The Guide of finishing Mgh Dermatology New Patient Online
If you are looking about Modify and create a Mgh Dermatology New Patient, here are the step-by-step guide you need to follow:
- Hit the "Get Form" Button on this page.
- Wait in a petient way for the upload of your Mgh Dermatology New Patient.
- You can erase, text, sign or highlight through your choice.
- Click "Download" to keep the forms.
A Revolutionary Tool to Edit and Create Mgh Dermatology New Patient
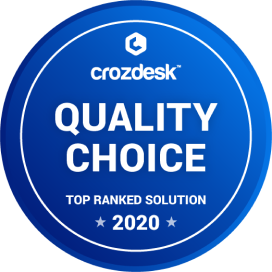
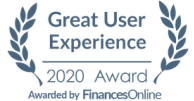
How to Easily Edit Mgh Dermatology New Patient Online
CocoDoc has made it easier for people to Fill their important documents by online website. They can easily Tailorize through their choices. To know the process of editing PDF document or application across the online platform, you need to follow this stey-by-step guide:
- Open CocoDoc's website on their device's browser.
- Hit "Edit PDF Online" button and Upload the PDF file from the device without even logging in through an account.
- Edit your PDF forms online by using this toolbar.
- Once done, they can save the document from the platform.
Once the document is edited using online browser, the user can export the form according to your ideas. CocoDoc provides a highly secure network environment for consummating the PDF documents.
How to Edit and Download Mgh Dermatology New Patient on Windows
Windows users are very common throughout the world. They have met a lot of applications that have offered them services in managing PDF documents. However, they have always missed an important feature within these applications. CocoDoc are willing to offer Windows users the ultimate experience of editing their documents across their online interface.
The way of editing a PDF document with CocoDoc is simple. You need to follow these steps.
- Pick and Install CocoDoc from your Windows Store.
- Open the software to Select the PDF file from your Windows device and continue editing the document.
- Fill the PDF file with the appropriate toolkit showed at CocoDoc.
- Over completion, Hit "Download" to conserve the changes.
A Guide of Editing Mgh Dermatology New Patient on Mac
CocoDoc has brought an impressive solution for people who own a Mac. It has allowed them to have their documents edited quickly. Mac users can make a PDF fillable online for free with the help of the online platform provided by CocoDoc.
To understand the process of editing a form with CocoDoc, you should look across the steps presented as follows:
- Install CocoDoc on you Mac in the beginning.
- Once the tool is opened, the user can upload their PDF file from the Mac with ease.
- Drag and Drop the file, or choose file by mouse-clicking "Choose File" button and start editing.
- save the file on your device.
Mac users can export their resulting files in various ways. Downloading across devices and adding to cloud storage are all allowed, and they can even share with others through email. They are provided with the opportunity of editting file through various ways without downloading any tool within their device.
A Guide of Editing Mgh Dermatology New Patient on G Suite
Google Workplace is a powerful platform that has connected officials of a single workplace in a unique manner. While allowing users to share file across the platform, they are interconnected in covering all major tasks that can be carried out within a physical workplace.
follow the steps to eidt Mgh Dermatology New Patient on G Suite
- move toward Google Workspace Marketplace and Install CocoDoc add-on.
- Attach the file and Push "Open with" in Google Drive.
- Moving forward to edit the document with the CocoDoc present in the PDF editing window.
- When the file is edited ultimately, save it through the platform.
PDF Editor FAQ
How can deep neural networks be applied to healthcare?
Throughout the course of comprehensive healthcare, many patients develop problems with their minds and bodies that can lead to severe discomfort, costly treatment, disabilities, and more. Predicting those escalations in advance offers healthcare providers the opportunity to apply preventative measure that might improve patient safety, and quality of care, while lowering medical costs. In simple terms, prediction using networks of big data used to evaluate specific people, and specific risk factors in certain illnesses could save lives, and avoid medical complications.Today, many prognostics methods turn to Artificial Neural Networks when attempting to find new insights into the future of patient healthcare. ANNs (Artificial Neural Networks) are just one of the many models being introduced into the field of healthcare by innovations like AI and big data. Their purpose is to transform huge amounts of raw data into useful decisions for treatment and care.What is a Neural Network?Understanding Neural Networks can be very difficult. After all, to many people, these examples of Artificial Intelligence in the medical industry are a futuristic concept.According to Wikipedia (the source of all truth) :“Neural Networks are a computational approach which is based on a large collection of neural units loosely modeling the way the brain solves problems with large clusters of biological neurons connected by axons. Each neural unit is connected with many others…These systems are self-learning and trained rather than explicitly programmed…”By Glosser.ca – Own work, Derivative of File:Artificial neural network.svg (https://commons.wikimedia.org/wiki/File:Artificial_neural_network.svg), CC BY-SA 3.0, LinkOne way to think of it is this: Imagine that a doctor wants to make a prediction regarding a patient’s health – for instance, whether she or he is at risk of suffering from a certain disease. How would a doctor be able to ascertain that information? In most cases, it would involve using blood tests, taking tests of the patient’s vitals, and more to identify features that have proven to be good predictors of patient health. However, what if doctors only know a handful of risk-factors for a specific disease – or worse, they don’t know the risk factors at all? It would be impossible to make predictions.ANNs help to provide the predictions in healthcare that doctors and surgeons simply couldn’t address alone. They work in moments wherein we can collect data, but we don’t understand which pieces of that data are vitally important yet. These abstractions can therefore capture complex relationships that might not be initially obvious – leading to better prediction for public health.What are the Possibilities for Neural Networks in Healthcare?Though they may seem like a futuristic concept, ANNs have been used in healthcare for several decades. In fact, the book “Neural Networks in Healthcare” covers the various uses of this system prior to 2006. Before 2006, the main successes of ANNs were found in areas like speech processing and image processing. Today, as new technologies emerge, capable of changing the way that we approach neural networks in the first place – it’s worth noting that there may be numerous new options for changing the industry. Today, the possibilities for Neural Networks in Healthcare include:Diagnostic systems – ANNs can be used to detect heart and cancer problems, as well as various other diseases informed by big data.Biochemical analysis – ANNs are used to analyze urine and blood samples, as well as tracking glucose levels in diabetics, determining ion levels in fluids, and detecting various pathological conditions.Image analysis – ANNs are frequently used to analyze medical images from various areas of healthcare, including tumor detection, x-ray classifications, and MRIs.Drug development – Finally, ANNs are used in the development of drugs for various conditions – working by using large amounts of data to come to conclusions about treatment options.Current Examples of Neural NetworksNeural networks can be seen in most places where AI has made steps within the healthcare industry. For instance, in the world of drug discovery, Data Collective and Khosla Ventures are currently backing the company “Atomwise“, which uses the power of machine learning and neural networks to help medical professionals discover safer and more effective medicines fast. The company recently published its first findings of Ebola treatment drugs last year, and the tools that Atomwise uses can tell the difference between toxic drug candidates and safer options.Similarly, options are being found that could insert neural networks into the realm of diagnostic. For instance, in 2014, Butterfly Networks, which are transforming the diagnostic realm with deep learning, devices, and the cloud, raised $100M for their cause. This organization currently works at the heart of the medicine and engineering sectors by bringing together world-class skills in everything from electrical engineering, to mechanical engineering, and medicine. At the same time, iCarbonX are developing artificial intelligence platforms to facilitate research