How to Edit The Form Vec B 31 easily Online
Start on editing, signing and sharing your Form Vec B 31 online refering to these easy steps:
- Push the Get Form or Get Form Now button on the current page to jump to the PDF editor.
- Wait for a moment before the Form Vec B 31 is loaded
- Use the tools in the top toolbar to edit the file, and the change will be saved automatically
- Download your completed file.
The best-rated Tool to Edit and Sign the Form Vec B 31
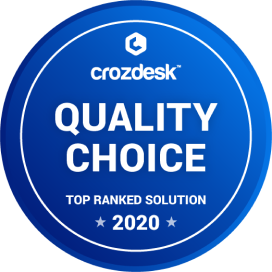
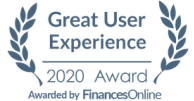
A quick direction on editing Form Vec B 31 Online
It has become really easy just recently to edit your PDF files online, and CocoDoc is the best tool you have ever used to make changes to your file and save it. Follow our simple tutorial to start!
- Click the Get Form or Get Form Now button on the current page to start modifying your PDF
- Add, change or delete your content using the editing tools on the toolbar on the top.
- Affter altering your content, add the date and make a signature to bring it to a perfect comletion.
- Go over it agian your form before you click on the button to download it
How to add a signature on your Form Vec B 31
Though most people are adapted to signing paper documents with a pen, electronic signatures are becoming more common, follow these steps to sign PDF online for free!
- Click the Get Form or Get Form Now button to begin editing on Form Vec B 31 in CocoDoc PDF editor.
- Click on the Sign tool in the tools pane on the top
- A window will pop up, click Add new signature button and you'll have three ways—Type, Draw, and Upload. Once you're done, click the Save button.
- Drag, resize and settle the signature inside your PDF file
How to add a textbox on your Form Vec B 31
If you have the need to add a text box on your PDF for customizing your special content, do some easy steps to get it done.
- Open the PDF file in CocoDoc PDF editor.
- Click Text Box on the top toolbar and move your mouse to position it wherever you want to put it.
- Write in the text you need to insert. After you’ve input the text, you can use the text editing tools to resize, color or bold the text.
- When you're done, click OK to save it. If you’re not happy with the text, click on the trash can icon to delete it and do over again.
A quick guide to Edit Your Form Vec B 31 on G Suite
If you are looking about for a solution for PDF editing on G suite, CocoDoc PDF editor is a commendable tool that can be used directly from Google Drive to create or edit files.
- Find CocoDoc PDF editor and establish the add-on for google drive.
- Right-click on a PDF document in your Google Drive and click Open With.
- Select CocoDoc PDF on the popup list to open your file with and allow access to your google account for CocoDoc.
- Modify PDF documents, adding text, images, editing existing text, mark up in highlight, fullly polish the texts in CocoDoc PDF editor before saving and downloading it.
PDF Editor FAQ
How do you tell if a matrix equation has an infinite number of solutions?
A matrix in itself does not have the property of having unique or infinite solutions. It is only the linear systems that has such properties. Not trying to disown your question, but in language of Maths it isdesirable to be addressed that way.for in instance a linear system ofx + 2y+ 3z= 32x +4y + z = 2x + 3y + 4z = 1can be written in its Augmented form as[math]\begin{bmatrix}1 & 2 & 3 & 3 \\2 & 4 & 1 & 2 \\1 & 3 & 4 & 1\end{bmatrix} [/math]from which u can proceed on to Gaussian elimination.OR also in Linear Algebra it is of form :[math]A \vec{x} = \vec{b}. [/math]where[math] \vec{x} = (x,y,z) [/math][math]\vec{b}= (3,2,1) [/math]A is just the 3 by 3 matrix above . The solution to equation is thus[math] \vec{x} = A^{-1} \vec{b}[/math]A linear systems will only have unique solutions if[math]det|A| \ne 0 [/math]Here [math]det |A| = 5[/math]Thus its solution [math]\vec{x} = (x,y,z) = (31/5, -14/5 , 4/5)[/math]
What is the mathematical intuition behind the determinant of a matrix? How was its definition conceived and why is it important? What does it mean intuitively?
