How to Edit and fill out Pathways To The Future Referral Form Online
Read the following instructions to use CocoDoc to start editing and signing your Pathways To The Future Referral Form:
- At first, seek the “Get Form” button and press it.
- Wait until Pathways To The Future Referral Form is appeared.
- Customize your document by using the toolbar on the top.
- Download your completed form and share it as you needed.
An Easy-to-Use Editing Tool for Modifying Pathways To The Future Referral Form on Your Way
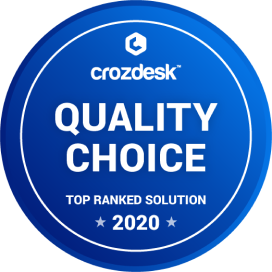
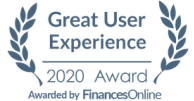
How to Edit Your PDF Pathways To The Future Referral Form Online
Editing your form online is quite effortless. You don't need to download any software through your computer or phone to use this feature. CocoDoc offers an easy tool to edit your document directly through any web browser you use. The entire interface is well-organized.
Follow the step-by-step guide below to eidt your PDF files online:
- Search CocoDoc official website from any web browser of the device where you have your file.
- Seek the ‘Edit PDF Online’ icon and press it.
- Then you will browse this cool page. Just drag and drop the document, or attach the file through the ‘Choose File’ option.
- Once the document is uploaded, you can edit it using the toolbar as you needed.
- When the modification is finished, tap the ‘Download’ option to save the file.
How to Edit Pathways To The Future Referral Form on Windows
Windows is the most widely-used operating system. However, Windows does not contain any default application that can directly edit template. In this case, you can download CocoDoc's desktop software for Windows, which can help you to work on documents effectively.
All you have to do is follow the instructions below:
- Download CocoDoc software from your Windows Store.
- Open the software and then select your PDF document.
- You can also upload the PDF file from Google Drive.
- After that, edit the document as you needed by using the varied tools on the top.
- Once done, you can now save the completed paper to your cloud storage. You can also check more details about how to edit PDFs.
How to Edit Pathways To The Future Referral Form on Mac
macOS comes with a default feature - Preview, to open PDF files. Although Mac users can view PDF files and even mark text on it, it does not support editing. Thanks to CocoDoc, you can edit your document on Mac instantly.
Follow the effortless guidelines below to start editing:
- To begin with, install CocoDoc desktop app on your Mac computer.
- Then, select your PDF file through the app.
- You can select the template from any cloud storage, such as Dropbox, Google Drive, or OneDrive.
- Edit, fill and sign your file by utilizing this amazing tool.
- Lastly, download the template to save it on your device.
How to Edit PDF Pathways To The Future Referral Form with G Suite
G Suite is a widely-used Google's suite of intelligent apps, which is designed to make your job easier and increase collaboration across departments. Integrating CocoDoc's PDF file editor with G Suite can help to accomplish work easily.
Here are the instructions to do it:
- Open Google WorkPlace Marketplace on your laptop.
- Search for CocoDoc PDF Editor and get the add-on.
- Select the template that you want to edit and find CocoDoc PDF Editor by selecting "Open with" in Drive.
- Edit and sign your file using the toolbar.
- Save the completed PDF file on your cloud storage.
PDF Editor FAQ
The saying goes "build a better mousetrap and the world will beat a path to your door". What 'better' thing would you like to see invented?
