pattern of results found in the first study. Finally, a study assessed analytical power for a sequential (three-path) two mediator model and compared power to detect the three-path mediated effect to power to detect both the test of the total effect and the test of the mediated effect for the single mediator model. Results indicated that the three-path mediated effect had more power than the mediated effect from the single mediator model and the test of the total effect. Practical implications of these results for researchers are then discussed.Go to:IntroductionMost research studies investigate the relation of an independent variable (X) and a dependent variable (Y). The independent variable X represents exposure to a randomized manipulation or an observed variable. An analysis such as linear regression quantifies the relation of X to Y as a regression coefficient and provides a statistical test of whether the coefficient is larger than expected by chance. Prior to such an undertaking, researchers conduct power calculations to assess the probability of observing a statistically significant relation of X to Y given a Type I error rate, sample size, variability, and effect size. Increasing the Type I error rate, increasing the sample size, increasing the effect size, or reducing variability increases power of a statistical test.As recognition of the importance of mediation analysis has increased, researchers have developed advanced statistical and conceptual models to investigate mediated effects (MacKinnon, 2008). In the mediation model, there is a hypothesized chain of relations between X and Y such that X first causes a change in a variable M that then causes a change in Y. This model explains how X affects Y by elaborating the relation to be X to M to Y. The mediator M is selected for change based on theory and prior empirical research. In addition to the strong theoretical and empirical rationale behind including the mediator, including a mediator may also increase the power to detect effects in some circumstances. This article describes the reason for this somewhat counterintuitive result, along with the conditions when this result occurs, and then describes the practical applications of these results for study planning.We present three studies to illustrate when power to detect the mediated effect is greater than power to detect the total effect. Study 1 compares power values for the mediated effect and total effect from a single mediator model to determine when power of the mediated effect is greater than power of the total effect. Study 2 compares power values for the total mediated effect to the total effect from a parallel two mediator model to determine when the test of the parallel two mediator model has more power than the test of the total effect. Study 3 investigates a sequential two mediator model and compares power values for the three-path mediated effect and the total effect to determine when power of the three-path mediated effect is greater than power of the total effect. Study 3 also compares power of the three-path mediated effect to power of the mediated effect from the single mediator model and power of the total effect to see how power increases differentially across models. Results from these studies reveal the nature of how models with one or two mediators increase power over the total effect. Finally, the practical use of these results is described through several research examples.The Single Mediator ModelFigure 1 shows the relation between an independent variable (X) and a dependent variable (Y), and a three variable model including X and Y when a mediator (M) is added to a model.An external file that holds a picture, illustration, etc.Object name is nihms-602858-f0002.jpgFigure 1Path diagrams for the regression and one mediator models. Adapted from MacKinnon, 2008.The following three regression equations are used to investigate mediation for a single mediator using notation in MacKinnon (2008):Y = i1 + cX + ε1 (1)Y = i2 + c’X + bM + ε2 (2)M = i3 + aX + ε3 (3)From Equation 1, i1 is the intercept for the equation, c is the relation between X and Y (also known as the total effect of X on Y), and ε1 is the variability in Y that is not explained by X. In this article the total effect refers to the c coefficient that represents the relation of X to Y, and applies to all subsequent models described. From Equation 2, b is the relation between M and Y adjusting for X, c' is the relation between X and Y adjusting for M (also known as the direct effect of X on Y that is not through M), and ε2 is the variability in Y that is not explained by its relations with X and M. From Equation 3, a is the relation between X and M, and ε3 is the variability in M that is not explained by X. The coefficients i2 and i3 are the intercepts for the equations. The