The Guide of completing Patient Id Log Online
If you are looking about Alter and create a Patient Id Log, heare are the steps you need to follow:
- Hit the "Get Form" Button on this page.
- Wait in a petient way for the upload of your Patient Id Log.
- You can erase, text, sign or highlight through your choice.
- Click "Download" to download the changes.
A Revolutionary Tool to Edit and Create Patient Id Log
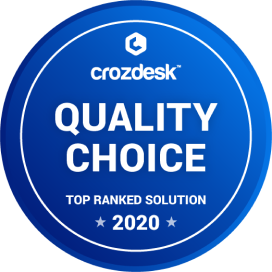
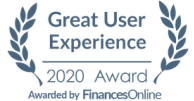
How to Easily Edit Patient Id Log Online
CocoDoc has made it easier for people to Fill their important documents with the online platform. They can easily Modify through their choices. To know the process of editing PDF document or application across the online platform, you need to follow these steps:
- Open CocoDoc's website on their device's browser.
- Hit "Edit PDF Online" button and Append the PDF file from the device without even logging in through an account.
- Edit your PDF online by using this toolbar.
- Once done, they can save the document from the platform.
Once the document is edited using online browser, you can download or share the file as what you want. CocoDoc provides a highly secure network environment for implementing the PDF documents.
How to Edit and Download Patient Id Log on Windows
Windows users are very common throughout the world. They have met millions of applications that have offered them services in modifying PDF documents. However, they have always missed an important feature within these applications. CocoDoc intends to offer Windows users the ultimate experience of editing their documents across their online interface.
The process of editing a PDF document with CocoDoc is simple. You need to follow these steps.
- Pick and Install CocoDoc from your Windows Store.
- Open the software to Select the PDF file from your Windows device and move on editing the document.
- Fill the PDF file with the appropriate toolkit appeared at CocoDoc.
- Over completion, Hit "Download" to conserve the changes.
A Guide of Editing Patient Id Log on Mac
CocoDoc has brought an impressive solution for people who own a Mac. It has allowed them to have their documents edited quickly. Mac users can make a PDF fillable with the help of the online platform provided by CocoDoc.
To understand the process of editing a form with CocoDoc, you should look across the steps presented as follows:
- Install CocoDoc on you Mac in the beginning.
- Once the tool is opened, the user can upload their PDF file from the Mac hasslefree.
- Drag and Drop the file, or choose file by mouse-clicking "Choose File" button and start editing.
- save the file on your device.
Mac users can export their resulting files in various ways. Not only downloading and adding to cloud storage, but also sharing via email are also allowed by using CocoDoc.. They are provided with the opportunity of editting file through multiple ways without downloading any tool within their device.
A Guide of Editing Patient Id Log on G Suite
Google Workplace is a powerful platform that has connected officials of a single workplace in a unique manner. When allowing users to share file across the platform, they are interconnected in covering all major tasks that can be carried out within a physical workplace.
follow the steps to eidt Patient Id Log on G Suite
- move toward Google Workspace Marketplace and Install CocoDoc add-on.
- Attach the file and Hit "Open with" in Google Drive.
- Moving forward to edit the document with the CocoDoc present in the PDF editing window.
- When the file is edited ultimately, share it through the platform.
PDF Editor FAQ
What is the most common thing a nurse might get fired for telling you?
Well this will sound like BS but technically it's true. Patients right to privacy or HIPAA bars people from looking at or passing on a patient's health information outside of those in charge of caring for the patient. It's a very serious issue as well as it should be. Now in the most literal sense a nurse without any reason to look at someone's health care information such as status, diagnosis, test results or other information can get in serious trouble including loss of job even if that nurse is working on the same floor as the patients room. Now does it get that serious, no. Like I say that's the most literal example. In a perfect world you wouldnt even tell your spouse or other family members but as we all know we do. Its important function is to prevent employees snooping on patients info. Example let's say someone you know, friend, neighbor etc comes to the hospital. Your dying to get the lowdown, what's going on with someone you otherwise would never find out. You cant peek and you shouldn't but we get tempted right ? Well the hospital I worked at had all patients info in a secure computer system. You had to log in and then enter patients ID info then if you didn't have a valid reason to look you were flagged and the hospital administrator would come calling. Example, you work in medical imaging. Your Ex comes to the ER. You want to peek so you log in to the system. If you didnt have a reason to look at the information your dying to see you lost your job, no questions asked. It was also multi level too. Patients info such as name, address etc. Then a second level you'd have to log in again to view health and physical info like what the ER physician charted. Log in again to view testing requests and reports. Tedious right ? Yep and you might have to log in several times in one session. You would do it every time you needed to access charts. I know of a couple people who lost their jobs being nosey. Now to be fair the patients I cared for was attached to me so I just had to zap the barcode and get whatever I needed to know. It automatically logged out after so long. Patients info is sacred and it should be. Anyway that's my take on it.Toodles
In predictive modeling, how can you perform accurate feature engineering/extraction? Was there a Kaggle competition where feature engineering/extraction helped you significantly improve your score?