Hello David,It wouldn’t be a possibility or positive use of my energy to be building a mousetrap for any reason. They should be footloose and fancy free just as the in the famous animation film known as “RATATOUILLE”.“Having said that I do have some creative ways of building patterns and pathways of light and vibrant attractive energy. That naturally attracts like-kinds, or highly energetic individuals. I’m not interested in trapping anything nor any other human being. Freedom to come, to go, to have the potential of sharing experiences with someone of mutually enhanced-embedded- codes. Codes of wisdom, friendship, compassion, humility, intelligence and one of life’s best feelings or experiences, one of shared happiness.So venturing forward to build what I deem to be another doorway to open, or pathway to choose, I will set up a few different types of scenarios.”Scenario ~ ONE(seven fictional scenarios in total)“A man who is a hard working pilot, traveling the globe inside of a nonstop gateway. He decides to settle down yet he hasn’t built any bridges inside of the world of interpersonal relationships. He’s a wee bit shy and awkward.How does he find a life partner and solid new pathways?He keeps doing what he knows best with one exception. He cuts back his flying hours, just the same as he was in a long-standing committed relationship. Next he refills his time with new adventures that he loves. Finds groups of individuals that share a passionate desire for experiences of mutual enjoyment. This process could take five or more years to literally reap the benefits. It’s an investment of the best kind. An investment of your own personal experience.”There can be no gain without change.Scenario ~ TWO“You’re the CEO of a flourishing technology company. After a ten year stretch it’s become a monotone. Also, even though you already have a 15% stake in the company you’re ready for a type of advancement, one that provides a complete startup experience.Without damaging what you have already built how do you proceed?I’d say slowly, carefully and thoughtfully. Doing so is ‘artful’ because you will need new talent. Accessing those that you already know within your own organization is a no-no. Why? Because starting fresh is important.So you use all forms of media and social media platforms to engage people for a think-tank. As that think-tank evolves your startup has a life. This is not setting up a mousetrap, it’s a creative liaison of free-thinkers. They could be paid or not.Sharing brings forth an abundance of concepts you may not have ever initiated yourself. My analysis during this process, would move rapidly as long as you’ve linked the right human energies with a viable concept. Now off for funding.The bee hive is born, honey to be extracted.”Senario ~ THREE“You’re the sixteen son of aboriginal parents. Your mother died during your birth and your father raised you. He was a tracker and a spiritually endowed King of your tribe.During his ceremonial burial you become despondent. Your only memory of a similar feeling was when you understood that you would never share a meal with your mother. You’d not have her embraces nor her feminine energies. Once completely dependent upon your father, now alone.Because of your fathers ability to provide for you, you learned English, French and Spanish. People would come from different cultures and countries to experience the outback of Australia.Weeks turn into months. The tribesmen of your village offer you sustenance and a unpaid position. You also have a second cousin living in Perth who has offered you a place to stay while you figure out what to do. You choose the latter.Luckily you secure a job tending gardens. As your just existing in a survival mode you decide to reinvent yourself. Taking the historical assets of your own life you create a unique form of tourism. That includes setting up a hostel with the villagers wher you grew up. This takes time and energy, elements already freely in existence by your village but little in the way of funds.Because of your language skills you’re able to connect with all sorts of interesting people of international lifestyles. You now dedicate yourself to understanding the WWW. This takes about six months. Then onto free social media platforms where you can announce your villages hostel and your own services. You’re now almost eighteen.All you still have is your own self. You’re on your way. There will be peaks and valleys however in a short period of time you’ve become a brand new version of you. You have built an entrepreneurial mousetrap. A beautiful one at that!”Senario ~ FOUR“One of many daughters and sons of a Chinese mogul. You father has seven wives all living in a luxurious imprisonments. You’re value is next to zero. Raised within all sort of inhuman activities, you eventually emerge as a ‘very basic’ educated adult woman. You’ve had twelve years of standardized education. Fortunately you excel in mathematics. Your other talent being a statistical visionary.Your brothers are all endowed with significant businesses, funding and properties. They take after their father in almost every aspect.Eventually, you escape China with your fathers third wife, (your mother) and two sisters. The first thing you do establish residency in Canada. You have only what you, your mother and sisters have accumulated over your lifetimes to date.You go to college(working full time) while achieving several scholarships you get an offer from Harvard Business School. There you complete your Masters. Next you move to California on another scholarship program supplied by San Francisco State University, where you acquire your PhD in International