How to Edit and fill out Behavior Frequency Data Sheet One Behavior Month Online
Read the following instructions to use CocoDoc to start editing and finalizing your Behavior Frequency Data Sheet One Behavior Month:
- In the beginning, look for the “Get Form” button and press it.
- Wait until Behavior Frequency Data Sheet One Behavior Month is appeared.
- Customize your document by using the toolbar on the top.
- Download your completed form and share it as you needed.
An Easy-to-Use Editing Tool for Modifying Behavior Frequency Data Sheet One Behavior Month on Your Way
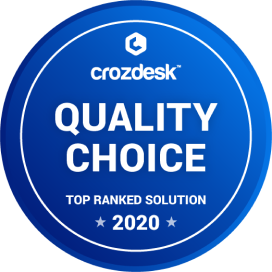
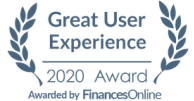
Open Your Behavior Frequency Data Sheet One Behavior Month with a Single Click
Get FormHow to Edit Your PDF Behavior Frequency Data Sheet One Behavior Month Online
Editing your form online is quite effortless. You don't need to download any software on your computer or phone to use this feature. CocoDoc offers an easy tool to edit your document directly through any web browser you use. The entire interface is well-organized.
Follow the step-by-step guide below to eidt your PDF files online:
- Search CocoDoc official website on your laptop where you have your file.
- Seek the ‘Edit PDF Online’ option and press it.
- Then you will browse this page. Just drag and drop the form, or choose the file through the ‘Choose File’ option.
- Once the document is uploaded, you can edit it using the toolbar as you needed.
- When the modification is finished, press the ‘Download’ option to save the file.
How to Edit Behavior Frequency Data Sheet One Behavior Month on Windows
Windows is the most widely-used operating system. However, Windows does not contain any default application that can directly edit template. In this case, you can download CocoDoc's desktop software for Windows, which can help you to work on documents quickly.
All you have to do is follow the instructions below:
- Download CocoDoc software from your Windows Store.
- Open the software and then choose your PDF document.
- You can also choose the PDF file from OneDrive.
- After that, edit the document as you needed by using the varied tools on the top.
- Once done, you can now save the completed form to your cloud storage. You can also check more details about how to edit PDF here.
How to Edit Behavior Frequency Data Sheet One Behavior Month on Mac
macOS comes with a default feature - Preview, to open PDF files. Although Mac users can view PDF files and even mark text on it, it does not support editing. By using CocoDoc, you can edit your document on Mac without hassle.
Follow the effortless instructions below to start editing:
- To start with, install CocoDoc desktop app on your Mac computer.
- Then, choose your PDF file through the app.
- You can select the template from any cloud storage, such as Dropbox, Google Drive, or OneDrive.
- Edit, fill and sign your file by utilizing some online tools.
- Lastly, download the template to save it on your device.
How to Edit PDF Behavior Frequency Data Sheet One Behavior Month via G Suite
G Suite is a widely-used Google's suite of intelligent apps, which is designed to make your work faster and increase collaboration across departments. Integrating CocoDoc's PDF editing tool with G Suite can help to accomplish work easily.
Here are the instructions to do it:
- Open Google WorkPlace Marketplace on your laptop.
- Search for CocoDoc PDF Editor and download the add-on.
- Select the template that you want to edit and find CocoDoc PDF Editor by selecting "Open with" in Drive.
- Edit and sign your file using the toolbar.
- Save the completed PDF file on your computer.
PDF Editor FAQ
What are some real-world examples of applications of machine learning in the field?
