How to Edit Your Funeral Letter Sample Online Easily Than Ever
Follow the step-by-step guide to get your Funeral Letter Sample edited with ease:
- Hit the Get Form button on this page.
- You will go to our PDF editor.
- Make some changes to your document, like highlighting, blackout, and other tools in the top toolbar.
- Hit the Download button and download your all-set document into you local computer.
We Are Proud of Letting You Edit Funeral Letter Sample With the Best Experience
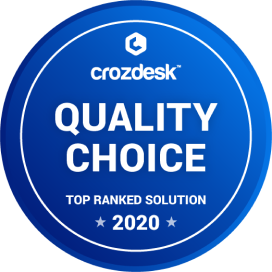
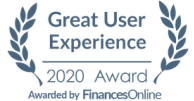
How to Edit Your Funeral Letter Sample Online
If you need to sign a document, you may need to add text, fill out the date, and do other editing. CocoDoc makes it very easy to edit your form just in your browser. Let's see how do you make it.
- Hit the Get Form button on this page.
- You will go to CocoDoc PDF editor web app.
- When the editor appears, click the tool icon in the top toolbar to edit your form, like adding text box and crossing.
- To add date, click the Date icon, hold and drag the generated date to the target place.
- Change the default date by changing the default to another date in the box.
- Click OK to save your edits and click the Download button for sending a copy.
How to Edit Text for Your Funeral Letter Sample with Adobe DC on Windows
Adobe DC on Windows is a useful tool to edit your file on a PC. This is especially useful when you finish the job about file edit in your local environment. So, let'get started.
- Click the Adobe DC app on Windows.
- Find and click the Edit PDF tool.
- Click the Select a File button and select a file from you computer.
- Click a text box to change the text font, size, and other formats.
- Select File > Save or File > Save As to confirm the edit to your Funeral Letter Sample.
How to Edit Your Funeral Letter Sample With Adobe Dc on Mac
- Select a file on you computer and Open it with the Adobe DC for Mac.
- Navigate to and click Edit PDF from the right position.
- Edit your form as needed by selecting the tool from the top toolbar.
- Click the Fill & Sign tool and select the Sign icon in the top toolbar to customize your signature in different ways.
- Select File > Save to save the changed file.
How to Edit your Funeral Letter Sample from G Suite with CocoDoc
Like using G Suite for your work to complete a form? You can make changes to you form in Google Drive with CocoDoc, so you can fill out your PDF just in your favorite workspace.
- Go to Google Workspace Marketplace, search and install CocoDoc for Google Drive add-on.
- Go to the Drive, find and right click the form and select Open With.
- Select the CocoDoc PDF option, and allow your Google account to integrate into CocoDoc in the popup windows.
- Choose the PDF Editor option to open the CocoDoc PDF editor.
- Click the tool in the top toolbar to edit your Funeral Letter Sample on the needed position, like signing and adding text.
- Click the Download button to save your form.
PDF Editor FAQ
How do I write a letter to my head teacher to ask for permission to attend my aunt's burial?
check these images. pick the one you need.Preview of funeral excuse letter for teachersyou can also check this outPreview of Excuse Letter to Attend Funeral - Sample Letters for Word & School
Is there any mathematical proof on why deep learning works?
I’ve answered a similar question before, but I’ll give it another whirl. As I have explained earlier, it all depends on what you mean by “works”. Depending on how you define that word, deep learning either “works” or it doesn't “work”. Let’s clarify with some examples.“Work” is defined as performance at labeling a pre-defined image or speech dataset, using test samples drawn from the same distribution as the training samples (e.g., the Imagenet task). In this case, it is clearly the case that deep learning “works”, in the sense that error rates are much lower than competing approaches (so far). Now, we get to the interesting part of your question: “why” does deep learning “work” when it does? This is a much harder question, and so far, there is no clear answer. The problems with coming up with a succinct explanation are many: deep learning involves gradient search in a highly non-convex very high dimensional space. Little can be said about finding any optima in such spaces using greedy methods. Some recent work has shown that deep learning “works” because saddle points are much more common than genuine local optima in very high dimensional spaces (because a local optima would require the error surface to increase along every dimension, which becomes rarer as the dimensionality gets into the millions or billions of dimensions). But, no one has given a clear explanation of what the dozens of layers in a deep Imagenet network are doing, other than the obvious one of the definition of the nodes in the network. There is a recent framework called “information bottleneck” that uses the power of information theory to shed some light on this matter. It looks promising, but it’s far from certain that it is the right framework. Why is this important? Well, recent work has shown that adding minute amounts of adversarial random noise is sufficient to throw off a deep learning image labeler, so that what it previously reliably labeled as a “dog” gets relabeled as a “computer”. This is bizarre behavior, because the amount of noise added is so small that humans don't even see the noise in the image. This is a perfect example of what Einstein called a “gedankenexperiment” (a thought experiment). To me, this suggests that no matter what the virtues of deep learning for vision may be, it cannot be how human vision works, because the failure modes are entirely different. Humans do not get fooled by small amounts of imperceptible random noise in an image, because our vision was evolved in the real world to keep us safe from predators. If an early human being could not reliably tell a “tiger” from a “cow”, he or she would get eaten. Simple as that. So, very quickly, biology evolved such that perception is highly reliable and works under all kinds of conditions (you can recognize a tiger is approaching even it is raining, or it is cloudy or it is sunny).