Corporate Finance. Working for different law firms in their accounting departments. Next you set up a small accounting firm.This becomes popular via word of mouth, referrals because of your genius. It becomes a financial honey-trap. By forty you’re living the lifestyle very similar to the one you originated from. You overcame the odds of being an Asian women and leaving home with nothing but your immediate family. This is how to invent oneself. This is how entrepreneurial thinkers and mostly those who are the ‘doers’ in life become successful, independent individuals.Self investment inside of true daily investments.”Senario ~ FIVE“A beautiful young woman from India, of low economic status, is able to qualify for medical school. Early during her internment she is raped after returning home late one evening from public transportation. It’s the only way that she’s able to get back and forth from the countryside. Normally leaving home at 05:45 and returning by 20:35.This woman dusts herself off under a group of trees. Returns home doing her best to cover her soiled clothing and battered body. She confides in no one, not her mother, grandmother or great- grandparents, nor her best and livelong friend.After a quick wash up in a communal bowl, she empties the contents and refills it. Since she used the extra water she knows that before anyone else awakens in the morning. This means that she must go to the local water pump in the darkness and refill what has been used in order to keep her situation a secret.Finally at midnight she makes her way to the bedroom, a small space that she’s shared with her two other sisterssince birth. In the darkness she relives her evenings waking nightmare. By four in the morning she’s up and on her way to the villages water. Each step is filled with fear. She completes her first task of the day and if off to school early.The weeks pass and turn into months as her belly grows. By the sixth month she has to tell her family of the situation. They don’t believe her. They shun her. She’s thrown out of the house.Most of us can well imagine all of different things that take place after this major, life changing event has taken place. Sharing a room with ten other women from her school she works right up to the day of her delivery. She has a baby boy. The moment she see his face she loves him.Determined to have a way to provide for herself and son she eventually completes her schooling and begins her internship which supplies a meager wage. She’s emotionally supported by her small community of friendships.She becomes a full fledged physician. Her secret being visible, yet the facts still not known. She has achieved the unlikely accomplishment of relative financial success in her life. Her wound is deep but gradually healed as she enjoys life with her companion and only son.Another story about reinventing oneself.”Senario ~ SIX“A young woman is longing for a change. Her life seems beautiful at times and otherwise terribly limited. She daughter of a long line of Quakers. Her favorite parts of her life are the animals and families that greet her daily. It’s just not enough.She makes plans to go and live with her second cousin in New York. Her family knows. They explain all of the ramifications of doing so. She’s determined.Arriving at the railway station her cousin greets her with her husband. Her cousin is a seamstress and her husband is a policeman. She acclimates. She integrates. She almost immediately becomes successful at making homemade pies and selling them.As the years pass she marries. Has a successful business of her own, four children. Two of sons go to live with her remaining Quaker family in Pennsylvania, they adapt and love the lifestyle. Her other two sons stay. One becomes a firefighter and the other a becomes a security guard and eventually opens his own business. They all marry and have children.This is called genetic reinvention. A highly regular and recommended societal advancement, one of which is laden with changes intertwined with one’s own history. All returning to a slice of homemade pie.”Senario ~ SEVEN“Living in the Congo on the continent of Africa is so seldom an easy experience for all of humanity, especially for the children and certainly women in particular. It’s a rarity to see any women live an extraordinary lifestyle. One of the few ways this can happen is because of their natural physical beauty.This story begins with a woman who is named Ah-Oh-Goo. Born in a place on the Congo River called Kinshasa which is located on the lower south-eastern part of that provenance. Her life is surrounded by poverty, sickness and hardships.By the age of ten shows a rare type of physical beauty. She’s seen by French foreigners from Paris. They purchase her from her family and go about the process of a legal adoption.Upon arrival in France she’s now eleven years old. Home schooled and eventually etiquette managed. Photographed immediately. By the age of twelve doing fashion shows and works as an artists model. Ah-Oh-Goo is now know by her new french name Bella which means beautiful. Her french parents make sure to save her earnings for her future.Bella becomes so popular by the age of sixteen she has the opportunity to get a New York agent and moves with her adoptive mother to the United States. Soon she becomes an overnight success so-to-speak, by her eighteenth birthday.Was it Bella’s choice to become a model? Most likely we would never know. I wonder how many Bella’s are in existence today?Another type of quasi-manufactured form of human invention. Modeling for the fashion industry and commerce.Thank you so much for reading my stories!Aster
How do you make an ML model?