intercepts are not involved in the estimation of mediated effects and could be left out of the equations, but are included here for completeness. Note that both c and c' are coefficients relating X to Y, but c' is a partial effect adjusted for the effects of the mediator. The mediation coefficients from Equations 2 and 3 are estimated using multiple regression or structural equation modeling. A classic example of a single mediator model is the mediation of the relationship between attitudes (X) and behavior (Y) by intentions (M) (Fishbein & Ajzen, 1975).The Parallel Two Mediator ModelThe parallel two mediator model is an extension of the single mediator model, where X is related to Y through a mediator (M1) and also through an additional mediator (M2). The mediators each have their own specific effects within this model (as opposed to transmitting the effect of X to M1 to M2 to Y), hence the use of the term parallel. The parallel two mediator model is depicted in Figure 2.An external file that holds a picture, illustration, etc.Object name is nihms-602858-f0003.jpgFigure 2Path diagram for the parallel two mediator model. Adapted from MacKinnon, 2008.The following equations represent the parallel two mediator model using notation from MacKinnon (2008):Y = i2 + c’X + b1M1 + b2M2 + ε2 (4)M1 = i3 + a1X + ε3 (5)M2 = i4 + a2X + ε4 (6)From Equation 4, c' is the relation between X and Y adjusting for M1 and M2, b1 is the relation between M1 and Y adjusting for M2 and X, b2 is the relation between M2 and Y adjusting for M1 and X, and ε2 is the variability in Y not explained by its relations with X, M1, and M2. From Equation 5, a1 is the relation between M1 and X, and ε3 is the variability in M1 that is not explained by X. From Equation 6, a2 is the relation between M2 and X, and ε4 is the variability in M2 not explained by X. The coefficients i2, i3, and i4 are the intercepts for the equations. An example of a parallel two mediator model is Salthouse's (1984) study looking at how the effect of age (X) on typing efficiency (Y) was mediated by reaction time (M1) and skill (M2).The Sequential Two Mediator ModelIn the sequential two mediator model, also referred to as the three-path mediator model (Taylor, MacKinnon, & Tein, 2008) or micromediational chain (Cook & Campbell, 1979), two mediators (M1 and M2) intervene between X and Y. This model is shown in Figure 3.An external file that holds a picture, illustration, etc.Object name is nihms-602858-f0004.jpgFigure 3Path diagram for the sequential two mediator model. Adapted from MacKinnon, 2008.The sequential two mediator model is represented by Equations 7, 8, and 9, using notation from MacKinnon (2008) and Taylor et al. (2008):Y = i2 + b4X + b3M2 + b6M1 + ε4 (7)M1 = i3 + b1X + ε2 (8)M2 = i4 + b2M1 + b5X + ε3 (9)From Equation 7, b4 is the relation between X and Y adjusting for M1 and M2, b3 is the relation between M2 and Y adjusting for X and M1, b6 is the relation between M1 and Y adjusting for M2 and X, and ε4 is the variability in Y not explained by its relations with X, M1, and M2. From Equation 8, b1 is the relation between M1 and X, and ε2 is the variability in M1 not explained by X. And from Equation 9, b2 is the relation between M2 and M1 adjusting for X, b5 is the relation between M2 and X adjusting for M1, and ε3 is the variability in M2 not explained by its relations with M1 and X. The coefficients i2, i3, and i4 are the intercepts for the equations.The sequential two mediator model contains several different effects of X on Y. In this model, b4 represents the direct effect of X on Y instead of c' as for the other models. The three effects that form the total mediated effect are the three-path mediated effect (b1b2b3), the two-path mediated effect passing through M1 (b1b6), and the two-path mediated effect passing through M2 (b5b3). The total mediated effect of X on Y passing through either mediator is the sum of the three mediated effects, b1b2b3 + b1b6 + b5b3. As in the two previous models, the total mediated effect is equal to the difference between the total and direct effects shown here in Equation 10:b1b2b3 + b1b6 + b5b3 = c − b4 (10)Taylor et al. (2008) describe a sequential two mediator model from Tekleab, Bartol, and Liu (2005) where the effect of pay (X) on turnover (Y) was mediated by perceived distributive justice (M1) and pay raise satisfaction (M2).Statistical PowerThe power of a statistical test is that test's ability to detect an effect when an effect is truly present in the population (Neyman & Pearson, 1933). Power depends on several key parameters involved in hypothesis testing, namely the Type I error rate, sample size, and effect size. The four parameters Type I error, sample size, effect size, and power are interdependent, and given any three, the fourth may be calculated. A power value of .80 is the