A Stepwise Guide to Editing The Understanding Functional Dependencies Via Constraint Handling
Below you can get an idea about how to edit and complete a Understanding Functional Dependencies Via Constraint Handling hasslefree. Get started now.
- Push the“Get Form” Button below . Here you would be transferred into a webpage that allows you to make edits on the document.
- Choose a tool you need from the toolbar that shows up in the dashboard.
- After editing, double check and press the button Download.
- Don't hesistate to contact us via [email protected] For any concerns.
The Most Powerful Tool to Edit and Complete The Understanding Functional Dependencies Via Constraint Handling
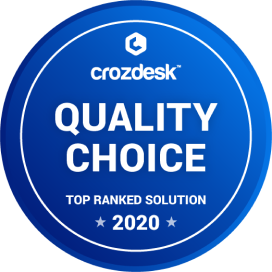
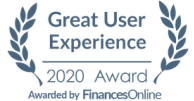
Edit Your Understanding Functional Dependencies Via Constraint Handling At Once
Get FormA Simple Manual to Edit Understanding Functional Dependencies Via Constraint Handling Online
Are you seeking to edit forms online? CocoDoc has got you covered with its detailed PDF toolset. You can accessIt simply by opening any web brower. The whole process is easy and convenient. Check below to find out
- go to the CocoDoc product page.
- Upload a document you want to edit by clicking Choose File or simply dragging or dropping.
- Conduct the desired edits on your document with the toolbar on the top of the dashboard.
- Download the file once it is finalized .
Steps in Editing Understanding Functional Dependencies Via Constraint Handling on Windows
It's to find a default application that can help make edits to a PDF document. Yet CocoDoc has come to your rescue. Take a look at the Handback below to find out possible approaches to edit PDF on your Windows system.
- Begin by obtaining CocoDoc application into your PC.
- Upload your PDF in the dashboard and make modifications on it with the toolbar listed above
- After double checking, download or save the document.
- There area also many other methods to edit PDF files, you can check this definitive guide
A Stepwise Guide in Editing a Understanding Functional Dependencies Via Constraint Handling on Mac
Thinking about how to edit PDF documents with your Mac? CocoDoc offers a wonderful solution for you.. It makes it possible for you you to edit documents in multiple ways. Get started now
- Install CocoDoc onto your Mac device or go to the CocoDoc website with a Mac browser. Select PDF file from your Mac device. You can do so by pressing the tab Choose File, or by dropping or dragging. Edit the PDF document in the new dashboard which includes a full set of PDF tools. Save the file by downloading.
A Complete Guide in Editing Understanding Functional Dependencies Via Constraint Handling on G Suite
Intergating G Suite with PDF services is marvellous progess in technology, with the power to cut your PDF editing process, making it easier and with high efficiency. Make use of CocoDoc's G Suite integration now.
Editing PDF on G Suite is as easy as it can be
- Visit Google WorkPlace Marketplace and search for CocoDoc
- install the CocoDoc add-on into your Google account. Now you can edit documents.
- Select a file desired by hitting the tab Choose File and start editing.
- After making all necessary edits, download it into your device.
PDF Editor FAQ
What are monads in functional programming and why are they useful? Are they a generic solution to the problem of state in FP or Haskell specific? Are they specific to Haskell or are they encountered in other FP languages?
There are a lot of monad tutorials out there. Too many, probably. I'm not terribly happy with most of the explanations though, so I'll throw my hat in too!The core problem, as I see it, is that the idea of a monad is very abstract. Trying to pin it down to a single concrete idea, a single analogy is doomed to fail.I've been thinking about how to explain abstract concepts like this for a while. To me, a good explanation has to have two parts: what it is and why we care. That is: a definition and examples. Far too often, I've found people leave out one or the other, but I need both to understand! If you just give me an abstract mathematical definition without context, I won't actually understand it, even if I can understand the parts. And if you only give me a bunch of examples but forget to explicitly define the idea, I might understand how it behaves, but I won't have any idea of what it actually is.So a good explanation has to have these two parts. The remaining question, then, is how to order them. Examples first, to motivate a definition? Start with the definition and "flesh it out" with examples? Somehow interleave the two?I've found that for me, the best approach is to open with a definition and expand on it. I won't understand the definition immediately, but I'll have it in the back of my mind for each example, and I'll refer back to it periodically.If you like the other order more, take a look at one of the best monad tutorials around: You Could Have Invented Monads! (And Maybe You Already Have.)So I'll introduce monads in two parts: first, I'll tell you what a monad is; next, I'll tell you why we care; finally, I'll walk through a few concrete examples.WhatSo what is a monad, at least in functional programming? Fundamentally, it's just a type m that has three particular functions defined on it. Note that m has to accept a type argument itself: you'd always use it as m Int, m String or m a. Then it just has to have some functions defined for it:return :: a -> m a fmap :: (a -> b) -> m a -> m b join :: m (m a) -> m a These functions also have to follow some laws. I usually don't think about these directly—it's enough to know that they relate to each other "reasonably"—so I'll talk about them later.The most important part is join, which captures a notion of "flattening" a structure. You go from a nested m (m a) to a single m a: you flatten out a level. If you want a single idea to hang onto, this is it: monads let you flatten them.As I alluded earlier, the definition probably seems dry and arbitrary right now. Don't worry about it. Just look back here as you're reading the rest of my post. Most of all, remember: a monad is just a type with some functions. That's all.WhySo why do we care about these particular functions? What's so special about them? How do they flow together? Why is the notion of "flattening" so important?The first two functions, return and fmap both serve a simple role: they allow us to transition from normal code a to code that uses m. return wraps a normal value, lifting it into the monad; fmap lifts normal functions to operate over these wrapped values. This becomes clearer when you add an extra set of parentheses to its type signature:fmap :: (a -> b) -> (m a -> m b) But what about flattening? That one's a bit less obvious.CompositionPerhaps the single most important idea in functional programming (or really all programming ever) is composition. And this is exactly what monads help with! In particular, the three functions let us use m as a customizable way to compose parts of our program, more versatile than just function composition.Normally, we just compose normal functions: given a -> b and b -> c, we can first apply one then the other to get a -> c. Simple but surprisingly useful.If a type m is a monad it means it gives us a new way to compose functions. It lets us compose functions of the form a -> m b: given a -> m b and b -> m c, we can get a -> m c. Note how the m in the middle gets swallowed up: this is the main difference with this form of composition.Since we have an instance of our m in the middle, we can insert different behavior as things get composed. This means that, for every different monad m, we get a new regime of composition.And this is exactly what join enables! join specifies a bit of computation that lets us "get rid" of an extra layer of m, and this is exactly what happens inside the composition. To compose two monadic functions like this, we start with fmap:f >=> g = \ x -> fmap g (f x) Try following along in GHCi, checking types as you go along.Remember that f :: (a -> m b) and g :: (b -> m c), which means that the type of the function above is:(>=>) :: (a -> m b) -> (b -> m c) -> (a -> m (m c)) We start with an a, pass it into f to get an m b and map over that to get an m (m c). And how do we go from this to the actual type we want? We need to flatten it!So we make the whole composition work properly and not have an extra m by throwing in a call to join:f >=> g = \ x -> join (fmap g (f x)) So: a monad gives us a new kind of "join-enabled composition", letting us customize what happens when things get composed by defining an appropriate join function.We can now view a -> m b as a special kind of function between a and b which just gets composed in a different way.BindSo, we have a new idea of composition: >=>. But we can actually have a new idea of function application too! This is called bind and is written as >>=. It's very useful for programming, but I find it harder to think about than join and >=>.What does this new sort of application mean? Well, it can't just be applying a -> m b to a normal a because we can already do that. Instead, it's applying a -> m b to an m a:(>>=) :: m a -> (a -> m b) -> m b In spirit, it's very similar to >=>: our new notion of application also "swallows" an intermediate m, so we can customize how it works.In fact, the definition is just like >=> but not wrapped in an extra lambda:x >>= f = join (fmap f x) This operator will come up in some of the practical examples.LawsNow, just before jumping into the examples, lets cover the monad laws. If you can't remember the details, don't worry: most of the time, how the laws need to apply in any given case is pretty intuitive.These laws are most easily described in terms of >=>:return >=> f = f f >=> return = f f >=> (g >=> h) = (f >=> g) >=> h In other words, return is both a right and a left identity for >=> and >=> is associative.Hey, these laws look very similar to how id and . (normal function composition) behave!'id . f = f f . id = f f . (g . h) = (f . g) . h This is just more evidence that monad provide a special kind of composition!ExamplesNow that we have the main idea, lets go on to see how it's used in practice with some examples!MaybePerhaps the simplest meaningful monad is Maybe, defined as follows:data Maybe a = Just a | Nothing Maybe wraps a normal type and also allows it to be null (ie Nothing): it's Haskell's option type.How do we make it a monad? Well, we know the three functions we need, so lets just implement those. We know that return just "wraps" a normal value in Maybe; the most natural way to do this is just Just (heh):return :: a -> Maybe a return x = Just x What about fmap? How do we apply a function to a Maybe value? Well, if we have a Just, we can just apply the function to its contents. But if we have Nothing, we don't have anything to apply the function to! We just have to return Nothing again:fmap :: (a -> b) -> Maybe a -> Maybe b fmap f (Just x) = Just (f x) fmap f Nothing = Nothing join is the final function. Here, we just have to consider all the possible cases and implement them in the most natural way:join :: Maybe (Maybe a) -> Maybe a join (Just (Just a)) = Just a join (Just Nothing) = Nothing join Nothing = Nothing Maybe is a nice example because, at every step, there was only one "reasonable" thing to do. In this