Let’s change the question on how we develop a Machine Learning project?IntroductionArtificial Intelligence (AI) and Machine Learning (ML) as an approach to AI, are all over the place. Anyone is interested to know what are they and how to use them. It is becoming a race between stakeholders to comprehend and make use of AI. Some people think Machine Learning is the panacea (the cure to everything)! BUT NOT SO FAST!What is the problem in adopting Machine Learning?Today, many businesses are craving the proper knowledge to develop applications that apply Machine Learning. The majority of the business owners believe Machine Learning influences their commerce drastically and positively. However, many companies have reasonable doubts about the business impact of Machine Learning projects. Due to the black-box nature of the Machine Learning, the stakeholders usually question the advances presented in Machine Learning projects and discard projects when the actual deployment does not meet the expectations. So, the problem is how to define, implement, deploy, and measure the impact of a Machine Learning project?What makes the problem a problem?The problem mentioned above requires proper planning and assessment. The proper planning, deployment, and evaluation are themselves complicated meandering processes. As we design the plan for our Machine Learning project, it’s essential to examine some emerging best practices and use-cases. This step puts our Machine Learning projects in an industrial context so that we can recognize, quantify, and maintain the business influence of the project. It is also vital to understand, manage, and alleviate the associated risks of a deployed complex Machine Learning system. We must frame and take the steps mentioned above in an organized manner. This effort demands a lot of attention and clarification.What is our approach?It is advantageous to showcase the bigger picture, to show how the numerous steps of a successful Machine Learning project are connected, and what is the order? In this article, we present the roadmap to a flourishing Machine Learning project. This roadmap also aims to help to improve the interaction between the Machine Learning experts and the business stakeholders, who are financially accountable for the expenses and profits of the system. Here, you will learn the following:The steps to plan, implement and deploy a Machine Learning projectThe approach to justify a Machine Learning project in a business setting by recognizing and evaluating its expected benefitsAddressing the common issues faced in Machine Learning projectsThe pathway to control and manage a deployed Machine Learning projectWho cares and gets benefit out of this article?It is essential to know who the audience of this article are? If you are in one of the below categories, then you may find this article useful:A developer looking for a roadmap to start.A Machine Learning expert that want to refresh your mindA manager trying to gain a broad knowledge of what needs to be done in a Machine Learning project and what are the missing elements?A business stakeholder trying to assess a project and you are interested to understand the big picture.An educator trying to spread the knowledge.Project Planning in Machine LearningWhy project planning?First, let’s explain what we are speaking about. Many people believe the project plan is the project schedule with some elements: The schedule of duties and dates that needs to be done. This schedule is a portion of the project plan, but not the whole thing. Project planning includes anything that is associated with project success. It’s the process which aims to build the actions needed for project development such as defining objectives, illuminate the scope of the work, and the critical activities. Any Machine Learning project requires project planning, as well. You wonder why? It is simple: It is a project!What is the task, feasibility and the requirements?A critical step is to define “what needs to be done precisely”? We simply need to know the inputs and outputs in the beginning.The overall architecture of a system. We first need to know what we want from the sytem by identifying the input and the desired output.Then we need to ask the following questions to clarify the feasibility and requirements:Data acquisition feasibilityHow complicated is it to obtain data?Should we perform a data labeling operation?How much data do we need?Is there privacy issues or any risk involved?PerformanceHow accurate the model should be?How fast the system should be?What concerns should be satisfied? Privacy maybe?Background researchWhat kind of similar projects or practices are available?Is the topic justified?Who are the competitors?Technical aspectsDo we have the necessary manpower?Do we have the computational resources?What are the setbacks in algorithm development?Are there any technical aspects that can be done in parallel?What needs to be delivered in terms of expectations?Define what needs to be explicitly delivered. This should be defined based on what is expected from the project. Examples are as follows:An accuracy equal or greater than 90%Privacy considerations needs a risk no greater than 0.01.A retrieval rate of 95%It is important to know that no all the times we can have a precise value regarding the deliverables.Data Matters the MostWhy we collect data and what are the methods?Data collection is a significant bottleneck in Machine Learning and an ongoing research problem in various scientific societies. There are mainly two causes that demonstrate data collection necessity. First, as Machine Learning