How to Edit and draw up Form Phd Dissertation Harvard University Online
Read the following instructions to use CocoDoc to start editing and finalizing your Form Phd Dissertation Harvard University:
- First of all, direct to the “Get Form” button and tap it.
- Wait until Form Phd Dissertation Harvard University is ready to use.
- Customize your document by using the toolbar on the top.
- Download your finished form and share it as you needed.
The Easiest Editing Tool for Modifying Form Phd Dissertation Harvard University on Your Way
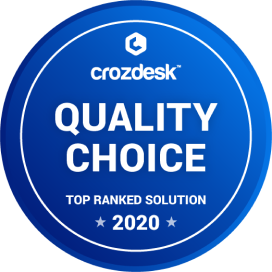
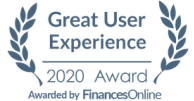
How to Edit Your PDF Form Phd Dissertation Harvard University Online
Editing your form online is quite effortless. There is no need to get any software via your computer or phone to use this feature. CocoDoc offers an easy tool to edit your document directly through any web browser you use. The entire interface is well-organized.
Follow the step-by-step guide below to eidt your PDF files online:
- Browse CocoDoc official website on your computer where you have your file.
- Seek the ‘Edit PDF Online’ button and tap it.
- Then you will open this free tool page. Just drag and drop the PDF, or choose the file through the ‘Choose File’ option.
- Once the document is uploaded, you can edit it using the toolbar as you needed.
- When the modification is completed, click on the ‘Download’ icon to save the file.
How to Edit Form Phd Dissertation Harvard University on Windows
Windows is the most conventional operating system. However, Windows does not contain any default application that can directly edit PDF. In this case, you can get CocoDoc's desktop software for Windows, which can help you to work on documents efficiently.
All you have to do is follow the steps below:
- Install CocoDoc software from your Windows Store.
- Open the software and then attach your PDF document.
- You can also attach the PDF file from URL.
- After that, edit the document as you needed by using the various tools on the top.
- Once done, you can now save the finished template to your cloud storage. You can also check more details about how can you edit a PDF.
How to Edit Form Phd Dissertation Harvard University on Mac
macOS comes with a default feature - Preview, to open PDF files. Although Mac users can view PDF files and even mark text on it, it does not support editing. Utilizing CocoDoc, you can edit your document on Mac quickly.
Follow the effortless steps below to start editing:
- To get started, install CocoDoc desktop app on your Mac computer.
- Then, attach your PDF file through the app.
- You can upload the PDF from any cloud storage, such as Dropbox, Google Drive, or OneDrive.
- Edit, fill and sign your template by utilizing some online tools.
- Lastly, download the PDF to save it on your device.
How to Edit PDF Form Phd Dissertation Harvard University through G Suite
G Suite is a conventional Google's suite of intelligent apps, which is designed to make your work more efficiently and increase collaboration across departments. Integrating CocoDoc's PDF document editor with G Suite can help to accomplish work handily.
Here are the steps to do it:
- Open Google WorkPlace Marketplace on your laptop.
- Look for CocoDoc PDF Editor and install the add-on.
- Upload the PDF that you want to edit and find CocoDoc PDF Editor by clicking "Open with" in Drive.
- Edit and sign your template using the toolbar.
- Save the finished PDF file on your laptop.
PDF Editor FAQ
Why do some schools offer classes and degrees in Government while other schools offer Political Science?
Hello Jessica:Why universities and colleges choose to name their programs either Political Science, or Government has more to do with their traditions than it does with the curriculums in those programs.Let me share my experience:I began my PhD program at UC Berkeley, and transferred to the Claremont Graduate University. I transferred because my Dissertation Chairman took a Sweet Heart Professorial job at Claremont and asked me (begged me, actually) to follow him and be his research assistant.UC Berkeley calls their program, Political Science. Claremont calls theirs, Government.My Chairman got his PhD at Stanford, where the same program is called Political Science. At Claremont, my Dissertation Committee consisted of 4 Profs, including my Chairman. Two of my Committee members got their PhDs at Harvard, where it’s called Government. My 4th Committee member got his PhD at Yale where the same program is called Political Science.One of my Committee members whose PhD was in Government from Harvard taught Political Science at Pomona College in the Claremont complex, and served on PhD Dissertation Committees at the Claremont Graduate University where, as noted above, it’s called Government.The course work I studied at UC Berkeley was in Political Science. When I transferred to Claremont, I continued this course work, but there, as noted previously, it’s called Government.In the U.S., the National Association that Profs who teach in this field belong to is called, The American Political Science Association (APSA). It’s not called, The American Government Association (AGA).Personally, I don’t see a dime’s worth of difference between the study of Political Science and the study of Government.As a student, whether you are in a Political Science program, or a Government program, you will study power relationships within society, i.e., who gets what and how much, when do they get it, how do they get it, and where do they get it.You will study the structure of the state, and the legal and constitutional framework of the state. You will study how elections are managed, and the rules governing how political parties are organized, and how they gain power.As well, the foundation of it all, foundation behind it, whether