case, "reasonable" meant not needlessly throwing away real values. As long as we keep values whenever we can, we only have one possible implementation of the monad functions.The next question to ask is "what does a -> Maybe b mean? It's a function that takes an a and may or may not return a b. It's a function that can fail.How do we compose two functions that can fail? Well, if they both succeed, they're just like normal functions. And if any one of them fails, if we ever get a Nothing well, we have no choice but to return Nothing for the whole thing.So Maybe gives us a type of composition and application for functions that can fail but propagating the failure through for you. It's very useful for avoiding deeply nested case expressions. We can transformcase f x of Nothing -> Nothing Just res -> case g res of Nothing -> Nothing Just res' -> case h res' of Nothing -> Nothing Just res'' -> ... into a much nicerx >>= f >>= g >>= h Maybe abstracts over function application and composition that automatically pipes possible Nothings through the whole computation, saving us from doing it manually and making the code much less noisy. It saves us from the equivalent ofif (x != null) { return ...; } else { return null; } EitherMaybe is great, but sometimes we want to fail in multiple different ways. This is where Either comes in: it's like Maybe but with the Nothing case annotated with an extra argument:data Either err a = Left err | Right a It uses the generic names Left and Right to show it isn't just about error handling, but we'll pretend it is.It actually forms a monad almost exactly like Maybe. If anything, it's easier: since we can't come up with a value of type err from thin air, we can only really implement these functions one way:return :: a -> Either err a return x = Right x fmap :: (a -> b) -> Either err a -> Either err b fmap f (Right x) = Right (f x) fmap f (Left err) = Left err join :: Either err (Either err a) -> Either err a join (Right (Right x)) = Right x join (Right (Left err)) = Left err join (Left err) = Left err In fact, Maybe can be seen as a special case of Either where the Left case doesn't carry extra information:type Maybe a = Either () a Either lets us abstract over error checking via return types. We can avoid the Go error-handling pattern:res, err = f(x) if err != nil { return nil } res2, err = g(res) if err != nil { return nil } res3, err = h(res) if err != nil { return nil } ... The moral equivalent of the above in Haskell could just be written asx >>= f >>= g >>= h even though they're both using return types to manage errors! Either abstracts over all the plumbing for us.ListSo far, we've seen how monads let us compose functions that might fail. What else can we do?Well, a simple one is the list type which is written as [a] in Haskell. We're going to approach lists with the same heuristic as for Maybe and Either: never throw away data unnecessarily. We also don't want to copy or rearrange data unnecessarily.With these conditions, return is pretty easy:return :: a -> [a] return x = [x] fmap, as the name implies, is just mapfmap :: (a -> b) -> [a] -> [b] fmap = map -- or fmap f [] = [] fmap f (x:xs) = f x : fmap f xs Finally, we have join which needs to flatten a list. Here's the most reasonable definition:join :: [[a]] -> [a] join [] = [] join (ls:rest) = ls ++ join rest Basically, we just take our list of lists and concatenate each of its items with ++.Now our next question: what does a -> [b] mean? It's a function that can return any number of results. In math, this is often called a nondeterministic function.What does it mean to compose non-deterministic functions like this? Well, to compose f :: a -> [b] with g :: b -> [c], we first pass in a value into f to get a bunch of bs, then we pass each b into g to get a whole bunch of cs and finally we return all of those cs. This is exactly what >=> for lists does.So the list monad gives us clean composition of functions that have any number of results. One cool thing to note is that this corresponds very closely to list comprehensions! In fact, we can rewrite a list comprehension in terms of return and >>= pretty easily:[f a b | a <- as, b <- bs] becomesbs >>= \ b -> as >>= \ a -> return (f a b) As you can see, this transformation is actually not list-specific at all. In fact, you can do this for any monad at all. Haskell's MonadComprehensions extension actually allows this: you can use list comprehension syntax for any monad at all, which is sometimes quite nice.ReaderNow lets look at a much trickier one. This is the function monad in the form of r -> a for some fixed r. (Just like Either err a had a fixed err.)What does return mean for this? Let's look at the type we want:return :: a -> (r -> a) We get a value and want to turn it into a function from r. Since we don't know anything particular about r, we can't do anything with that argument but ignore it:return x = (\ r -> x) How about fmap? Figuring it out on your own is actually a good exercise. Try it on your own before continuing.Again, we want to look at the type:fmap :: (a -> b) -> (r -> a) -> (r -> b) Hey, that type looks a familiar! It's just like function composition:(.) :: (b -> c) -> (a -> b) -> (a -> c) And, in fact, that's exactly what fmap is for (r -> a):fmap = (.) -- or fmap f g = \ x -> f (g x) Finally, we need join. Again, the type is going to be very helpful:join :: (r -> (r -> a)) -> (r -> a) We take in a function f with two arguments and need to use it to produce a function of one argument. Since we can't magically produce a value of type r, there is actually only one reasonable implementation of join:join f = \ x -> f x x So now we see that, indeed, (r -> a) is a