Machine learning plays a very crucial role in today’s time knowing well how it has changed and improved how various processes of different industries run.Below are various applications of machine learning according to Machine Learning: Real-World Applications - DZone AIImage RecognitionOne of the most common uses of machine learning is image recognition. There are many situations in which you can classify the object as a digital image. For digital images, the measurements describe the outputs of each pixel in the image.In the case of a black and white image, the intensity of each pixel serves as one measurement. So, if a black and white image has N*N pixels, the total number of pixels and hence measurement is N2.In the colored image, each pixel is considered as providing three measurements of the intensities of three main color components, i.e. RGB. So in the N*N colored image, there are three N2 measurements.Face detection: The category might be face present vs. no face present. There might be a separate category for each person in a database of several individuals.Character recognition: We can segment a piece of writing into smaller images, each containing a single character. The categories might consist of the 26 letters of the English alphabet, the ten digits, and some special characters.Speech RecognitionSpeech recognition (SR) is the translation of spoken words into text. It is also known as automatic speech recognition (ASR), computer speech recognition, or speech to text (STT).In speech recognition, a software application recognizes spoken words. The measurements in this application might be a set of numbers that represent the speech signal. We can segment the signal into portions that contain distinct words or phonemes. In each segment, we can represent the speech signal by the intensities or energy in different time-frequency bands.Although the details of signal representation are outside the scope of this article, we can represent the signal by a set of real values.Speech recognition applications include voice user interfaces. Voice user interfaces include voice dialing, call routing, and domotic appliance control. It can also be used for simple data entry, preparation of structured documents, speech-to-text processing, and planes.Medical DiagnosisML provides methods, techniques, and tools that can help solving diagnostic and prognostic problems in a variety of medical domains. It is being used to analyze important clinical parameters and their combinations for prognosis, i.e. predicting disease progression, extracting medical knowledge for research, therapy planning, support, and overall patient management. ML is also being used for data analysis, such as detection of regularities in data by appropriately dealing with imperfect data, for interpreting continuous data used in the Intensive Care Unit, and for intelligent alarming, resulting in effective and efficient monitoring.It is argued that the successful implementation of ML methods can help the integration of computer-based systems in the healthcare environment providing opportunities to facilitate and enhance the work of medical experts and ultimately to improve the efficiency and quality of medical care.In medical diagnosis, the main interest is in establishing the existence of a disease followed by its accurate identification. There is a separate category for each disease under consideration and one category for cases in which no disease is present. Here, machine learning improves the accuracy of medical diagnosis by analyzing data of patients.The measurements in this application are typically the results of certain medical tests (i.e. blood pressure, temperature, blood tests), medical diagnostics (such as medical images), the presence/absence/intensity of various symptoms, and basic physical information about the patient (age, sex, weight, etc.). On the basis of the results of these measurements, the doctors narrow down on the disease inflicting the patient.Statistical ArbitrageIn finance, statistical arbitrage refers to automated trading strategies that are typical for the short-term and involve a large number of securities. In such strategies, the user tries to implement a trading algorithm for a set of securities based on quantities such as historical correlations and general economic variables. These measurements can be cast as a classification or estimation problem. The basic assumption is that prices will move toward a historical average.We apply machine learning methods to obtain an index arbitrage strategy. In particular, we employ linear regression and support vector regression (SVR) onto the prices of an exchange-traded fund and a stream of stocks. By using principal component analysis (PCA) in reducing the dimension of feature space, we observe benefits and note the issues in the application of SVR. To generate trading signals, we model the residuals from the previous regression as a mean reverting process.In the case of classification, the categories might be sold, bought, or not touched for each security. In the case of estimation, one might try to predict the expected return of each security over a period of time. In this case, one typically needs to use the estimates of the expected return to make a trading decision (buy, sell, etc.)Learning AssociationsLearning association is the process of developing insights into various associations between products. A good example is how seemingly unrelated products may reveal an association to one another when analyzed in relation to the buying behaviors of customers.This application of machine learning involves studying the association between the products people buy and is also known as basket analysis. If a buyer buys X, would they buy Y because of a relationship that can be identified between them? Knowing these relationships could help in suggesting an associated product to the customer. For a higher likelihood of the