standard for adequate power of a hypothesis test in the social sciences (Cohen, 1988). The power to detect the total effect of X on Y, c, is the probability of observing a statistically significant t value given the noncentral distribution for the true total effect of X on Y. The probability that both a and b are statistically significant is the product of the probability to detect each effect separately given that power to detect a and b are independent (Tofighi, MacKinnon, & Yoon, 2009). For the sequential two mediator model, the probability that b1, b2, and b3 are statistically significant is the product of the probability to detect each effect separately given that all paths are uncorrelated. The mediated effect ab is the product of two random variables and the total mediated effect a1b1 + a2b2 is the sum of two products of two random variables. The power to detect these effects is not equal to the probability of observing a statistically significant t value given the noncentral distribution (as it would be for one random variable), because the distribution of the product of two random variables is non-normal (Aroian, 1944; Craig, 1936). Appendix A contains additional details on power computations.Tests of MediationThere are different approaches to estimating mediated effects from the regression models (MacKinnon et al., 2002). For the single mediator model, the product of the aˆ and bˆ coefficients (aˆbˆ) is an estimator of the mediated effect. The effect of X on Y after adjustment for M (cˆ′) is an estimator of the direct effect. If a mediated effect is present and cˆ′ is zero then there is complete mediation. If a mediated effect is present and cˆ′ is not zero then there is partial mediation. The mediated effect is also equal to the difference between the cˆ and cˆ′ coefficients, cˆ−cˆ′. As a result, the total effect cˆ breaks down into a direct effect cˆ′ and a mediated effect aˆbˆ. For the multiple regression equations described above, aˆbˆ=cˆ−cˆ′ and therefore cˆ=cˆ′+aˆbˆ. The rationale behind the aˆbˆ mediation measure is that mediation depends on the extent to which the independent variable affects the mediator (coefficient aˆ) and the extent to which the mediator affects the dependent variable (coefficient bˆ). The aˆbˆ measure reflects how much a one unit change in X affects Y indirectly through M, and the cˆ−cˆ′ measure reflects how much M explains the relation between X and Y. The joint significance test of mediation (MacKinnon, Lockwood, Hoffman, West, & Sheets, 2002) tests whether the aˆ path is statistically significant and whether the bˆ path is statistically significant, and rejects the hypothesis of no mediation if both paths are statistically significant. In addition to the joint significance test, a commonly implemented test of mediation assesses significance of the mediated effect aˆbˆ by dividing the effect by its multivariate delta standard error based on first derivatives, sab=a2s2b+b2s2a−−−−−−−−−√ (Sobel, 1982).For the parallel two mediator model, the estimator of the mediated effect of M1 is the product of aˆ1 and bˆ1, and the mediated effect of M2 is the product of aˆ2 and bˆ2. Individually, these are the specific mediated effects (aˆ1bˆ1 and aˆ2bˆ2) and together, they are the total mediated effect (aˆ1bˆ1+aˆ2bˆ2). In this model, as cˆ is the total effect and cˆ′ is the direct effect of X on Y adjusting for M1 and M2, aˆ1bˆ1+aˆ2bˆ2=cˆ−cˆ′. As there is no test of joint significance for the total mediated effect aˆ1bˆ1+aˆ2bˆ2, the product of coefficients test assesses significance of the total mediated effect by dividing the total mediated effect by its multivariate delta standard error based on first derivatives, sa1b1+a2b2=s2a1b21+s2b1a21+s2a2b22+s2b2a22+2a1a2sb1b2−−−−−−−−−−−−−−−−−−−−−−−−−−−−−−−√ (MacKinnon, 2008).The bootstrap test also assesses significance of the total mediated effect for the parallel two mediator model by bootstrapping confidence intervals around aˆ1bˆ1+aˆ2bˆ2. The bootstrap test generates asymmetric confidence intervals based on the distribution of the mediated effect instead of assuming a normal distribution (MacKinnon et al., 2002; MacKinnon, Lockwood, & Williams, 2004). To obtain a bootstrap estimate of confidence limits for the mediated effect for a sample of size N, the bootstrap procedure takes a bootstrap sample of size N with replacement from the original sample and estimates a mediated effect. This process is repeated a large number of times to create a set of bootstrapped estimates that form a distribution, and that distribution is used to calculate confidence limits for the mediated effect (MacKinnon, 2008). MacKinnon (2008) and MacKinnon et al. (2002) contain additional information on significance tests for these models.For the sequential two mediator model, a joint significance test evaluates the