monad. But what does this mean? We can think of value of type (r -> a) as values of type a in the context of r. That is, they're normal values of a that can also depend on a value of r. They can "read" from the environment, which is why (r -> a) is often called the "reader monad".The reader monad allows us to pipe this environment through a whole bunch of values and functions that all depend on it. The result of x >>= f >>= g >>= h lets us pass in a single value of r that is first given to x then passed into the result of f then the into the result of g and so on.WriterAnother type to look at is (w, a) for a fixed w. This might seem a little weird, but as we'll see it naturally forms a monad and can actually be pretty useful!So how do we do return? Immediately, we run into a problem: we would need to manufacture a value of type w, but we can't. So, in fact, we need to add a constraint to w: it has to be a type that has a special value of some sort to use with return. This is provided by the Monoid class which has mempty:mempty :: Monoid w => w We'll just use this; as we'll see later, the other part of the monoid will also come in useful.Given a free w value, return is straightforward:return :: Monoid w => a -> (w, a) return x = (mempty, x) Now fmap, which we can only really do in one way:fmap :: (a -> b) -> (w, a) -> (w, b) fmap f (w, x) = (w, f x) Finally, we need to do join:join :: (w, (w, a)) -> (w, a) We have *two* values of type w. We could just throw one away, but, as a rule, losing information unnecessarily is bad. So, instead, we will use the other part of Monoid:mappend :: Monoid w => w -> w -> w It's just an arbitrary way to combine two values of the type into a third. We can use it to turn the two levels of w in (w, (w, a)) into one:join (w_1, (w_2, x)) = (mappend w_1 w_2, x) So, as long as w is a Monoid, (w, a) is a monad. But what does this mean?It lets us string an extra channel of output through our whole computation, combining it using mappend at every step. It's often called the "writer monad" because we can "write" to this extra channel of output at every step. To be useful, we have to actually have an extra function for writing:tell :: Monoid w => w -> (w, ()) tell output = (output, ()) This lets us inject values into the output stream. This newly added output will be mappended onto the rest of the output from our computation.This is useful for purely functional logging. If we have a bunch of function f, g, h that all want to log some Strings in addition to returning something, we can write them by using tell ["Message"] and then automatically pipe all the strings through using the same code we've already seen a few times before:x >>= f' >>= g' >>= h' For lists (ie [String] in this example), mappend is just ++, so this expression becomes:(log ++ logF ++ logG ++ logH, (h (g (f x)))) (Where f, g and h are the function parts of f', g' and h' that only do the actual computation and not the logging.)It's pretty neat how we assembled the log in parallel to actually applying the base functions. And thanks to laziness, we will only evaluate as much of the log as we use: we aren't wasting too many resources on making a log we will never use!StateSo, we've seen how to compose functions that read and functions that write. Can we do both at once? Why, that would be a function that can both read and write! Sounds like mutable state.This is, in fact, exactly what the State type is: it's a combination of both reader and writer using the same type for both:type State s a = s -> (s, a) Conceptually, a value of type s -> (s, a) is an a that can depend on and/or modify a value of type s.Since we're always going to have a value of type s passed in—that's the s -> part of the type—we don't need the Monoid constraint any more.With that in mind, here are the monadic functions which are largely the combinations of their reader and writer versions. Note how return and fmap don't modify the state at all; only join can affect it.return :: a -> (s -> (s, a)) return x = \ s -> (s, x) fmap :: (a -> b) -> (s -> (s, a)) -> (s -> (s, b)) fmap f x = \ s -> let (s', a) = x s in (s', f a) -- remember that the stateful value is a function itself! join :: (s -> (s, (s -> (s, a)))) -> (s -> (s, a)) join x = \ s -> let (s', x') = x s in x' s' join is rather confusing, so take the time to work out what it does on paper. Basically, we start with a nested state function that depends on s twice. To turn this into a single level of state dependency, we have to take a value of type s and string it through both levels: that's all the join code is doing.We also need some primitive ways to access and modify the state, similar to tell. It's easiest to think about two of them: get to read in the current state and set to change it:get :: (s -> (s, s)) get = \ s -> (s, s) Note how get doesn't change the state at all. It just takes the state and moves it to the value "channel" as well, exposing it to the functions in the computation.set :: s -> (s -> (s, ())) set newS = \ s -> (newS, ()) set takes the new value of the state and creates a new stateful value. This value ignores the state that's passed into it, replacing it with the new state. We also don't have a meaningful result for this, so we just put a () into the result channel.We can combine get and set into some useful functions like modify:modify :: (s -> s) -> (s -> (s, ())) modify f = get >>= set . f -- or modify f = \ s -> (f s, ()) The state monad lets us compose stateful functions, and manages passing the state through each one automatically. Hopefully a pattern is now emerging: monads give us new ways of composing functions, usually with more structure.ProceduresNow we're going to talk