customer buying it, it can also help in bundling products for a better package.This learning of associations between products by a machine is called learning associations. Once we find an association by examining a large amount of sales data, big data analysts can develop a rule to derive a probability test in learning a conditional probability.ClassificationClassification is the process of placing each individual from the population under study in many classes. This is identified as independent variables.Classification helps analysts use measurements of an object to identify the category to which that object belongs. To establish an efficient rule, analysts use data. Data consists of many examples of objects with their correct classification.For example, before a bank decides to disburse a loan, it assesses customers on their ability to repay the loan. By considering factors such as customer's earning, age, savings, and financial history, we can do it. This information is taken from the past data of the loan. Hence, the seeker uses this data to create a relationship between customer attributes and related risks.PredictionConsider the example of a bank computing the probability of a loan applicant faulting the loan repayment. To compute the probability of the fault, the system will first need to classify the available data in certain groups. It is described by a set of rules prescribed by the analysts.Once we do the classification, as per needs, we can compute the probability. These probability computations can compute across all sectors for varied purposesThe current prediction is one of the hottest machine learning algorithms. Let's take an example of retail. Earlier, we were able to get insights like sales reports from last month/year/5 years/Diwali/Christmas/etc. This type of reporting is called historical reporting. But currently, business is more interested in finding out what their sales will be next month/year/Diwali/etc. This way, businesses can make required decisions (related to procurement, stocks, etc.) on time.ExtractionInformation extraction (IE) is another application of machine learning. It is the process of extracting structured information from unstructured data — for example, web pages, articles, blogs, business reports, and e-mails. The relational database maintains the output produced by the information extraction.The process of extraction takes input as a set of documents and produces structured data. This output is in a summarized form such as an Excel sheet or a table in a relational database.Extraction has become key in the big data industry.As we know, a huge volume of data is generated all the time, and most of this data is unstructured. The first key challenge is handling unstructured data. Now, the conversion of unstructured data to structured form based on some pattern so that the same can stored in RDBMS.Apart from this, in current days, data collection mechanisms are also changing. Previously, we collected data in batches like end-of-day (EOD), but now, business want the data as soon as it is getting generated, in real-time.RegressionWe can apply machine learning to regression, as well.Assume that x= x1, x2, x3, ... xn are the input variables and y is the outcome variable. In this case, we can use machine learning technology to produce the output (y) on the basis of the input variables (x). You can use a model to express the relationship between various parameters as below:Y=g(x) where g is a function that depends on specific characteristics of the model.In regression, we can use the principle of machine learning to optimize parameters and to cut the approximation error and calculate the closest possible outcome.We can also use machine learning for function optimization. We can choose to alter the inputs to get a better model. This gives a new and improved model to work with. This is known as response surface design.Machine Learning: Real-World Applications - DZone AIHands-on Project - Data Preparation, Modeling & Visualization
How can statistics tell us about causality?
It really can't. Along with the excellent technical answers already listed, I'd like you to do a thought experiment with me.Let's say you own a nice car. It's got a great paint job, it's in great shape, and you like to keep it cleaned and detailed. You have to wash it more than you want to, because it seems like whenever you get the car clean, it rains or you get mud on it one way or the other. After a year or so, you start joking that it seems like the best way to know if it will rain is to clean your car--the rain will follow.Because you're the curious sort, you decide to do a very simple analysis of the two--obviously, washing your car is the independent variable, since it's something you can manipulate, and rain is the dependent variable.You discover, by god, that there is an 89% chance that it will rain within a day of you washing your car, and that the effect is even statistically significant. You are, quite literally, a weather demi-god (or maybe you've upset one).This is usually where people scoff and say "well, we know what causes rain and it obviously isn't whether or not someone washes their car"--good, and they're right. Washing your car is unrelated to the weather.How do we know washing your car cause rain? After all, it meets the bar for being statistically significant AND it's highly correlated using the test of your choice.But why?Why isn't the test good enough by itself?It isn't good enough by itself because testing whether or not something is causal is reliant on things outside the test. In this case, it also happens to be a badly designed test, but the analogy stands.For all the technical reasons listed in other answers, all we have at best are some really suggestive patterns in variation. Decisions on causation are made outside the test, and when you get to a certain point in studying things, you realize that there's a whole lot more "we think this is the way it works" than there is "we're pretty sure." There's actually very little "we know this to be true" in the study of the sciences, and what there is is bound to a specific context and some very specific requirements.Probability is highly, highly context dependent, and so are our measures. I, you, and anyone else who studies probability can set up experiments any way we damn well please and play with the components until we get the answer we like. I've always liked this graph as a demonstration of that:(Thank you, Tyler Vigen. Image link: Spurious Correlations)Look how closely related those two distributions are!What is the presumptive context, here? What is the narrative between per capita consumption of cheese and death by bed sheet entanglement? (The answer is humor, but I'm deliberately trying to make thinking stats measure correlation strange,* because it is, as you learn about stats, an understandable** and yet strange assumption.)The narrative is the non-technical reason you can't use probability to find causality, and I'm deliberately pitching this in layman's English (the technical reasons have been admirably covered, I'm just mopping up.) When you go about setting an experiment up, you're creating an artificial little world that has, at best, a tangential relationship to the bombardment of chance on every day occurrences. You spend months carefully figuring out how to compensate, isolate, manipulate and/or negotiate circumstances so that you can rule out as many things as possible and get as close as you can to the thing you want to measure.The measuring process is effected by you, your choices, the materials involved, a series of global (by which I mean encompassing or effecting all) conditions true of the particular event and population, and things as disparate as regular human error or something you haven't thought of at all.It's also effected by time, un-looked for patterns in variation that may effect the thing you're trying to measure and/or anything you can't keep out. The classic solution is to make sure it's so random in there that anything ordered will stand out.Let's come back to the cheese and bed sheets. Both are from US data, both cover the years 2000-2009, both are drawn from nationally recognized reporting agencies (USDA and the CDC), and both concern human behavior.***What are some general reasons we might be able to rule out cheese causing death by bed sheets or, since this is just frequency, death by bed sheets causing cheese consumption?As an exercise, here are some reasons we might rule out that one of these causes the other:people don't usually eat cheese in bed, putting some physical distance between the cheese and the sheets (We can't place the cheese at the scene of the crime.)people can't eat cheese while dying of bed sheets (Their mouths are busy.)people seem to be able to eat cheese regularly without suffocating (or Wisconsin would be in trouble.)lactose intolerant people, who presumably don't eat cheese, may not be as likely to suffocate in their sheets. We don't know if they suffer the same rate o' death by sheets.suffocation in sheets is much less likely than cheese consumption, comparing per capita data to raw numbers of people who suffocated in their sheets (we can't, of course, rule out cheese sneaking back in there in the middle of the night and offing them as revenge for being chewed on)****Here's a few reasons we might choose to believe that there was a relationship:heightened obesity caused by high per capita cheese consumptionheightened cardiovascular issues caused by high per capita cheese consumptiona rash of stress eating and gym avoidancea rash of cheese eating and overdoses of sleep medicationsmalicious sheets and cheese that just wants to be near you******In order to even measure, you have to start making decisions about what seems likely so you'll know what to measure. You'll have to start introducing other things, as well, like obesity (which, FYI, is not in this data) or sentient cheese.If you want to do so responsibly, you'll use the categories listed in the more technical answers: you'll want Hill's criteria, listed in another answer.You'll notice, however, in Hill's criteria, there are several categories that require you to make judgement calls.For the original thought experiment, involving washing your car and rain, we have temporal precedence, strength, a mild amount of coherence, and that's pretty much it.For cheese and bed sheets, we have strength, consistency, and arguably a biological gradient such that increased cheese consumption is paired with increased death by sheet.The judgement call comes in when we start talking about plausibility. Both examples are, fortunately, fairly likely to be considered implausible, and we can discard them (but the cheese may still be guilty.)In a completely non-technical way, that's one of the fundamental reasons statistics are not useful for causation in the strict (mathematical) sense, just suggestive in a practical sense. It has to be judged, finally, by you, for plausibility.It also helps to compare formal logical causation to this, and I promise I'll stop about the cheese (I make no such promises about sheets).Statistics are like the wilder cousin of mathematics and logic, where the answer is pretty much always "maybe." In logic, and parts of mathematics, there are statements that can be said to be causal in a formal, rigorous fashion. There are two conditions on those causal relationships that determine the nature of their relationship: necessity and sufficiency.To say something is necessary is to say that whatever it is that's happening doesn't happen without the first thing existing and/or being true. To say it is sufficient is to say that the thing that is happening has a truth value that is dependent on the first thing.The classic example from logic is the syllogism: if A, then B.You should read this : if A exists