is overgrowing, researchers encounter numerous distinct applications without enough labeled data. Second, unlike traditional Machine Learning based on feature engineering, powerful data-driven techniques (deep learning) demand vast amounts of data. Interestingly, the management community, and not the Machine Learning community, originates the recent developments in data collection advancements due to the setbacks in handling large amounts of data. Due to the needs mentioned above, data collection is a crucial element to develop any effort built upon Machine Learning.There are mainly three approaches to data collection. First, if the purpose is to explore new datasets, the data acquisition methods can be utilized to identify, expand, or produce datasets. Second, if the datasets are available, data labeling methods can be adapted to label data samples. Finally, instead of labeling new datasets, improving existing data, or its usage might be desired. The three techniques mentioned above can be used together. For example, a developer might be researching new data labeling methods in addition to searching for new datasets.Data preparationStakeholders, nowadays, usually utilize Machine Learning for supervised learning for which labeled training data is required. The growth of deep learning based models demonstrates this hunger for data as they often require massive amounts of training data. This new trend raises the issues of data security and privacy. Furthermore, the advent of further regulations like General Data Protection Regulation (GDPR), and the importance of managing risk add to the great importance of data management.In data preparation, we essentially prepare the data for being ingested by the machine. In case of supervised learning, we MUST associate data instances to their corresponding labels as required by supervised training. Next, the data should be split into the training and testing sets. The training set supposed to be a representative of the real-world application.Companies are usually interested in augmenting the available data sets with new data to potentially improve existing Machine Learning models. There is a developing interest in exploring alternative ways to prepare data as well (such as synthetic data generation). There are important questions about data that business associates are usually asking themselves:1. How exactly can we augment the existing data?2. Do we need external data sources for data augmentation?3. Can new decentralization technologies (such as blockchain) can come to our rescue for having secure data exchange pipeline?Data integrationIt is critical to determine the data feature representation of the machine input. Despite amazing deep learning performance regarding the on-the-fly data feature learning, it is still imperative to put special attention to how the data is represented to the machine. As for now, the Machine Learning algorithms’ precision strongly depends on how the input representation. Typically, we transform the input data into feature vectors. Debates continue regarding the feature space size. As a rule of thumb, we should avoid an input feature space which is too large due to the curse of dimensionality; however, it should contain enough information necessary for predictive analysis.Data usually comes from a variety of sources before being used in Machine Learning models. As data matters the most in Machine Learning, determining how to design, develop, and manage robust data pipelines is vital. It is critical to decide on the data feature representation of the machine input. Despite amazing deep learning performance regarding the on-the-fly data feature learning, it is still imperative to put special attention to how the data is represented to the machine. This requirement is apart from having clean labeled data. Even in the unsupervised task (for which there is no need for labeled data), data preparation and processing is a crucial element.Over the last decade, many business stakeholders have started introducing (especially for public sale) data platforms for business intelligence and business analytics. More recently, companies have begun to move toward platforms with support for open source Machine Learning libraries, project management, and model evaluation and visualization.Let's Search, Find, and Pick a Model Before Creating One!Now it’s the time to choose or model. Remember, re-inventing the wheel is usually the last step, not the first one!Start with something simple!We may have heard this expression before. It is truly a golden rule to start with any project. Assume we want to develop a sophisticated Machine Learning project. It is daunting to even think about the numerous elements of the project that we have no clue about. However, a critical point is this: Complicated things are made of many simple parts! That’s all! By knowing this fact, we should start breaking the project into its simplest elements. Then, we begin to perform a simple task as a part of whole.The first model presents the most significant aid to the project development and the final product it is not necessary to make it complicated. In fact, in the initial stages, we just learn by doing. Henceforth, a lot of things may change in the middle of the way. So, do NOT be picky! There are things that needs to be determined first, that helps the future integration of the developed parts:Implement a simple working exampleSelecting the simplest optimization objectiveWhat is the emaning of good or bad in terms of system outcome?How to integrate the simple elements to build a more complex component?Deteremine if the components of the project can be integrated in the final product or not?After the aforementioned steps, now we have a clear high-level understanding of different elements of the project and we can dig more into the practical aspects.Set and define the baselines modelsBaselines are beneficial for determining a lower bound of demanded performance as well as the desired performance level. The are some approaches to set the baseline model:(1) The first important element is to examine the literature to find a baseline based on the available models for similar tasks/datasets.