its called Political Science or Government, is Political Theory (some call it Political Philosophy).Political Theory/Political Philosophy is the core thinking and the core writing that’s occurred over thousands of years about how societies organize and manage themselves, or, contrarily, how they are organized and managed.The former societies fall under the heading of Self-Ruled where the People in the Society are Sovereign and in charge. The latter governments are categorized as Ruled by where the people are not sovereign and are not in charge. Rather, in the Ruled by category, the people are ruled by an authoritarian or dictatorial system of government.In conclusion, as I said above, there’s no difference in the course work, or in the programs between Political Science, and Government.To reiterate, why universities and colleges choose one name over the other has more to do with their traditions than it does with their curriculums.NOTE: The Claremont Complex consists of 5 independent Undergraduate Colleges, and two PhD and Masters degree granting Graduate Universities. Also, each school in the complex has it’s own Administration, Board of Directors, and Faculty. Undergraduate students may take classes from any of the Undergrad Colleges, though their Bachelor’s Degree is awarded to them by the Undergraduate College in which they’re enrolled. Graduate students may form their PhD Dissertation Committees with Profs from any of the Undergrad Colleges, though their Dissertation Chairman must be from the Graduate University in which they’re enrolled. It’s a model patterned after Oxford University in England. As for rankings, Forbes, and US News & World Report consistently rank the 5 Undergrad Colleges in the top tier of U.S. Undergrad Colleges. U.S. News & World Report evaluates and ranks each PhD program at the Graduate University separately. In their ranking, the PhD program in Government ranks in the top tier PhD Political Science/Government programs in the U.S. That said, my wife says I should have stayed at Berkeley. I remind her that we wouldn’t have met if I had. Besides, compared to Claremont, the weather in Berkeley is miserable. Also, the mountains behind Claremont are spectacular, and snow capped in the winter. Downhill snow-skiing is an hour away. I spent many hours on the nearby slopes.Semper Fi,JE-PhD—Political Science (Political Theory, Economics, History, Mathematics)“Old Corps, New Corps, Same Corps”
Which are the top 20 papers related to AI (both machine learning & symbolic), so that I can cover the basics and choose a niche for my research?
Ah, the sort of challenging question that I like to ponder about on an otherwise lazy Saturday morning in the San Francisco Bay Area! I began my career in AI as a young Master’s student in Indian Institute of Technology, Kanpur, actually enrolled as a EE major, but got enchanted by Hofstadter’s Godel, Escher and Bach book into studying AI. That was 1982, so I’ve been working in AI and ML for the past 36 years. Along the way, I’ve read, oh, easily about 10,000 papers or so, give or take a few hundred. So, among these thousands of papers, now, I have to pick the “top 20 papers”, so that you, the interested Quora reader, can get a glimpse of what attracts someone like me to give up everything in pursuit of this possibly idealized quest to make machines as smart as humans and other animals. Now, there’s a challenge I can't resist.OK, any list like this is going to be 1) hopelessly biased by my personal choices 2) not entirely representative of modern AI. 3) a VERY long read! Remember that a lot of us who got into AI in the late 1970s or early 80s did so far before there was any commercial hope that AI would pay off. We were drawn to the scientific quest underlying AI: how to build a theory that explain how the brain works, how the mind was the result of the brain etc. None of us had any clue, it is safe to say, that in the early 21st century, AI would become a hugely profitable venture.But, I’m going to argue that now more than ever, it is vitally important for those entering the AI field to understand 1) where did the ideas for AI come from 2) that insights into the brain come from many fields, from neuroscience and biology to psychology and economics and from mathematics as well, so my choice of papers reflects that, and I’ve chosen papers from multiple academic fields. I’ve also not shied away from papers that are critical of things that you might believe in deeply (e.g., the power of statistical machine learning to solve potentially any AI problem).I’ll try to pepper the list with my chatty commentary as well, so it’s not going to be one of those all too boring “here’s 20 things you should know about blah”, which is all too often what you see on the web. But, with my commentary, this is going to be a really long reply. What I want to give you a glimpse of is the panoply of fascinating characters who made up this interdisciplinary quest to understand brains from a scientific and computational point of view, how diverse their backgrounds were, and what an amazingly accomplished set of minds they were. It is to their credit that AI has come along as quickly as it has, barely 60 years since it began. Without such a dazzling collection of minds working on the problem, we would have probably taken much longer to make any real progress.The list is somewhat historical and arranged chronologically as far as it is possible. I’ve also tried to keep in mind that the point of this list is that it should be comprehensible to a newbie entering the field of AI, so much as I like to include some heavy hitting math papers (of which I’ve selected a couple), I’ve included a few very sophisticated highly technical papers, since you need to get a sense of what AI is in the 21st century. So, the readability of this