I will take a shot at this from my experience winning KDD CUP’s back in the days (2007–09 and the publications I have on this). But before I go into the tricks of the trade, beware that what one does for competitions is not necessarily the right thing for building a model ‘in the real world’.First of, in principle, if you have a universal function approximator (neural networks, decision trees, also read about VC dimensions if you want to know more) - a model that can express anything, and infinite data, you do not really need to worry about feature engineering. This is basically what we see with the advances of deep learning. Decades of research on feature construction from images have become entirely obsolete….But in reality you usually do not have the luxury of ‘infinite data’. And all of a sudden having a super powerful model class that can express anything is no longer the obvious best choice (see my answer to my prefered algorithm). The reason is the bias variance tradeoff. With great power comes great responsibility … and in the world of predictive modeling overfitting. For instance you may find yourself in a position where a linear model might be the best choice. In fact I won most of my competitions with smart feature construction for linear models.The answer on how to construct features depends VERY MUCH on which type of model you want to use and the strategies for trees are very different from those for linear models. Essentially you are trying to make it easy for a model type to find a relationship that is there. Linear models are great at taking differences, trees are terrible at it. Say you want a model to predict whether a company is profitable - this is simply a question whether revenue is greater than cost. If you have both features, this is really easy for a linear model and really hard for a tree to learn. So you can help the tree by adding differences of pairs of numeric features if you suspect they could matter. Linear models on the other hand have a really hard time with nonlinear relationships (I know I am stating the obvious). Say in health you know that both being too heavy and too light is a problem - it is therefore a good idea to include the square of weight. What about interaction effects (the infamous XOR problem)? Same story, you need to include pairwise products in the model to make it easy for a linear model to find.That also means that there is a good amount of domain knowledge that comes to bear when deciding what might matter and next thinking about whether your model could easily take advantage of this if given the information. In general - having good hypothesis of what might influence the outcome is a great place to start thinking about features.In competitions, something else comes into play - can you find weakness in the data to exploit? Almost all datasets carry traces of their creation and often by exploiting those, you can get better performance. This is know as leakage. In reality you do not want to exploit leakage because it does not really improve your generalization performance in the real world, but in competitions all is game. But before you get all excited, these days Kaggle is trying very hard to remove all such leaked information.So now to a few examples from winning competitions based on feature construction in combination with mostly linear models (logistic, linear svm, etc.) We usually tried all other model classes as well but often ended up with linear (and good feature construction) having the best performance.In a task to predict breast cancer based on 117 features from fMRI data our team was apparently the only one to observe that the patient ID was super predictive. So we added the patient ID range to the model and got a 10% performance increase. Finding accidental information in supposedly random identifiers is a common problem and always suggests that something is wrong in the dataset construction. BTW: we also tried to construct many other features that did not add any value.On a telecom task with 50K anonymous features two members of our team independently tried to do a feature ranking and while we agreed somewhat, there were a number of features that had high mutual information and low AUC as individual contributors. This essentially means that we have a highly non-monotonic relationship. It turned out that in some features somebody had replaced missing values with some method and you could see spikes in the histogram. Those values with spikes were highly predictive but the linear model could obviously not learn from it - so we used decision trees on such single features as a means of discretizing the numeric feature and feed it into the linear models.Common generic tricks to help linear models: discretize numeric variables, cap extreme values of linear and add indicator, include interaction effects, include log/squares, replace missing by zero and include indicator as well as interaction effect.Another broad class of techniques in feature construction deal with the very common case of relational model where a large part of the information is either in a network structure or in 1-n or n-m relationships with the main entity for which you are trying to make a prediction. These cases are notoriously difficult and I spend my entire PhD on trying to create methods for automated feature construction.To conclude, smart feature construction makes much more of a difference than fancy algorithms (that was until deep learning came along, now I am no longer sure …)
When a person dies with their mouth open how long after death will it be difficult to close it?
I am not sure why you would ask this question, but suggest a Licensed Funeral Director may help you better, or do an online search. You have heard the previous answers which are accurate, but have not been told the usual procedure of nursing staff after a patient dies. First, all personal articles and clothing are removed. Then if the eyes are opened, with gentle traction you close them. Next a sling of sorts is placed under the jaw and ties to the top of the head. The arms are stretched and the wrists are crossed and tied together, as are the ankles. The person has a toe tag which is filled out with identification and tied to one of the big toes. The body is placed in a shroud made of a plastic material, and wrapped in it and secured shut. A second, outer identification tag is secured to the outside of the shroud. Some hospitals drape a sheet over the obviously dead body and transport it on a stretcher to the hospital morgue where it is placed inside a refrigerator on a tray or slab. The outside of the door is marked with the patient ID once again, and recorded in a log. Some hospitals have a special hospital stretcher which hides the fact that there is a dead body on it, and this is transported to the morgue. Of course, the family contacted the funeral home, and they may call and ask that the deceased be kept where they are, and they will come immediately and pick up the body. The funeral director wires shut the jaws anyways, so the issue of rigor mortis is one of practicality in moving and lifting/transporting the body.
- Home >
- Catalog >
- Life >
- Log Template >
- Issue Log Template >
- problem log template >
- Patient Id Log