A Premium Guide to Editing The Learning Image Segmentation And Hierarchies By Learning - Mit
Below you can get an idea about how to edit and complete a Learning Image Segmentation And Hierarchies By Learning - Mit step by step. Get started now.
- Push the“Get Form” Button below . Here you would be brought into a splashboard allowing you to make edits on the document.
- Select a tool you like from the toolbar that emerge in the dashboard.
- After editing, double check and press the button Download.
- Don't hesistate to contact us via [email protected] if you need some help.
The Most Powerful Tool to Edit and Complete The Learning Image Segmentation And Hierarchies By Learning - Mit
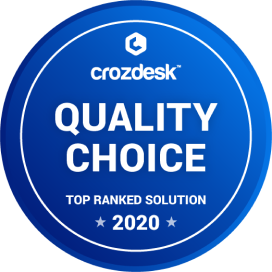
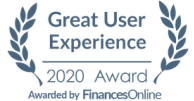
Modify Your Learning Image Segmentation And Hierarchies By Learning - Mit Within seconds
Get FormA Simple Manual to Edit Learning Image Segmentation And Hierarchies By Learning - Mit Online
Are you seeking to edit forms online? CocoDoc can be of great assistance with its powerful PDF toolset. You can utilize it simply by opening any web brower. The whole process is easy and fast. Check below to find out
- go to the CocoDoc's free online PDF editing page.
- Import a document you want to edit by clicking Choose File or simply dragging or dropping.
- Conduct the desired edits on your document with the toolbar on the top of the dashboard.
- Download the file once it is finalized .
Steps in Editing Learning Image Segmentation And Hierarchies By Learning - Mit on Windows
It's to find a default application able to make edits to a PDF document. However, CocoDoc has come to your rescue. View the Guide below to know ways to edit PDF on your Windows system.
- Begin by downloading CocoDoc application into your PC.
- Import your PDF in the dashboard and conduct edits on it with the toolbar listed above
- After double checking, download or save the document.
- There area also many other methods to edit PDF documents, you can check this post
A Premium Guide in Editing a Learning Image Segmentation And Hierarchies By Learning - Mit on Mac
Thinking about how to edit PDF documents with your Mac? CocoDoc has got you covered.. It allows you to edit documents in multiple ways. Get started now
- Install CocoDoc onto your Mac device or go to the CocoDoc website with a Mac browser. Select PDF sample from your Mac device. You can do so by clicking the tab Choose File, or by dropping or dragging. Edit the PDF document in the new dashboard which encampasses a full set of PDF tools. Save the content by downloading.
A Complete Advices in Editing Learning Image Segmentation And Hierarchies By Learning - Mit on G Suite
Intergating G Suite with PDF services is marvellous progess in technology, able to chop off your PDF editing process, making it quicker and more convenient. Make use of CocoDoc's G Suite integration now.
Editing PDF on G Suite is as easy as it can be
- Visit Google WorkPlace Marketplace and locate CocoDoc
- establish the CocoDoc add-on into your Google account. Now you are all set to edit documents.
- Select a file desired by hitting the tab Choose File and start editing.
- After making all necessary edits, download it into your device.
PDF Editor FAQ
I want to learn Artificial Intelligence and Machine learning. Where can I start?
It is a long process and one need to spend few years over 2000 hours in AI and another 2000 hours in Deep Learning to get good at it. It is highly mathematical oriented and parts are driven by biology and those who do not know foundations of Physiology will find great difficulty in relating real world problems in mechanisation.Artificial Intelligence is a broad subject and an old one with research has been done over 60 years based on machines, based on brain it over 100 years old. Operations of a machine mimic some skills of humans have been defined by Artificial Intelligence as first proposed by John McCarthy in 1956. His published a paper called “SOME PHILOSOPHICAL PROBLEMS FROM THE STANDPOINT OF ARTIFICIAL INTELLIGENCE” in 1969, while working in computer science dept., of famous Standford University in Silicon Valley, CA, USA. Source: https://www.csee.umbc.edu/courses/771/spring03/papers/mcchay69.pdfHe said, “ We may regard the subject of AI as beginning with Turing's article Computing Machinery and Intelligence (Turing 1950) and with Shannon's (1950) discussion of howa machine might be programmed to playchess.”Basic view of intelligence is epistemological and the heuristic way according to Macarthy. The epistemological part is the representation of the world in such a form that the solution of problems follows from the facts expressed in the representation. The heuristic part is the mechanism that on the basis of the information solves the problem and decides what to do. Most of the work in articial intelligence so far can be regarded as devoted to the heuristic part of the problem.Source: Minsky, M. (1961), “Steps towards Artificial Intelligence”, Proceedings of the I.R.E., 49, 8-30.Alan Turing created a machine that just can plug in a electrical socket run forever off course doing some really came later but Turning test are critcial in AI. Source: Turing, A.M. (1950), “Computing machinery and intelligence”, Mind, 59, 433-60.First