top 20 list varies widely: some papers are easy to get through in a Sunday afternoon. Others — well, let’s say that you’ll need several weeks of concentrated reading to make headway, assuming you have the math background. But, there are not many of the latter, so don't worry about not having the right background (yet).Let’s begin, as they say, at the beginning…..A logical calculus of the ideas immanent in nervous activity, by Warren McCullough and Walter Pitts (Univ. of Chicago), vol. 5, pp. 115–133, 1943. (http://www.cs.cmu.edu/~./epxing/Class/10715/reading/McCulloch.and.Pitts.pdf). This is the first great paper of modern computational neuroscience, written by two brilliant researchers, one senior and distinguished (McCullough), the other, Pitts, a dazzling prodigy who had had no education of any sort, but talked his way into a position with McCullough. Pitts grew up in inner city Detroit, and because he was mercilessly beaten up by gang members who were older than him, he took refuge in the Detroit Public Library. It is rumored he devoured all 1000+ pages of Bertrand Russell and Alfred North Whitehead’s Principia Mathematica in one marathon reading session of several days and nights. This is not easy reading — it is a dense logical summary of much of modern math. Pitts was brave enough, even through he was barely in high school and had had no education, to audaciously write to Bertrand Russell in England, then a famous literary figure would go on to win the Nobel Prize in literature, as well as a great mathematician, pointing out a few errors and typos in the magnum opus. This young boy so impressed Russell that later he wrote him a glowing recommendation to work with Warren McCullough. Thus was born a great collaboration, and both moved shortly to MIT, where they came under the influence of none other than Norbert Wiener, wunderkind mathematician who invented the term “cybernetics” (the study of AI in man and machines). McCullough was a larger than life character who worked all night, and seemingly subsisted on diet of “Irish whiskey and ice cream”. Pitts wrote a dazzlingly beautiful PhD thesis on “three-dimensional neural nets”, and then, as a tragic Italian opera would have it, everything fell apart. Wiener and McCullough had a falling out (so petty was the reason that I will not repeat it here), and thus, McCullough stopped being actively working with Pitt, and Pitt sort of just faded away, but sadly, not before burning the only copy of his unpublished PhD dissertation before he defended it. No copy has yet been found of this work. Read the tragic story here — warning: keep a box of Kleenex handy for at the end, you will cry! — The Man Who Tried to Redeem the World with Logic - Issue 21: Information - Nautilus (read also the classic paper “What the Frog’s Eye tells the Frog’s Brain” https://hearingbrain.org/docs/letvin_ieee_1959.pdf by the same duo. A great modern paper is the recent breakthrough in biology at Caltech where the facial code used in primate brains to identify faces has finally been cracked — The Code for Facial Identity in the Primate Brain. — showing that in one narrow area, we may know what the human eye is telling the human brain, almost 60 years after McCullough and Pitts asked the question).Steps towards Artificial Intelligence, Marvin Minsky, Proceedings of the IRE, January 1960 (http://worrydream.com/refs/Minsky%20-%20Steps%20Toward%20Artificial%20Intelligence.pdf). Many date the beginning of AI formally to this article, which really outlined the division of AI into different subfields, many of which are still around, so this paper really can be said to have been the first to lay out the modern field of AI in its current guise. Minksy was a prodigy who did a PhD in math at Princeton (like many others in AI currently and in the past), and after a dazzling postdoc at Harvard as a Fellow (where he did early work in robotics), started the highly influential MIT AI Lab, which he presided over for a number of decades. He was a larger than life character, and those who knew him well had a large stock of stories about him. Among the best I’ve heard is one where he was interviewing a faculty candidate — a rather nervous young PhD who was excitedly explaining his work on the blackboard — when the student turned around, he discovered he was alone in his office. Minksy had disappeared during his explanation. The student was mortified, but Minksy later explained that what the student had told him sounded so interesting that Minksy had to step outside and take a walk to think the ideas over. Minsky was a polymath, at home in theoretical computer science where wrote some influential papers and a book, in psychology where he was an avid disciple of Freud and wrote a paper on AI and jokes and what it meant about the subconscious, in education where he pioneered new educational learning technology, and many other fields.Programs with Common Sense, John McCarthy, in Minsky, Semantic Information Processing, pp. 403–418, 1968. (http://www-formal.stanford.edu/jmc/mcc59.ps) McCarthy was the other principal founder of AI, who after a short period of working at MIT, left to found the Stanford AI Lab, which in due course proved to be just as influential as its East coast cousin. McCarthy above all was a strong believer in the power of knowledge, and in the need for formal representations of knowledge. In this influential paper, he articulates his ideas for a software system called “an Advice Taker”, which can be instructed to do a task using hints. The Advice Taker is also endowed with common sense, and can deduce obvious conclusions from the advice given to it. For example, a self-driving car can be given the official rules of the road, as well as some advice about how humans drive (such as “in general, humans do not follow the speed limit