relating to the treatment of various diseases and preventative care. The company believe that soon they will be able to help enable the future of truly personalized medicine.The Future of Healthcare…Perhaps the most significant problem with ANNs is that the learned features involved when it comes to assessing huge amounts of data can sometimes be difficult to interpret. This is potentially why ANNs are more commonly used during situations wherein we have a lot of data to ensure that the observed data doesn’t contain too many “flukes”. Think of it this way – if you toss a coin three times and receive “tails” every time, this doesn’t mean that a coin only has a “tails” side. It just means that you need further evaluation and more testing to get a proper reading of probability.ANNs are going to need some tweaking if they’re going to become the change that the healthcare industry needs. However, alongside new AI developments, it seems that neural networks could have a very important part to play in the future of healthcare.Healthcare organizations of all sizes, types, and specialties are becoming increasingly interested in how artificial intelligence can support better patient care while reducing costs and improving efficiencies.Over a relatively short period of time, the availability and sophistication of AI has exploded, leaving providers, payers, and other stakeholders with a dizzying array of tools, technologies, and strategies to choose from.Just learning the lingo has been a top challenge for many organizations.There are subtle but significant differences between key terms such as AI, machine learning, deep learning, and semantic computing.Understanding exactly how data is ingested, analyzed, and returned to the end user can have a big impact on expectations for accuracy and reliability, not to mention influencing any investments necessary to whip an organization’s data assets into shape.In order to efficiently and effectively choose between vendor products or hire the right data science staff to develop algorithms in-house, healthcare organizations should feel confident that they have a firm grasp on the different flavors of artificial intelligence and how they can apply to specific use cases.Deep learning is a good place to start. This branch of artificial intelligence has very quickly become transformative for healthcare, offering the ability to analyze data with a speed and precision never seen before.But what exactly is deep learning, how does it differ from other machine learning strategies, and how can healthcare organizations leverage deep learning techniques to solve some of the most pressing problems in patient care?DEEP LEARNING IN A NUTSHELLDeep learning, also known as hierarchical learning or deep structured learning, is a type of machine learning that uses a layered algorithmic architecture to analyze data.In deep learning models, data is filtered through a cascade of multiple layers, with each successive layer using the output from the previous one to inform its results. Deep learning models can become more and more accurate as they process more data, essentially learning from previous results to refine their ability to make correlations and connections.Deep learning is loosely based on the way biological neurons connect with one another to process information in the brains of animals. Similar to the way electrical signals travel across the cells of living creates, each subsequent layer of nodes is activated when it receives stimuli from its neighboring neurons.In artificial neural networks (ANNs), the basis for deep learning models, each layer may be assigned a specific portion of a transformation task, and data might traverse the layers multiple times to refine and optimize the ultimate output.These “hidden” layers serve to perform the mathematical translation tasks that turn raw input into meaningful output.An illustration of a deep learning neural networkSource: University of Cincinnati“Deep learning methods are representation-learning methods with multiple levels of representation, obtained by composing simple but non-linear modules that each transform the representation at one level (starting with the raw input) into a representation at a higher, slightly more abstract level,” explains a 2015 article published in Nature, authored by engineers from Facebook, Google, the University of Toronto, and Université de Montréal.“With the composition of enough such transformations, very complex functions can be learned. Higher layers of representation amplify aspects of the input that are important for discrimination and suppress irrelevant variations.”This multi-layered strategy allows deep learning models to complete classification tasks such as identifying subtle abnormalities in medical images, clustering patients with similar characteristics into risk-based cohorts, or highlight relationships between symptoms and outcomes within vast quantities of unstructured data.Unlike other types of machine learning, deep learning has the added benefit of being able to decisions with significantly less involvement from human trainers.While basic machine learning requires a programmer to identify whether a conclusion is correct or not, deep learning can gauge the accuracy of its answers on its own due to the nature of its multi-layered structure.“With the composition of enough such transformations, very complex functions can be learned.”Deep learning also requires less preprocessing of data. The network itself takes care of many of the filtering and normalization tasks that must be completed by human programmers when using other machine learning techniques.“Conventional machine-learning techniques are limited in their ability to process natural data in their raw form,” said the article from Nature.“For decades, constructing a pattern-recognition or machine-learning system required careful engineering and considerable domain expertise to design a feature extractor that transformed the raw data (such as the pixel values of an image) into a suitable internal representation or feature vector from which the learning subsystem, often a classifier, could detect or classify patterns in the input.”Deep learning networks, however, “automatically discover the representations needed for detection or classification,” reducing the need for supervision and speeding up the process of extracting actionable insights from datasets that have not been as extensively curated.Naturally, the mathematics involved in developing deep learning models are extraordinarily intricate, and there are many different variations of networks that leverage different sub-strategies within the field.The science of deep learning is evolving very quickly to power some of the most advanced computing capabilities in the world, spanning every industry and adding significant value to user experiences and competitive decision-making.WHAT ARE THE USE CASES FOR DEEP LEARNING IN HEALTHCARE?Many of the industry’s deep learning headlines are currently related to small-scale pilots or research projects in their pre-commercialized phases.However, deep learning is steadily finding its way into innovative tools that have high-value applications in the real-world clinical environment.Some of the most promising use cases include innovative patient-facing applications as well as a few surprisingly established strategies for improving the health IT user experience.Imaging analytics and diagnosticsOne type of deep learning, known as convolutional neural networks (CNNs), is particularly well-suited to analyzing images, such as MRI results or x-rays.CNNs are designed with the assumption that they will be processing images, according tocomputer science experts at Stanford University, allowing the networks to operate more efficiently and handle larger images.As a result, some CNNs are approaching – or even surpassing – the accuracy of human diagnosticians when identifying important features in diagnostic imaging studies.In June of 2018, a study in the Annals of Oncology showed that a convolutional neural network trained to analyze dermatology images identified melanoma with ten percent more specificity than human clinicians.Even when human clinicians were equipped with background information on patients, such as age, sex, and the body site of the suspect feature, the CNN outperformed the dermatologists by nearly 7 percent.“Our data clearly show that a CNN algorithm may be a suitable tool to aid physicians in melanoma detection irrespective of their individual level of experience and training,” said the team of researchers from a number of German academic institutions.In addition to being highly accurate, deep learning tools are fast.Researchers at the Mount Sinai Icahn School of Medicine have developed a deep neural network capable of diagnosing crucial neurological conditions, such as stroke and brain hemorrhage, 150 times faster than human radiologists.