significance of the bˆ1, bˆ2, and bˆ3 coefficients separately (MacKinnon et al., 2002; Taylor et al., 2008). If all paths are statistically significant, mediation is present. For information on other tests of mediation for the sequential two mediator model, see Taylor et al. (2008).Causal assumptions of mediationThis article aims to illustrate when the tests of mediation have more power than the test of the total effect. For the single mediator model, we make several assumptions regarding the causal relations among variables for identification of effects following VanderWeele and Vansteelandt (2009): (1) no confounders of the X to M relation, (2) no confounders of the M to Y relation, (3) no confounders of the X to Y relation, and (4) no effects of X on confounders that then affect the relation of X to Y. Randomization of X satisfies assumptions 1 and 3 which allows for estimators aˆ and cˆ to be treated as causal effects. Randomization of X does not satisfy assumptions 2 and 4 which are required for interpretation of bˆ and cˆ′ as causal effects. Statistical methods that accommodate confounders as well as methods that assess sensitivity may address the violation of these assumptions (Imai, Keele, & Tingley, 2010). Satisfying causal assumptions of mediation becomes more complex with multiple mediators, and is an active area of research (Albert & Nelson, 2011; Avin, Shpitser, & Pearl, 2005; Imai & Yamamoto, 2013; Lange, Rasmussen, & Thygesen, 2013; Robins, 2003).Increased Power Through Inclusion of Additional VariablesThe literature on how including additional variables in statistical analyses can be beneficial is extensive. This idea can be traced back to the foundations of path analysis when it was suggested that researchers should analyze complex or indirect relations among variables, rather than analyzing only direct influences (Wright, 1921; Wright, 1934). R. A. Fisher also summarized the concept: “…When asked in a meeting what can be done in observational studies to clarify the step from association to causation, Sir Ronald Fisher replied: `Make your theories elaborate'” (R. A. Fisher, cited by Cochran, 1965, p. 252). Literature in epidemiology also discussed the idea of making theories elaborate, where researchers proposed that it may be necessary to study the effects of a factor on a disease outcome through multiple channels, requiring more complex theories (Hill, 1965; Yerushalmy & Palmer, 1959). Research on causal inference argues that planned statistical analyses of observational studies should be based on elaborate theories (Rosenbaum, 2010), and that certain definitions of causality are inadequate because they do not address underlying causal mechanisms (Cox, 1992).The increase in power resulting from the inclusion of covariates is particularly well-documented. One of the primary uses of a covariate in analysis of covariance (ANCOVA) is to increase the precision of a randomized experiment by reducing error variability (Cochran, 1957; Cox, 1960; Huck, 1972). Miller and Chapman (2001) agree that the main goal of using ANCOVA should be to increase power to detect effects instead of using it to control for group differences, as is often done in practice. Although theory must determine whether inclusion of a mediator is appropriate and inclusion of a covariate may be data-driven, both serve to increase statistical power to detect effects in some situations.Surrogate endpoints are also frequently used in medical research as a means of increasing power. Researchers may wish to study the effect of X on an outcome Y which is difficult or costly to measure, so they will find an intermediate endpoint Z that is a surrogate for Y such that X affects Z as it would Y, and Z is a predictor of Y (Prentice, 1989, p. 432). For example, the presence of polyps has been used as a surrogate endpoint for the outcome of colon cancer (Freedman & Schatzkin, 1992). Sample size or effect size increases through the use of surrogate endpoints (thereby increasing power).Related to these concepts is the idea of an intensive design: including intermediate points of measurement and using the weighted average of those responses for each subject as an outcome instead of just one response (Kraemer & Thiemann, 1989). The intensive design increases power without requiring an increased sample size over a posttest-only randomized experimental design (Kraemer & Thiemann, 1989), and also increases power over a pretest-posttest design given certain conditions, although this design requires more measurement points (Maxwell, 1998; Venter, Maxwell, & Bolig, 2002).Increased Power Through Inclusion of MediatorsIn light of this research, it follows that including a mediator would increase power in some situations. Cox (1960) showed that including intermediate variables in the relation between X and Y would increase