question is how to create an algorithm for an intelligent process, the basis of AI.Next how to formulate a problem or a process. Propose a solution and develop a prototype and test itHow does an eye work lot more complex than an camera. How do photos process in a smart phone is also complex?Storing in a creative way and process thru a microprocessor is the focus now. But select a specific photo is not still there but get photos by date or by group is only possibility now. In Brian, think an incident and close eyes you pictures, HOW? Hypothalamus and Neocortex work synchronizely in CNS to produce is what baffling many scientists. There “ thousands of processes we do how” is the study of AI.There are 7 million rods and more than a million cones in each besides Ganglion Cells, Bipolar cells etc to decide our view into retina eventually store in cortex.The process of getting information, process and transmit is done in any nerve called “Synapse” with Dendrite (with small wires) , Nucleus and Axon (a long part)Lynn Conway, a famous mainframe designer at IBM, also worked at XEROX at Palo Alto and Carver Mead a professor at Cal Tech, a top five research universities i. USA, created AI based eye in latest 1980s but it can only less than 20 percent of our eye. There are the “First” authors VLSI, especially Conway preached MIT, Berkeley, UCLA, etc to teach VLSI. Then came expert systems, neural networks, fuzzy logic etc.Introduction to VLSI Systems by Carver Mead & Lynn Conway, Addison-Wesley Longman Publishing Co., Inc. Boston, MA, USA ©1979ISBN:0201043580This book was cited over 4400 in Research.How you make a machine to bring coffee from a flask. Open the top cup by rotating clockwise, get a mug, bend flask, pour Coffee, close lid, navigates where you are? All thus done now so called Robotics but not cheap.How do we understand this simply process but a complex system is THE foundation of AI.There are variety coffee machines made in USA, while I was there for different dose if coffee or sugar or temperatures of for Indians mix milk. How can AI can be used, a simple way is Fuzzy logic (FL). At a machine, a person takes a coffee he can say coffee need darker or more temp or less sugar. The machine with processor and memory can create new set of values for three variables of coffee, sugar, temperature, a fuzzy way of doing AI. In 1964, Lotfi Zadeh developed FL.Honey Bees collect honey through flowers using earth magnetic field. But how do they find, get back, communicate and establish a nest just like a group of human develop a family. Few algorithms were developed on this and Artificial Neural Networkes were created.Read about Marvin Lee Minsky (August 9, 1927 – January 24, 2016) was an American cognitive scientist concerned largely with research of artificial intelligence (AI)A great book by Gerald M. Edelman, “A Remembered Present” who won a Noble Prize in Medicine.Ex: “Creating and simulating neural networks in the honeybee brain using a graphical toolchai”, http://greenbrain.group.shef.ac.uk/wp-content/uploads/2013/11/SFN_2013_GB.pdfDeep Blue, a super computer made by IBM, almost beat any Grand Master, Vishwanth Anand played many times, list only few times, a great example of a billion dollar AI investment on research.“Artificial Intelligence and Human Thinking “ by Robert Kowalski, Imperial College London United Kingdom [email protected]. According to Kowalski, “Research in AI has built upon the tools and techniques of many different disciplines, including formal logic, probability theory, decision theory, management science, linguistics and philosophy. However, the application of these disciplines in AI has necessitated the development of many enhancements and extensions. Among the most powerful of these are the methods of computational logic. I will argue that computational logic, embedded in an agent cycle, combines and improves upon both traditional logic and classical decision theory. I will also argue that many of its methods can be used, not only in AI, but also in ordinary life, to help people improve their own human intelligence without the assistance of computers.” According him that Abductive Logic Programming form of compututational Logic embeds agent cycle shows in the following figure.A basic paper in AI can be easily accessed throughj Reasearch Gate: Artificial Intelligence by Mariam Khaled Alsedrah, The American University of the Middle East, December 2017. She told that AI works based on several models such as: Ant Colony Algorithm, Immune Algorithm, Fuzzy Algorithm, Decision Tree, Genetic Algorithm, Particle Swarm Algorithm, Neural Network, and Deep Learning.Neural networks deal with higher millions neurons that process information in CNS if Brain so called nerves. They are an essential part of AI nowadays. From a synaptic weight to summation, to back propagation to networking is the complex maze of ANN. I worked on two ANN projects at Motorola in CMOS VLSI. First chip has 1,15,200 logic gates and 2nd chip had over 1 million logic gates and fabricated 1994.A Hopfield network is a form of recurrent artificial neural network popularized by John Hopfield in 1982 and serve as content-addressable ("associative") memory systems with binary threshold nodes.Areas of of AI1. Language