A Quick Guide to Editing The Task Analysis For Setting The Table
Below you can get an idea about how to edit and complete a Task Analysis For Setting The Table quickly. Get started now.
- Push the“Get Form” Button below . Here you would be transferred into a page making it possible for you to make edits on the document.
- Choose a tool you want from the toolbar that appears in the dashboard.
- After editing, double check and press the button Download.
- Don't hesistate to contact us via [email protected] if you need further assistance.
The Most Powerful Tool to Edit and Complete The Task Analysis For Setting The Table
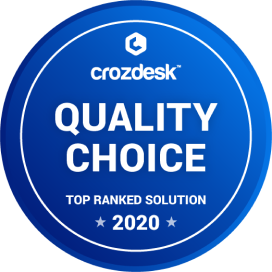
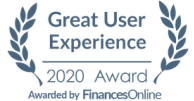
A Simple Manual to Edit Task Analysis For Setting The Table Online
Are you seeking to edit forms online? CocoDoc can help you with its Complete PDF toolset. You can make full use of it simply by opening any web brower. The whole process is easy and quick. Check below to find out
- go to the free PDF Editor page.
- Upload a document you want to edit by clicking Choose File or simply dragging or dropping.
- Conduct the desired edits on your document with the toolbar on the top of the dashboard.
- Download the file once it is finalized .
Steps in Editing Task Analysis For Setting The Table on Windows
It's to find a default application that can help make edits to a PDF document. Luckily CocoDoc has come to your rescue. View the Manual below to find out possible approaches to edit PDF on your Windows system.
- Begin by obtaining CocoDoc application into your PC.
- Upload your PDF in the dashboard and make modifications on it with the toolbar listed above
- After double checking, download or save the document.
- There area also many other methods to edit your PDF for free, you can check this page
A Quick Guide in Editing a Task Analysis For Setting The Table on Mac
Thinking about how to edit PDF documents with your Mac? CocoDoc offers a wonderful solution for you.. It enables you to edit documents in multiple ways. Get started now
- Install CocoDoc onto your Mac device or go to the CocoDoc website with a Mac browser. Select PDF document from your Mac device. You can do so by clicking the tab Choose File, or by dropping or dragging. Edit the PDF document in the new dashboard which includes a full set of PDF tools. Save the file by downloading.
A Complete Manual in Editing Task Analysis For Setting The Table on G Suite
Intergating G Suite with PDF services is marvellous progess in technology, with the power to reduce your PDF editing process, making it faster and more cost-effective. Make use of CocoDoc's G Suite integration now.
Editing PDF on G Suite is as easy as it can be
- Visit Google WorkPlace Marketplace and find CocoDoc
- install the CocoDoc add-on into your Google account. Now you can edit documents.
- Select a file desired by hitting the tab Choose File and start editing.
- After making all necessary edits, download it into your device.
PDF Editor FAQ
Why is pixelated camouflage better?
It really isn’t. It’s not that the pixels are bad though, either.Using square “bits” vs. some other shape isn’t really all that important when it comes do effective camo designs.What digital camo brought to the table was a more scientific analysis of how to distribute color and pattern. This concept dates to the 1970’s, and at the time computers weren’t really up to the task of complex shapesA camo pattern needs to do several things:Have a reasonable color match. This, fundamentally, was the problem with UCP. Bright red and orange isn’t going to be a good combination, regardless of what else you do.Break up movement. This relies on non-recurring patterns and the right pattern density.Break up shapes. This again relies on the right pattern density, and an understanding of how light interacts with different colors.Keep in mind that all of this is dependent on other factors, and it’s all compromise. Larger things tend to get larger patterns, because smaller pattern start to average out and not be as effective. Thermal and night vision are factors. Anticipated opponents are a factor as well.The infamous WW1 dazzle “camouflage” is an example. It doesn’t really hide the ship (Though at a distance, it will break up its location on the horizon), and that really isn’t its job. It’s job is to make it difficult to tell range and bearing… Or, basically, break up movement. If you make an enemy vessel fire an extra sighting round or two, you have a big advantage.One should also be careful when comparing pictures of camo. Too many of them are taken from entirely too close. Camo is unlikely to be effective at five yards.This is a better (if somewhat humorous) picture:But we should be careful with this as well. Cadpat here is sitting in an area that’s shaded. If he were to switch places with Tiger, Tiger would likely disappear.One should be especially cautious of this when looking at manufactures comparisons, all of which are inevitably set up to make their own product look good.And again, there is really no single universal pattern. I live in the high desert, in terrain not unlike Afghanistan, and here, multicam works very well… It’s likely less effective in jungle or arctic conditions.