on most highways, but tend to drive 5–10 miles above the speed limit”). Critical to McCarthy’s conceptualization, it would not be sufficient to have a neural net learn the driving task. Knowledge had to represented explicitly so it could be reasoned about. He says something profound in the paper, which may shock most modern ML researchers. He says in page 4 in italics (emphasis) that “In order for a program to be capable of learning something it must first be capable of being told it”! By this definition, McCarthy would not view most deep learning systems as really doing “learning” (for none of the deep learning systems can be told what they learn). McCarthy was also famous for his work on lambda calculus, inventing the programming language LISP, using which much of AI research was then carried out. Most of my early research in AI was done using LISP, including my first (and most highly cited paper) work on using reinforcement learning to teach robots in the early 1990s at IBM.Why should Machines Learn, by Herbert Simon, in Michalski, Carbonell, and Mitchell (editors), Machine Learning, 1983 (http://digitalcollections.library.cmu.edu/awweb/awarchive?type=file&item=33928). Herb Simon was a Nobel laureate in Economics, who spent his entire academic career at Carnegie Institute of Technology (later Carnegie Mellon University), doing much to build the luster and prestige of this now world-class university. He was one of the true polymaths, at home in half a dozen departments, from computer science to economics to business administration and psychology, in all of which he made foundational contributions. He was a gifted speaker, and I was particularly fortunate to be able to attend several presentations by Simon during the mid 1980s when I spent several years at CMU. In this article, Simon asks the question that very few AI researchers today bother asking: why should machines learn? According to Simon, why should machines, which can be programmed, bother with this slow and tedious form of knowledge acquisition, when something far quicker and more reliable is available. You’ll have to read the article to find his answers, but this article is valuable for giving perhaps the first scientific definition of the field of machine learning, a definition that is still valid today. Simon made many other contributions to AI, including his decades long collaboration with Allan Newell, another AI genius at CMU, whose singular ability in asking the right questions, made him a truly gifted researcher. It is rumored that computer chess came to life when Allan Newell mentioned casually in a conversation in the CMU CS common room about how the branching factor of chess is not all difficult to emulate in hardware, a comment that Hans Berliner followed up on in bringing the first modern chess player, Deep Thought, to fruition (the same CMU team went to IBM, built Deep Blue which of course beat Kasparov).Non-cooperative games, PhD thesis, John Nash, Princeton. (Non-Cooperative Games) John Nash came to Princeton as a 20 year old mathematician from Carnegie Institute of Technology in 1948 with a one line recommendation letter: “This man is a genius”. His PhD thesis would fully affirm his alma mater’s assessment of his capabilities. Nash took the work of von Neumann and Morgenstern’s zero-sum games into a whole new level with his dazzling generalization, which would earn him a Nobel prize decades later. Most of Nash’s history has been recounted in Sylvia Nasar’s wonderful biography A Beautiful Mind (later made into a movie starring Russell Crowe as John Nash). Legend had it that von Neumann himself did not think much of Nash’s work, calling it “another fixed point theorem”. Nash finished his ground breaking thesis in less than a year from start to finish. He arrived in Princeton in September 1948, and in November 1949, Solomon Lefschetz, a distinguished mathematician communicated the results of Nash’s thesis to the National Academy of Sciences meeting. Today, billions of dollars of product (from wireless cellular bandwidths to oil prospects) are traded using Nash’s ideas of game theory. The most influential model in deep learning today is the Generative Adversarial Network (GAN), and the key question being studied for GANs is whether and when do they converge to a Nash equilibrium. So, 70 years after Nash defended his short but Nobel prize winning thesis at Princeton, his work is still having a huge impact in ML and AI. Nash’s work also become a widely used framework to study evolutionary dynamics, giving rise to a new field called evolutionary game theory, pioneer by John Maynard Smith. Game theory is a crucial area for not only AI but also for CS. It has been said that the “Internet is just a game. We have to find what the equilibrium solution is”. Algorithmic game theory is a burgeoning area of research, studying things like “The Price of Anarchy”, or how solutions to hard optimization problems can be solved by letting millions of agents make locally selfish decisions. Nash’s PhD advisor at Princeton was Tucker, who Nash called “The Machine”. His second reader of his PhD thesis was Turkey, who can be called one of the fathers of modern machine learning, since he invented exploratory data analysis at Princeton (and later also invented the Fast Fourier Transform).Maximum likelihood from Incomplete Data using the EM Algorithm, Dempster, Laird, and Rubin (Journal of the Royal Statistical Society, Series B, 1977) (Maximum Likelihood from Incomplete Data via the EM Algorithm). In the mid 1980s, ML took a dramatic turn, along with AI, towards the widespread use of probabilistic and statistical methods. One of the most influential models of machine learning during the 1990s was based on Fisher’s notion of maximum likelihood estimation. Since most interesting probabilistic