“Work” is defined as solving some specific task, e.g. learning to play Atari video games or other types of games such as Go or backgammon. In this case, the report card for deep learning is a bit mixed. Certainly, the work on Alpha Go (Zero) by Deep Mind is a sensational demonstration of the power of deep learning, but it was combined with many other tricks (sampling methods for estimating utility functions from rollouts, reinforcement learning algorithms, etc.). In Atari, some games are learned extremely well, better than human level performance, but require a sample complexity of millions of steps of training, about 1000x the number that humans need to learn these games (from recent studies done at MIT and Berkeley). Games that are trivial for humans — for example, Montezuma’s revenge — are completely beyond the first deep reinforcement learning systems, although newer approaches that use other tricks are beginning to make a dent. The real problem here is that humans look at a video screen and “see” the environment at a far richer level than any deep learning system. They understand causality, they understand geometry, they understand what “monsters” are, what “ladders” are and all the common objects simulated in the environment (keys, doors, etc.). Deep RL does not understand anything about the domain when it starts to learn. Each image is just a vector of pixels, which are essentially meaningless. So, in some very obvious way, the problem being solved by deep RL systems when they learn to play Atari is completely fictitious since humans don't solve the problems the same way. Consequently, as the Berkeley and MIT studies have shown, humans learn some of these video games in minutes, and deep RL systems take far far longer, and sometimes fail completely. So, here, the question of whether deep learning works is not yet proven. I would say the same is true in natural language processing, where deep learning approaches are being actively studied, but haven’t quite made the same impact as they have in vision and speech.“Work” is defined as being able to match the learning capacity of a normal human child, as he or she acquires full functionality in perception and object recognition, language, motor skills, social interaction, tool use, commonsense causal knowledge, and a dozen more areas. Here, which many would view as the true test for AI systems, we are arguably completely in the dark. Deep learning is too sample hungry to be even remotely biologically plausible — for example, a child can recognize the big yellow McDonald sign in just a few sightings. A child requires perhaps one or two examples to recognize what a dog is or what a cat is. It is routine for deep learning systems to require tens of thousands of samples before they can reliably recognize letters or digits. Some work at MIT has shown that part of the problem is that humans are extraordinarily good at understanding general concepts like symmetry. So, if you present objects in a very canonical normalized form, even simple methods like nearest neighbors achieve high accuracies after dozens of examples. However, if objects are seen “in the wild” in any configuration, the performance drops down to random guessing even after 50 examples. So, humans are amazingly good at ‘subtracting” out “confounders” like the orientation of an object or its apparent size from the essence of what the object is. If you look at the work of developmental researchers like Jean Piaget in Switzerland, who is the true pioneer in studying children’s cognitive abilities as they grow, or more recent work by Alison Gopnik at Berkeley, you come away with the clear impression that there is so much more going on “under the hood” in a child than pure deep learning style feature learning. Children actively experiment with the world, acting like scientists, forming hypotheses and using their actions in the world to prove or disprove them. One of the major conclusions from Piaget’s work is that learning in a child proceeds in phases, and children all over the world seem to show the same phases (since the experiments he pioneered are so simple they can be done in any school or home environment, and his studies have been replicated many times). At some point, children learn the concept of what an “object” is, and what the relations among objects are. This type of abstract learning is completely beyond a deep learning system currently, since they can be taught to recognize concrete objects like “car” or “tiger”, but not the abstract concept of an object. Similarly, children learn causality very early in life, and know when a phenomenon is normal and follows the laws of physics, vs. when it is somewhat strange (like an object that when dropped, rises instead of falling down).So, where does this leave AI? Well, to use a well-known pun, we are caught between a “rock” and a “hard place”. On the one hand, deep learning works well enough in many areas that it can be used to build self-driving cars (the recent failures here are worrying of course), train speech recognition systems and language modeling systems (the recent Google Duplex demo probably used deep learning), and play video games etc. So, there is enough financial incentive to continue investing in deep learning and using it in lots of real world applications. However, on the flip side, its limitations are becoming all the more apparent and its lack of biological plausibility is also a source of concern. But, a true competitor has not yet emerged, and this is the great opportunity that awaits the new generation of AI researchers. As the deep learning pioneer Geoff Hinton himself said, “science proceeds one funeral at a time”, and undoubtedly deep learning will get its burial at some point when a better approach is invented. Going back over the last three decades of my involvement in AI and ML, I have seen many approaches rise and fall, and there is no reason to believe that deep learning is here to stay. But, it might be for many tasks the current best thing we can do, while we explore and try to invent a better solution.
What is the most inappropriate clothing you have seen someone wear at a funeral?
Yes. I was standing in honor of a U.S. Marine, who was KIA, with my brother and sister Patriot Guard Riders. People were coming in with their pants hanging below their ass, oversized hockey shirts, raggedy jeans. One woman was dressed in a strapless sparkly dress the type for clubbing, hose, spiked heels with her bra traps showing.One woman who I originally thought looked very presentable had the words SAMPLE in big block letters down the back of her black pants outfit.What really pissed me off was when the widow and her 2 little kids pulled up in the funeral limo, the morons gathered around her snapping pictures like obnoxious paparazzis.
- Home >
- Catalog >
- Business >
- Letter Template >
- Donation Letter Sample >
- Charity Donation Email >
- donation email sample >
- Funeral Letter Sample