“Our data clearly show that a CNN algorithm may be a suitable tool to aid physicians in melanoma detection irrespective of their individual level of experience and training.”The tool took just 1.2 seconds to process the image, analyze its contents, and alert providers of a problematic clinical finding.“The expression ‘time is brain’ signifies that rapid response is critical in the treatment of acute neurological illnesses, so any tools that decrease time to diagnosis may lead to improved patient outcomes,” said Joshua Bederson, MD, Professor and System Chair for the Department of Neurosurgery at Mount Sinai Health System and Clinical Director of the Neurosurgery Simulation Core.Deep learning is so adept at image work that some AI scientists are using neural networks to create medical images, not just read them.A team from NVIDIA, the Mayo Clinic, and the MGH & BWH Center for Clinical Data Science has developed a method of using generative adversarial networks (GANs), another type of deep learning, which can create stunningly realistic medical images from scratch.The images use patterns learned from real scans to create synthetic versions of CT or MRI images. The data can be randomly generated and endlessly diverse, allowing researchers to access large volumes of necessary data without any concerns around patient privacy or consent.These simulated images are so accurate that they can help train future deep learning models to diagnose clinical findings.“Medical imaging data sets are often imbalanced as pathologic findings are generally rare, which introduces significant challenges when training deep learning models,” said the team. “We propose a method to generate synthetic abnormal MRI images with brain tumors by training a generative adversarial network.”“This offers an automatable, low-cost source of diverse data that can be used to supplement the training set. For example, we can alter a tumor’s size, change its location, or place a tumor in an otherwise healthy brain, to systematically have the image and the corresponding annotation.”Such a strategy could significantly reduce of AI’s biggest sticking points: a lack of reliable, sharable, high-volume datasets to use for training and validating machine learning models.Natural language processingDeep learning and neural networks already underpin many of the natural language processing tools that have become popular in the healthcare industry for dictating documentation and translating speech-to-text.Because neural networks are designed for classification, they can identify individual linguistic or grammatical elements by “grouping” similar words together and mapping them in relation to one another.This helps the network understand complex semantic meaning. But the task is complicated by the nuances of common speech and communication. For example, words that always appear next to each other in an idiomatic phrase, may end up meaning something very different than if those same words appeared in another context (think “kick the bucket” or “barking up the wrong tree”).While acceptably accurate speech-to-text has become a relatively common competency for dictation tools, generating reliable and actionable insights from free-text medical data is significantly more challenging.Unlike images, which consist of defined rows and columns of pixels, the free text clinical notes in electronic health records (EHRs) are notoriously messy, incomplete, inconsistent, full of cryptic abbreviations, and loaded with jargon.Currently, most deep learning tools still struggle with the task of identifying important clinical elements, establishing meaningful relationships between them, and translating those relationships into some sort of actionable information for an end user.A recent literature review from JAMIA found that while deep learning surpasses other machine learning methods for processing unstructured text, several significant challenges, including the quality of EHR data, are holding these tools back from true success.“Researchers have confirmed that finding patterns among multimodal data can increase the accuracy of diagnosis, prediction, and overall performance of the learning system. However, multimodal learning is challenging due to the heterogeneity of the data,” the authors observed.Accessing enough high-quality data to train models accurately is also problematic, the article continued. Data that is biased or skewed towards particular age groups, ethnicities, or other characteristics could create models that are not equipped to accurately assess a broad variety of real-life patients.Still, deep learning represents the most promising pathway forward into trustworthy free-text analytics, and a handful of pioneering developers are finding ways to break through the existing barriers.A team from Google, UC San Francisco, Stanford Medicine, and the University of Chicago Medicine, for example, developed a deep learning and natural language processing algorithm that analyzed more than 46 billion data points from more than 216,000 EHRs across two hospitals.The tool was able to improve on the accuracy of traditional approaches for identifying unexpected hospital readmissions, predicting length of stay, and forecasting inpatient mortality.“This predictive performance was achieved without hand-selection of variables deemed important by an expert, similar to other applications of deep learning to EHR data,” the researchers said.“Instead, our model had access to tens of thousands of predictors for each patient, including free-text notes, and identified which data were important for a particular prediction.”While the project is only a proof-of-concept study, Google researchers said, the findings could have dramatic implications for hospitals and health systems looking to reduce negative outcomes and become more proactive about delivering critical care.Drug discovery and precision medicinePrecision medicine and drug discovery are also on the agenda for deep learning developers. Both tasks require processing truly enormous volumes of genomic, clinical, and population-level data with the goal of identifying hitherto unknown associations between genes, pharmaceuticals, and physical environments.Deep learning is an ideal strategy for researchers and pharmaceutical stakeholders looking to highlight new patterns in these relatively unexplored data sets – especially because many precision medicine researchers don’t yet know exactly what they should be looking for.“Our model had access to tens of thousands of predictors for each patient, including free-text notes, and identified which data were important for a particular prediction.”The world of genetic medicine is so new that unexpected discoveries are commonplace, creating an exciting proving ground for innovative approaches to targeted care.The National Cancer Institute and the Department of Energy are embracing this spirit of exploration through a number of joint projects focused on leveraging machine learning for cancer discoveries.The combination of predictive analytics and molecular modeling will hopefully uncover new insights into how and why certain cancers form in certain patients.Deep learning technologies will accelerate the process of analyzing data, the two agencies said, shrinking the processing time for key components from weeks or months to just a few hours.The private sector is similarly committed to illustrating how powerful deep learning can be for precision medicine.A partnership by GE Healthcare and Roche Diagnostics, announced in January of 2018, will focus on using deep learning and other machine learning strategies to synthesize disparate data sets critical to developing precision medicine insights.The two companies will work to combine in-vivo and in-vitro data, EHR data, clinical guidelines, and real-time monitoring data to support clinical decision-making and the creation of more effective, less invasive therapeutic pathways.“By leveraging this combined data set using machine learning and deep learning, it may be possible in the future to reduce the number of unnecessary biopsies that are performed due to suspicious findings in the mammograms and possibly also reduce mastectomies that are performed to combat ductal carcinoma in situ, a condition that may evolve into invasive breast cancer in some cases,” said Nadeem Ishaque, Chief Innovation Officer, GE Healthcare Imaging.A separate study, conducted by researchers from the University of Massachusetts and published in JMIR Medical Informatics, found that deep learning could also identify adverse drug events (ADEs) with much greater accuracy than traditional models.