The Gram Zeppi answer is enlighting , but I want to use another similar approach, even more intuitive, but informal and not so techie.The determinant, usually algebraically defined, will be defined here as Gram Zeppi said, as representing an n-dimensional volume, from the abstraction of seeing the columns as n-dimensional vectors, forming the edges of a hyper parallelepiped expressed by determinant.In this case there are basic properties, which serve as a starting point to deduce the Laplace Expansion, usually taught in the definition of Determinants in schools and universities.1) The volume of a transposed matrix (when one changes rows to columns) is equal to the original matrix volume. In fact, it is expected by symmetry. Thus, the properties valid for columns, described below, are also valid for rows.2) The vectors in the columns must be independent linearly, so that the volume is different from [math]0[/math]. For example, In [math]2D[/math], two collinear vectors (multiples) don’t form a parallelogram. In the [math]3D[/math] case, three coplanar vectors (the situation that happens when one vector can be obtained by the linear combination of the other 2) or 2 collinear vectors generates a null volume.In general case ([math]N[/math] dimensions) any subset of columns from n-dimension determinant where one column can be obtained by linear operators (sequence of sums and scalar multiplications) using the rest of colums subset, make the volume flat in [math]N[/math] dimensions, because, in that case, at least 1 dimension will be missing because at least 1 vector is redundant and don’t points to a different dimension.[math]\bigstar \bigstar \bigstar [/math]Before proceeding with more properties, one must learn to calculate volume with [math]N[/math] dimensions using [math]N-1[/math] dimensions volumes.First of all, we don’t use here cross product, because it only exist for 3 dimensions (and for 7 …). Also, we don’t use more determinants, because it would be a recursive reasoning.For clearer understanding, let’s imagine a matrix 3x3 with a left vector [math]\mathbf {\vec{a} = [a_1,a_2,a_3]}[/math]and to the right two additional vectors: [math]\mathbf {\vec{b} = [b_1,b_2,b_3]}[/math] and [math]\mathbf {\vec{c}= [c_1,c_2,c_3]}[/math] in 3D space. Let’s forget the left vector [math]\mathbf {\vec{a}}[/math] for a while.If the vector [math]\mathbf {\vec{b}}[/math] and [math]\mathbf {\vec{c}}[/math] are independent linearly, they are contained in a plane inside 3D space. Now we will find the area spanned by [math]\mathbf {\vec{b}}[/math] and [math]\mathbf {\vec{c}}[/math]. It wouldn’t be necessary, because we could use 2D space using vector module, but it’s interesting to see how it’s possible to avoid cross product usage at all.First, we will find a normal [math]\mathbf {\vec{n} = [n_1,n_2,n_3]} [/math]that is perpendicular to [math]\mathbf {\vec{b}}[/math] and [math]\mathbf {\vec{c}}[/math] simultaneously. So [math]\mathbf {\vec{n} \bullet \vec{b} = 0}[/math] and [math]\mathbf {\vec{n} \bullet \vec{c} = 0}[/math].Therefore[math]\mathbf {b_1 n_1 + b_2 n_2 + b_3 n_3 = 0\quad}[/math] and[math] \mathbf {\quad c_1 n_1 + c_2 n_2 + c_3 n_3 = 0} [/math]As it only matters the normal direction, one can consider [math]\mathbf {n_1 = 1}[/math] and solve the above equations.Now, we need find an orthogonal [math]\mathbf {\vec{o}}[/math] coplanar to [math]\mathbf {\vec{b}}[/math] (could be [math]\mathbf {\vec{c}}[/math] , whatever) and orthogonal to [math]\mathbf {\vec{n}} [/math]To find [math]\mathbf {\vec{o}}[/math], we use the same kind of equations above with [math]\mathbf {\vec{n}}[/math] and [math]\mathbf {\vec{b}}[/math] coefficients. After we find [math]\mathbf {\vec{o}}[/math], we normalize to [math]\mathbf {\|[/math][math]b[/math][math]\|}[/math] magnitude. So[math] \mathbf {Area_{bc} = \vec{o} \bullet \vec{c}}[/math]Why? Because dot product projects [math]\mathbf {\vec{c}}[/math] in [math]\mathbf {\vec{o}}[/math] (normal to [math]\mathbf {\vec{b}}[/math]) multiplying by [math]\mathbf {cos \theta}[/math], the angle between [math]\mathbf {o}[/math] and [math]\mathbf {c}[/math], resulting in the parallelogram height that will be multiplied by [math]\mathbf {\|\vec{o}\|}[/math], that is [math]\mathbf {\vec{b}}[/math] basis.To get the volume (our initial goal) the normal [math]\mathbf {\vec{n}}[/math], has to be normalized with magnitude equal to area spanned by [math]\mathbf {\vec{b}}[/math] and [math]\mathbf {\vec{c}}[/math] producing [math]\mathbf {\vec{n_{bc}}}[/math], so[math] \mathbf {Volume =\vec{n_{bc}} \bullet \vec{a}}[/math]For what reason? if [math]\mathbf {\vec{a}}[/math] in column [math]1[/math] was a vector with magnitude [math]1[/math] normal to plane that contains [math]\mathbf {\vec{b}}[/math] and [math]\mathbf {\vec{c}}[/math] , the volume generated would be equal (in value) to [math]\mathbf {Area_bc}[/math] in plane. It’s a [math]3D[/math] solid with height [math]1[/math] at right angle so the volume is the basis area [math]\times [/math]height.Generally the vector [math]\mathbf {\vec{a}}[/math] is oblique to the basis [math]\mathbf {\vec{b} \vec{c}}[/math], so if the angle between [math]\mathbf {\vec{a}}[/math] and basis [math]\mathbf {\vec{b} \vec{c}}[/math] is [math]\mathbf{\phi}[/math], the volume is [math]\mathbf {Area_{bc} \, * \|\vec{a}\|cos\phi}[/math]We know to calculate [math]3D[/math] volumes. By induction, we want to show that if we know calculate hyperplane volume in [math]N-1[/math] dimensions, we will know to calculate in [math]N[/math] dimensions.The argument below is a kind of repetition, but within an abstract dimension, as any dimension above [math]3[/math].We get a determinant with [math]N[/math] dimensions. In the same way, we separate the first column vector [math]\mathbf {\vec{a}}[/math] in the left (the same reasoning is also true for lines for symmetry ), Analogously the other vectors to the right forming the edges of a hyperplane [math]V[/math] with [math]N-1[/math] dimensions, the we know how to calculate the volume!So, in the same way, we find the normal vector [math]\mathbf {\vec{n}}[/math] with a similar procedure seen above (just more equations) and we normalize magnitude vector [math]\mathbf {\vec{n}}[/math] to [math]N-1[/math] dimensions volume obtaining [math]\mathbf {\vec{n_v}}[/math].The same above procedure can be done. The volume for [math]n[/math] dimensions with additional vector [math]\mathbf {\vec{a}}[/math] is simply:[math] \mathbf {Volume =\vec{n_v} \bullet \vec{a}}[/math]At short, knowing how to calculate volume and normal in [math]n-1[/math] dimensions, allow easily calculate volume in [math]n[/math] dimensions.So far, we have said that it is possible to calculate the volume from a determinant , but we don’t yet link this to the determinant calculation, which we will do later.