How to Edit and draw up Practice B Graphing Relationships Online
Read the following instructions to use CocoDoc to start editing and finalizing your Practice B Graphing Relationships:
- Firstly, find the “Get Form” button and press it.
- Wait until Practice B Graphing Relationships is ready.
- Customize your document by using the toolbar on the top.
- Download your finished form and share it as you needed.
The Easiest Editing Tool for Modifying Practice B Graphing Relationships on Your Way
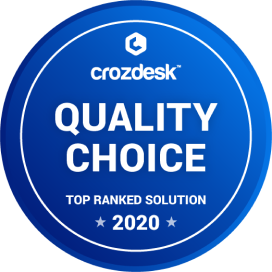
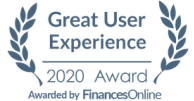
How to Edit Your PDF Practice B Graphing Relationships Online
Editing your form online is quite effortless. No need to download any software through your computer or phone to use this feature. CocoDoc offers an easy tool to edit your document directly through any web browser you use. The entire interface is well-organized.
Follow the step-by-step guide below to eidt your PDF files online:
- Browse CocoDoc official website on your device where you have your file.
- Seek the ‘Edit PDF Online’ icon and press it.
- Then you will open this free tool page. Just drag and drop the form, or upload the file through the ‘Choose File’ option.
- Once the document is uploaded, you can edit it using the toolbar as you needed.
- When the modification is completed, tap the ‘Download’ option to save the file.
How to Edit Practice B Graphing Relationships on Windows
Windows is the most conventional operating system. However, Windows does not contain any default application that can directly edit form. In this case, you can download CocoDoc's desktop software for Windows, which can help you to work on documents effectively.
All you have to do is follow the steps below:
- Install CocoDoc software from your Windows Store.
- Open the software and then append your PDF document.
- You can also append the PDF file from Dropbox.
- After that, edit the document as you needed by using the a wide range of tools on the top.
- Once done, you can now save the finished PDF to your computer. You can also check more details about how to edit pdf in this page.
How to Edit Practice B Graphing Relationships on Mac
macOS comes with a default feature - Preview, to open PDF files. Although Mac users can view PDF files and even mark text on it, it does not support editing. Through CocoDoc, you can edit your document on Mac without hassle.
Follow the effortless guidelines below to start editing:
- In the beginning, install CocoDoc desktop app on your Mac computer.
- Then, append your PDF file through the app.
- You can upload the form from any cloud storage, such as Dropbox, Google Drive, or OneDrive.
- Edit, fill and sign your template by utilizing some online tools.
- Lastly, download the form to save it on your device.
How to Edit PDF Practice B Graphing Relationships on G Suite
G Suite is a conventional Google's suite of intelligent apps, which is designed to make your workforce more productive and increase collaboration with each other. Integrating CocoDoc's PDF file editor with G Suite can help to accomplish work handily.
Here are the steps to do it:
- Open Google WorkPlace Marketplace on your laptop.
- Look for CocoDoc PDF Editor and get the add-on.
- Upload the form that you want to edit and find CocoDoc PDF Editor by selecting "Open with" in Drive.
- Edit and sign your template using the toolbar.
- Save the finished PDF file on your device.
PDF Editor FAQ
What are the differences between a Graph database and a Triple store ?