(2) Using off-the-shelf libraries and their implemented models. We can recall Scikit-Learn as one of the well-known Machine Learning libraries.(3) Research the real-world application to find acceptable performance level. It is worth understanding how well human can perform for the task at hand. There are areas in which the AI is already outperforms human such as image and object recognition, video games, and speech and lipreading technology.Gain knowledge by reproducing the ready-to-used modelsIf we are utilizing a known model, we must ensure that the model’s performance on a benchmark dataset [Understanding the Purpose and Use of Benchmarking, Benchmarking] makes sense by comparing it with the reported results in the literature. Thanks to the open source platforms such as GitHub, nowadays, we can find the implementation of the majority of known models available and ready-to-use.The term benchmarking is utilized in Machine Learning as a referral to the evaluation and comparison of methods and algorithms regarding their capability to learn content in ‘benchmark’ datasets that are considered to be the ‘standards’ for their specific characteristics. Benchmarking could be considered as a sanity check to validate if a new method is able to reliably find simple patterns that existing methods are known to identify. In another word, benchmarking is the approach to identify the respective strengths and weaknesses of a given methodology in contrast with others.Benchmark datasets typically take one of three forms:The first is accessible, well-studied real-world data, taken from different real-world problem domains of interest.The second is simulated data, or data that has been artificially generated, often to ‘look’ like real-world data, but with known, underlying patterns.Third form is the toy data, which we will define here as data that is also artificially generated with a known embedded pattern but without an emphasis on representing real-world data.EvaluationThe goal of model evaluation is to determine if it will perform a reasonable job of predicting the target on new unseen data. As real-world samples have unknown target values, we should evaluate the accuracy of the Machine Learning model using data with already known target values (test set). With the assumption that we used a proper test set (the very first condition is that the model never saw any of the test set samples in the training phase), this predictive analysis can showcase the model efficiency.After the training is finished, we send the model the test observations for with known target values (ground truth). Then the model makes the predictions and compare them versus the known target value. Ultimately, we measure a metric as the indication of the model effectiveness to match between the predicted and ground truth values.Generally speaking, an effective evaluation usually contains the following elements:A prediction accuracy metric to show the overall effectiveness of the modelVisualizations of the model efficiencyInvestigating the impact of different model parametersConfirming the validity of the evaluation using various methods such as benchmarking, etc.Rethink about the ModelAssuming, after performing all the previous steps, running the model, debugging, and evaluation, we have a model set and operating at a decent or even under-optimized level. Now, it the time to rethink the model and try to make it better. There are many approaches to model refinement. Model refinement, in general, refers to guide specialists to enhance the performance and robustness of a Machine Learning models. Model refinement include many different considerations. We address some of the most important ones below.How data is affecting the model development?Do we need to investigate the affect of data on the model? The short answer is yes, Always! It is very important to investigate the utilized data once again. This step aims to help us understand if (1) we need more data, (2) different kind of data, (3) reformat the data, and many more. In more details, the following aspects needs further attention:Did we use sufficient data for the evaluation of the model? If the number of records in the evaluation data is much smaller than the ones used in training data, then we are using too limited data for evaluation. As a rule of thumb, usually, the portion of the test/train samples is [latex]\frac{1}{4}[/latex], respectively.Do we have the same data class distribution for the training and evaluation sets? It is crucial to investigate the distribution of the training and evaluation data attributes to validate the similarly in both data sources. As an example, assume we have a binary classification for which we have "apple" and "banana" images as the two classes (attributes here). The machine should classify the test images as one of the two categories. If we train the model with a target distribution different from the evaluation data, then the machine tends to make the decisions based on the training data statistics with very different statistics