“By leveraging this combined data set using machine learning and deep learning, it may be possible in the future to reduce the number of unnecessary biopsies.”The tool combines deep learning with natural language processing to comb through unstructured EHR data, highlighting worrisome associations between the type, frequency, and dosage of medications. The results could be used for monitoring the safety of novel therapies or understanding how new pharmaceuticals are being prescribed in the real-world clinical environment.Clinical decision support and predictive analyticsIn a similar vein, the industry has high hopes for the role of deep learning in clinical decision support and predictive analytics for a wide variety of conditions.Deep learning may soon be a handy diagnostic companion in the inpatient setting, where it can alert providers to changes in high-risk conditions such as sepsis and respiratory failure.Researchers from the MIT Computer Science and Artificial Intelligence Laboratory (CSAIL) have created a project called ICU Intervene, which leverages deep learning to alert clinicians to patient downturns in the critical care unit.“Much of the previous work in clinical decision-making has focused on outcomes such as mortality (likelihood of death), while this work predicts actionable treatments,” said PhD student and lead author Harini Suresh. “In addition, the system is able to use a single model to predict many outcomes.”The tool offers human clinicians a detailed rationale for its recommendations, helping to foster trust and allowing providers to have confidence in their own decision-making when potentially overruling the algorithm.Google is also on the leading edge of clinical decision support, this time for eye diseases. The company’s UK-based subsidiary, DeepMind, is working to develop a commercialized deep learning CDS tool that can identify more than 50 different eye diseases – and provide treatment recommendations for each one.In a supporting study published in Nature, DeepMind and Moorfields Eye Hospital found that the tool is just as accurate as a human clinician, and has the potential to significantly expand access to care by reducing the time it takes for an exam and diagnosis.“Currently, eye care professionals use optical coherence tomography (OCT) scans to help diagnose eye conditions. These 3D images provide a detailed map of the back of the eye, but they are hard to read and need expert analysis to interpret,” explained DeepMind.“The time it takes to analyze these scans, combined with the sheer number of scans that healthcare professionals have to go through (over 1,000 a day at Moorfields alone), can lead to lengthy delays between scan and treatment – even when someone needs urgent care. If they develop a sudden problem, such as a bleed at the back of the eye, these delays could even cost patients their sight.”With deep learning, the triage process is nearly instantaneous, the company asserted, and patients do not have to sacrifice quality of care.“This is a hugely exciting milestone, and another indication of what is possible when clinicians and technologists work together,” DeepMind said.WHAT IS THE FUTURE OF DEEP LEARNING IN HEALTHCARE?As intriguing as these pilots and projects can be, they represent only the very beginning of deep learning’s role in healthcare analytics.Excitement and interest about deep learning are everywhere, capturing the imaginations of regulators and rule makers, private companies, care providers, and even patients.The Office of the National Coordinator (ONC) is one organization with particularly high hopes for deep learning, and it is already applauding some developers for achieving remarkable results.In a recent report on the state of AI in the healthcare setting, the agency noted that some deep learning algorithms have already produced “transformational” outcomes.“There have been significant demonstrations of the potential utility of artificial Intelligence approaches based on deep learning for use in medical diagnostics,” the report said.“Where good training sets represent the highest levels of medical expertise, applications of deep learning algorithms in clinical settings provide the potential of consistently delivering high quality results.”The report highlighted early successes in diabetic retinal screenings and the classification of skin cancer as two areas where deep learning may already be changing the status quo.On the clinical side, imaging analytics is likely to be the focal point for the near future, due to the fact that deep learning already has a head start on many high-value applications.“Applications of deep learning algorithms in clinical settings provide the potential of consistently delivering high quality results.”But purely clinical applications are only one small part of how deep learning is preparing to change the way the healthcare system functions.The strategy is integral to many consumer-facing technologies, such as chatbots, mHealth apps, and virtual personalities like Alexa, Siri, and Google Assistant.These tools have the potential to radically alter the way patients interact with the healthcare system, offering home-based chronic disease management programming, 24/7 access to basic triage, and new ways to complete administrative tasks.By 2019, up to 40 percent of businesses are planning to integrate one or more of these popular consumer technologies into their internal or external workflows.Customer support and communication are two early implementations. But with market-movers like Amazon rumored to start rolling out more consumer-facing health options to patients, it may only be a matter of time before chatting with Alexa becomes as common as shooting the breeze with a medical assistant.Voice recognition and other analytics based on deep learning also have the near-term potential to provide some relief to physicians and nurses struggling with their EHRs.Google appears particularly interested in capturing medical conversations in the clinic and using deep learning to reduce administrative burdens on providers.One recent research paper illustrated the potential to use deep learning and NLP to understand casual conversation in a noisy environment, giving rise to the possibility of using an ambient, intelligent scribe to shoulder the onus of documentation.“We wondered: could the voice recognition technologies already available in Google Assistant, Google Home, and Google Translate be used to document patient-doctor conversations and help doctors and scribes summarize notes more quickly?” a Google team posited.“While most of the current automatic speech recognition (ASR) solutions in medical domain focus on transcribing doctor dictations (i.e., single speaker speech consisting of predictable medical terminology), our research shows that it is possible to build an ASR model which can handle multiple speaker conversations covering everything from weather to complex medical diagnosis,” the blog post says.Google will work with physicians and data scientists at Stanford to refine the technology and understand how it can be best applied to the clinical setting.“We hope these technologies will not only help return joy to practice by facilitating doctors and scribes with their everyday workload, but also help the patients get more dedicated and thorough medical attention, ideally, leading to better care,” the team said.EHR vendors are also taking a hard look at how machine learning can streamline the user experience by eliminating wasteful interactions and presenting relevant data more intuitively within the workflow.“Taking out the trash” by using artificial intelligence to learn a user’s habits, anticipate their needs, and display the right data at the right time is a top priority for nearly all of the major health IT vendors – vendors who are finding themselves in the hot seat as unhappy customers plead for better solutions for their daily tasks.Both patients and providers are demanding much more consumer-centered tools and interactions from the healthcare industry, and artificial intelligence may now be mature enough to start delivering.“We finally have enough affordable computing power to get the answers we’re looking for,” said James Golden, PhD, Senior Managing Director for PwC’s Healthcare Advisory Group, to Healthcare IT Analytics News on Healthcare BI, Population Health and Data Management in February of 2018.“When I did my PhD in the 90s on back propagation neural networks, we were working with an input layer, an output layer, and two middle layers,” he recalled.“That’s not extremely complex. But it ran for four days on an Apple Lisa before producing results. I can do the same computation today in a picosecond on an iPhone. That is an enormous, staggering leap in our capabilities.”The intersection of more advanced methods, improved processing power, and growing interest in innovative methods of predicting, preventing, and cheapening healthcare will likely bode well for deep learning.With an extremely high number of promising use cases, strong investment from major players in the industry, and a growing amount of data to support cutting-edge analytics, deep learning will no doubt play a central role in the quest to deliver the highest possible quality care to consumers for decades to come.References :What Is Deep Learning and How Will It Change Healthcare?https://royaljay.com/healthcare/neural-networks-in-healthcare/
What are some signs of a really bad selfie?