understanding: The ability to "know and understand" while responding to the natural language, in English by SIRI in Apple iPhone. AI has a significant research and applications in processing spoken language or written language or mother tounge: Language Translation , Semantics Processing, Vocabulary building of specific individual, Information Retrieval, etc.2. Problem solving: Formulate a problem in a specific situation, develope a solution meeting with a criteria, identify what new information is needed to formulate and the barriers to be identified to obtain it. Inductive and Deductive logic, Resolution-Based Theorem Proving and Heuristic Search.3. Perception: he Pattern recognistion is critical and models have to use and need to develop further to analyze a sensed scene and how accurately it represents the process of living mind.4. Learning and adaptive systems: The ability to adapt behavior based on previous experience, and to develop general rules concerning the world based on such experience.5. Modeling: Identify a representation and a set of rules to predict the behavior and relationship between real objects or entities.6. Robots: A machine with intellengent abilities at some level with HUMAN (currenlty 1 to 2 percent) to move around but in defense over a terrain and capture data on specific objects of exploration, they deal with transportation and navigation. A specific area of Robots growing with AI is Industrial Automation (e.g., Honda uses Robots to paint all two wheeler, used in process control across many sectors, Heavily used in assembly. It is in many defense or aerospace for security and authentification.7. Games: Chess and Checkers are the first products of games to use AI methods. It allows learning abilities of Chess or Bridge. Performance is monitored, errores are corrected. Lot programming has been involved besides tough algorithms.See “One Hundred Year Study on Artificial Intelligence (AI100),” Stanford University, accessed August 1, 2016, One Hundred Year Study on Artificial Intelligence (AI100) |.Deep LearningDEEP LEARNING: A REVIEW (PDF Available) by Rocio Vargas, Ramon Ruiz and Amir Mosavi in Advances in Intelligent Systems and Computing 5(2) · August 2017, Deep learning is an emerging area of machine learning (ML) research. It comprises multiple hidden layers of artificial neural networks. The deep learning methodology applies nonlinear transformations and model abstractions of high level in large databases. The recent advancements in deep learning architec-tures within numerous fields have already provided significant contributions in artificial intelligence. This article presents a state of the art survey on the contributions and the novel applications of deep learning. The following review chronologically presents how and in what major applications deep learning algorithms have been utilized. Furthermore, the superior and beneficial of the deep learning methodology and its hierarchy in layers and nonlinear operations are presented and compared with the more conventional algorithms in the common applications. The state of the art survey further provides a general overview on the novel concept and the ever-increasing advantages and popularity of deep learning.1. Spherical CNNs Researchers at the University of Amsterdam have developed a variation of convolution neural networks (CNN) known as Spherical CNNs. These CNNs work with images which are spherical in shape (3D). For example, images from drones and autonomous cars generally cover many directions and are three-dimensional. Regular CNNs are applicable only to two-dimensional images, and imposing 3D features from images mentioned in this example may literally fail in a DL model. This is where Spherical CNNs were envisioned. In the paper, the researchers conceptualise spherical features with the help of the Fourier Theorem, as well as an algorithm called Fast Fourier Transform. Once developed, they test the CNNs with a 3D model and check for accuracy and effectiveness.The concept of Spherical CNNs is still at a nascent stage. With this study, it will definitely propel the way CNNs are perceived and used. You can read the paper here.2. Can Recurrent Neural Networks Warp Time? Not just ML and AI researchers, even sci-fi enthusiasts can quench their curiosity about time travel, if they possess a strong grasp of concepts like neural networks. In a research paper published by Corentin Tallec, researcher at University of Paris-Sud, and Yann Ollivier, researcher at Facebook AI, they explore the possibility of time warping through recurrent neural networks such as Gated Recurrent Units (GRUs) and Long Short Term Memory (LSTM) networks. The self-learning capabilities present in these models are analysed. The authors have come up with a new concept called ‘Chrono Initialisation’ that derives information from gate biases of LSTM and GRUs. This interesting paper can be read here.3. Learning How To Explain Neural Networks: PatternNet And PatternAttribution We are yet to fully understand why neural networks work exactly in a particular way. Complex ML systems have intricate details which sometimes astonish researchers. Even though there are systems which decode neural networks, it is difficult at times to establish relationships in DL models. In