The Guide of completing Sample Will Pdf Online
If you are curious about Fill and create a Sample Will Pdf, here are the simple steps you need to follow:
- Hit the "Get Form" Button on this page.
- Wait in a petient way for the upload of your Sample Will Pdf.
- You can erase, text, sign or highlight of your choice.
- Click "Download" to keep the files.
A Revolutionary Tool to Edit and Create Sample Will Pdf
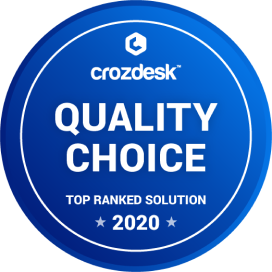
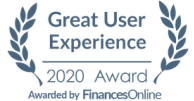
How to Easily Edit Sample Will Pdf Online
CocoDoc has made it easier for people to Customize their important documents on online website. They can easily Customize through their choices. To know the process of editing PDF document or application across the online platform, you need to follow this stey-by-step guide:
- Open the official website of CocoDoc on their device's browser.
- Hit "Edit PDF Online" button and Attach the PDF file from the device without even logging in through an account.
- Edit your PDF documents by using this toolbar.
- Once done, they can save the document from the platform.
Once the document is edited using online website, the user can export the form according to your ideas. CocoDoc promises friendly environment for implementing the PDF documents.
How to Edit and Download Sample Will Pdf on Windows
Windows users are very common throughout the world. They have met a lot of applications that have offered them services in managing PDF documents. However, they have always missed an important feature within these applications. CocoDoc intends to offer Windows users the ultimate experience of editing their documents across their online interface.
The process of editing a PDF document with CocoDoc is very simple. You need to follow these steps.
- Choose and Install CocoDoc from your Windows Store.
- Open the software to Select the PDF file from your Windows device and go on editing the document.
- Customize the PDF file with the appropriate toolkit showed at CocoDoc.
- Over completion, Hit "Download" to conserve the changes.
A Guide of Editing Sample Will Pdf on Mac
CocoDoc has brought an impressive solution for people who own a Mac. It has allowed them to have their documents edited quickly. Mac users can fill PDF forms with the help of the online platform provided by CocoDoc.
In order to learn the process of editing form with CocoDoc, you should look across the steps presented as follows:
- Install CocoDoc on you Mac firstly.
- Once the tool is opened, the user can upload their PDF file from the Mac quickly.
- Drag and Drop the file, or choose file by mouse-clicking "Choose File" button and start editing.
- save the file on your device.
Mac users can export their resulting files in various ways. Downloading across devices and adding to cloud storage are all allowed, and they can even share with others through email. They are provided with the opportunity of editting file through different ways without downloading any tool within their device.
A Guide of Editing Sample Will Pdf on G Suite
Google Workplace is a powerful platform that has connected officials of a single workplace in a unique manner. If users want to share file across the platform, they are interconnected in covering all major tasks that can be carried out within a physical workplace.
follow the steps to eidt Sample Will Pdf on G Suite
- move toward Google Workspace Marketplace and Install CocoDoc add-on.
- Select the file and click "Open with" in Google Drive.
- Moving forward to edit the document with the CocoDoc present in the PDF editing window.
- When the file is edited completely, save it through the platform.
PDF Editor FAQ
What is the difference between a probability density function and a cumulative distribution function?
A PDF answers the question: "How common are samples at exactly this value?" A CDF answers the question "How common are samples that are less than or equal to this value?" The CDF is the integral of the PDF.Here's the PDF of various normal distributions:Here's the CDF of the same normal distributions:
How do I convert pdf. files to word. or txt. without keeping the pictures? Is there a program or a way to convert batches of pdf. files at a time?
Check out RasterMaster at Snowbound.com. You can extract text from a PDF file and save it as text. The vector, text search, and batch convert samples will get you started. From there you can name the output files as you please.It does require some coding, but RasterMaster does the heavy lifting. You can use your choice of Java, C, C#, or VB.
What are some common errors in machine learning caused by poor knowledge of statistics?