about the only monad here that has any magic: IO. The idea is that IO a represents a procedure that, when run, produces a value of type a. The procedure has access to the runtime system, so it can do "extra-language" things like talk to the operating system, get input, print output and so on: all these are impossible to define in terms of just pure Haskell.Since IO is an abstract type, we don't know—and don't care—about how the monadic functions are implemented. Instead, I'll just talk about what they do.return :: a -> IO a creates the empty procedure that just does nothing except return the given value. This is essentially where its name comes from, coincidentally.fmap :: (a -> b) -> IO a -> IO b takes a procedure that gives us an IO a and creates a new one that first runs the IO a then passes its result into the function to get a b. Since this whole thing is still a procedure itself, it's an IO b: the b never "escapes" into normal code.Finally, we have join :: IO (IO a) -> IO a. This is a slightly odd way of sequencing procedures: we take in a procedure that returns a procedure and create one that runs both of them.Honestly, join does not make too much sense for IO. But >>= does: it's a way to apply procedures, as if they were functions! This lets us write complicated programs by combining these procedures in a systematic way to produce a single big procedure.In fact, this is how the entire Haskell program actually gets run. main :: IO a is just the big procedure; when you run a Haskell executable, the runtime system executes main, which likely involves both executing IO procedures inside main and evaluating normal Haskell expressions.Just like the writer and state monads, IO needs some primitive values like tell, get and set. But, unlike the earlier examples, it doesn't have one primitive value or two primitive values: it has hundreds. Every system call, every runtime functions are all primitive IO values. getLine, getChar, print... The monad machinery just lets us combine these primitive operations in different ways as well as letting us glue them together with normal (ie pure) Haskell code.Do-notationOne thing I haven't really mentioned is do-notation, which is some syntax sugar Haskell provides to make code using monads look more like an imperative program. It actually works like the list comprehension, but in reverse:do x <- xs y <- ys return (f x y) becomes xs >>= \ x -> ys >>= \ y -> return (f x y) It also allows you to ignore the value of an expression:do something somethingElse is the same assomething >>= \ _ -> somethingElse This turns every monad into an imperative-looking DSL. Once you understand the stuff I explained above, I think do-notation is quite easy to deal with.ConclusionThere are actually a whole bunch more monads I haven't talked about. We can use them for parsing, for logic programming, for mutable references, for callCC... Lots of interesting things.Moreover, we can actually combine monads. This gives rise to "monad transformers". For example, we can layer Maybe onto another monad by wrapping the inner monad's functions in checks for Nothing.Ultimately, just remember that a monad is a type with several functions defined on it. This type gives us a new way to compose and apply functions with custom behavior during the application/composition "step".I hope this gives you a good understanding of monads in practice. I know the answer's a bit long, but I've thought a lot about how to explain this idea. I just hope it wasn't too much! I think this is the longest Quora answer I've ever written, by a fair margin :).
What is the "best" mathematical tool for solving dynamic optimization problems?
I am not sure if I am understanding your question details correctly, but I am assuming that you have a differential equation, i.e.:[math]\dot{x}(t) = f(x(t),u(t))[/math]where the controls [math]u(t)[/math] represent your authority over the system (i.e., decision variables), and the states [math]x(t)[/math] represent system behavior, which you want to influence via [math]u(t)[/math] (with [math]t[/math] being time). If this is the case, this setting falls under the field of optimal control. You can consult these lecture notes on nonlinear and dynamic optimization, and also there are a lot of relevant material (lecture notes, slides, etc.) in this website of one of the leading groups in this field.Best mathematical tool to solve such problems depends, among other things, on the dynamics (i.e., the function [math]f(.)[/math] that describes how the system evolves over time) and constraints (if there are any). Dynamics are important because they appear in the dynamic optimization problem as equality constraints, thus dictating to a great extent how difficult the resulting problem will be.For example, if the dynamics are nonlinear, the resulting problem is guaranteed to be nonconvex due to the nonlinear equality constraints. If this is the case you need to use solvers that can handle nonconvex problems (e.g. IPOPT). If you use matlab or python, you can use the free casadi toolbox together with IPOPT to write high performance code quickly, for solving nonlinear optimal control problems. See example code here (you need casadi, and either python or matlab to use these).Another interesting case is when you have binary decision variables in addition to continuous ones. Then this is falls under the hybrid systems/hybrid control field, and demands the use of mixed integer programming (MIP) solvers, such as CPLEX or Gurobi. If this is the case, and you are using matlab, I’d recommend using the free YALMIP toolbox for optimization modeling, and call a good MIP solver via YALMIP. See an example with binary variables here.