precision. MacKinnon et al. (2002) identified the tests of mediation with the best power and Type I error rates, and found that due to the requirement of a significant X to Y relation, the Baron and Kenny (1986) and Judd and Kenny (1981) causal steps tests for mediation are underpowered. Fritz and MacKinnon (2007) replicated this result, finding that when a and b paths were small and c' was zero, the sample size required to detect the mediated effect at .80 power for the Baron and Kenny test was 20886. Shrout and Bolger (2002) also stated that power to detect mediation is greater than power to detect a total effect of X on Y in some situations, and gave an example of a situation where power to detect the a and b effects would be greater than power to detect the c total effect. This means that some tests of mediation detect significant mediated effects when the relation between X and Y is nonsignificant (Fritz, Cox, & MacKinnon, 2013; MacKinnon, 2008, 394–395; Shrout & Bolger, 2002; Taylor et al., 2008). It follows that including a mediator, or mediators, results in increased power over the total effect in some situations. The purpose of this article is to describe when these situations occur.Go to:Study 1As described above, there is evidence that the single mediator model will have more power to detect effects than the total effect under certain circumstances. Study 1 compared power values of the mediated effect ab and the total effect c for the single mediator model to determine when the mediated effect has more power.MethodAnalytical power for the single mediator model was calculated based on derivations in Appendix A. Both SAS and R programs to compute analytical power for the single mediator model may be downloaded at Research in Prevention Laboratory (MacKinnon). For the single mediator model, population variances and covariances for X, M, and Y were calculated based on different combinations of population path parameters and sample sizes. The covariance matrix for the single mediator model is given in MacKinnon (2008). Sample sizes of 50, 100, 200, 500, 1000, and 5000 were chosen based on those commonly found in the social sciences and in mediation research (Fairchild, MacKinnon, Taborga, & Taylor, 2009; MacKinnon et al., 2002; MacKinnon et al., 2004). Population path parameters were chosen in accordance with those used in prior research on mediation models for the single mediator model (0, .14, .39, and .59) for each of the a, b, and c' paths (Fritz & MacKinnon, 2007; MacKinnon et al., 2002). This 4 × 4 × 4 × 6 factorial design resulted in 384 combinations of path parameters and sample size.The predicted variances and covariances were then used to calculate zero-order and first-order partial correlation effect sizes corresponding to the population a and b paths as found in MacKinnon (2008). The variances and covariances for the single mediator model were also used to calculate the true a and b coefficients and their true standard errors. Power was then calculated in two ways. First the correlations corresponding to a and b coefficients were used to calculate a z test, which was then used to compute analytical power. Then the path coefficients a and b and their true standard errors were used to calculate a t test, which was used to compute analytical power. The z and t tests yielded the same power values. Analytical formulas for power of the joint significance test of mediation for the single mediator model are also found in Wang and Xue (2012). For both methods of computing analytical power, the individual power values for a and b were multiplied to calculate analytical power, analogous to the joint significance test.ResultsSingle mediator model analytical resultsAnalytical power of the test of the total effect and the joint significance test for the mediated effect from the single mediator model where c' is zero or small are shown in Table 1 (for all cases where power of the test of the mediated effect was greater than power of the test of the total effect, the direct effect c' was always zero or small; when c' is equal to zero this indicated full mediation). Only power of the models is considered in this table (combinations where a or b is zero are measures of Type I error rate).Table 1Analytical Power of the Mediated and Total Effects for the Single Mediator Model at c′ = 0 and c′ = . 