How to Edit and sign Term And Coverage Guarantee Is In Place For 10 Years For Online
Read the following instructions to use CocoDoc to start editing and writing your Term And Coverage Guarantee Is In Place For 10 Years For:
- In the beginning, look for the “Get Form” button and click on it.
- Wait until Term And Coverage Guarantee Is In Place For 10 Years For is shown.
- Customize your document by using the toolbar on the top.
- Download your customized form and share it as you needed.
An Easy Editing Tool for Modifying Term And Coverage Guarantee Is In Place For 10 Years For on Your Way
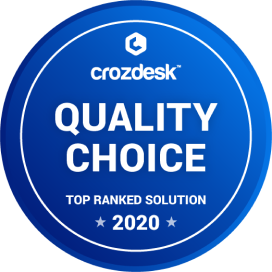
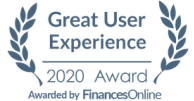
Open Your Term And Coverage Guarantee Is In Place For 10 Years For Instantly
Get FormHow to Edit Your PDF Term And Coverage Guarantee Is In Place For 10 Years For Online
Editing your form online is quite effortless. You don't have to install any software with your computer or phone to use this feature. CocoDoc offers an easy tool to edit your document directly through any web browser you use. The entire interface is well-organized.
Follow the step-by-step guide below to eidt your PDF files online:
- Find CocoDoc official website on your laptop where you have your file.
- Seek the ‘Edit PDF Online’ option and click on it.
- Then you will visit this awesome tool page. Just drag and drop the document, or select the file through the ‘Choose File’ option.
- Once the document is uploaded, you can edit it using the toolbar as you needed.
- When the modification is done, press the ‘Download’ button to save the file.
How to Edit Term And Coverage Guarantee Is In Place For 10 Years For on Windows
Windows is the most widespread operating system. However, Windows does not contain any default application that can directly edit document. In this case, you can install CocoDoc's desktop software for Windows, which can help you to work on documents productively.
All you have to do is follow the guidelines below:
- Get CocoDoc software from your Windows Store.
- Open the software and then choose your PDF document.
- You can also choose the PDF file from OneDrive.
- After that, edit the document as you needed by using the diverse tools on the top.
- Once done, you can now save the customized form to your cloud storage. You can also check more details about how can you edit a PDF.
How to Edit Term And Coverage Guarantee Is In Place For 10 Years For on Mac
macOS comes with a default feature - Preview, to open PDF files. Although Mac users can view PDF files and even mark text on it, it does not support editing. By using CocoDoc, you can edit your document on Mac instantly.
Follow the effortless instructions below to start editing:
- To start with, install CocoDoc desktop app on your Mac computer.
- Then, choose your PDF file through the app.
- You can attach the document from any cloud storage, such as Dropbox, Google Drive, or OneDrive.
- Edit, fill and sign your paper by utilizing this CocoDoc tool.
- Lastly, download the document to save it on your device.
How to Edit PDF Term And Coverage Guarantee Is In Place For 10 Years For via G Suite
G Suite is a widespread Google's suite of intelligent apps, which is designed to make your work faster and increase collaboration across departments. Integrating CocoDoc's PDF editor with G Suite can help to accomplish work effectively.
Here are the guidelines to do it:
- Open Google WorkPlace Marketplace on your laptop.
- Seek for CocoDoc PDF Editor and download the add-on.
- Attach the document that you want to edit and find CocoDoc PDF Editor by choosing "Open with" in Drive.
- Edit and sign your paper using the toolbar.
- Save the customized PDF file on your computer.
PDF Editor FAQ
Is it guaranteed that China's economy will surpass the United States' in terms of nominal GDP?