After having 2 very good conferences, I was screened out 2 times from the same SSB. What could be possible reasons for this?
Ever played/seen find the coin game? The picture might help you recollect what it is.Now, imagine when you join the game, the glasses are already on the table and you don’t know which one has coin.The moderator juggles the three in a very fine way, and then you are to choose one. You choose the one which has the coin. But, (remember when you joined the game, the glasses were already on the table), can you tell me what is under the other two glasses?NO!The SSB perspective:The Glasses are the Psychiatrist, the Group Task Officer (GTO), the Interviewing Officer (IO). They juggle up a varied number of tests to test your caliber for commanding men. *The table is set*Now, comes the mistake that we generally make, i.e., resonating the success of our visible operation as the success of all the tasks. The only visible performance we have is when the GTO analysis us. If we perform well in them, a false alarm sets off. The fellow candidates and we ourselves imagine oneself to be better than others. *Glass with coin is lifted up*However, we tend to forget the winner is one who predicts what is beneath the three glasses, or at least 2/3. *Other two are forgotten*Now, with you my friend, I would wish to know how do you rate your SSB performances better, when only 33.33% of your performance is visible?Cheers! :)
Are you a self-made data scientist? How did you do it?
Yes, I think I qualify to answer this question. Prior to my current role at Kabbage, I had a (sucessful) 8 year long career in designing graphics, mobile and server processors. The entire process took nearly a year with my interview period lasting roughly 2–3 months. I ended up attending 5 interviews on site and had offers from 3. Here is my story.The Motivation Phase (1 month)As a computer engineering graduate, I had shockingly minimal exposure to statistics and no exposure to machine learning. Initially, the buzz around data science in the tech media got me interested in the area. I pored over blogs, news media and articles online trying to define to myself the idea of a ‘data scientist’ and what makes one successful in the role. This included exploring stories about data science making a difference in diverse fields from healthcare to recruiting to marketing to education to everything in between. Gradually, the hype turned to genuine potential in my mind. This phase of setting the motivation was hugely important and set up the intrinsic drive to succeed. Otherwise, it would have been highly likely that I would have given up the endeavor before seeing it through to the end.Next, I set out to figure out the skills required to become a data scientist. The most popular idea on the net is that a data scientist is a super-human who sits at the intersection of programming, statistical (and ML), math, business domain and communication skills. Additionally, I would also like to throw in familiarity with big data tools like Hadoop/Spark/AWS to the mix based on my experience. I could only check off the software engineering skills (hacking skills) in this Venn diagram! One of my primary complaints with my prior job as a computer architect was that I felt that my learning curve was saturating. With such a vast skills requirement, I knew that there would always something new for me to learn for a very long time!The Knowledge Phase (9 months)The knowledge phase involved signing up for and completing a number of courses online through Udacity (U), Coursera (C) and edX (E). While Coursera was somewhat dry and theoretical and focused on the traditional lecture format, both Udacity and EdX focussed on a more interactive learning experience with short videos and tons of built in quizzes and programming questions to help with learning by doing. While this phase lasted a long time, it was because there were huge knowledge gaps to fill. I was also mostly taking these classes in the evenings or weekends after my regular work hours aka spare time. My focus was on statistics, machine learning, exploratory data analysis and some advanced topics (since I already had the programming and software engineering skills from my prior job).List of classes I took but not necessarily in that order (not just skim, but actually complete all the lectures, assignments and mini-projects for the most part):Descriptive and Inferential Statistics (U) - CriticalMachine Learning by Sebastian Thrun (U) - Critical/PracticalExploratory Data Analysis (U) - CriticalA/B Testing (U) - Nice to KnowRecommender Systems (C) - Nice to KnowText Mining and Analytics (C) - Skimmed/Nice to KnowMachine Learning by Andrew Ng (C) - Critical/TheoreticalIntroduction to Hadoop (U) - Critical/Nice to knowIntroduction to Big Data (E) - Critical/Nice to knowBig Data Analysis with Apache Spark (E) - Nice to knowAlgorithms I & II (C) - Mostly for interview purposesThe Skill Building Phase (6 months)This was a super interesting phase.After arming myself with the necessary fundamentals and practical tools (Python, R, mathematical and statistical base), it was time to put them to work. Enter Kaggle. For those not in the know, Kaggle is an online competitive platform for budding and experienced data scientists. Its a chance to get your hands dirty with real datasets from real companies to solve real problems using insights gleaned from data. Most of all, it is a vibrant community of like minded people having fun and learning from each other. Kaggle was single handedly successful in teaching me the nuances of experiment design, data