14N = 50 N = 100 N = 200 N = 500 N = 1000 N = 5000c ′ π c π ab π c π ab π c π ab π c π ab π c π ab π c π aba = .14, b = .14 0 .034 .025 .038 .078 .046 .249 .063 .766 .089 .986 .278 10.14 .189 .025 .341 .078 .598 .249 .939 .766 .999 .986 1 1a = .14, b = .39 0 .054 .117 .072 .269 .106 .499 .204 .875 .361 .993 .949 10.14 .235 .117 .427 .269 .716 .499 .980 .875 1 .993 1 1a = .14, b = .59 0 .070 .153 .104 .279 .168 .499 .354 .875 .612 .993 .999 10.14 .257 .153 .467 .279 .763 .499 .989 .875 1 .993 1 1a = .39, b = .14 0 .056 .117 .077 .269 .115 .499 .225 .875 .400 .993 .969 10.14 .259 .117 .471 .269 .767 .499 .990 .875 1 .993 1 1a = .39, b = .39 0 .161 .551 .285 .928 .509 .999 .883 1 .994 1 1 10.14 .454 .551 .754 .928 .965 .999 1 1 1 1 1 1a = .39, b = .59 0 .271 .720 .492 .963 .789 1 .992 1 1 1 1 10.14 .575 .720 .870 .963 .993 1 1 1 1 1 1 1a = .59, b = .14 0 .081 .153 .124 .279 .208 .499 .445 .875 .732 .993 1 10.14 .323 .153 .577 .279 .866 .499 .998 .875 1 .993 1 1a = .59, b = .39 0 .308 .720 .554 .963 .848 1 .997 1 1 1 1 10.14 .640 .720 .915 .963 .997 1 1 1 1 1 1 1a = .59, b = .59 0 .526 .941 .829 1 .986 1 1 1 1 1 1 10.14 .800 .941 .981 1 1 1 1 1 1 1 1 1Open in a separate windowNote. Analytical power of the total effect is represented here as 'πc', and analytical power calculated using regression coefficients and their standard errors is represented here as 'πab'.Combinations of parameters where the test of the mediated effect had more power than the test of the total effect are underlined. When a and b were small (a = b = .14), the test of the mediated effect had more power than the test of the total effect at larger sample sizes. The test of the total effect had more power than the test of the mediated effect at smaller sample sizes when a and b were small. For example, in Table 1 for the case where a and b were small (a = b = .14), the test of the total effect had more power than the test of the mediated effect for a sample size of 50. The test of the mediated effect had more power than the test of the total effect in every other sample size. However, when a and b were large (a = b = .59), the test of the mediated effect had more power than the test of the total effect at smaller sample sizes. For a = b = .59, the test of the mediated effect had more power than the test of the total effect in sample sizes up to 200. At sample sizes larger than 200, the test of the total effect and the joint significance and product of coefficients tests all had power of approximately one for large a and b. In summary, two patterns of results emerged for the single mediator model. The test of the mediated effect had more power than the test of the total effect when sample size was large and coefficients were small, and when sample size was small and coefficients were large. At large sample sizes with large coefficients, power of both tests approached one. Additionally, the test of the mediated effect had more power than the test of the total effect only in cases where c' was zero or small.The analytical power results were replicated in an empirical simulation study that is not reported here. Results from the empirical simulation are available at Research in Prevention Laboratory (MacKinnon).Comparison of standard errors of total and mediated effectsFor the majority of the combinations of parameters and sample sizes studied, the test of mediation was more powerful than the test of the total effect when c' was equal to zero, and therefore when the total and mediated effects were equal (that is, ab = c). For the test of the mediated effect to be more powerful than the test of the total effect, the t test of significance for ab must be larger than the t test of significance for c. Because the tests of significance are computed by dividing the effects by their standard errors, it follows that if the test of the mediated effect is more powerful than the test of the total effect, the standard error of the total effect must be larger than the standard error of the mediated effect.Table 2 shows a comparison of the analytical standard errors of c and ab for the single mediator model next to power of the tests of c and ab, for combinations where a and b are greater than zero and c' was equal to zero at N = 100 (a subsample of the combinations used in the results above).Table 2Comparison of Power and Standard Errors of ab and c for the Single Mediator Model Where c' = 0 and N = 100a b ab c sc sab π c π ab.14 .14 .020 .020 .102 .020 .038 .078.14 .39 .055 .055 .108 .042 .072 .269.14 .59 .083 .083 .117 .061 .104 .279.39 .14 .055 .055 .102 .042 .077 .269.39 .39 .152 .152 .108 .056 .285 .928.39 .59 .230 .230 .117 .072 .492 .963.59 .14 .083 .083 .102 .062 .124 .279.59 .39 .230 .230 .108 .072 .554 .963.59 .59 .348 .348 .117 .085 .829 1When ab was equal to c and c' was equal to zero, the standard error of c was larger than the standard error of ab. Furthermore, when the standard error of c was larger than the standard error of ab, power of the test of ab was larger than power of the test of c.A further study of situations where the test of the mediated effect had more power than the test of the total effect at smaller sample sizes and smaller coefficients showed that although the standard error of c was always larger than the standard error of ab when ab and c are equal, when a