Graph databases arent completely synonymous with triple stores for these reasons:1. Typically, triple stores are encountered in the RDF/Semantic web context - where the natural storage model for RDF is a list of triples/quads (hence 'triplestore'). The fact that the 'object' of a triple:man a :animal - :animal, in this case, can be the subject of another obviously lends itself to a graph structure, but a specific flavor of graphs - directed, edge-labeled graphs.2. There are clearly more kinds of graphs than just edge-labeled directed graphs - undirected edge weighted graphs are a very common counterexample. The RDF triple model (in current form) doesn't allow for simple representation of such graphs without hoop-jumping with extraneous nodes (blank nodes). Even then, you could argue reasonably that<a> 0.44355 <b> isnt the same graph structure as<a> :hasWeightNode _:blankNode.<b> :hasWeightNode _:blankNode._:blankNode :hasWeight "0.44355".3. Also, the RDF data model allows 'parallel' edges between nodes - 2 subjects can be related to each other via multiple distinct predicates that have no relationship with each other in a semantic sense.4. Triple stores are really just a list of graph edges - but in practice, most of the edges turn out to be spurious - they're usually 'properties' of a node and not first class citizens of the graph structure itself. In RDF, for example:a :hasAge "24".:a foaf:knows :b.are both 'equal' as triples and hence considered graph edges, but only the second one represents connectivity in a graph sense - the first 'edge' isnt really an edge but a property of :a with no meaning outside of :a. In RDF, both are called 'properties' but the first type is called a 'literal' while the second is an 'object property'. In Neo4j (AFAIK) - the distinction is made between relationships (second edge) vs. properties (first edge). The 'Property Graph' model is an alternate abstract data model for graph data - TinkerPop, Neo4j etc are in some sense variants of this model, unlike RDF-based triple stores. If I understand correctly, the key idea in Property Graphs is to explicitly separate out node/edge specific key/value data from the underlying graph structure as a design-time decision.5. Having labeled edges means that your RDF graph is really a composite graph across several edge types - eg a triple store full of edges like:a :hasChild :b:a :isManagerOf :cactually can be thought of as a composite of 2 graphs partitionable by each predicate, unless :hasChild and :isManagerOf are semantically related.The reason #4 and #5 are important is that structural measures like centrality, degree arent immediately meaningful if applied naively on RDF graphs - unless care is taken to separate the 'graphy' portion of the graph from semantic 'noise'. For example, in #5 - the node :a would naively have a degree of 2, but you would reasonably think that :hasChild edges constitute a separate graph from :isManagerOf.6. SPARQL, as someone else has pointed out, is at its core, a declarative language for subgraph pattern matching in RDF graphs. Just as RDF is a particular kind of graph, SPARQL allows a particular kind of graph operation - pattern matching. Triple stores (standalone or built on existing db platforms) are optimized for this operation. SPARQL is certainly capable of post-processing a retrieved subgraph pattern - the latest iteration has support for aggregate-type operations like sum, count, avg etc. However, other graph operations, particularly computing graph-structural metrics like centrality are hard to do in SPARQL since they require some form of iteration/looping - in other words, like vanilla SQL, SPARQL is not Turing-complete. To mitigate this, certain platforms notably TopQuadrant have constructed iterative processing over RDF/triplestores. But the language itself doesnt yet allow constructs like SQL's 'WITH' clause that could be used to do so.
What is an entropy of Graph? Is it related to concept of entropy in Information Theory?
First of all, are entropy and information theory related? Yes, and if they are, they are also in graph theory. To let von Neumann explain this relationship: "Entropy is the difference between the information provided by the macroscopic description and the microscopic one".von Neumann says the entropy of a system measures how much information the modelisation of a system does not provide. With microscopic description we mean a description of the system which coincides with the best one –the one which allows us to identify each element, and to know everything about it. If the system (in our case the graph) provides us with the same amount of information, its entropy is zero. If not, the entropy is bigger than zero.Before going to graphs, let us consider a relatively simple system, like a perfect gas (point-like particles interacting elastically).The best microscopic description of a gas is the one which gives, for each labelled particle, the exact position and speed. If a little demon could provide us a table with position and speed of all particles, we would have zero entropy. (Boltzmann himself uses the imaginary capability of identifying each particle in the ergodic principle, to eventually give a statistical definition of entropy, and soon after Maxwell introduces the knowledgeable demon's paradox).As found by Maxwell, a perfect gas is potentially a zero-entropy system. Given its boundary conditions (position and speed of each particle), we could uniquely identify each particle and follow their evolution, and extract all desired energy from it.Something similar can be applied to graphs. Only, it gets a bit more complex. While a gas is perfectly defined by the properties of its constituents –the pointlike particles– a graph is not.Graphs are defined by the relationships between its constituents. In fact, we use graphs to describe systems which show