and is true, B exists and is true.In the case of rain and the car, can we say if the car is washed, then it will rain?Obviously not. Ditto for the cheese.We could say, based on the graph, that cheese may be sufficient for death by bed sheets (but should be prepared to be laughed at.) We cannot say, under any circumstances, that if cheese is consumed, death by sheets occurs.It might be sufficient, but it is definitely not necessary. You can have either without the other, AND the frequency suggests that you can have a whole lot of cheese without death by sheet.That is, by the way, a problem at the heart of causation that merits years of really difficult study.The only thing we can say is something about sufficiency. We can almost never say anything about necessity.Imagine, if you will, trying to ensure that both sufficiency and necessity are met in a lab experiment.When you're done with the headache (and I do encourage you to actually try, because it's important to get your hands dirty with this issue), you should take a moment and appreciate the messy beauty that is getting to apply a subject of any rigor to causation in a complex field of variation.Take a day at some point and start trying to track the variables converging on your cup of coffee/tea/cocoa and its appearance in your hand. Statistics is a subject for people who feel comfortable, or at least are willing, to look infinity in the eye.And don't worry--I won't say it gets easier (because it's always infinity and its looking right at you), but you do learn to navigate more skillfully.If you're up to it, I highly recommend reading some of the stats classics (particularly John Maynard Keyes' A Treatise on Probability and his fisking of LaPlace, and the more modern Probability Theory: The Logic of Science by E.T. Jaynes.)There. It's probably more than you ever wanted to know, but I hope it was helpful.___________________________* Probability is the answer to the question "how can I make my world utterly unstable." The whole answer is "by studying whether or not something happens rather than assuming it does."The madness sets in shortly after you start having to prove methods and by the time you're writing a dissertation, you're a lost cause.I escaped into engineering, which is surely more sane.Ha. Ha ha. HAHAHAHAHA----oh god, I'm procrastinating studying Taylor series and writing self assembling trees in C..........................* help *** As you study stats, you go groping about in a vast sea of strangeness, looking for landmarks. We want it to be causal because the sea is vast, dark, and full of sharks who have a nasty habit of doing things like pointing out that the molecules in our boat are only conveniently together.*** If it concerns humans, you do the best you can to isolate, but you are just not going to get fully consistent data. Humans are messy little patterns with a loose, if recognizable, organizing structure for their behaviors.**** I'm lactose intolerant. Trust me, cheese is vengeful.***** If cheese just wants to be near you, be very careful. It might be preparing to suffocate you in your bed sheets.
What are the SEO benefits of using a blog for a website?
Blogging and SEO go hand in hand.In essence, content marketing is all about storytelling - and it's been around ever since humans could speak.In the last 5 years, however, content marketing has gained tremendous momentum.From a killer blog post to a killer Tweet, content marketing has become one of the most effective ways for businesses to reach their target audience and drive traffic to their websites.Search engines were around before this surge in content creation. And, they remain as important as ever, continuing to deliver the most useful, relevant information to users - many of them your target audience.But a myth floats around in many marketing circles when it comes to search engines and content.It goes like this:SEO and content marketing are the same.That, essentially, you can use the terms interchangeably. That, if you do one, then you don’t need the other. That, if you have an SEO consultant working on your marketing strategy, then there's no need for an actual content marketer.Uhmm…Not always.Sure, content marketing and SEO are both partners working on the same team.Both have their own talents and ability to put together a kick ass marketing strategy that brings a business results. And both know that search engines are the most powerful channels for delivering readers to the content that's produced.What's more? SEO and content marketing bring staple, repeat traffic to your website and foster relationships with your target audience.But, while content marketing and SEO can work together brilliantly, savvy marketers know that each has a distinct role in bolstering a company's visibility.Content can exist without SEO, but SEO brings eyeballs to that content. And where would an SEO consultant find himself--in this modern era of search engine optimization--without a great content marketer who knows how to write high-quality content?Take it from Rand Fishkin, who writes,It’s a ‘huge challenge to get high authority links without content marketing.'I’ve shown you 4 tactics to build links without writing fresh content.But there are limitations to them.In this response, I'm going to provide you with an SEO cheat sheet that boosts your content marketing efforts and improving your overall blogging strategy.1. Google Hummingbird brought this important factor into focus, in its continued effort to take care of its usersBack in the day, many an SEO consultant's role was stuffing keywords into one blog post after another in an aim (and game) to boost search results.This gave birth to what's known as 'black hat SEO,' where marketers shunned the human experience and manipulated search engines. A blog post wasn't about engagement and reaching one's target audience through useful information.It was about driving search results.Soon