[math]\bigstar \bigstar \bigstar[/math]All that reasoning helps to justify the properties below:3) Out of any signal convention (discussed later), simple column switching (base volume vectors that defines a volume) does not change the volume in module, because different vector picking orders don’t change the geometric form.4) If a column is multiplied by [math]\mathbf {\alpha}[/math], the determinant is also multiplied by [math]\mathbf {\alpha}[/math]. According to property 3, one can change the column multiplied by [math]\mathbf {\alpha}[/math] by the first column and use the linearity of the scalar product:[math]\mathbf {\vec{N_V} \bullet \alpha \,\vec{a} = \alpha (\vec{V_{N-1}} \bullet \vec{a})}[/math]5) If 3 matrices [math]\mathbf {A}[/math], [math]\mathbf {[/math][math]B[/math][math]}[/math] and [math]\mathbf {C}[/math] differ only by column [math]\mathbf {k}[/math] where[math]\mathbf {C [., t_1 + t_2, .] = A [., t_1, .] + [/math][math]B[/math][math] [., t_2, .]}[/math] then [math]\mathbf {Det (C) = Det (A) + Det ([/math][math]B[/math][math])}[/math]( . indicates 0 or more columns, [math]\mathbf {t_1+t_2}[/math], [math]\mathbf {t_1}[/math] and [math]\mathbf {t_2}[/math] are in column [math]\mathbf {k}[/math])According to property 3, change the column [math]\mathbf {k}[/math] to the first one, then use the linearity of the scalar product:[math] \vec{N_V} \bullet (\vec{a_1} + \vec{a_2}) = \vec{N_V} \bullet \vec{a}[/math]6) The inversion of 2 columns (or lines) reverses the value of determinant [math]\mathbf {A}[/math].This shows that some signal convention needs to be adopted in order to respect this property.Let’s prove it.Suppose that the column [math]\mathbf {J}[/math] = column [math]\mathbf {K}[/math] (where [math]\mathbf {J < K)}[/math]In this case, by property 2, the determinant is [math]0[/math]Assume two values [math]\mathbf {t}[/math] and [math]\mathbf {u}[/math] that add up to give the value of the repeated column. (In the below formulas, dot ([math]\mathbf {.}[/math]) indicates 0 or more hidden columns and the displayed columns are respectively the columns [math]\mathbf {J}[/math] and [math]\mathbf {K}[/math]).[math]\mathbf {\mid.,\,t + u,\,.,\,t + u,\,.\mid\quad(A )\quad = }[/math][math]\mathbf {\qquad \mid.,\, t,\, .,\, t,\,.\mid\quad(A_1)\quad+}[/math][math]\mathbf {\qquad \mid.,\, t,\, .,\, u,\,.\mid\quad(A_2)\quad+}[/math][math]\mathbf {\qquad \mid.,\, u,\, .,\, t,\, .\mid\quad(A_3)\quad+}[/math][math]\mathbf {\qquad \mid.,\, t,\, .,\, t,\, .\mid\quad(A_4)}[/math][math] \mathbf {A_1}[/math] and[math] \mathbf {A_4}[/math] are null because they have equal columns. Thus [math]\mathbf {A_2 = -A_3}[/math], so that the determinant cancels out. The only difference between [math]\mathbf {A_2}[/math] and [math]\mathbf {A_3}[/math] is the swap of 2 columns.[math]\bigstar \bigstar \bigstar[/math]We define above the fundamental determinant properties, based on the geometric paradigm. Now we are ready to prove the Laplace Expansion, again using the finite induction.Imagine a matrix [math]M[/math] with two dimensions [math]\mathbf {[\vec{v}, \vec{w}]}[/math], Its determinant defines a parallelogram. The vector [math]\mathbf {\vec{v} = [v_1 v_2]}[/math] has a normal given by [math]\mathbf {[-v_2, v_1]}[/math], which is the basis of the parallelogram formed by[math] \mathbf {\vec{v}}[/math] and [math]\mathbf {\vec{w}}[/math] in the direction of the parelogram.Thus the scalar product [math]\mathbf {[-v_2, v_1]\bullet [w_1 w_2] = v_1w_2 - v_2w_1}[/math], which corresponds to the projection of the lateral of the parelogram in the height multiplied by the base of the parallelogram, gives the parallelogram area.[math]\mathbf {\begin{bmatrix} v_1&w_1\\v_2&w_2 \end{bmatrix} = \begin{bmatrix} v_1&w_1\\0&w_2 \end{bmatrix}+\begin{bmatrix} 0&w_1\\v_2&w_2 \end{bmatrix}}[/math]It is clear that the same can be found by the Laplace Expansion with [math]\mathbf {v_1 w_2}[/math]and [math]\mathbf {v_2 w_1}[/math], except that in the second case there was one line change (2 with 1), according to Theorem 6, by inverting the signal of the determinant, because there are odd permutations, so determinant is [math]\mathbf {v1.w2 - v2.w1}[/math]Let's insert a new dimension from the left and the base in the dimension [math]N-1[/math], which is supposed to apply the Laplace Expansion correctly:From this it must be proved for case [math]N[/math], illustrated here by a matrix [math]M[/math] with [math]N = 3[/math].