compared to the evaluation set. Getting back to our example, assume 50% of the training data consists of "apply" images, and only 10% of evaluation data have "apple" images. In this setup, the evaluation quality suffers.Are we really using the best model?This is a million dollar question? Usually, we can never find the best model due to many reasons. To count a few: (1) There are numerous models there. We simply don’t have time to investigate them all!, (2) A model by itself may have a lot of hyperparameters. It is hard to tune and optimize them, (3) Regarding the task at hand; we may not have the necessary skilled human resources to research and suggest the top picks. However, there are some grounds to investigate which may bring more insight.Change the model capacityA suboptimal model capacity can cause overfitting or underfitting. Hurdles such as overfitting and underfitting are associated with the capacity of a Machine Learning model to build related information based on a set of training examples. Underfitting refers to the inability of a Machine Learning algorithm to understand and gather enough solid knowledge from the training data for generalization to the test data [lack of capacity]. On the other hand, overfitting happens when a Machine Learning model relies too much on the specific or general knowledge in the training data and fails to generalize and utilize its knowledge for the new unseen test queries [overly allocated capacity]. The question is how to understand we are encountering overfitting or underfitting?We usually evaluate the performance of a Machine Learning model based on the following two factors: (1) The Training Error and (2 ) the difference between the training and test errors. In the general case, underfitting has happened when factor (1) cannot converge to get an acceptable level. On the other hand, overfitting occurred when the training loss converges (factor (1) is satisfied) but the testing error stuck and is not behaving decreasing manner ( factor (2) has not been satisfied). In the case of underfitting, the model does not have enough capacity to learn, and in the case of overfitting, the model is absorbing too much non-informative information.In this experiment, the test loss (orange) is decreasing along with the training loss (blue). In this case, we have a balance with neither overfitting nor underfitting.How about researching deep to find a more advanced model?It is becoming tough to stay up to date with recent progress happening in machine learning. Day by day, we see fantastic innovations with new applications. However, most of these progressions are concealed inside a large number of research articles and technical documents. It is essential to dig deep into the new research to see if we can find a better model doing what we desire to do.Model Management and MaintenanceSometimes we think it’s done! But not so fast! Deploying a Machine Learning model is not merely equivalent to establish and evaluate the model. The entire lifecycle, including model deployment and operations, should be continuously managed. Without sufficient maintenance, Machine Learning models are prone to decay. This deterioration in predictive ability emerges when by the change of environmental conditions. The chance of model decay raises when a Machine Learning model’s performance has not been monitored for a while. There are specific matters that can affect a machine learning model’s performance which need special attention.Data dependency in Machine LearningThis is serious because even “enhancements” in input data may have harmful effects on the Machine Learning system because it might be trained using the previous data, which may differ from the new data in many characteristics. For example, assume a model is trained using image data, which has ten different classes. Augmenting the data with new classes results in the trained machine failing to classify new classes for which the machine does not have any knowledge.Behavior changesOne of the essential features of deployed Machine Learning systems is that they usually change their behavior as they update. It is hard to anticipate before actually utilizing the machine in the real world. Assuming a system performance is bound to another system output. For example, a scam detector aims to find the scam emails generated by another intelligent bot. What if the fake scam generator becomes stronger through time and we do not monitor the behavior of the detector?SummaryThis study set out to determine and address different elements of a successful Machine Learning project. We described how to frame, implement, evaluate, and manage a Machine Learning project. The evidence from this study suggests that the steps to conduct a Machine Learning project are not finalized. We have always had to get back to previous stages and make refinements and modifications.Finally, a number of important limitations need to be considered. First, the current study has only examined the big picture and some general advice regarding each of the Machine Learning project elements and ignore the technical details which are beyond the scope of this article. Second, the current research was not explicitly designed to describe evaluation metrics related to the assessment of a Machine Learning project. The evaluation details by itself need a lot of elaboration, and we will address that in future articles. Finally, further research should, therefore, concentrate on the investigation of how to manage a deployed Machine Learning project.Original Post: How to Frame, Organize and Manage a Competitive Machine Learning Project?