models in AI had latent (unobserved) variables, maximum likelihood could not be directly applied. The EM algorithm, popularized by three Harvard statisticians, came the rescue. It is probably the most widely used statistical method in ML in the past 25 years, and well worth knowing. This paper, which is cited over 50,000 times on Google Scholar, requires a certain level of mathematical sophistication, but it is representative of modern ML, and much of the edifice of modern ML is based on ideas like EM. A very simple way to think of EM is in terms of “data hallucination”. Let’s say you want to compute the mean of 20 numbers, but forgot to measure the last 5 numbers. Well, you could compute the mean over the 15 numbers only, or you could do something clever, namely put in an initial guess of the mean for each of the missing 5 numbers. This leads to an easy recurrence relation that lets you find the true mean. In the one dimensional case, this happens to be the same as ignoring the last 5 numbers, but in the two dimensional case, where one or the other dimension may be different, EM finds a different solution.A Theory of the Learnable, by Les Valiant, Communications of the ACM, 1984. (https://people.mpi-inf.mpg.de/~mehlhorn/SeminarEvolvability/ValiantLearnable.pdf). George Orwell wrote a brilliant novel about the rise of the all powerful all knowing Government, which spies on everyone. Well, in the same year of the novel, Les Valiant, a brilliant computer scientist at Harvard proved that Orwell’s fears could not be completely realized due to intrinsic limitations on what can be learned from data in polynomial time. That is, even if the Government could spy on individuals, it is possible to construct functions whose identity may be hidden because it would require intractable computation to discover them. Valiant’s work lead to his winning the Turing award several decades later, computer science’s version of the Nobel Prize. What Valiant did in this landmark paper was articulate a theory of machine learning that is analogous to complexity theory for computation. He defined PAC learning, or probably approximately correct learning, as a model of knowledge acquisition from data, and showed examples where a class of functions was PAC learnable, and also speculated about non-learnable functions. Valiant’s work in the past three decades has been hugely influential. For example, the most widely used ensemble method in ML is called boosting, and came out as a direct result of PAC learning. Also to be noted is that support vector machines or SVMs were justified using the tools of PAC learning. This is a short but beautifully written paper, and while it is not an easy read, your ability to understand and grasp this paper will make the difference between whether you are a ML scientist or an ML programmer (not to make any value judgements of either, the world needs plenty of both types of people!).Intelligence without representation, Rodney Brooks, IJCAI 1987 Computers and Thought Award lecture (http://www.fc.uaem.mx/~bruno/material/brooks_87_representation.pdf). Brooks based his ideas for building “behavior-based robots” on ethology, the study of insect behavior. What ethologists found was that ants, bees, and lots of other insects were incredibly sophisticated in their behaviors, building large complex societies (ant colonies, bee hives), and yet their decision making capacity seemed to be based on fairly simple rules. Brooks took this type of idea to heart, and launched a major critique at the then representation heavy apparatus of modern knowledge-based AI. He argued that robots built using knowledge-based AI would never function well enough in the real world to survive. A robot crossing the road that sees a truck and begins to reason about what it should do would get flattened by the truck before its reasoning engine came up with a decision. According to Brooks, this failure was due to a misunderstanding of how brains are designed to produce behavior. In animals, he argued, behaviors are hard wired in a layered highly modularized form, so that complexity emerges from the interleaving of many simple behaviors. One of his early PhD students, Jonathan Connell, showed that you can design a complex robot, called Herbert (after Herb Simon), that could do a complex task of searching an indoor building for soda cans and picking them and throwing them into trash, all the while having no explicit representation anywhere of the task. Later, after Jon Connell graduated, he came to work for IBM Research, where he and I collaborated on applying RL to teach behavior-based robots new behaviors. Brooks was a true pioneer of robotics, and inserted a real-world emphasis in his work that was till then sorely lacking. He had a common-sense wisdom about how to apply the right sort of engineering design to a problem, and was not enamored of using fancy math to solve problems that had far simpler solutions. Much of the success of modern autonomous driving systems owes something to Brooks’ ideas. It is possible that the tragic accident in Arizona involving an Uber vehicle might have been averted had that particular vehicle been outfitted with a behavior-based design (which countermands bad decisions, like the one the Uber vehicle allegedly made, of labeling he pedestrian as a false positive).Natural Gradient Works Efficiently in Learning, Amari, Neural Computation, 1989 (http://citeseerx.ist.psu.edu/viewdoc/download?doi=10.1.1.452.7280&rep=rep1&type=pdf). One of the living legends of statistics is the Indian scientist C.R. Rao, now in his 90s, who basically has done the most since Fisher in building up the edifice of modern statistics. C. R. Rao invented much of modern multivariate statistics as a young researcher at Univ. of Cambridge, England, due to his study of fossils of