an emergent properties: the information stored in the whole structure is bigger than the sum of the information stored in each element. This means that we need to look at the whole structure, not at its elements one by one, as we do with gas!To describe a node in a graph, we could use its number of connections, its centrality, its average distance from all nodes, clusterization of its connections et cetera. But not necessarily these properties will univocally describe that node. How can we understand how well a node of a graph is defined by the structure of the graph (aka the topology)?To understand that, let us start having a look at the most trivial graph: a triangle.However precisely one describes a node using the properties of the graph, it is impossible to provide enough information to identify it. All nodes are the same –they have two connected nodes, and those connected nodes are connected between themselves by a single link.More formally, we say that any of the six permutations of the nodes will produce the same graph. Even more formally, there are six adjacency preserving bijections, and the set of these bijections form the automorphism group (a transformation onto itself) of the graph. Graphically, this means that if someone swaps the labels A and B, and "fixes" the graph, in this case rotating the triangle around the high, we would not notice that A and B are now different nodes than earlier. The graph is symmetric under that transformation.We can represent that through matrices. The adjacency matrix of the triangle is:A B CA 0 1 1B 1 0 1C 1 1 0It's clear that if you swap the first two columns and the first two rows, i.e. swap node A with node B, you get the same matrix:B A CB 0 1 1A 1 0 1C 1 1 0In practice, this is a "high entropy" graph, because does not provide much information about its constituents –we better add a label to them, as the graph's topology does not help!Let us take now a more funny, and complex, graph. One with directed connections, and loops, which says "C talked to itself, and A talked twice to B". Graphically:The adjacency graph of the graph is the following:A B CA 0 2 0B 0 0 0C 0 0 1It is evident that there are no permutations which would leave the matrix unchanged. In this case descriptions like "the node with two incoming connections", or "two outcoming", or "in a loop", would leave no doubt about which node we are talking about. The macroscopic description, through the graph, is the same as the microscopic one –with the label. This is a zero-entropy graph!How well we can identify nodes is therefore related to entropy: in this sense, complex graphs, where the connections are particularly "telling", are very informative of their constituents, and store more information than simple ones.We can now compute the entropy according to von Neumann. The uncertainty of a microscopic description (the labelled one) is zero. We therefore only need to compute the uncertainty on the macroscopic one.In our simple non-directed triangular graph, we have six possible permutations of the nodes, therefore[math]S_{full\ triangle} = - log(⅙) = log(6) [/math][math][/math][math] [/math][math][/math][math][/math]We could also see at it in a different way. The uncertainty on the first node is ⅓. Once we know the identity of the first node, the uncertainty on the second is ½, and then the identity of the third node is determined –therefore [math]S_{full\ triangle} = -log (⅓) - log( ½ )- log( 1) [/math][math][/math][math][/math].Nonetheless, we are normally not interested in identifying each node in the graph, but would be happy with less. If you are interested in a certain characteristic of the nodes, and not in identifying them, you can simply refer to the entropy of the probability density function of that characteristic. Say, for instance, you are only interested in knowing the number of connections for each node. As often happen, you don't know the number of connections of each node, but you know that the probability of a node having [math]k[/math] connections is [math]p_k[/math]. In this case, the entropy will be:[math]S_{connections} = [/math][math][/math][math] \sum_k -p_k\cdot log (p_k)[/math]
What is the "important social networking patent" owned by Reid Hoffman and Mark Pincus?
The sixdegrees patent is the fundamental patent of social networking. I had the lucky pleasure of working with Reid and Mark on this so I may be biased — and I am attorney-bound not to disclose client confidences— but as far as I know is the real deal.Let's back up a bit. Social graphs are perhaps 120 years old. Anthropologists, economists, and immunoligists have been making graphs of social relationships since the turn of the 20th Century. The so-called social graph existed without their graphing it, a web of interconnections. The innovation was, they graphed it! They went out in the field, learned about people's (or animals, viruses, or anything) relations with each other, and made a graph of it.What sixdegrees discovered was that instead of generating the graph through observation by experts, you could have a self-creating graph by having the subjects themselves create the connections. Instead of some Harvard anthropologist check off on a paper notebook that person A knows person B, maybe person A just sends person B an email saying "care to join me on LinkedIn?" Person B responds, and voila! You have a link on the social graph.This is one of those patents where you say, no way. This is a fundamental law of mathematics, of understanding things. But then, look at the year. 1990-f*cking-7. Okay, for real, they truly invented this. That means Facebook, google, Microsoft, and more or less everyone else is is practicing the sixdegrees patent.
- Home >
- Catalog >
- Life >
- Log Template >
- Activity Log Template >
- Blank Running Log >
- running log printable >
- Practice B Graphing Relationships