pre-processing, feature engineering, model validation, and ensemble building. The gamification of the task at hand (leaderboard, rankings, forums etc) made the experience hugely rewarding and fun at the same time. Not to mention that you can use your achievements on Kaggle to get recruiter eyeballs. For more details on this, see: Vijay Sathish's answer to Do recruiters really care about Kaggle achievements or successfully completed courses in Coursera?The second phase of the skills building involved trying to get my hands dirty with real world data science projects. One of the drawbacks with Kaggle is that the problem is already defined, the data is provided to you (and mostly in clean format), and success is defined. In the real world, translating a business problem into a data science task, identifying data sources, extracting the data from multiple sources, data cleaning, defining metrics for success, find ground truth or labels are equally critical tasks. The model building and validation part is probably the easiest task. I identified several problem areas in my field at Oracle related to processor workload analysis, performance coverage analysis, and workload sampling - defined the problem, identified the datasets and metrics and got to work using supervised. unsupervised learning techniques and visualizations to tackle the issues at hand. Most of my team being fellow computer architects had minimal or no experience in machine learning, so this was unchartered territory. The insights I brought to the table from a different perspective was hugely rewarding and my colleagues looked at me with new respect. I was essentially disrupting computer architecture within the team! This also further strengthened my resolve to pursue data science as a full time job.The Interview Phase (3 months)The interview phase is the signaling phase. It is about signaling to the recruiter that you have the skills required on paper and convincing your future manager and team mates that you can execute on those skills on the job. The Kaggle achievements (my profile: VijaySathish | Kaggle and see: Vijay Sathish's answer to How can a beginner train for machine learning contests? What are the fundamental ideas, tools, and information resources I need to start building expertise in machine learning?) and my data science projects at work helped garner the attention of recruiters and get my foot in the door. My theoretical grounding from the coursework and all the experience picked up from actually executing the various data science projects helped convince my interviewers.I applied for interviews in discrete waves. This means that I would apply to 10–15 companies per week and wait for responses. If I got 2–3 responses, I would stop applying for sometime, otherwise I would apply for another handful next week. I also focused on the skills required of the job, and the specific industry while applying because the job ‘Data Scientist’ can take on a surprisingly wide range of possibilities depending on the company. (For example, a job description that included prior experience of NLP, deep learning or computer vision would be outside my expertise /skills, while a job that primarily involved querying databases, A/B testing or product analytics felt more like a traditional analyst role which was not what I was looking for.) I mostly used AngelList and LinkedIn to apply for data science jobs and focussed on medium stage startups. Early stage startups typically have little or no software infrastructure setup, so you would spend most of your time on software engineering rather than data science tasks. This is fine for some people, but this was not my primary focus for my first data science job. Big companies like Google, Facebook, Microsoft etc. have a higher bar and it would be almost impossible to even get past the recruiter stage given their vast candidate pool.Data Science is an upcoming field and attracts professionals from diverse fields. The single most important skill in the interview (and perhaps also at work) is storytelling. You are the best person to market yourself. Highlight your competencies, your motivation for the job and what you can bring to the table. Wrap an interesting narrative around your favorite project. On my phone screen with my (to be) manager at Kabbage, I started with the story of solving the problem of computational resources and time for the company. I explained how I used workload clustering to pick and choose representative workloads from a huge workload space to monitor for weekly performance regressions and brought down computation requirements by 10x. I explained how I used our in-house performance simulator and our experiment logs to extract the data for this study. I could tell that my manager started very skeptical (because she was new to the field of computer architecture), but by the end, she came away very impressed with my effort. I can say that I had won more than 50% of the battle by that point.In summary, transitioning to data science from a lateral field required immense patience, but it was also an extremely rewarding journey for me in the end. (On transitioning, see: Vijay Sathish's answer to Should I start as a Data Analyst or Software Engineer to become a Data Scientist?)Finally, if you are a self taught data scientist and think you have what it takes, check us out and apply for these awesome roles: DataScientist@Kabbage and DataEngineer@Kabbage.
- Home >
- Catalog >
- Business >
- Job Application Form >
- Target Job Application Form >
- Target Application Form >
- target application status >
- Task Analysis For Setting The Table