In the antique, pre-smartphone days, human beings with intense frame-image troubles may typically slip away from elegance or work to test their appearance within the rest room mirror. Now, says psychologist Hilary Weingarden, "We're seeing a number of sufferers who are changing some of that with taking selfies repeatedly, for once in a while up to hours on stop in the course of the day, to test their appearance at exclusive angles and in unique lighting fixtures."Snapchat dysmorphia is the unofficial time period for the outcomes that social media platforms and their easy-to-use filters will have on frame photo problems. It received foreign money final yr in a JAMA article and a couple of headlines.More from WBURNurse Jennifer Maillet prepares addiction medicinal drugs at the Franklin County Jail in Greenfield. (Deborah Becker/WBUR)Mass. Has The First Jail In The Country That's Also A Licensed Methadone Treatment ProviderPlayCommonHealth04:51in 18 hoursNew Device Could Reduce MRI Scanning Times From An Hour To 5 MinutesCommonHealthNov 9, 2019CDC Identifies 'Strong Culprit' In Vaping IllnessesCommonHealthNov 8, 2019MGH Settles With Doctor Critical Of Concurrent Surgery For $13 MillionCommonHealthNov eight, 2019Now, Weingarden and co-workers from Massachusetts General Hospital have posted a practical guide for cosmetic surgeons on the way to understand and treat patients whose appearance issues appear to go beyond usual discontent to a possible prognosis of "body dysmorphic disorder," or BDD.The psychologists had been triggered, they write, by way of final 12 months's caution that sufferers are an increasing number of asking surgeons to assist them appear to be the "greater, regularly unrealistic, versions of themselves" created with Instagram and Snapchat filters.Get the contemporary fitness, medicinal drug and technological know-how information sent in your inbox every week with CommonHealth's newsletter. Sign up now.Filters can be used to feature cute animal ears or flower crowns, but they also can beautify skin, slim faces, extend eyes and lips. They can offer users an idealized model of themselves — until they go back to the actual international.There is not any facts on whether social media is spurring a upward thrust in body dysmorphia, Weingarden says."But anecdotally, what we're seeing is that technology, and additionally social media, have turn out to be extra included into BDD sufferers' signs and rituals," she says. "And it is beginning to also shape their expectations of what it's 'regular' to look like."It became one element whilst simplest movie star photos were edited to appearance perfect, she says. Now, with the assist of picture-enhancing apps, it's sufferers' peers as nicely."That can truely amp up the notion machine that we should not simply appearance top, however we need to appearance ideal in our every day life," she says.Social media systems generally tend to intertwine with sufferers' symptoms, she adds, due to the fact in the event that they submit a selfie on Instagram or Facebook, they're probably to experience nevertheless worse approximately themselves if it garners little wonderful reaction.Dr. Neelam Vashi, an accomplice professor of dermatology at Boston University and co-author of final 12 months's article, says she first noticed a shift approximately 4 or five years in the past: A cosmetic surgery patient came in with a selfie in place of the usual image of a celebrity.Patients also started asking for assist to appearance higher of their selfies, she says, "and then, all of a surprising, with these Snapchat-filtered pix, pronouncing 'Oh my gosh, I need to appear to be this.' "Sometimes the angles of selfies motive issues, Vashi says, due to the fact they generally tend to magnify noses, and a patient may ask for surgical treatment that would make them look better in a selfie but actually deliver them an abnormally small nostril."What mirror are we reflecting ourselves in now?" Vashi asks approximately social media use. "What are we trying to prove and what are we seeking to see? I think it may make people sense simply horrific approximately themselves."What To Do?So while does everyday discontent approximately appearance move into pathology?In their new guide, Weingarden and colleagues recommend that surgeons use a screening questionnaire, and if a patient checks high quality for probable BDD, carefully endorse that psychological treatment might be a good first step.Vashi suggests that docs start with a simple set of questions: Do you consider your appearance plenty and want you may think about it much less? Does it disenchanted you plenty? Has it brought on you troubles with your work or college or courting? Are there things you keep away from due to your appearance?"And if they say, 'Yeah, I cry each day,' nicely, that could be a sign to maybe then take it to the subsequent level and deliver them the questionnaire," she says.People with frame dysmorphia "are not desirable applicants for beauty remedies, because with this disease, the root trouble is psychological instead of a true appearance flaw," she says. "So it really cannot be constant cosmetically."Body dysmorphia impacts an expected 2 percentage of the populace, however about 13 percentage of folks that are looking for beauty surgery. There appears to be a genetic detail involved, says Vashi, who edited a ebook on beauty and BDD, and sufferers regularly describe events or feedback that seemed to trigger the disease.Surgeons should now not be surprised if sufferers face up to mental treatment, Weingarden says, because many firmly trust the trouble is a bodily illness, now not a perceptual issue.But "there clearly are powerful treatments obtainable," she says. Cognitive-Behavioral Therapy, specifically, she says: It can help sufferers truth-test their thoughts approximately their appearance, face conditions they generally tend to keep away from, and provide them "perceptual retraining."For instance, Weingarden says, when dysmorphia patients look in the replicate, they tend to fixate on small info. So part of the education involves "learning a way to appearance inside the reflect otherwise," refraining from looking up close with vibrant lights and as a substitute "stepping back and looking at yourself holistically, from top to backside," and greater objectively, with out applying emotional labels.Work is beneath manner on a cognitive-behavioral app that would help patients as nicely, she says.The issues that filtered selfies improve for BDD patients might be visible as intense examples of the selfie technology writ big."We're living in a place in which we are all continuously glued to photographs of everyone," Weingarden says. "It's difficult for the average character no longer to constantly examine themselves to others in this period. And when you have a vulnerability to look distress, it's got to be just truely difficult."
Has AI and machine learning led to any significant changes in healthcare?