human bones from Ethiopia. In a classic paper written in his 20s, C. R. Rao showed that the space of probability distributions is curved, like Einstein’s space-time, and has a Riemannian inner product defined on the space of tangents at each point on its surface. He later showed how the Fisher information metric could be used to define this inner product. Amari, a brain science researcher in Japan, used this insight to define natural gradient methods, a widely used class of methods to train neural networks, where the direction pursued to modify the weights at any given point is not the Euclidean direction, but the direction that is based on analyzing the curved structure of the underlying probability manifold. Amari showed natural gradient often works better, and later wrote a highly sophisticated treatise on information geometry, expanding on his work on natural gradients. Many years later, in 2013, a group of PhD students and I showed that natural gradient methods could actually be viewed as special cases of a powerful class of dual space gradient methods called mirror descent, invented by Russian optimization researchers Nemirovksy and Yudin. Mirror descent has now become a basis for one of the most widely used gradient methods in deep learning called ADAGRAD by Duchi (now at Stanford), Hazan (now at Princeton) and Singer (now at Google). It is very important to understand these various formulations of gradient descent methods, which requires exploring some beautiful connections between geometry and statistics.Learning to Predict by the Methods of Temporal Differences, by Richard Sutton, Machine Learning journal, pp 9–44, 1988 (https://pdfs.semanticscholar.org/9c06/865e912788a6a51470724e087853d7269195.pdf). TD learning remains the most widely used reinforcement learning method, 34 years after they were invented by UMass PhD student Richard Sutton, working in collaboration with his former PhD advisor, Andrew Barto, both of whom can be said to have laid the foundations of the modern field of RL (on whose work the company Deep Mind was originally formed, and then acquired by Google). It is worth noting that Arthur Samuel in 1950s experimented with a simple form of TD learning, and used it to teach an IBM 701 to play checkers, which can be said to be the first implementation of both RL and ML in the modern era. But Rich Sutton brought TD -learning to life, and if you read the above paper, you’ll see the mathematical sophistication he brought to its study was far beyond Samuel. TD learning is now far beyond this paper, and if you want to see how mathematically sophisticated its modern variants are, I will point you to the following paper (which builds on the work of one of my former PhD students, Bo Liu, who brought the study of gradient TD methods to a new level with his work on dual space analysis). Janet Yu has written a very long (80+ pages) dense mathematical treatise on the modern version of gradient TD, which you have to be very strong in math to understand fully ([1712.09652] On Convergence of some Gradient-based Temporal-Differences Algorithms for Off-Policy Learning). TD remains one of the few ML methods for which there is some evidence that it is biologically plausible. The brain seems to encode TD error using dopamine neurotransmitters. The study of TD in the brain is a very active area of research (see http://www.gatsby.ucl.ac.uk/~dayan/papers/sdm97.pdf).Human learning in Atari, Tsividis et al., AAAI 2017 (http://gershmanlab.webfactional.com/pubs/Tsividis17.pdf). Deep reinforcement learning was popularized in a sensational paper in Nature (Human-level control through deep reinforcement learning) by a large group of Deep Mind researchers, and it is by now so well known and cited that I resisted the temptation to include it in my top 20 list (where most people would put it). It has led to large numbers of follow on papers, but many of these seem to miss the fairly obvious fact that there is a huge gulf between the speed at which humans learn Atari games and TD Q-learning with convolutional neural nets does so. This beautiful paper by cognitive scientists at MIT and Harvard shows that humans learn many of the Atari games in a matter of minutes in real time play, whereas deep RL methods require tens of millions of steps (which would be many months of human time, perhaps even years!). So, deep RL cannot be the ultimate solution to the Atari problem, even if it is currently perhaps the best we can do. There is a huge performance gap between humans and machines here, and if you are a young ML researcher, this is where I would go to make the next breakthrough. Humans seem to do much more than deep RL when learning to play Atari.Distributed Optimization and Statistical Learning via the Alternating Direction Method of Multipliers, Boyd et al., Foundations and Trends in Machine Learning, 2011 (Distributed Optimization and Statistical Learning via the Alternating Direction Method of Multipliers, which has MATLAB code as well). The 21st century has arrived, and with it, the dawn of cloud computing, and machine learning is poised to exploit these large numbers of cloud based computational structures. This very long and beautifully written paper by Stanford optimization guru Stephen Boyd and colleagues shows how to design cloud based ML algorithms using a broad and powerful framework called Alternating Direction Method of Multipliers (or ADMMs). As the saying goes in the Wizard of Oz, “we are no longer in Kansas, Toto”. Namely, with this paper, we are now squarely in modern machine learning land, where the going gets tough (but, then as the saying goes, “the tough get going”). This is a mathematically deep and intense paper, of more than 100 pages, so it is not an easy read (unless, that is, your are someone like Walter Pitts!). But, the several