Let us go back to the year 2008.The US Gdp was 14 trillion.China? 4 trillion.In 2019, the US Gdp increased to 22 trillion, a growth of 8 trillion over a decade.China leapt forward by 10 trillion, increasing to 14 trillion in the same period.Despite the giant move, china's gdp per capita is only a sixth of the US today. There is plenty of room for wage expansion in the coming decades.Why am I confident China will catch up and surpass the US?Because I trust my own eyes.That 10 trillion in gdp drove real change in the way the Chinese people live.China had no high speed rail in 2008. Today, they have more than the rest of the world put together, and they make their own world class trains, tracks and signaling equipment.In 2008, Beijing didn't have Daxing airport, and Shenzhen didn't have 5g. The latter is the only city to achieve blanket 5g coverage worldwide today.In 2008, the Chinese were still buying things with bundles of cash. Today, urban China has transitioned into a cashless society.In 2008, there were barely any ev anywhere. Today, even the buses and trucks are being electrified.I can go on but my eyes tell me gdp growth brings tangible, measurable improvements to the Chinese way of life, yiyo or year in, year out. China is being remade every day, driven by an irrepressible wave of youthful energy.How much change has 8 trillion wrought on American society in the same period? Hyperloop, SpaceX and the rise of social media and big data?Where has all the money gone? America is stuck in a time loop.I see one society marching in step to better their lives together.I see the other marching in place to a cacophony of drumbeat, failing to come to agreement what is the best way forward.How can you fail to turn your head east and witness the dragon taking flight after a centuries long slumber?Forget the chatter. Listen to your eyes.
Why is the p-value criticized? And what are the possible alternatives?
Here’s the short story: the [math]p[/math]-value is a measure that’s used in null-hypothesis significance testing (NHST) that has several weaknesses: it ignores prior knowledge; it encourages the use of cooking-book-style statistical recipes; it answers the wrong question; it wrongly emphasizes straw-man hypotheses; it’s a form of “proof by approximate contradiction” (which is statistically unsound); and more.What can we do instead? One simple alternative that addresses many of the issues with [math]p[/math]-values is to estimate parameters instead of trying to accept/reject discrete hypotheses. More importantly, though, it’s best to keep in mind that data is messy and there is no statistical method that will deliver certainty.Now, the detailed story is long. But if you’re dedicated enough, here it is below. I’ll start by briefly explaining what the [math]p[/math]-value is, how and why it’s used, and some common misconceptions. Then I’ll write about the failings of this approach. And I’ll end with some discussion of alternatives.What is the [math]p[/math]-value?The [math]p[/math]-value is the probability of obtaining a measurement result that is at least as extreme as what was actually measured, under the assumption that the “null” hypothesis [math]H_0[/math] is true.What is the “null” hypothesis, you ask? In Fisher’s original formulation, the null hypothesis was supposed to be the default, accepted idea; the thing you’d believe if the data didn’t change your mind. There’s good reason for this (more below), but this concept is often either ignored or applied very loosely nowadays. Instead, people often (mistakenly) claim that the null hypothesis has to be the assumption that nothing happens, or that there’s no difference between two groups, or that a certain parameter is zero (also known as the “nil” hypothesis). None of these restrictions are either necessary or sufficient for a proper application of [math]p[/math]-values.Null hypothesis significance testing (NHST)The [math]p[/math]-value is typically used in a hypothesis testing framework, in which obtaining a low [math]p[/math]-value — that is, roughly speaking, observing a measurement result that is unlikely given the null — allows us to “reject” the null hypothesis. The logic has a misleading simplicity to it: if I believe something that says X will probably happen, and Y happens instead, then chances are what I believed was wrong. I’ll explain below some ways in which this logic can fail.(xckd: Null Hypothesis)The alternative hypothesisIt’s common to learn about and even use [math]p[/math]-values regularly, all while ignoring an essential concept that is at work behind the scenes: the alternative hypothesis. Confusion abounds about what this is. Some say it has to be the negation of the null hypothesis, but this is unnecessary and even counterproductive. Instead, think of the alternative as simply a second hypothesis, [math]H_1[/math], that we wish to contrast with the null, and that we think might be true instead of the null.So, where does the alternative hypothesis lurk? The answer lies in a seemingly innocuous phrase in the definition of the [math]p[/math]-value:a result that is at least as extreme as what was measuredDid you catch that? What’s an “extreme” result? Who decides if a result is extreme or not?By now, you can probably guess the answer: it’s the alternative hypothesis. What we call “extreme” actually means “more in line with the alternative than with the null”.Let’s look at an example. Suppose we’re testing whether a new COVID-19 treatment works. We would split people into two groups: a control group that doesn’t receive the treatment, and