or b approached zero the power of the test of ab became smaller than power of the test of c. This pattern occurred for cases where a or b was very small but not zero. For example at N = 100, when a was equal to .14 and b was equal to .001, c was equal to .0014. The standard error of ab was .01422 and the standard error of c was .10102 so the standard error of ab was smaller than the standard error of c, but power of the test of ab was equal to .00714 and power of the test of c was equal to .02508. Although power of the test of c approached 0.025 for very small coefficients, power of the test of ab decreased to below 0.025.Type I error ratesFor all sample sizes, when a = b = 0 the test of joint significance had Type I error rates of .001. This result is a replication of previous literature on Type I error rates for tests of mediation (MacKinnon et al., 2002).DiscussionA comparison of analytical power of the mediated and total effects shows that the mediated effect has increased power with small parameter sizes and large sample size, and with large parameter sizes and small sample size. Furthermore, the conditions for when power of the test of the mediated effect will be greater than power of the test of the total effect are also found in the comparison of standard errors of c and ab, and therefore in terms of variances and covariances among variables. This means that given an expected covariance matrix among variables based on previous literature, it would be possible to use those variances and covariances to determine if the standard error of c will be larger than the standard error of ab. If it is and ab and c are expected to be equal, the test of mediation will have more power than the test of the total effect.Proximal Versus Distal Mediators: Effects on PowerWhen b is larger than a (that is, M is closer in time or more highly related to Y than to X), M may be considered a distal mediator. When a is larger than b (that is, M is closer in time or more highly related to X than to Y), M may be considered a proximal mediator. According to previous research, the test of ab will be more powerful for models with distal mediators than for models with proximal mediators due to collinearity between X and M (Hoyle & Kenny, 1999; Kenny & Judd, 2013). When collinearity between X and M is high, the standard error of the b path is increased, leading to a less powerful test of significance. Results from Fritz and Mackinnon (2007) support this point, demonstrating that required sample size is larger for conditions where a is larger than b.As the test of ab is more powerful when b is larger than a, it follows that when comparing power of the test of ab to power of the test of c, the gain in power over the test of c would be greater in conditions where b is larger than a. This gain in power is seen in the results from this study. For example, in Table 1 at N = 200, a = .39, b = .59, c' = 0, adding a mediator increased power to detect effects by .211, and at N = 200, a = .59, b = .39, c' = 0, adding a mediator increased power to detect effects by 0.152. The increase in power to detect effects was larger for the condition where b was larger than a.Go to:Study 2Study 1 demonstrated that power to detect the mediated effect was greater than power to detect the total effect for the single mediator model. Study 2 aims to demonstrate that having two mediators also increases statistical power to detect effects, and identifies when the test of the total mediated effect a1b1 + a2b2 will have more power than the test of the total effect c for the parallel two mediator model.MethodDetermination of path parameters for the parallel two mediator modelThe parallel two mediator model covariance matrix is shown in Table 3. Covariance algebra was used to derive formulas for the variance of each variable in the parallel two mediator model, and the covariances between each pair of variables.Table 3Covariance Matrix for the Parallel Two Mediator ModelAn external file that holds a picture, illustration, etc.Object name is nihms-602858-t0001.jpgThe variances and covariances were then used to derive full and partial correlation effect sizes for the parallel two mediator model where ρ is the correlation. Formulas for the effect sizes are as follows:ρXM1=σXM1σ2X−−−√σ2M1−−−√(11)ρM1Y.XM2=ρM1Y.X−ρM1M2.XρM2Y.X1−ρ2M1M2.X−−−−−−−−−√1−ρ2M2Y.X−−−−−−−−√(12)ρXM2=σXM2σ2X−−−√σ2M2−−−√(13)ρM2Y.XM1=ρM2Y.X−ρM1M2.XρM1Y.X1−ρ2M1M2.X−−−−−−−−−√1−ρ2M1Y.X−−−−−−−−√(14)ρXY.M1M2=ρXY.M1−ρXM2.M1ρYM2.M11−ρ2XM2.M1−−−−−−−−−√1−ρ2YM2.M1−−−−−−−−−√(15)Where Equation 11 is the zero-order correlation effect size for a1, Equation 12 is the second-order partial correlation effect size for b1, Equation 13 is the zero-order correlation effect size for a2, Equation 14 is the second-order partial correlation effect size for b2, and Equation 15 is the