weeks or months you spend reading it will greatly improve your ability to see how to exploit modern optimization knowledge to speed up many machine learning methods. What is provided here is a generic tool box, and you can design many specialized variants (including Hadoop based variants, as shown in the paper). To understand this paper, you need to understand duality theory, and Boyd himself has written a nice book on convex optimization to help you bridge that chasm. The paper is highly cited, for good reason, as it is a model of clarity.Learning Deep Architectures for AI, by Bengio, TR 1312, Univ. of Montreal (https://www.iro.umontreal.ca/~lisa/pointeurs/TR1312.pdf) (also a paper published in the journal Foundations and Trends in Machine Learning). Bengio has done more than almost anyone else in popularizing deep learning, and is also one of its primary originators and innovators. In this paper, he lays out a compelling vision for why AI and ML systems need to incorporate ideas from deep learning, and while many of the specifics he says have changed due to the rapid progress in deep learning in the last few years, this paper is a classic that bears well. This paper was written as counter point to the then popular approach of shallow architectures in machine learning, such as kernel methods. Bengio is giving another of his popular tutorials on deep learning at the forthcoming IJCAI conference in July in Sweden, in case you are interested in attending the conference or the tutorial. I don't have to say much more about deep learning, as it is the subject of a barrage of publicity these days. Suffice it to say that today AI is very much in the paradigm of deep learning (meaning a framework in which every problem is posed as a problem of deep learning, whether it is the right approach or not!). Time will tell how well deep learning survives in its current form. There are beginning to be worries about the robustness of deep learning solutions (the Imagenet architectures seem very vulnerable to random noise, which humans can’t even see, let along respond to), and the sample complexity seems formidable still. Scalability remains an open question, but deep learning has shown remarkable performance in many areas, including computer vision (if you download the latest version of MATLAB R2018a, you can run the demo image recognition program with a web cam with objects in your own house, and decide for yourself how well you think deep learning works in the real world).Theoretical Impediments to Machine Learning, with Seven Sparks from the Causal Revolution, by Judea Pearl, Arxiv 2018. ([1801.04016] Theoretical Impediments to Machine Learning With Seven Sparks from the Causal Revolution). Pearl is in my view the Isaac Newton of AI. He developed the broad probabilistic framework of graphical models, which dominated AI in the 1990s-2010s. He subsequently went into a different direction with his work on causal models, and now argues that probabilities are “an epiphenomenon” (or a surface property, of a much deeper causal truth). Pearl’s work on causal models has yet to gain the same traction in AI as his earlier work on graphical models (which is a major subfield in both AI and ML). Largely, the reasons have to do with the sort of applications that causal models fit well with. Pearl is focusing on domains like healthcare, education, climate change, societal models etc. where interventions are needed to change the status quo. In these hugely important practical applications, he argues that descriptive statistics is not the end goal, but causal models are. His 2009 2nd edition of Causality is still the most definitive modern treatment of the topic, and well worth acquiring.Prospect Theory: An Analysis of Decisions under Risk, by Daniel Kahneman and Amos Tversky, Econometrica, pp. 263–291, 1979. Daniel Kahneman received the Nobel prize in Economics for this work, with his collaborator Amos Tversky (who sadly died, and could not share in the prize). In this pathbreaking work, they asked themselves the simple question: how do humans make decisions under uncertainty? Do they follow the standard economic model of maximizing expected utility? If I gave you the choice between two outcomes: choose Door 1, and with 50% probability, you get no cash prize, or you get $300; alternatively if you choose Door 2, you get a guaranteed prize of $100. It perhaps won't surprise you that many humans choose Door 2, even through expected utility theory shows you should choose Door 1( since the expected utility is $150, much higher than Door 2). What’s going on? Well, humans tend to be risk averse. We would rather have the $100 for sure, than risk getting nothing with Door 1. This beautiful paper, which has been cited over 50,000 times, explores such questions in a number of beautiful simple experiments that been repeated all over the world with similar results. Well, here’s the rub. Much of the theory of modern probabilistic decision making and reinforcement learning in AI is based on maximizing expected utility (Markov decision processes, Q-learning, etc.). If KT is right, then much of modern AI is barking up the wrong tree! If you care about how humans actually make decisions, should you continue to chose an incorrect approach? Your choice. Read this paper and decide.Towards an Architecture for Never-ending Language Learning, Carlson et al., AAAI 2010. Humans learn over a period of decades, but most machine learning systems learn over a much shorter period of time, often just a single task. This CMU effort led by my former PhD advisor, Thomas Mitchell, explores how a machine learning system can learn over a very long period of time, by exploring the web, and learning millions of useful facts. You can interact with the actual NELL system online at Carnegie Mellon University. NELL is