a treatment group that does. We would measure the recovery times for people in the two groups. Now we’re facing our first issue: the data is two-dimensional! What is “extreme” in two dimensions?So here’s the first place where the alternative hypothesis comes in: we’re going to define a so-called “test statistic”, a single number that summarizes the data that we have in a way that allows us to test the null hypothesis. If my alternative hypothesis is that the drug shortens recovery time, then a reasonable idea for a test statistic would be to take the difference between the recovery time in the treatment group and the recovery time in the control group. This is a number, and if we assume that the null hypothesis is “the treatment does nothing”, we would expect this number to be close to zero. What is “more extreme”? Well, the alternative is that the treatment works, which means that the difference (recovery_time_treatment - recovery_time_control) would be negative; thus, “more extreme” means “more negative”.Notice how if this wasn’t a treatment but a risk factor for COVID-19, we would have instead said that “more positive” is more extreme.But there’s more! Imagine that we have a treatment that doesn’t shorten the average recovery time, but it reduces its variability, a bit like the red vs. blue distributions below:If we wanted to test for the alternative “variability in recovery times is smaller in the treatment group”, then we wouldn’t take the different between mean recovery times. Instead, we might take a ratio of variances as a test statistic. The point here is that the alternative hypothesis is instrumental in deciding (a) how to collapse our data to a single number; and (b) what we mean by “extreme” values of this number.That said, it’s worth keeping in mind that the null and the alternative hypotheses enter asymmetrically in this formulation of NHST (due to R. Fisher): the null must be a precise, mathematical statement that can be used to calculate probabilities; while the alternative can be vague and need only help us decide on a summary statistic to use and what to count as “more extreme”. (This is different from the Neyman-Pearson approach to significance testing, where both the null and the alternative are taken seriously. That methodology is used far less often in practice, though, so I will focus on Fisher’s approach for now.)This odd relation between NHST and the alternative hypothesis is dangerous because people often either forget the importance of the alternative — and then get confused about such questions as “should I use a one-tailed or a two-tailed test” — or conversely, believe that rejecting the null is paramount to accepting the alternative. In fact, in some fields scientists often start with an idea that explains the data, call this the alternative hypothesis, then formulate a null hypothesis that contradicts it, and then devise a test. When they find a small [math]p[/math]-value in that test, they erroneously conclude that they have found evidence for their initial hypothesis, when in fact they at most found evidence for the entire class of hypotheses that would have led to the same definition of “more extreme”.So what’s great about null-hypothesis significance testing? Why do people use it?Coverage guaranteesThere is a very nice, mathematical reason for which methods such as NHST are preferred by many statisticians: the coverage guarantees.(xkcd: Coverage)Ok, different kind of coverage.You see, null-hypothesis significance testing is basically an algorithm. It tells you that, after you make a measurement, you need to calculate the [math]p[/math]-value associated with the null hypothesis [math]H_0[/math], and then declare that you reject [math]H_0[/math] if [math]p[/math] is small enough, say, [math]p<\alpha[/math], and otherwise do nothing (or “fail to reject”). The value [math]\alpha[/math] here is called the significance level.Now, the magical thing is that, once you set the significance level, if you keep applying this same algorithm to every decision you’re making, you’re guaranteed to wrongly reject the null just a fraction [math]\alpha[/math] of the time.This is amazing, right? Using a very simple algorithm, and choosing a small-enough [math]\alpha[/math], we can make sure to put a comfortable upper bound on how often we’re wrong!Or… can we? Doesn’t that sound a bit too good to be true? Well, it does, but it is true, in a sense. It’s a mathematical theorem: if the assumptions are obeyed, then the coverage guarantee holds. Now, the devil is in those assumptions, but for now, let’s take a moment to appreciate what we have before we go on and ruin it.SimplicityOne sociological advantage of the [math]p[/math]-value is that they’re easy to use. Tests for many different kinds of null hypotheses have already been devised and implemented in widely available statistical software, and most research problems can be expressed in a form that is at least superficially compatible with one of those tests. That means that the statistical analysis of your data can be a sort of mindless thing: search for a good-enough test, run it, and check whether the [math]p[/math]-value is below or above the significance threshold. Is it ideal to use an off-the-shelf method like that? No. But it sure is convenient.Think of it as eating at McDonalds: it’s not earth-shattering, but it’s fast, cheap, always there, and not entirely unsatisfying, either.But what exactly is wrong with [math]p[/math]-values?Sociological issuesMuch like eating at McDonalds, there’s nothing wrong with using [math]p[/math]-values sparingly and in the proper contexts. So let’s start with the ways in which people misuse [math]p[/math]-values.