Top 12 Ways Artificial Intelligence and Machine learning Will Impact HealthcareArtificial intelligence is poised to become a transformational force in healthcare. How will providers and patients benefit from the impact of AI-driven tools?my other blog:Top 12 Ways Artificial Intelligence Will Impact HealthcareThe healthcare industry is ripe for some major changes. From chronic diseases and cancer to radiology and risk assessment, there are nearly endless opportunities to leverage technology to deploy more precise, efficient, and impactful interventions at exactly the right moment in a patient’s care. As payment structures evolve, patients demand more from their providers, and the volume of available data continues to increase at a staggering rate, artificial intelligence is poised to be the engine that drives improvements across the care continuum. AI offers a number of advantages over traditional analytics and clinical decision-making techniques. Learning algorithms can become more precise and accurate as they interact with training data, allowing humans to gain unprecedented insights into diagnostics, care processes, treatment variability, and patient outcomes. At the 2018 World Medical Innovation Forum (WMIF) on artificial intelligence presented by Partners Healthcare, a leading researchers and clinical faculty members showcased the twelve technologies and areas of the healthcare industry that are most likely to see a major impact from artificial intelligence within the next decade. Every member of this “Disruptive Dozen” has the potential to produce a significant benefit to patients while possessing the potential for broad commercial success, said WMIF co-chairs Anne Kiblanksi, MD, Chief Academic Officer at Partners Healthcare and Gregg Meyer, MD, Chief Clinical Officer. With the help of experts from across the Partners Healthcare system, including faculty from Harvard Medical School (HMS), moderators Keith Dreyer, DO, PhD, Chief Data Science Officer at Partners and Katherine Andriole, PhD, Director of Research Strategy and Operations at Massachusetts General Hospital (MGH), counted down the top 12 ways artificial intelligence will revolutionize the delivery and science of healthcare. UNIFYING MIND AND MACHINE THROUGH BRAIN-COMPUTER INTERFACES Using computers to communicate is not a new idea by any means, but creating direct interfaces between technology and the human mind without the need for keyboards, mice, and monitors is a cutting-edge area of research that has significant applications for some patients. Neurological diseases and trauma to the nervous system can take away some patients’ abilities to speak, move, and interact meaningfully with people and their environments. Brain-computer interfaces (BCIs) backed by artificial intelligence could restore those fundamental experiences to those who feared them lost forever. “If I’m in the neurology ICU on a Monday, and I see someone who has suddenly lost the ability to move or to speak, we want to restore that ability to communicate by Tuesday,” said Leigh Hochberg, MD, PhD, Director of the Center for Neurotechnology and Neurorecovery at MGH. “By using a BCI and artificial intelligence, we can decode the neural activates associated with the intended movement of one’s hand, and we should be ablhttps://tech-starist.blogspot.com/2021/03/top-12-ways-artificial-intelligence.htmlThe healthcare industry is ripe for some major changes. From chronic diseases and cancer to radiology and risk assessment, there are nearly endless opportunities to leverage technology to deploy more precise, efficient, and impactful interventions at exactly the right moment in a patient’s care.As payment structures evolve, patients demand more from their providers, and the volume of available data continues to increase at a staggering rate, artificial intelligence is poised to be the engine that drives improvements across the care continuum.AI offers a number of advantages over traditional analytics and clinical decision-making techniques. Learning algorithms can become more precise and accurate as they interact with training data, allowing humans to gain unprecedented insights into diagnostics, care processes, treatment variability, and patient outcomes.At the 2018 World Medical Innovation Forum (WMIF) on artificial intelligence presented by Partners Healthcare, a leading researchers and clinical faculty members showcased the twelve technologies and areas of the healthcare industry that are most likely to see a major impact from artificial intelligence within the next decade.Every member of this “Disruptive Dozen” has the potential to produce a significant benefit to patients while possessing the potential for broad commercial success, said WMIF co-chairs Anne Kiblanksi, MD, Chief Academic Officer at Partners Healthcare and Gregg Meyer, MD, Chief Clinical Officer.With the help of experts from across the Partners Healthcare system, including faculty from Harvard Medical School (HMS), moderators Keith Dreyer, DO, PhD, Chief Data Science Officer at Partners and Katherine Andriole, PhD, Director of Research Strategy and Operations at Massachusetts General Hospital (MGH), counted down the top 12 ways artificial intelligence will revolutionize the delivery and science of healthcare.UNIFYING MIND AND MACHINE THROUGH BRAIN-COMPUTER INTERFACESUsing computers to communicate is not a new idea by any means, but creating direct interfaces between technology and the human mind without the need for keyboards, mice, and monitors is a cutting-edge area of research that has significant applications for some patients.Neurological diseases and trauma to the nervous system can take away some patients’ abilities to speak, move, and interact meaningfully with people and their environments. Brain-computer interfaces (BCIs) backed by artificial intelligence could restore those fundamental experiences to those who feared them lost forever.“If I’m in the neurology ICU on a Monday, and I see someone who has suddenly lost the ability to move or to speak, we want to restore that ability to communicate by Tuesday,” said Leigh Hochberg, MD, PhD, Director of the Center for Neurotechnology and Neurorecovery at MGH.“By using a BCI and artificial intelligence, we can decode the neural activates associated with the intended movement of one’s hand, and we should be able to allow that person to communicate the same way as many people in this room have communicated at least five times over the course of the morning using a ubiquitous communication technology like a tablet computer or phone.”Brain-computer interfaces could drastically improve quality of life for patients with ALS, strokes, or locked-in syndrome, as well as the 500,000 people worldwide who experience spinal cord injuries every year.DEVELOPING THE NEXT GENERATION OF RADIOLOGY TOOLSRadiological images obtained by MRI machines, CT scanners, and x-rays offer non-invasive visibility into the inner workings of the human body. But many diagnostic processes still rely on physical tissue samples obtained through biopsies, which carry risks including the potential for infection.Artificial intelligence will enable the next generation of radiology tools that are accurate and detailed enough to replace the need for tissue samples in some cases, experts predict.We want to bring together the diagnostic imaging team with the surgeon or interventional radiologist and the pathologist,” said Alexandra Golby, MD, Director of Image-Guided Neurosurgery at Brigham & Women’s Hospital (BWH). “That coming together of different teams and aligning goals is a big challenge.”