a fascinating example of how the tools of modern computer technology, namely the world wide web, makes it possible to design ML systems that can run forever. NELL could potentially live longer than any of us, and constantly acquire facts. One issue, at the heart of recent controversies, is “fake news”, of course. How does NELL know what it has learned is true? The web is full of fake assertions. NELL currently uses a human vetting approach of deciding which facts it learns are really to be trusted. Similar systems can be designed for image labeling, language interactions, and many others.Topology and Data, by Gunnar Carlson, Bulletin of the American Mathematical Society, April 2009 (http://www.ams.org/images/carlsson-notes.pdf). The question that many researchers are interested in knowing the answer to is: where is ML going in the next decade? This well known Stanford mathematician is arguing in favor of the use of more sophisticated methods from topology, a well developed area of math that studies the abstract properties of shape. Topology is what mathematicians use to decide that a coffee cup (with a handle) and a doughnut are essentially the same, since one can be smoothly deformed into the other without cutting. Topology has one great strength: it can be used to analyze data even when standard smoothness assumptions in ML are not possible to make. It goes without saying that the mathematical sophistication needed here is quite high, but Carlson refrains from getting very deep into the technical subject matter, giving for the most part, high level examples of what structure can be inferred using the tools of computational topology.2001: A Space Odyssey, book by Arthur C. Clarke, and movie by Stanley Kubrick. My next and last choice of reading — this has gone on long enough, and both you and I are getting a bit tired by now — is not an AI paper, but a movie and the associated book. The computer HAL in Kubrick’s movie 2001 is to my mind the best exemplar of an AI based intelligent system, one that is hopefully realizable soon. 2001 was released in 1968, exactly 50 years from now, and its 50th anniversary was marked recently. Many of my students and colleagues, I find, have not seen 2001. That is indeed a sacrilege. If you are all all interested in AI or ML, you owe it to yourself to see this movie, or read the book, and preferably do both. It is in my mind the most intelligent science fiction movie ever made, and it puts all later movies to shame (no, there is no silly laser sword fights or fake explosions or Darth Vaders here!). Instead, Stanley Kubrick designed the movie to be as realistic as the technology from 1960s would allow, and it is surprisingly modern even today. HAL is of course legendary from his voice (“I’m sorry Dave” is now available as a ring tone on many cellphones). But, HAL is also a great example of how modern AI will work with humans, and help assist many functions. Many long voyages into space, such as Mars or beyond, cannot be done with a HAL, as humans will have to sleep or be in hibernation to save on storage for food etc. There is a nice book by Stork on doing a scene by scene analysis of HAL in the movie, with where AI is in the 21st century. This book is also worth acquiring.OK, I’m ending this uber long reply two papers short of the required 20, but I’m sure I’ve given you plenty of material to read and digest. I did also cheat a bit here and there, and gave you multiple papers to read per bullet. Happy reading. Hope your journey into the fascinating world of AI is every bit as rewarding and fun as mine has been over the past 30+ years.
How many languages does the chief translator of the European Parliament speak?
http://This Man Speaks 32 Different LanguagesYouTubeIoannis Ikonomou (Greek: Ιωάννης Οικονόμου; born 1964 in Heraklion, Crete) is a Greek translator who has been working for the European Commission in Brussels since 2002. Considered a notable contemporary example of a polyglot, he knows 32 living languages including Greek, English, German, Italian, Spanish, French, Finnish, Danish, Russian, Swahili, Hebrew, Arabic, Mandarin and Bengali, and reportedly as many as 47 languages including dead languages like Old Church Slavic. He speaks 21 out of 24 official EU languages. He considered Mandarin language as the most complicated language to learn. Chinese is also his favorite language. He is the only in-house translator of European Commission who is trusted to translate classified Chinese documents.The EU institutions employ around 4300 translators and 800 interpreters on its permanent staff. The estimated cost of all language services (translation and interpreting) in all EU institutions adds up to less than 1% of the annual general budget of the EU. Divided by the population of the EU, this comes to around €2 per person per year.Inspired by foreign tourists visiting Crete, he began to study foreign languages at a young age: English at age five, when he moved to Athens with his family, German at seven, Italian at 10, Russian at 13, East African Swahili at 14, and Turkish at 16. He had learned 15 languages by the age of 20. He studied linguistics at the University of Thessaloniki before pursuing an MA in Middle Eastern languages and cultures at Columbia University in the United States. He continued with a PhD in Indo-European linguistics at Harvard University. The subject of his dissertation in Harvard was a text by Zarathustra written in Avestan, a form of Old Iranian.He is married to his husband, Tomek, who is Polish. To maintain his language skills, he stays up every night to chat online with native speakers from around the world. His favorite
- Home >
- Catalog >
- Business >
- Cv Template >
- Academic Cv Template >
- Academic Cv >
- academic cv template word >
- Form Phd Dissertation Harvard University