The most common and basic mistake which I saw people with poor knowledge of statistics make is to apply different ML tools on data without understanding the importance of dimensionality constant and data’s probability distribution.1. Dimensionality constant and the Curse of Dimensionality: Dimensionality constant gives a metric to understand if enough data is available to apply a certain statistical algorithm. It is basically related to the size of the data matrix. If there are 100 features (say 100 stocks from S &P) and 500 samples (500 daily return values of each stock), then the size of the data matrix will be 100 x 500 and dimensionality constant will be 100/500 = 1/5. This also means that in given 100-dimensional feature space, each basis feature has 500 samples located in that 100-dimensional space. The value of dimensionality constant can greatly affect the performance of any statistical tool. Most of the derived multivariate asymptotic results in statistics assume that dimensionality constant is close to zero (i.e. both number of features and number of samples are asymptotically large but the number of samples is much larger than the number of features). The most widely used methods like multivariate regression also perform well only under this condition. This simply means that it is important to have enough samples for each feature to accurately understand the role of that feature in regression or any other statistical setup.If the dimensionality constant is too large, it means that there are more features but samples per features are very less. In this case, commonly applied methods like covariance analysis, PCA, linear regression, etc. can give highly inaccurate results. There is a whole field dedicated to deriving results when enough samples per feature are not available. In fact, as the number of features (or dimensions) increases, the total number of samples required increases exponentially. This is also studied under the topic called the Curse of Dimensionality! This can be easily seen from the above image[1]which shows that as the dimension increasesl from 1D to 3D, more samples are required to explain the basic useful geometrical information.The best theoretical case will be that dimensionality constant is close to zero but this is not always good for real-world applications. For example, in case of financial data, if we will take many years of daily return (i.e. a large number of samples for each stock) then it can give misleading correlations and information. Many financial applications require to only consider recent data to avoid including bias from history. In such a case, dimensionality constant approaching to one can be better than zero. So, it is crucial to decide what is the desired number of samples per feature for the given application. So, sometimes right number (range) of samples are required to correctly model correlations among multivariate data (not too many and not too less).2. Normality of data: Most of the widely used ML algorithms including most famous ones like linear regression requires error (or noise) distribution to be Gaussian distributed. Have a look at following three histograms which represent the same data. The data[2] is the noise samples from underwater measurement in an urban water supply system pipeline (it was collected by me).The first image shows the histogram of noise samples with both x and y-axis in linear scale. At first glance, the data looks nearly gaussian. In the second image, I overlapped a Gaussian density curve (dotted red line) with the same mean and variance as the noise data. Now, it can be seen that data histogram is not perfectly Gaussian and there is a little deviation from gaussianity (blue curve different from the red curve). In the third image, I changed y-axis from linear to log scale. Now, it can be seen that there is a huge difference in the shape of the noise data histogram and gaussian distribution. The tails of the data are significantly diverging from the gaussian nature. It turns out that a much complex class of distributions known as alpha-stable distribution perfectly fits this noise histogram[3]. This is the case of heavy-tailed data distribution which is looking almost gaussian in linear scale but after superimposing gaussian curve and changing from linear to log scale, it turns out to be much different from a gaussian distribution. In fact, the heaviness of tails in the above case is quite huge and collecting more samples shows that the tails of the data distribution decay much slower than the Gaussian distribution even for very large values of noise amplitudes. This means that the probability of seeing outliers (outside gaussian curve) is significant even at very large noise amplitudes.Applying standard signal processing or ML algorithms which are optimally derived for gaussian nature of data can be suboptimal in this case. In fact, for heavy-tailed distributions, the most commonly used tools like covariance analysis, PCA, etc are ineffective because the data with heavy-tailed distribution (like Cauchy, alpha-stable, etc) have extremely high variance (theoretically infinite variance) which leads to undefined or highly unstable covariance.So, the common mistake here is to interpret any bell-like curve as gaussian which might be far from reality. The first step should be to use standard tests like Kolmogorov–Smirnov test to check the nature of the distribution. If the sample size is too small then even these tests are not reliable and if the sample size is too big (like in the above image, there are around 10 million samples) then these tests might take hours to give results. In such scenarios, small tricks like overlapping standard density curves on the empirical distribution or changing scales of the y-axis might give a better and quick approximation.3. Histogram, normalized histogram and probability density function (pdf): In any programming language, there are multiple options to select the “type” in the argument of histogram function. This is important. In the default setting, the y-axis of histogram simply represents “relative frequency” or “normalized count”, i.e. y-axis value for each bin represents “discrete probability mass” (occurrence of certain event in that bin width/total occurrences). This means that the y-axis values of all bins should give one on summation. This is also called relative frequency histogram. Another type of histogram is called probability density histogram in which the y-axis value of each bin gives “density” value rather than probability mass. This means y-axis value of each bin is equal to (discrete probability mass or normalized count)/bin width. For this type of histogram, the total area of all the histogram bars should sum to one. Finally, the last type is called probability density function (pdf) which is a continuous function and provide density at each value of a random variable (RV). The pdf usually have an analytical expression with few parameters to control its shape and properties. The total area under this function curve should integrate to be one.To sum up, the first type of histogram simply tells us how many incidences of event occurred inside a bin and this count is divided by total incidences to get normalized count. The second type goes one step further and divide the normalized count by bin width to get the probability density instead of probability mass. The last one is a theoretical continuous function and completely describes a RV (few exceptions).The biggest mistake made by the beginners is to overlap theoretical pdf over relative frequency histogram. An empirical histogram can be compared to the theoretical pdf only if the histogram is of “density” type rather than the normalized count type.Some other common mistakes are:4. Ignoring sampling error[4]5. Choosing the wrong Loss function[5]6. Correlation vs Causation[6]Footnotes[1] Escaping the Curse of Dimensionality[2] Measurement and Characterization of Acoustic Noise in Water Pipeline Channels[3] Measurement and Characterization of Acoustic Noise in Water Pipeline Channels[4] http://www.cs.cmu.edu/~tom/10601_sp08/slides/evaluation-2-13.pdf[5] 5 Regression Loss Functions All Machine Learners Should Know[6] Correlation is not causation
- Home >
- Catalog >
- Legal >
- Will And Trust Form >
- Sample Codicil To Will >
- sample codicil to change executor >
- Sample Will Pdf