Why are exponential families so awesome?
Yes, they are definitely awesome.But... why so awesome?Because it elegantly covers almost all distribution we’ve encountered, providing flexibility to apply them and contextualizing each as a natural case to handle certain problems. To make this clear, I’ll start in familiar territory - the normal distribution. I’ll show how the exponential family is secretly operating in the background and how, in fact, it’s flexible enough to handle a much wider range of problems.Before diving in, I'll confess that this is a long answer, but for good reason. If you can master the exponential family, you immediately understand a wide class of distributions. On a per minute-of-study basis, it's a worthwhile purchase.Let’s get started.What familiar territory?Let's say we've come across a list of continuous numbers that 'look' normally distributed. Our goal is to determine which distribution generated these numbers. That is, we speculate a distribution (normal in this case) and determine which parameters of that distribution make the most sense according to the data. 'Makes the most sense' translates to picking the maximum likelihood parameters.Now, before you say, 'just use the empirical mean/variance', let's think about exactly what we’re doing. We are concerned with finding the parameters [math]\mu[/math] and [math]\sigma^2[/math] that maximize the likelihood of our data. That is, find [math]\mu^*[/math] and [math]\sigma^{2*}[/math]:[math]\mu^*,\sigma^{2*} = \textrm{argmax}_{\mu,\sigma^2} \prod_i^N \mathcal{N}(x_i [/math][math][/math][math]| \mu,\sigma^2)[/math]The answer happens to be the empirical mean and variance, but that solution doesn't generalize, so forget it! Let's scan for values of [math]\mu[/math] and [math]\sigma^2[/math] until we find a combination that yields a distribution that looks like the histogram of our numbers, like this:(LL means log-likelihood, which is the log of the thing we’re optimizing).Looks like we found a good fit (see the red). Easy right? Great, now I'm going to do the exact same thing, but I'll rewrite some of the algebra:Let’s relabel:You'd agree that if knew [math]\theta_1[/math] and [math]\theta_2[/math], I'd know [math]\mu[/math] and [math]\sigma^2[/math], right? So let's reason in terms of those variables - instead of searching for [math]\mu[/math] and [math]\sigma^2[/math], let's look for [math]\theta_1[/math] and [math]\theta_2:[/math]Why did we just do this silly rewrite? Because nearly every distribution we've heard of can be re-worked into this form. In other words, we can do the equivalent of finding [math]\mu^*[/math] and [math]\sigma^{2*}[/math], but for a huge range of distributions.So what is the fully general, fully awesome exponential family?In the exponential family, the probability of a vector [math]\mathbf{x}[/math] according to a parameter vector [math]\boldsymbol{\theta}[/math] is:[math]Z(\boldsymbol{\theta})[/math] is called the partition function and it's there to ensure that [math]p(\mathbf{x}|\boldsymbol{\theta})[/math] sums to 1 over [math]\mathbf{x}[/math]. That is:[math]\nu(d\mathbf{x})[/math] refers to the 'measure' of [math]\mathbf{x}[/math]. It's there to generalize the idea of 'summing over all possible events' to the correct domain (discrete, continuous or subsets of either). So when we say we 'know' [math]\nu(d\mathbf{x})[/math], that means we know how to sum over all possible [math]\mathbf{x}[/math]'s appropriately. It also may determine the 'volume' of [math]\mathbf{x}[/math], though we can get that work done with [math]h(\mathbf{x})[/math].In fact, let's make that separation and say that's what [math]h(\mathbf{x})[/math] is - it's the volume of [math]\mathbf{x}[/math]. Think of this as the component of [math]\mathbf{x}[/math]'s likelihood that isn't due to it's parameters. This will become more clear with an example.[math]T(\mathbf{x})[/math] is called the 'vector of sufficient statistics'. This is a measurement of our data that indicates all that our parameters care about when determining likelihood. In other words, if two vectors have the same sufficient statistics, then all the other ways in which they may be different do not change their likelihood in the eyes of the parameters. [1]We need to cover a few extra details regarding the parameters:We'll only consider the space of parameters for which the partition function is finite. If we look at the partition function, it's quite easy to imagine an integral that diverges. This space of 'legal' parameters is called the natural parameter space.There should be no linear dependencies between the parameters (or sufficient statistics) in this representation, meaning we should be free to move all elements of [math]\boldsymbol{\theta}[/math]. Said differently, knowing one subset of parameters should never fix the others. If this is true, the representation is said to be minimal. There are a two reasons for this. First, we never lose any ability to represent distributions by enforcing it. Second, since we will ultimately be searching over the space of [math]\boldsymbol{\theta}[/math], we will have the benefit that different [math]\boldsymbol{\theta}[/math]'s always imply different distributions.That last point will guide how we should think: The length of [math]\boldsymbol{\theta}[/math] will