How can I invest $100?
3 Ways To Invest $100 And Turn It Into $1.4 Million In Under 2 YearsAll great wealth starts somewhere. Investment and compounding money are the well established pathway's to wealth. All exponential money generation is responsible for the wealth people have. In this article we explore 3 ways to invest $100To compound your money passively is one thing and you will get a certain result. Usually very low. To invest $100 in the traditional investment vehicles which offer returns of 5% to 10% would almost be futile. At 10% investing $100 you would have to wait 100 years to have a total of $1.4 million dollars.If you had to wait 100 years at 10% you would probably say to yourself....well....I cant take it with me. What is interesting is that when we do these calculation's to look at compounding interest, which is getting paid interest upon interest. We forget that it is not the years that matter but the number of multiplication's. Essentially, we can look at the above example and ask...why do those 100 multiplications have to be years? Why can't they be week's? Or day's?The problem becomes interesting. Investing $100 to make $1.4 million dollar's in 100 years is unworkable. But to make $1.4 million in 100 week's or just 2 years, now it gets kinda exciting doesn't it?When we forget about banks and traditional investment vehicle's we start to think, well, how CAN I invest $100 and end up with $110 by the end of the week? Because, if I can do that, there is nothing to stop me from making $1.4 million dollars in 2 years. The reason why is because the proportional increase is the same...it is always 10% so no matter what level you are at, $100 or $100,000 you are still after that same proportion or 10%Another way to look at it, is what if we could raise the compounder? What if instead of 10% we manufacture 20% per week. That's just $20 in the first week, in the second week it's $22 and so on. This does sound do-able.Actually it is very do-able. Think outside the square and develop a strategy that can make you $10 from investing $100 If you can do that, you have a unit of value in the form of an investment vehicle that could conceivably make you $1.4 million in 2 years or less.Essentially you want to look at business model's that are automated or semi-automated and that is a big clue. Because if it isn't automated you must start from scratch every week. But if it is automated, then it becomes passive and that means you can add to it every week. So the one big answer that I can give you is look at the Internet. The Internet's very nature is that it is automated. It's like one big vending machine and all the user's do all the work themselves and serve themselves.So here are 3 way's you can consider.EbayYou can look at purchasing thing's for under value and resell them for 10% percent more after all cost's. In this way you can progressively trade up and up from cars to land to houses to businesses.Article'sBuy 300 word articles for $5 and submit them to article directories on the Internet. You leave a link in the article going to a vendors site that you are an affiliate of. Say 100 articles bring in $1000 of commission's that means your return is 100% So with your $100 you buy 20 article's which give you a return of $200, then you buy 40 more article's that give you $400 and so on.AdvertisingAdvertise the products of a vendor you are an affiliate of. Google Adwords, MSN adcenter, and Yahoo advertising all provide a way to place a small ad in their search results. So when someone searches for "blue widget" and you sell blue widget's you will be right there and get the referral commission. If you spend $100 on advertising and get a return of 50% you can easily compound that money into $1.4 million dollars by adding to your advertising and finding more and more products to represent.If you need money now, like I mean in the next hour, try what I did. I am making more money now than in my old business and you can too, read the amazing, true story, in the link below. When I joined I was skeptical for just ten seconds before I realized what this was. I was smiling from ear to ear and you will too.Imagine doubling your money every week with no or little risk! To discover a verified list of Million Dollar Corporations offering you their products at 75% commission to you. Click the link below to learn HOW you will begin compounding your capital towards your first Million Dollars at the easy corporate money program.Source : http://www.mocxie.com/wealthbuilding/3-Ways-To-Invest-100-dollars-And-Turn-It-Into-1-4-Million-dollars-In-Under-2-Years.php
- Home >
- Catalog >
- Business >
- Report Template >
- Status Report Template >
- Weekly Project Status Report Template >
- one page project status report template >
- Pathways To The Future Referral Form