[math]\mathbf {\begin{bmatrix} u_1&\mid&v_1&w_1\\u_2&\mid&v_2&w_2 \\u_3&\mid&v_3&w_3\end{bmatrix}}[/math]Using the sum theorem, we separate the matrices[math]\qquad \qquad M_1 \qquad\qquad\qquad\qquad M_2 \qquad\qquad\qquad\qquad M_3 \\ \begin{bmatrix} u_1&\mid&\mathbf{v_1}&\mathbf{w_1}\\0&\mid&v_2&w_2 \\0&\mid&v_3&w_3\end{bmatrix}+\begin{bmatrix} 0&\mid&v_1&w_1\\u_2&\mid&\mathbf{v_2}&\mathbf{w_2}\\0&\mid&v_3&w_3\end{bmatrix}+\begin{bmatrix} 0&\mid&v_1&w_1\\0&\mid&v_2&w_2\\ u_3&\mid&\mathbf{v_3}&\mathbf{w_3}\end{bmatrix}[/math]Looking at the matrices above [math]\mathbf {M_1}[/math], [math]\mathbf {M_2}[/math] and [math]\mathbf {M_3}[/math], it is clear that the bold components of [math] \mathbf {[v_1, v_2, v_3]}[/math] and [math]\mathbf {[w_1, w_2, w_3]}[/math] in the same line of non-zero value of column 1 don’t contribute at all for volume formation, by not bringing the point out of the pure axis expressed in column 1.The figure above helps the reader understand because an pure dimensional vector like [math]\mathbf {\vec{A}}[/math] (parallel to a canonical axis, [math]z[/math] in this case) don’t need to handle with same dimension components from other vectors. The only effect is shearing through this dimension, what don’t create or destroy any volume.Then we have[math]\mathbf{M_1}:\quad\begin{bmatrix} v_2&w_2\\v_3&w_3 \end{bmatrix}[/math]is a parallelogram of area [math]\mathbf {v_2w_3 - v_3w_2}[/math] in [math]\mathbf {plane_{23}}[/math] in a coplanar vector of this magnitude [math]\bullet[/math] (scalar product) a vector [math]\mathbf {\bot}[/math] to [math]\mathbf {plane_{23}}[/math] with magnitude [math]\mathbf {u_1}[/math] that results in [math]\mathbf {u_1 (v_2w_3 - v_2w_3)}[/math] with order: [math]\mathbf {123}[/math] (no row swap)[math]\mathbf{M_2}:\quad \begin{bmatrix} v_1&w_3\\v_1&w_3 \end{bmatrix}[/math]is a parallelogram of area [math]\mathbf {v_1w_3 - v_3w_1}[/math] in [math]\mathbf {plane_{13}}[/math] in a coplanar vector of this magnitude [math]\bullet[/math] (scalar product) vector [math]\mathbf {\bot}[/math] to [math]\mathbf {plane_{13}}[/math] with magnitude [math]\mathbf {u_2}[/math] that results in [math]\mathbf {u_2 (v_1w_3 - v_1w_3)} [/math]with order: [math]\mathbf {213}[/math] (1 row swap)[math]\mathbf{M_3}:\quad \begin{bmatrix} v_2&w_3\\v_2&w_3 \end{bmatrix}[/math]is a parallelogram of area [math]\mathbf {v_1w_2 - v_2w_1}[/math] in [math]\mathbf {plane_{12}}[/math] in a coplanar vector of this magnitude [math]\bullet[/math] (scalar product) vector [math]\mathbf {\bot}[/math] to [math]\mathbf {plane_{12}}[/math] with magnitude [math]\mathbf {u_3}[/math] that results in [math]\mathbf {u_3 (v_1w_2 - v_1w_2)} [/math]with order: [math]\mathbf {321}[/math] (2 rows swaps)In [math]\mathbf {M_2}[/math] there is a single row swap that reverses the signal, thus[math]\mathbf {Det(M) = u_1 (v_2 w_3 - v_2 w_3) - u_2 (v_1w_3 - v_1w_3) + u_3 (v_1w_2 - v_2w_1)}[/math]This corresponds to the Laplace Expansion for [math]\mathbf {N = 3}[/math] because[math] \mathbf {Det (M) = u_1 Minor_{11} - u_2 Minor_{21} + u_3 Minor_{31})}[/math]Where the first subscript in Minor is the line reference and the second subscript is column reference.In the general n-dimension matrix case, first separate a matrix [math]M[/math] into a sum of M matrices, each one with just one value of column 1.