second-order partial correlation effect size for c'. Appendix B shows how the effect size formulas were produced from the variances and covariances between variables.Regression coefficients for this model were derived based on the above formulas that correspond approximately to Cohen's (1988) small, medium, and large correlation effect sizes. Parameters were identified based on the correlation results that would be as close to Cohen's guidelines for small, medium, and large effect sizes (.1, .3, and .5) as possible. It was determined that for a1, b1, a2, and b2, path coefficients of .101, .314, and .577 corresponded to small, medium, and large effects. For c', path coefficients of .131, .400, and .740 corresponded to small, medium, and large effects. These path coefficients were necessary for generating data to calculate empirical power for the parallel two mediator model.Empirical power for the parallel two mediator modelEmpirical power was calculated by generating data with a known mediated effect and computing the proportion of times the test for mediation was statistically significant. Sample sizes chosen for the parallel two mediator model were 50, 100, 200, 500, and 1000. Parameter values (described above) were 0, .101, .314, and .577 for the a1, a2, b1, and b2 coefficients, and 0, .131, .400, and .577 for the c' coefficient. This 5 × 45 design resulted in 5120 combinations of parameters and sample size, and 500 datasets were simulated for each combination. All variables were simulated to be continuous for both models, and all tests of significance were two-tailed. The product of coefficients test was used to assess significance for the parallel two mediator model. In this study, power was assessed for testing two null hypotheses: H0: c = 0 for the test of the total effect and H0: a1b1 + a2b2 = 0 for the product of coefficients test of significance. For each combination of parameters and sample size, the proportion of times out of that the test was significant out of 500 generated datasets was the empirical power for combinations with non-zero path parameters (the Type I error rate for combinations with path parameters equal to zero).BootstrapBootstrap estimation was used to calculate power for the total mediated effect because there is not a joint significance test for the parallel two mediator model, and the z test using the multivariate delta method standard error for the total mediated effect is known to be inaccurate. Bootstrap estimation was conducted for 10 combinations of parameters and sample size to compare to power of the total effect and total mediated effect. The combinations were randomly selected from the combinations where the total mediated effect was more powerful than the total effect based on the empirical power results. One sample of data was simulated for X, M1, M2, and Y based on the mediation equations, and then bootstrap estimation was conducted for that single dataset by sampling with replacement from that sample of data to get N observations, forming the first bootstrap sample. In each bootstrap sample, the values of a1, b1, a2, b2, and c were generated and saved. This process of randomly sampling with replacement and saving values of the coefficients was repeated 1000 times to create 1000 bootstrap samples for the first simulated data set, and the saved bootstrapped values of a1, b1, a2, b2, and c were used to create confidence intervals for the total mediated effect a1b1 + a2b2 and the total effect c. This process of simulating a dataset and generating a bootstrap confidence interval was repeated 1000 times, simulating 1000 datasets. The means of the number of times the confidence intervals did not include zero from the 1000 simulated datasets were the bootstrapped power values of the total and total mediated effects.ResultsParallel two mediator model empirical resultsTable 4 contains empirical power values for both the product of coefficients test of the total mediated effect for the parallel two mediator model and the test of the total effect where c' = 0 or .131 (corresponding to zero or small effect sizes for c', which were the only values of c' at which the test of the total mediated effect was found to have more power than the test of the total effect). To reduce the amount of information presented, only combinations where a1 = b1 and a2 = b2 are included. Combinations of parameters and sample size where the test of the total mediated effect was more powerful than the test of the total effect are underlined, and combinations of parameters and sample size where the test of the total mediated effect exceeded .80 when the test of the total effect did not are underlined and italicized.
- Home >
- Catalog >
- Legal >
- Affidavit Form >
- Non Collusion Affidavit >
- how to fill out a non collusion affidavit >
- Sc 990 Filing Requirements