“If we want the imaging to give us information that we presently get from tissue samples, then we’re going to have to be able to achieve very close registration so that the ground truth for any given pixel is known.”Succeeding in this quest may allow clinicians to develop a more accurate understanding of how tumors behave as a whole instead of basing treatment decisions on the properties of a small segment of the malignancy.Providers may also be able to better define the aggressiveness of cancers and target treatments more appropriately.Artificial intelligence is helping to enable “virtual biopsies” and advance the innovative field of radiomics, which focuses on harnessing image-based algorithms to characterize the phenotypes and genetic properties of tumors.EXPANDING ACCESS TO CARE IN UNDERSERVED OR DEVELOPING REGIONSShortages of trained healthcare providers, including ultrasound technicians and radiologists can significantly limit access to life-saving care in developing nations around the world.More radiologists work in the half-dozen hospitals lining the renowned Longwood Avenue in Boston than in all of West Africa, the session pointed out.Artificial intelligence could help mitigate the impacts of this severe deficit of qualified clinical staff by taking over some of the diagnostic duties typically allocated to humans.For example, AI imaging tools can screen chest x-rays for signs of tuberculosis, often achieving a level of accuracy comparable to humans. This capability could be deployed through an app available to providers in low-resource areas, reducing the need for a trained diagnostic radiologist on site.“The potential for this tech to increase access to healthcare is tremendous,” said Jayashree Kalpathy-Cramer, PhD, Assistant in Neuroscience at MGH and Associate Professor of Radiology at HMS.Source: World Medical Innovation Forum 2018However, algorithm developers must be careful to account for the fact that disparate ethnic groups or residents of different regions may have unique physiologies and environmental factors that will influence the presentation of disease.“The course of a disease and population affected by the disease may look very different in India than in the US, for example,” she said.“As we’re developing these algorithms, it’s very important to make sure that the data represents a diversity of disease presentations and populations – we can’t just develop an algorithm based on a single population and expect it to work as well on others.”REDUCING THE BURDENS OF ELECTRONIC HEALTH RECORD USEEHRs have played an instrumental role in the healthcare industry’s journey towards digitalization, but the switch has brought myriad problems associated with cognitive overload, endless documentation, and user burnout.EHR developers are now using artificial intelligence to create more intuitive interfaces and automate some of the routine processes that consume so much of a user’s time.Users spend the majority of their time on three tasks: clinical documentation, order entry, and sorting through the in-basket, said Adam Landman, MD, Vice President and CIO at Brigham Health.Voice recognition and dictation are helping to improve the clinical documentation process, but natural language processing (NLP) tools might not be going far enough.“I think we may need to be even bolder and consider changes like video recording a clinical encounter, almost like police wear body cams,” said Landman. “And then you can use AI and machine learning to index those videos for future information retrieval.“And just like in the home, where we’re using Siri and Alexa, the future will bring virtual assistants to the bedside for clinicians to use with embedded intelligence for order entry.”Artificial intelligence may also help to process routine requests from the inbox, like medication refills and result notifications. It may also help to prioritize tasks that truly require the clinician’s attention, Landman added, making it easier for users to work through their to-do lists.CONTAINING THE RISKS OF ANTIBIOTIC RESISTANCEAntibiotic resistance is a growing threat to populations around the world as overuse of these critical drugs fosters the evolution of superbugs that no longer respond to treatments. Multi-drug resistant organisms can wreak havoc in the hospital setting, and claim thousands of lives every year.C. difficile alone accounts for approximately $5 billion in annual costs for the US healthcare system and claims more than 30,000 lives.Electronic health record data can help to identify infection patterns and highlight patients at risk before they begin to show symptoms. Leveraging machine learning and AI tools to drive these analytics can enhance their accuracy and create faster, more accurate alerts for healthcare providers.“AI tools can live up to the expectation for infection control and antibiotic resistance,” Erica Shenoy, MD, PhD, Associate Chief of the Infection Control Unit at MGH.“If they don’t, then that’s really a failure on all of our parts. For the hospitals sitting on mountains of EHR data and not using them to the fullest potential, to industry that’s not creating smarter, faster clinical trial design, and for EHRs that are creating these data not to use them…that would be a failure.”CREATING MORE PRECISE ANALYTICS FOR PATHOLOGY IMAGESPathologists provide one of the most significant sources of diagnostic data for providers across the spectrum of care delivery, says Jeffrey Golden, MD, Chair of the Department of Pathology at BWH and a professor of pathology at HMS.“Seventy percent of all decisions in healthcare are based on a pathology result,” he said. “Somewhere between 70 and 75 percent of all the data in an EHR are from a pathology result. So the more accurate we get, and the sooner we get to the right diagnosis, the better we’re going to be. That’s what digital pathology and AI has the opportunity to deliver.”Analytics that can drill down to the pixel level on extremely large digital images can allow providers to identify nuances that may escape the human eye.“We’re now getting to the point where we can do a better job of assessing whether a cancer is going to progress rapidly or slowly and how that might change how patients will be treated based on an algorithm rather than clinical staging or the histopathologic grade,” said Golden. “That’s going to be a huge advance.”Artificial intelligence can also improve productivity by identifying features of interest in slides before a human clinician reviews the data, he added.“AI can screen through slides and direct us to the right thing to look at so we can assess what’s important and what’s not. That increases the efficiency of the use of the pathologist and increases the value of the time they spend for each case.”BRINGING INTELLIGENCE TO MEDICAL DEVICES AND MACHINESSmart devices are taking over the consumer environment, offering everything from real-time video from the inside of a refrigerator to cars that can detect when the driver is distracted.In the medical environment, smart devices are critical for monitoring patients in the ICU and elsewhere. Using artificial intelligence to enhance the ability to identify deterioration, suggest that sepsis is taking hold, or sense the development of complications can significantly improve outcomes and may reduce costs related to hospital-acquired condition penalties.Source: Thinkstock“When we’re talking about integrating disparate data from across the healthcare system, integrating it, and generating an alert that would alert an ICU doctor to intervene early on – the aggregation of that data is not something that a human can do very well,” said Mark Michalski, MD, Executive Director of the MGH & BWH Center for Clinical Data Science.Inserting intelligent algorithms into these devices can reduce cognitive burdens for physicians while ensuring that patients receive care in as timely a manner as possible.ADVANCING THE USE OF IMMUNOTHERAPY FOR CANCER TREATMENTImmunotherapy is one of the most promising avenues for treating cancer. By using the body’s own immune system to attack malignancies, patients may be able to beat stubborn tumors. However, only a small number of patients respond to current immunotherapy options, and oncologists still do not have a precise and reliable method for identifying which patients will benefit from this option.Machine learning algorithms and their ability to synthesize highly complex datasets may be able to illuminate new options for targeting therapies to an individual’s unique genetic makeup.“Recently, the most exciting development has been checkpoint inhibitors, which block some of the proteins made by some times of immune cells,” explained Long Le, MD, PhD, Director of Computational Pathology and Technology Development at the MGH Center for Integrated Diagnostics. “But we still don’t understand all of the disease biology. This is a very complex problem.”