determine how many degrees of freedom we have over our probability distributions. So when we determine [math]T(\mathbf{x})[/math], we need to think carefully first about its length.Is there anything helpful yet?Before diving into examples, we can immediately notice one thing useful. In this form, think about how the probability of a sample combines to form the probability of all our data:Look! That relabeling shows that the probability of all our data is just a new distribution in the exponential family. Easy!Since determining the MLE will involve maximizing the log of this, call that [math]\mathcal{L}_N(\boldsymbol{\theta})[/math]. That is:… but how does this relate to the normal distribution?So when we fitted our normal distribution, we were quietly making choices with respect to the exponential family form. That is, we were making assertions that implied specific settings to our exponential family. Those assertions were:1. [math]x[/math] could be any real valued number: that sets [math]\nu(dx)[/math] which determines how we'll do our integration. That is, we'll integrate over the real line.2. It's normally distributed, which dictates a few things:It has two degrees of freedom, so that tells us the length of [math]T(x)[/math] and [math]\boldsymbol{\theta}[/math].[math]T(x) = [x,x^2][/math], which means probabilities are influenced by parameters only via a linear relationship with these measurements.[math]h(x) = 1[/math], which means that the difference in likelihood between two [math]x[/math] values is due entirely to the parameters. In other words, all [math]x[/math]'s have the same size.From here, the definition of the exponential family will dictate the rest. That is:Now that we have this, we can determine our objective [math]\mathcal{L}_N(\boldsymbol{\theta})[/math]:Now picking the best [math]\theta_1[/math] and [math]\theta_2[/math] (maximizing [math]\mathcal{L}_N(\boldsymbol{\theta})[/math]) will be the exact same procedure we did earlier.What's so useful about the generalization?The reason for this rephrasing is it reveals all the remarkable degrees of freedom the exponential family rewards us. We didn't need to say [math]x[/math] was real valued - that was our choice. We didn't need to say the sufficient statistics were [math][x,x^2][/math], we could have picked anything! So let's use that flexibility in another example. Let's say I come across data like this:Hmm, these aren't numbers.. Let’s charge forward anyway, resolving the choices of the exponential family:1. [math]\nu(dx)[/math] should mean we sum over the two possible events ([math]x=A[/math] or [math]x=B[/math]).2. I suspect only one degree of freedom is appropriate here. With that, I suggest we use an indicator function:which can be represented as [math]\mathbb{1}[x=A].[/math]3. Outside of something that relates to the parameters, I have no reason to think [math]x=A[/math] has a greater volume than [math]x=B[/math] (or visa versa), so let's say [math]h(x)=1[/math].We've made all our choices - What does this imply about [math]Z(\boldsymbol{\theta})[/math]?And now we can write [math]p(x|\theta)[/math]:Or, written out more explicitly:Since we can choose [math]\theta[/math] to yield any value in [math][0,1][/math] for [math]p(x=A|\theta)[/math], we see this distributions just assigns a constant probability to the events [math]x=A[/math] and [math]x=B[/math] - in other words, we’ve landed on the Bernoulli distribution!So to pick the most likely parameters, we optimize [math]\mathcal{L}_N(\theta)[/math], just like we did in the case of the normal. Here, there is a simple analytic solution (just set the derivative to zero and see what falls out).So one set of choices led us directly to the normal distribution while another set led us to the Bernoulli distribution.*Yawn*... Try something harder!What if we came across data like this:Well this is odd. Now [math]\mathbf{x}[/math] is a length 3 vector and it's got this unusual constraint where the elements sum to 10. Ok, deep breath - let's start from our familiar spot:1. How should we think about [math]\nu(d\mathbf{x})[/math]? How should we think about all possible events that we should sum over? Said differently, what are the legal observations we could make for this data? Well, it's any 3 nonnegative integers such that their sum is 10. Those are:So I just need to sum over all these. Let's call this set [math]\mathcal{X}[/math].2. How many degrees of freedom should our modeling allow? Hmm, I suspect there'll be a parameter for each column, but the constraint that their sum is 10 will subtract one, so let’s say there are 2 degrees of freedom.3. What is [math]T(\mathbf{x})[/math]? The most natural thing I can think of is the data itself, so let's go with the first two elements of [math]\mathbf{x}[/math]. We aren't losing information regarding the last element, since our distribution will also 'know' that the sum is 10.4. What is [math]h(\mathbf{x})[/math]? In other words, should one observation of [math]\mathbf{x}[/math] ever be considered more likely than another, regardless of the parameters? From this angle, I have no clue, but it's not simple enough for me to say [math]h(\mathbf{x})=1[/math]. To crystallize our understanding, let's think about how we would generate [math]\mathbf{x}[/math] ourselves. One way is to make 