enough, Google launched an attack on black hats with its Panda algorithm update. Then, the search engine forever changed the landscape of SEO and content marketing with the introduction of Hummingbird.The update has introduced a new factor that has dramatically altered the way users interact with search engines - and how both SEO and content marketing strategists do their jobs.I am talking about context.No longer does Google rely on the use of EXACT keywords on a page to judge its relevance and bring you search results. Also? You can no longer rely on stating facts on your webpage to get first page rankings.As a content marketer and SEO strategist, you also need to understand the intent behind the user’s search.Ask “why” people are searching for the information, instead of simply answering “what.”Google relies on the searcher's keyword history, personal data, past behavior and other data, to give searchers the most relevant links to their queries.So, when you search for “Chinese food," you’re understood to be looking for nearby restaurants. And, that’s why Google pops up its map results.But, when you search for “great Chinese food”, Google serves you articles on Chinese cuisines.So, what does context mean for your content marketing strategy?Craft conversational content that reads naturally. Instead of using keywords exactly and repeatedly in your article, break down each word in the query to make it meaningful.Your aim should be to explore the relationships between the individual keywords and make your page match that meaning.Traditional keyword research hasn’t lost its shine. And, you should continue to use keyword research to develop your content subjects and shape your marketing strategy, taking keyword volume into account with each blog post and piece of content you publish.But, also use tools, like FAQFox, to find the exact questions that your audience is searching with. Then, seek to answer them in your blog post with an eye on value and relevancy.Brian Dean used this marketing strategy (although without a tool) to get a berth in the top spot in Google.Another great content marketing tip? Sprinkling LSI keywords (technical word for synonyms) in your content, rather than repeating your primary keyword, also helps Google to understand its theme - while also helping you see a rise in your search results.And, since long-tail keywords tell much more about the searcher, it’s easier to create focused-content for them.Remember, your marketing strategy should be about leveraging a blog post or other piece of content to meaningfully engage with your target audience. Those search results will come naturally if you incorporate long-tail variations of you target keyword. Google will reward you for this marketing effort.But, as much as we can discuss keywords alone, it’s the user demography that really matters in understanding intent and creating real search results.So, as a content marketer, you’ve got to develop a clear profile of your customer.Start by listing their demographic data and major pain points.You also need a better understanding of the kind of keywords that your target demographic is typing inside Google (and their stage in your funnel). Then, target these keywords, providing relevant info.If you’re willing to do some technical wizardry to up your changes of reaching your target audience, then here’s a strategy Andrew Ruegger uses for pulling this demographic data from Google's Keyword Planner.Enter your keyword and click on the ‘Get ad group ideas’ button.Now, you’ll get the following screen with some age, gender and device info.Since these charts are appearing, the data on all keywords is going to be present in the source code. You just need to capture it and get your clean dataset of demographic info.When you collect data for all of the keywords (it took Andrew 4 to 5 hours to collect data for approximately 700 keywords), you can get great insights.Here’s how Andrew's data on “smartphones” looked (they used TIBCO spotfire for visualization).They also created filters for the user’s stage in their funnel.And, here’s the demographic data, filtered by keyword.Andrew found interesting insights from this analysis, including the fact that Google phones and MetroPCS had the largest group of older searchers, while Apple and Samsung had the youngest group (and they were also searched 10% more on mobile devices).Care to dig into the demographic data for your keywords? It might one of the top content marketing strategies around.2. Connect your content with a larger audience with keyword dataThe major reason for performing keyword research isn’t to please search engines, even if it will have an impact on your search results.Instead, this content marketing strategy is about finding the EXACT phrases being used by your target audience to express their pain points, then incorporating them into your blog post and other content so that it resonates with your audience.Copyblogger sums it up aptly, “Keyword Research is essentially market research.”When you write in the language that your audience is using in search engines, you’ll not just end up writing engaging content, but you’ll also get higher search traffic.Suppose you, as a content marketer, decided to use the phrase “power toothbrush,” in your company's new dental product description.But, when you plugged the keyword in the Keyword Planner, you found that people are searching for a related term “electric toothbrush,” 37 times more.Given that both the keywords are relevant to your product/content theme:Isn’t it a no-brainer which keyword you should incorporate in your product copy or blog post?In a practical content marketing scenario, you’ll also need to assess the keyword competition (off-page SEO), as well as the on-page factors.Only then can you find the right keywords that are within your reach and that make sense for you to target.Low competition keywords generally contain pages that have Page Authority