this paper, scholars at Technical University in association with researchers at Google Brain, present two techniques called PatternNet and PatternAttribution which explain linear models. The paper discusses a host of previously established factors such as signal estimators, gradients and saliency maps among others. You can read the paper here.4. Lifelong Learning With Dynamically Expandable Networks: Lifelong learning was a concept first conceived by Sebastian Thrun in his book Learning to Learn. He offered a different perspective of the conventional ML. Instead of ML algorithms learning one single task, he emphasises on machines taking a lifelong approach wherein they learn a variety of tasks over time. Based on this, researchers from KAIST and Ulsan National Institute of Science and Technology developed a novel deep network architecture called Dynamically Expandable Network (DEN) which can dynamically adjust its network capacity for a series of tasks along with requisite knowledge-sharing between them. DEN has been tested on public datasets such as MNIST, CIFAR-100 and AWA for accuracy and efficiency. It was evaluated for factors including selective retraining, network expansion and network timestamping (split/duplication). This novel technique can be read here.5. Wasserstein Auto-Encoders: Autoencoders are neural networks which are used for dimensionality reduction and are popularly used for generative learning models. One particular type of autoencoder which has found most applications in image and text recognition space is variational autoencoder (VAE). Now, scholars from Max Planck Institute for Intelligent Systems, Germany, in collaboration with scientists from Google Brain have come up with the Wasserstein Autoencoder (WAE) which utilises Wasserstein distance in any generative model. In the study, the aim was to reduce optimal transport cost function in the model distribution all along the formulation of this autoencoder. After testing, WAE proved to be more stable than other autoencoders such as VAE with lesser architectural complexity. This is a great improvement in autoencoder architecture. Readers can go through the paper here.Endnote: All of these papers present a unique perspective in the advancements in deep learning. The novel methods also provide a diverse avenue for DL research. Machine learning and artificial intelligence enthusiasts can gain a lot from them when it comes to latest techniques developed in research. By the research of Abhishek Sharma in data science. Source: Top 5 Deep Learning Research Papers You Must Read In 2018Most cited deep learning papers (since 2012) posted by Terry Taewoong Um.Following figure shows deep learning with it’s nature of workinghe repository is broken down into the following categories:Understanding / Generalization / TransferOptimization / Training TechniquesUnsupervised / Generative ModelsConvolutional Network ModelsImage Segmentation / Object DetectionImage / Video / EtcRecurrent Neural Network ModelsNatural Language ProcessSpeech / Other DomainReinforcement Learning / RoboticsMore Papers from 2016For instance, the first category contains the following articles:Distilling the knowledge in a neural network (2015), G. Hinton et al. [pdf]Deep neural networks are easily fooled: High confidence predictions for unrecognizable images (2015), A. Nguyen et al. [pdf]How transferable are features in deep neural networks? (2014), J. Yosinski et al. [pdf]CNN features off-the-Shelf: An astounding baseline for recognition (2014), A. Razavian et al. [pdf]Learning and transferring mid-Level image representations using convolutional neural networks (2014), M. Oquab et al. [pdf]Visualizing and understanding convolutional networks (2014), M. Zeiler and R. Fergus [pdf]Decaf: A deep convolutional activation feature for generic visual recognition (2014), J. Donahue et al. [pdf]Deep learning (Book, 2016), Goodfellow et al. (Bengio) [html]Deep learning (2015), Y. LeCun, Y. Bengio and G. Hinton [html]Deep learning in neural networks: An overview (2015), J. Schmidhuber [pdf]SEE this site: Awesome - Most Cited Deep Learning PapersTop DSC ResourcesArticle: Difference between Machine Learning, Data Science, AI, Deep Learnin...Article: What is Data Science? 24 Fundamental Articles Answering This QuestionArticle: Hitchhiker's Guide to Data Science, Machine Learning, R, PythonTutorial: Data Science Cheat SheetTutorial: How to Become a Data Scientist - On Your OwnTutorial: State-of-the-Art Machine Learning Automation with HDTCategories: Data Science - Machine Learning - AI - IoT - Deep LearningTools: Hadoop - DataViZ - Python - R - SQL - ExcelTechniques: Clustering - Regression - SVM - Neural Nets - Ensembles - Decision TreesLinks: Cheat Sheets - Books - Events - Webinars - Tutorials - Training - News - JobsLinks: Announcements - Salary Surveys - Data Sets - Certification - RSS Feeds - About UsNewsletter: Sign-up - Past Editions - Members-Only Section - Content Search - For BloggersDSC on: Ning - Twitter - LinkedIn - Facebook - GooglePlusFollow us on Twitter: @DataScienceCtrl | @AnalyticBridge
- Home >
- Catalog >
- Legal >
- Affidavit Form >
- Affidavit Of Domicile >
- affidavit of domicile morgan stanley >
- Learning Image Segmentation And Hierarchies By Learning - Mit