10 draws (from some distribution I don't yet know) and then aggregate the results. So, for example, [math]AABBCABABC \implies [4,4,2][/math]. The useful thing here is that all possible sequences will map to all events of [math]\mathcal{X}[/math]. From this angle, do some observations seem more likely then others, even though we can't reference the distribution that generates a single draw? Well, some observations are mapped to by more sequences than others. For example, the only thing that maps to [math][10,0,0][/math] is [math]AA \cdots A[/math] while [math][9,1,0][/math] is mapped to by 10 sequences ([math]BA\cdots A,AB\cdots A,\cdots, AA\cdots B[/math]). So it seems the latter observation is 10 times bigger than the former. Notice this is true despite not knowing the parameters. So let's make [math]h(\mathbf{x})[/math] the number of sequences that map to [math]\mathbf{x}[/math]. If we remember some combinatorics, that’s not too hard:Ok, we've made all our choices so we should be good to go. The partition function is this guy:If you're wondering how I got from the second line to the third, the answer is... I don't know. I just, from an entirely separate arena, happen to know that's true. So, now we may write the expression for the probability:At this point, we have everything we need to optimize [math]\mathcal{L}_N(\boldsymbol{\theta})[/math]. But if you're feeling uneasy with this rather unusual form that's followed from our rather usual choices, I can tell you something relaxing. This is actually identical to this form:where [math]\theta[/math] has been rewritten in terms of probabilities [math]p_1[/math], [math]p_2[/math] and [math]p_3[/math]. These probabilities refer to the chances of a particular draw when we were generating our sequences earlier. In other words, this was just the multinomial distribution.Ok, but what else?These examples should communicate the flexibility of the exponential family. To cover more ground with fewer words, I'll just show you a taste of the diversity of distributions given by different choices of [math]h(\mathbf{x})[/math] and [math]T(\mathbf{x})[/math]. From the wiki page:Whoa! That sample is almost a college semester worth of distributions - and we can handle any of them! All we need to do is turn the dials on this exponential machine.Any other insights?There is some intuition that remains to be had. When we 'pick the most appropriate [math]\boldsymbol{\theta}[/math]', we were maximizing this guy:(which we called [math]\mathcal{L}_N(\boldsymbol{\theta})[/math]). Up until this point, we have been optimizing this rather blindly. But in reality, the first step is to compute the gradient, so let's start there:We got this. Let's figure out [math]\frac{\partial \log Z(\boldsymbol{\theta})}{\partial \boldsymbol{\theta}}[/math]:It's a bit sneaky, but the integrand just became a vector (due to multiplication by [math]T(\mathbf{x})[/math]). If we look closely, it's just a probability weighted average of [math]T(\mathbf{x})[/math]. In other words, it's the expectation of [math]T(\mathbf{x})[/math] under the parameters [math]\boldsymbol{\theta}[/math]. That is:Stare at that. How wild is that?! A derivative, a log and normalizer on the left and on the right… the expectation of our sufficient statistics? Did not see that coming! [2]Back to the gradient: A rescaled version (scaling doesn't matter) is:Awesome! - we have this super general optimization problem and out pops this very short, very intuitive expression for the gradient. The gradient tells us what direction (in the space of [math]\boldsymbol{\theta}[/math]'s) to move. This says 'move in the direction that reduces the biggest differences between our observed sufficient statistics and those expected under the current choice of [math]\boldsymbol{\theta}[/math]’.Another way to see this is that at the maximum, we know the gradient is zero. So when we found our best parameters, those parameters will dictate an expectation of sufficient statistics that are equal to our averaged observed sufficient statistics. Nice and natural!What's the catch?This, unfortunately, isn't a cure all solution. There's a catch - the normalizer [math]Z(\boldsymbol{\theta})[/math]. The difficulty is it's a summation over [math]\mathcal{X}[/math], which can be exponentially large. For example, think about our multinomial problem. If we had just 10 categories and they summed to 40, we already have over a billion elements in [math]\mathcal{X}[/math]. In other circumstances, this issue can be much worse.Footnotes[1] If you’re unsatisfied with this explanation, I don’t blame you. Sufficient statistics have a definition that would distract from the meat of this post.[2] You can take this a step further and discover the Hessian matrix (higher dimensional analog of the second derivative) is the covariance matrix of [math]T(\mathbf{x})[/math]. Wild.Sources[1] Kevin Murphy's Machine Learning: A Probabilistic Perspective (chapter 9)[2] This pdf floating around online, which I suspect is by the demigod Michael I Jordan.[3] The wiki page and the army of smart people who organized it. Wikipedia, just like the exponential family, you're awesome.
- Home >
- Catalog >
- Business >
- Agreement Template >
- Cohabitation Agreement >
- Understanding Functional Dependencies Via Constraint Handling