“We definitely need more patient data. The therapies are relatively new, so not a lot of patients have actually been put on these drugs. So whether we need to integrate data within one institution or across multiple institutions is going to be a key factor in terms of augmenting the patient population to drive the modeling process.”TURNING THE ELECTRONIC HEALTH RECORD INTO A RELIABLE RISK PREDICTOREHRs are a goldmine of patient data, but extracting and analyzing that wealth of information in an accurate, timely, and reliable manner has been a continual challenge for providers and developers.Data quality and integrity issues, plus a mishmash of data formats, structured and unstructured inputs, and incomplete records have made it very difficult to understand exactly how to engage in meaningful risk stratification, predictive analytics, and clinical decision support.“Part of the hard work is integrating the data into one place,” observed Ziad Obermeyer, MD, Assistant Professor of Emergency Medicine at BWH and Assistant Professor at HMS. “But another problem is understanding what it is you’re getting when you’re predicting a disease in an EHR.”“You might hear that an algorithm can predict depression or stroke, but when you scratch the surface, you find what they’re actually predicting is a billing code for stroke. That’s very different from stroke itself.”Relying on MRI results might appear to offer a more concrete dataset, he continued.“But now you have to think about who can afford the MRI, and who can’t? So what you end up predicting isn’t what you thought you were predicting. You might be predicting billing for a stroke in people who can pay for a diagnostic rather than some sort of cerebral ischemia.”EHR analytics have produced many successful risk scoring and stratification tools, especially when researchers employ deep learning techniques to identify novel connections between seemingly unrelated datasets.But ensuring that those algorithms do not confirm hidden biases in the data is crucial for deploying tools that will truly improve clinical care, Obermeyer maintained.“The biggest challenge will be making sure exactly what we’re predicting even before we start opening up the black box and looking at how we’re predicting it,” he said.MONITORING HEALTH THROUGH WEARABLES AND PERSONAL DEVICESAlmost all consumers now have access to devices with sensors that can collect valuable data about their health. From smartphones with step trackers to wearables that can track a heartbeat around the clock, a growing proportion of health-related data is generated on the go.Collecting and analyzing this data – and supplementing it with patient-provided information through apps and other home monitoring devices – can offer a unique perspective into individual and population health.Artificial intelligence will play a significant role in extracting actionable insights from this large and varied treasure trove of data.But helping patients get comfortable with sharing data from this intimate, continual monitoring may require a little extra work, says Omar Arnaout, MD, Co-director of the Computation Neuroscience Outcomes Center and an attending neurosurgeon at BWH.“As a society, we’ve been pretty liberal with our digital data,” he said. But as things come into our collective consciousness like Cambridge Analytica and Facebook, people will become more and more prudent about who they share what kinds of data with.”However, patients tend to trust their physicians more than they might trust a big company like Facebook, he added, which may help to ease any discomfort with contributing data to large-scale research initiatives.“There’s a very good chance [wearable data will have a major impact] because our care is very episodic and the data we collect is very coarse,” said Arnaout. “By collecting granular data in a continuous fashion, there’s a greater likelihood that the data will help us take better care of patients.”MAKING SMARTPHONE SELFIES INTO POWERFUL DIAGNOSTIC TOOLSContinuing the theme of harnessing the power of portable devices, experts believe that images taken from smartphones and other consumer-grade sources will be an important supplement to clinical quality imaging – especially in underserved populations or developing nations.The quality of cell phone cameras is increasing every year, and can produce images that are viable for analysis by artificial intelligence algorithms. Dermatology and ophthalmology are early beneficiaries of this trend.Researchers in the United Kingdom have even developed a tool that identifies developmental diseases by analyzing images of a child’s face. The algorithm can detect discrete features, such as a child’s jaw line, eye and nose placement, and other attributes that might indicate a craniofacial abnormality. Currently, the tool can match the ordinary images to more than 90 disorders to provide clinical decision support.“The majority of the population is equipped with pocket-sized, powerful devices that have a lot of different sensors built in,” said Hadi Shafiee, PhD, Director of the Laboratory of Micro/Nanomedicine and Digital Health at BWH.“This is a great opportunity for us. Almost every major player in the industry has started to build AI software and hardware into their devices. That’s not a coincidence. Every day in our digital world, we generate more than 2.5 million terabytes of data. In cell phones, the manufacturers believe they can use that data with AI to provide much more personalized and faster and smarter services.”Source: ThinkstockUsing smartphones to collect images of eyes, skin lesions, wounds, infections, medications, or other subjects may be able to help underserved areas cope with a shortage of specialists while reducing the time-to-diagnosis for certain complaints.“There is something big happening,” said Shafiee. “We can leverage that opportunity to address some of the important problems with have in disease management at the point of care.”REVOLUTIONIZING CLINICAL DECISION MAKING WITH ARTIFICIAL INTELLIGENCE AT THE BEDSIDEAs the healthcare industry shifts away from fee-for-service, so too is it moving further and further from reactive care. Getting ahead of chronic diseases, costly acute events, and sudden deterioration is the goal of every provider – and reimbursement structures are finally allowing them to develop the processes that will enable proactive, predictive interventions.Artificial intelligence will provide much of the bedrock for that evolution by powering predictive analytics andclinical decision support tools that clue providers in to problems long before they might otherwise recognize the need to act.AI can provide earlier warnings for conditions like seizures or sepsis, which often require intensive analysis of highly complex datasets.Machine learning can also help support decisions around whether or not to continue care for critically ill patients, such as those who have entered a coma after cardiac arrest, says Brandon Westover, MD, PhD, Director of the MGH Clinical Data Animation Center.Typically, providers must visually inspect EEG data from these patients, he explained. The process is time-consuming and subjective, and the results may vary with the skill and experience of the individual clinician.“In these patients, trends might be slowly evolving,” he said. “Sometimes when we’re looking to see if someone is recovering, we take the data from ten seconds of monitoring at a time. But trying to see if it changed from ten seconds of data taken 24 hours ago is like trying to look if your hair is growing longer.”“But if you have an AI algorithm and lots and lots of data from many patients, it’s easier to match up what you’re seeing to long term patterns and maybe detect subtle improvements that would impact your decisions around care.”Leveraging AI for clinical decision support, risk scoring, and early alerting is one of the most promising areas of development for this revolutionary approach to data analysis.By powering a new generation of tools and systems that make clinicians more aware of nuances, more efficient when delivering care, and more likely to get ahead of developing problems, AI will usher in a new era of clinical quality and exciting breakthroughs in patient care.
- Home >
- Catalog >
- Life >
- Medical Forms >
- Medical History Form >
- Patient History Form >
- Mgh Dermatology New Patient