How to Edit Your Presentation Feedback Online On the Fly
Follow the step-by-step guide to get your Presentation Feedback edited with ease:
- Hit the Get Form button on this page.
- You will go to our PDF editor.
- Make some changes to your document, like adding text, inserting images, and other tools in the top toolbar.
- Hit the Download button and download your all-set document into you local computer.
We Are Proud of Letting You Edit Presentation Feedback Seamlessly
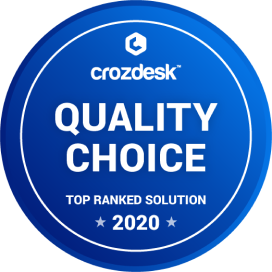
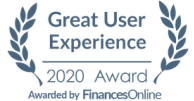
How to Edit Your Presentation Feedback Online
If you need to sign a document, you may need to add text, complete the date, and do other editing. CocoDoc makes it very easy to edit your form just in your browser. Let's see the simple steps to go.
- Hit the Get Form button on this page.
- You will go to this PDF file editor web app.
- When the editor appears, click the tool icon in the top toolbar to edit your form, like adding text box and crossing.
- To add date, click the Date icon, hold and drag the generated date to the target place.
- Change the default date by changing the default to another date in the box.
- Click OK to save your edits and click the Download button for sending a copy.
How to Edit Text for Your Presentation Feedback with Adobe DC on Windows
Adobe DC on Windows is a useful tool to edit your file on a PC. This is especially useful when you have need about file edit in your local environment. So, let'get started.
- Click the Adobe DC app on Windows.
- Find and click the Edit PDF tool.
- Click the Select a File button and select a file from you computer.
- Click a text box to edit the text font, size, and other formats.
- Select File > Save or File > Save As to confirm the edit to your Presentation Feedback.
How to Edit Your Presentation Feedback With Adobe Dc on Mac
- Select a file on you computer and Open it with the Adobe DC for Mac.
- Navigate to and click Edit PDF from the right position.
- Edit your form as needed by selecting the tool from the top toolbar.
- Click the Fill & Sign tool and select the Sign icon in the top toolbar to customize your signature in different ways.
- Select File > Save to save the changed file.
How to Edit your Presentation Feedback from G Suite with CocoDoc
Like using G Suite for your work to complete a form? You can integrate your PDF editing work in Google Drive with CocoDoc, so you can fill out your PDF just in your favorite workspace.
- Go to Google Workspace Marketplace, search and install CocoDoc for Google Drive add-on.
- Go to the Drive, find and right click the form and select Open With.
- Select the CocoDoc PDF option, and allow your Google account to integrate into CocoDoc in the popup windows.
- Choose the PDF Editor option to open the CocoDoc PDF editor.
- Click the tool in the top toolbar to edit your Presentation Feedback on the target field, like signing and adding text.
- Click the Download button to save your form.
PDF Editor FAQ
What are the most common topics omitted from machine learning courses?
That was exactly the question I tried to answer in a presentation I gave some months ago (see slides here http://www.slideshare.net/xamat/10-lessons-learned-from-building-machine-learning-systems). The premise was that there are many great books to learn ML, but hardly any of them tell you about important issues that you can only learn after years "in the job". To summarize, here are some of the practical topics I came up with:When does it pay off to increase your data, when does it pay off to increase your model complexity, and when does it pay off to do both.How to be smart about reducing data size and dimensionalityDefining your problem in terms of good training and testing data is sometimes half of the solutionMost real ML systems are subject to presentation feedback bias. Dealing with it though navigational models or explore/exploit strategies is usually a topic that you won't see in most courses.Many times, the most important thing to solve your ML-related problem is to have the right evaluation approach and metric. There is very little time devoted to this in most ML courses.Pretty often ML algorithms need to be implemented in a distributed/parallelized fashion. I don't think this is dealt with in most courses.Hyperparameter optimization, perhaps with Bayesian Optimization or Multi-armed bandit approaches is seldom discussed.Most practical ML systems need to do part of their computations offline and others online. Learning how to design algorithms that can deal with both scenarios is important and seldom taught.I know there are some courses that might mention related issues, but given how important I have found some of those issues to be, I wish they would get more attention.
What does the concept of presentation-feedback bias refer to in the context of machine learning?
Presentation (feedback) bias refers to an effect that is present in any user-facing machine learning system. Roughly speaking, presentation bias is derived from the fact that we can only receive user feedback on items that have been presented to the user. Even in those that are shown, the probability of receiving user feedback is also affected by where the item is shown. This is a subclass of presentation bias known as "position bias".Presentation bias has been known to affect machine learning systems and how user preferences are gathered (see for example this publication: Presentation bias is significant in determining user preference for search results-A user study).The simplest way to address the issue, without altering the machine learning model itself, is to implement some form of "presentation discounting system" (see this recent publication by LinkedIn researchers: Page on cs.ubc.ca). However, there have been many other approaches to account for this effect. For example, in the "Competitive Collaborative Filtering" approach (see Page on eeshyang.com), they include the effect of the "competition between items" in a Collaborative Filtering setting.Another way to address the problem is by directly affect the presentation by introducing some alteration. The easiest approach is to introduce some form of randomness. There are approaches to do this with minimal effect on the user (see Page on cornell.edu). A better, and more informed, approach is to use some form of multi-armed bandits method to tradeoff exploitation of the model with explorations of items that otherwise would not get shown (Page on cornell.edu). Of particular interest is the so-called Thompson Sampling (see Recommendations with Thompson Sampling for example).Another important issue to keep in mind when dealing with the presentation bias in a machine learning setting is its effect on the selection of negative examples for the model. For example, if negative examples are only taken from items that are presented (and not chosen by the user), we will be biasing our model against items that are potentially much better than those that were not shown in the first place.I don't have an example of a machine learning system "gone-wrong" because of this, but you can imagine that there are many ML applications out there that are not taking into account any of this.
What are some Quantified Self apps beyond health?
We created Quantified Impressions to analyze, benchmark, and track how well you present yourself. We provide personalized presentation feedback and analytics to enterprises, executives, and individuals.
- Home >
- Catalog >
- Miscellaneous >
- Evaluation Form >
- Training Evaluation Form >
- Sample Training Evaluation Form >
- training feedback form for trainees >
- Presentation Feedback