How to Edit The Dl1 conviniently Online
Start on editing, signing and sharing your Dl1 online with the help of these easy steps:
- Push the Get Form or Get Form Now button on the current page to jump to the PDF editor.
- Wait for a moment before the Dl1 is loaded
- Use the tools in the top toolbar to edit the file, and the added content will be saved automatically
- Download your completed file.
The best-rated Tool to Edit and Sign the Dl1
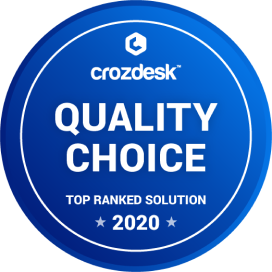
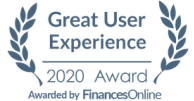
A quick direction on editing Dl1 Online
It has become very simple in recent times to edit your PDF files online, and CocoDoc is the best PDF editor for you to make a lot of changes to your file and save it. Follow our simple tutorial to start!
- Click the Get Form or Get Form Now button on the current page to start modifying your PDF
- Add, change or delete your text using the editing tools on the tool pane above.
- Affter altering your content, add the date and draw a signature to make a perfect completion.
- Go over it agian your form before you click on the button to download it
How to add a signature on your Dl1
Though most people are adapted to signing paper documents by handwriting, electronic signatures are becoming more regular, follow these steps to sign PDF online!
- Click the Get Form or Get Form Now button to begin editing on Dl1 in CocoDoc PDF editor.
- Click on the Sign tool in the tool box on the top
- A window will pop up, click Add new signature button and you'll have three ways—Type, Draw, and Upload. Once you're done, click the Save button.
- Drag, resize and settle the signature inside your PDF file
How to add a textbox on your Dl1
If you have the need to add a text box on your PDF for making your special content, do some easy steps to complete it.
- Open the PDF file in CocoDoc PDF editor.
- Click Text Box on the top toolbar and move your mouse to position it wherever you want to put it.
- Write in the text you need to insert. After you’ve put in the text, you can utilize the text editing tools to resize, color or bold the text.
- When you're done, click OK to save it. If you’re not happy with the text, click on the trash can icon to delete it and start over.
A quick guide to Edit Your Dl1 on G Suite
If you are looking about for a solution for PDF editing on G suite, CocoDoc PDF editor is a recommended tool that can be used directly from Google Drive to create or edit files.
- Find CocoDoc PDF editor and establish the add-on for google drive.
- Right-click on a PDF document in your Google Drive and click Open With.
- Select CocoDoc PDF on the popup list to open your file with and allow access to your google account for CocoDoc.
- Modify PDF documents, adding text, images, editing existing text, mark up in highlight, polish the text up in CocoDoc PDF editor before pushing the Download button.
PDF Editor FAQ
In multilayer neural networks, are weights in hidden layers closer to the input updated more strongly than weights in layers closer to the output?
Typically, the learning rate is a single global variable, meaning all weights are updated the same. However, you've touched on an interesting challenge in neural nets - the vanishing gradient problem. If you're curious about that too, keep reading:Consider this four-layer network. We update weights by backpropagating the gradient from the output layer to the input layer.The gradient of the output with respect to the input is:[math]d(out)/d(in) = d(out)/dL3 * dL3/dL2 * dL2/dL1 * dL1/d(in)[/math]where each derivative is represented by a Jacobian matrix. If the partial derivatives are between -1 and +1, you end up getting a vanishingly small gradient for the first input layers. For example (using scalars for simplicity), if all of our partial derivatives are 0.1, we will find:[math]d(out)/dL3 = 0.1[/math][math]d(out)/dL2 = 0.1 * 0.1 = 0.01[/math][math]d(out)/dL1 = 0.1 * 0.1 * 0.1 = 0.001[/math][math]d(out)/d(in) = 0.1 * 0.1 * 0.1 * 0.1 = 0.0001[/math]So what is the consequence of this? If you train this network and plot how quickly the three layers learn with time (btw, I'm borrowing these images from here - Neural networks and deep learning), you get something like this:This is a problem! Your first layer is the one with access to the most raw data. If it's not learning quickly, a lot of the information from your input may get lost before it even reaches layer 2. If that happens, it doesn't matter how fast the later layers are learning, because the information they need has been lost in transmission.So why don't we just compensate by scaling up the learning rate for earlier layers? Because the magnitude of the gradient is sometimes greater than 1 too. This could mean that instead of 0.1, 0.01, 0.001, and 0.0001, your gradients exhibit the opposite problem: they are now 10, 100, 1000, 10000. This is called the exploding gradient problem. And together, the two problems form a catch-22.So what can be done? Currently, the most popular solution is to use a neuron activation function that keeps the magnitudes of the partial derivatives near 1.Here is one well-known activation function, called the sigmoid function:And here are two more, called ReLU and PReLU:Sigmoid was popular for a while, but it turns out that saturating at +/- 1 tends to produce a lot of tiny gradients, which got us the vanishing gradient problem. People often don't use sigmoid anymore.ReLU is a super popular choice today. For positive activations, you get a partial derivative of 1, so it keeps gradients from either exploding or vanishing. It's also really easy to implement computationally.A possible downside for ReLU is that for negative activations, the gradient disappears entirely. This has the result that, once a neuron's input falls below 0, the neuron turns off and never activates again. This can be inefficient, because you end up with a network that takes up a lot of space in memory, but whose connections are effectively sparse.One possible alternative is PReLU, where the slope below 0 is some fraction of the slope above 0. This introduces additional complexity, and more parameters which need to be learned, but it's an option that people are exploring right now.If you're curious where to start, I'd suggest using ReLU neurons and a single global learning rate (usually between 1e-2 and 1e-4 depending on how you set up your problem). From here, you can tweak things to suit your needs.Be sure to keep up on new research - these could always change! ;-)Links:Great explainer on vanishing/exploding gradients (also, where I got my best pictures): Neural networks and deep learningThe images of the activation functions came from:Sigmoid functionBenchmarking ReLU and PReLU using MNIST and Theano
How can I draw an economic diagram for seasonal unemployment?
Consider this diagram:Source: S-cool, the revision website (<< oh and you might want to read that)We start out with an equilibrium between Supply (SL) and Demand (DL1) at the point (L1, W1), i.e. L1 people are employed at real wage W1.Consider a tropical island where most labor is in the tourism sector. During winter there are less tourists and so labor demand drops. The demand for labor moves from DL1 to DL2 at a new wage W2. So the new equilibrium is (L3, W2). The seasonal unemployment is then L1-L3 persons.However, this assumes that the real wage adjusts immediately. But wages are "sticky", i.e. they take some to adjust due to, for instance, contracts which have been negotiated and set for a certain period of time. So if the wage doesn't move when demand drops from DL1 to DL2, the new equilibrium will be at (L2, W1) and the added unemployed number L1-L2.
What would be the change in elongation if a prismatic bar under axial load at two end is drilled half of its length from one side?
Treat the bar as two prismatic bars of equal length and calculate the deformations of each bar using the formula dL = PL/AE. Let L = the total length of the bar so the length of one section is L/2. If Aa is the cross sectional area of the drilled portion then its deformation dL1 = PL/(2AaE) and the deformation of the undrilled portion is dL2 = PL/(2AbE) where Ab is the cross sectional area of the undrilled bar and E is the modulus of elasticity of the bar material. The total deformation is dL1 + dL2