and Domain Authority that is less than 10 (as displayed by Mozbar) in search results.Most industries won’t have such low authority domains in the search results (at least on the first page).You’ll most likely be competing with brands. So, you also need to assess the type of content that’s ranking for your target keyword right now.Take your marketing effort further by finding the right balance between your resources, budget and desirable traffic. I show you the 4 steps for effective keyword analysis here.3. Find your ideal content format for every piece and reflect on your content frequencyThere are so many ways to present your content. You can write a blog post, craft an infographic, produce a video, record a podcast and do so much more.How do you decide the ideal format for every piece?There are a couple of ways. Let’s look at both of them.1. Look at the existing pieces of content that rank for your target keyword (as we have discussed in point #2 above).Let's suppose that your target keyword is “how to create an infographic.” Then, you’ll find the following results.As you can see, people are mainly looking for tools to create infographics. If you don’t have an infographic creation tool, then you can either compile a list of creation tools, like Creativebloq, or share templates for easy creation, like HubSpot.Another great content marketing strategy? Consider creating a video tutorial.Note that if your target keyword competition consists of eCommerce listings, then you can't beat it with a piece of content. So, drop the keyword from your targeting list.Overall, as I mentioned in point #1, you’ll need to serve the user, first and foremost, by finding his or her intent behind the search.2. Look at your historical analytics data.When I plug my website inside BuzzSumo, I can clearly see that my target audience loves reading about tools (2 articles on tools made it to the top).Further, they like in-depth articles - all of the above 4 articles are 4000+ words. (Unless you're Seth Godin, gone are the days when a 300-500 word blog post worked.) Including the comments and other text, all of the webpages contain at least 6000 words.Similarly, at Quick Sprout, 3 of my top 5 posts have been about tools.If you’re eager about exact numbers, then I would suggest that you log into Google Analytics and find out the characteristics of posts that have received maximum conversions.Note: You also need to consider your content marketing goals and available resources for choosing a format. I used to publish many infographics at Quick Sprout. But, I noticed their fading ROI when many marketers started using them.So, even though some of my most popular posts were infographics, I stopped producing them. Instead, I pivoted to mostly long-form content, which provides a better ROI. In other words? Stay apprised of your industry.Next, you also need to decide the content frequency that's wanted by your target audience.As I’ve already shown you in my content frequency article, if you get a majority of your traffic from search engines, then you can get away with posting a smaller number of articles.But, if you’re a viral website relying on social media for your traffic (think BuzzFeed), then you’ll need to pump out much more content.Find out the breakdown of your traffic numbers from your Google Analytics reports and start with a frequency that suits your budget and available time.Once comfortable with your publishing schedule, I would recommend performing a test to find out if your optimal publishing frequency is higher or lower.In any case, one content marketing strategy that should never be ignored is this: pushing out more quality content is helpful for giving you more chances to rank in search engines.Depending on the format of your content, you may need to stay more or less consistent in your publishing.Note that crafting a piece of content isn’t a one-time thing, either. HubSpot repurposes one piece into different formats, to appeal to different personas in different stages of their funnel.4. Perform regular SEO audits and technical SEO optimizationOnce your content marketing efforts have gained momentum, you should regularly check your search console and GA reports.Find the keywords for which your content is getting ranked and discovered.Then, be sure that you incorporate long-tail and LSI versions of these keywords in your content.Such content 'spring cleaning' will help you earn more search engine traffic. I’ve used this strategy to grow my traffic from 120,365 visitors to 174,496 visitors, in a year.Finally, I would recommend that you take technical optimization into account.As much as search engines have gotten smarter in understanding content on webpages… you can still take the lead, by leveraging schema markup and fixing user experience issues on your website.HubSpot increased their organic traffic, by over 50%, with 7 technical SEO updates.Similarly, Ahrefs also fixed their broken links, other technical SEO issues and sped up their site.They even deleted 179,158 words worth of content from their blogs.The result?89% growth in search traffic, within 3 months.ConclusionLet me recap the 4 necessary SEO considerations for ensuring stellar content marketing:ContextKeyword volume and competitionContent format and content frequencySEO audit for content spring cleaning and technical SEOOne last, quick tip: To accelerate your content marketing strategy, consider checking out a Google search operator to narrow your search queries and discover prime ideas for link building.Taking care of these aspects will put you ahead of most of the competition - you know, those folks who believe that content marketing and SEO are one and the same. Your overall marketing strategy will flourish because of it.
- Home >
- Catalog >
- Life >
- Parenting Chart >
- Behavior Charts >
- Behavior Tracking Chart >
- behavior data collection sheets >
- Behavior Frequency Data Sheet One Behavior Month