[math] \mathbf{M}:\quad \begin{bmatrix}M_{11}&...&M_{1N}\\...&...&...\\M_{LI}&...&M_{LN}\\...&...&...\\ M_{N1}&...&M_{NN}\end{bmatrix} \quad=\quad \Sigma_{I=I,N} \begin{bmatrix}0 \; or\; M_{1,1}(I=1) &...&M_{1N} \\...&...&... \\0 \; or\; M_{L,1}(I=L)&...&M_{LN}\\...&...&... \\0 \; or\; M_{N,1}(I=N)&...&M_{NN}\end{bmatrix}[/math]The volume of the total determinant, will be the sum of several volumes obtained for each matrix, multiply the column value (only non-zero component of first column in each matrix) by the hypervolume (determinant) associated in n-1 dimensions submatrix, with the right convention of signal.As above, this submatrix from column 2 until column N is a square matrix, because it discard the line containing values of the same line to the non-zero value in the first column, as it does not contribute to moving away from the pure axis expressed in column 1.From top to bottom, the number of swaps is incremented by 1, alternating the positive (initial) signal with the negative signal in the corresponding part.In the end, the term is positive with an even number of row swaps (permutations) and negative the term with an odd number of row swaps or permutations.So we have the Laplace Expansion:[math]\mathbf {Det (M) = \Sigma_{L = 1, N} (-1)^{L + C} M[L, 1] Minor_{L1}}[/math]With transpose and column or row swap properties, it’s easy to proof that this formula can by applied to any row or column basis.[math]\bigstar\bigstar\bigstar[/math]We will now prove one of the most important properties form the determinants that states:A determinant of a matrix product is the product of the determinants of the matrices.Linear Transformations are one the most important fields in Linear Algebra and all applied sciences. The geometric approach for determinants and this theorem are essentials to Linear Transformations because help to understand and value the transformations and its compositions.Below I display a geometric proof what can be formally formulated with integrals.Suppose an matrix [math]\mathbf {A}[/math] with [math]\mathbf {N}[/math] dimensions.a) If [math]\mathbf {[/math][math]B[/math][math]}[/math] is a matrix representing a cube with all dimensions = 1, this corresponds to the identity matrix [math]\mathbf {I}[/math] with volume 1. It’s easy to notice this, because this represents the standard axis of all dimensions.When we multiply a matrix [math]\mathbf {A}[/math] by [math]\mathbf {I}[/math] we get the matrix [math]A[/math] itself.Since the determinant represents the volume of matrix [math]\mathbf {A}[/math], then in this case[math] \mathbf {Det (A * I) = Det (A) * Det (I) = Det (A)}[/math]b) If the cube can be represented by a form with all dimensions [math]\mathbf {=\beta}[/math], that is, a matrix [math]\mathbf {[/math][math]B[/math][math]}[/math] with all its main diagonal [math]= \mathbf {\beta}[/math], then by linearity, and considering that all [math]\mathbf {N}[/math] lines are multiplied by [math]\mathbf {\beta}[/math], we have :[math]\mathbf {Det (A * [/math][math]B[/math][math]) = Det (A * \beta I) = \beta^N Det (A * I) = \beta^ N Det (A)}[/math][math]\mathbf {Det (A) * Det ([/math][math]B[/math][math]) = Det (A)\beta^N Det (I) = \beta^N Det (A)}[/math]c) Any N-dimensional shape represented by a matrix can be broken down into an amount [math]\mathbf {s}[/math] of small cube matrices [math]\mathbf {B_k}[/math] ([math]\mathbf {k}[/math] is an index from 1 to s) of dimension [math]\mathbf {\beta}[/math] (diagonal matrix with only [math]\mathbf {\beta}[/math]value), where [math]\mathbf {\beta}[/math] is arbitrarily small and therefore [math]\mathbf {s}[/math] arbitrarily large.Look at the volume below the colorful surface, above the plane [math]\mathbf {z=0}[/math] (vertical axis), below the plane [math]\mathbf {y=0}[/math] (negative y) to down and left, right to the place [math]\mathbf {x=0}[/math] (positive x). Imagine put inside this volume thousands and thousands ([math]\mathbf {s}[/math]) of tiny cubes.[math]\mathbf {Det(B) = Det(B_1) + ... + Det(B_s)}[/math][math]\mathbf { Det (A) * Det ([/math][math]B[/math][math]) = Det (A) * (Det (B_1) + ... + Det (B_s)) = s Det (A) \beta^N \qquad}[/math] (c1)[math]\mathbf { Det (A * [/math][math]B[/math][math]) = Det (A * (B1 + ... + B_s)) = Det (A * B_1) + ... + Det (A * B_s)} [/math]By (b) above[math]\mathbf {= \beta^N Det(A) + ... + \beta^N Det(A)\qquad}[/math] ([math]s[/math] times)[math]\mathbf {= s Det (A) \beta^N \qquad}[/math](c2)So by (c1) and (c2)[math] \mathbf {Det (A * [/math][math]B[/math][math]) = Det (A) * Det ([/math][math]B[/math][math])}[/math][math]\bigstar \bigstar \bigstar[/math]Notice that based on a few properties, which make very logical in the context of the geometric interpretation of determinants, we went able to deduce the Laplace Expansion and the important property above that relates Linear Transformations with Determinants.On the other hand, when a determinant is defined by the Laplace Expansion, that seems convolute for the layman, all properties are derived from it, including the geometric interpretation above. (and it is not an easy proof because it depends on many matrix transformations).However this is an inverted approach because Laplace Expansion is a consequence from geometric properties of determinants that is highly useful and desirable to Linear Algebra, not the opposite (Laplace Expansion would be an exoteric truth and, thanks God, geometric interpretation emerges as a derivation.The conventional way it’s a very demotivating approach for those who study Math.
Can one define a cross product of a vector "a" of length 3 and 3x3 matrix (second-order tensor) "A", such that result is 3x3 matrix "B" whose columns are cross-products of "a" and columns of "A?
I realize that I'm not adding much to the conversation, but since you asked in a comment to Ron Davis's answer about the form of his solution without regard for contravariant and covariant indices, i.e., the cartesian component form of it, I think it would be[math]\begin{align}B_{km} = \epsilon_{kij}a_i A_{jm} \equiv (a \times A)_{km}\,.\end{align} \tag*{}[/math]An explicit form of the matrix cross product so defined can be written as[math]\begin{align}B & = a \times A, \notag \\&= \begin{bmatrix}0 & -a_3 & a_2\\ a_3 & 0 &-a_1\\ -a_2 & a_1 & 0\end{bmatrix} \begin{bmatrix}A_{11} & A_{12} & A_{13}\\ A_{21} & A_{22} & A_{23}\\ A_{31} & A_{32} & A_{33}\end{bmatrix}, \notag \\&= \begin{bmatrix} a_2A_{31} - a_3A_{21} & a_2A_{32} - a_3A_{22} & a_2A_{33} - a_3A_{23} \\a_3A_{11} - a_1A_{31} & a_3A_{12} - a_1A_{32} & a_3A_{13} - a_1A_{33} \\ a_1A_{21} - a_2A_{11} & a_1A_{22} - a_2A_{12} & a_1A_{23} - a_2A_{13} \end{bmatrix} \,.\end{align} \tag*{}[/math]The matrix [math]a[/math] is the antisymmetric matrix whose elements are the cartesian components of vector [math]\vec{a},[/math] arranged to give the components of the usual vector cross product when multiplying with the column matrix of components of a vector [math]\vec{b}.[/math]Regarding your comment to Vance Faber's answer, I'm not sure that the above is much help in generalizing the idea in the direction you suggest there.
- Home >
- Catalog >
- Business >
- Job Application Form >
- Subway Job Application Form >
- Subway Employment Form >
- subway job application online >
- Form Vec B 31