(xkcd: Clickbait-Corrected p-Value)One such issue is known as p-hacking. You see, the NHST algorithm is clear: reject null if [math]p < \alpha[/math], do not reject otherwise. But people get attached to ideas. So, if the [math]p[/math]-value ends up being just above the significance level [math]\alpha[/math], we might start to wonder: did we make a mistake? should we have used a higher significance level? should we have analyzed the data in a different way? And so on.(xkcd: P-Values)It’s important to understand that there’s nothing fundamentally wrong with this. Science isn’t straightforward. Sometimes we do make a mistake. Sometimes we do use the wrong method. Sometimes there’s just bad luck and our data fail to see an effect that is there. It’s good for the scientist to keep all of these things in mind. But the problem is that if she acts on them, the coverage guarantees that we had are lost. And of course, this can also be used maliciously: a researcher can intentionally remove part of the data, or change the analysis or the significance level used in order to obtain a statistically significant result.A related issue is that of multiple comparisons. From the very definition of the [math]p[/math]-value we see that, if we apply the null-hypothesis significance testing algorithm to, say, 100 different problems, we will reject the null hypothesis approximately [math]100\alpha[/math] times even if all the 100 null hypotheses were true.(xkcd: Significant)So, another way to abuse NHST is to try many similar experiments, and cling on to the one that does yield a statistically significant result.Again, it should be emphasized that there’s nothing wrong with doing this in an exploratory phase: we often have only a vague idea of what we’re looking for before running an experiment, and only after seeing the data can we formulate a more specific hypothesis for what’s going on. The problem occurs when we treat the results of such multiple comparisons as the final result (without appropriate corrections for the multiple comparisons) instead of seeing them as an exploratory step that needs further research to be substantiated. In this case as well, the issue can be either unintentional or fraudulent — with people intentionally reporting only one of hundreds of experiments that happened to “reach statistical significance”.And then there is of course the simplicity issue which we listed as a reason people use the method. The flip side of that is that it gives people a very simple number that is very tempting to latch on to. We run an experiment and we get [math]p=10^{-4}[/math], and all of a sudden we think we’ve “shown” some fact — when reality is much murkier than that.Prior knowledgeSo let’s now delve a bit into the mathematical details of what the [math]p[/math]-value is and isn’t telling us. After all, if the [math]p[/math]-value was indeed as magical as people thought, it wouldn’t be a problem that it’s used so often.One of the most glaring issues with NHST is that it completely ignores prior knowledge. Suppose we were testing the null hypothesis that “nothing moves faster than the speed of light in vacuum, 300,000 km/s”, and after we run the experiment, we find something that’s moving at 300,001 km/s. It was a very sensitive experiment, so we calculate the [math]p[/math]-value and we find [math]p=10^{-6}[/math]. Should we reject the null hypothesis?Well, something very similar to this actually happened some years ago (Faster-than-light neutrino anomaly). And the, perhaps surprising, answer is that we probably should not reject the null — and indeed most physicists dismissed the finding. Say what?!(xkcd: Neutrinos)You see, this isn’t the first measurement that tested [math]H_0[/math]. There are literally thousands of experiments that confirm both this specific hypothesis, and the more general theoretical framework from which it was obtained (the theory of relativity). Shouldn’t all this prior knowledge account for something? Of course it should! It’s surely unlikely to see particles moving so fast if the theory of relativity is true. But it’s even more unlikely that every other experiment was wrong! Extraordinary claims require extraordinary evidence.(xkcd: Frequentists vs. Bayesians)In the neutrino case, it turns out the (erroneous) result was due to a loose cable. The theory of relativity is fine.Coverage broken promisesSo, remember the coverage guarantees that sounded a bit too good to be true? Well… here’s why they aren’t as useful as they might seem.When you reject a hypothesis depending on whether [math]p<\alpha[/math], you’re ensuring that you won’t be wrong more than a fraction [math]\alpha[/math] of the time — of the time when the null hypothesis is true!You read that right. To obtain the coverage guarantee you have to assume that the null hypothesis is true. This should be clear since that’s the assumption that we’re making to define the [math]p[/math]-value.You would be entitled to think: “well, if we know that the null is true, why are we testing it?!”. And if we did know for absolute sure, there would indeed be no point. But we’re never 100% sure of anything, right? The coverage guarantees offered by the [math]p[/math]-value are most useful when we’re testing a well-established idea (e.g., nothing travels faster than the speed of light in vacuum) that nevertheless could conceivably fail to be true. (Of course, then we have to make damn sure we don’t have any loose cables in our experiment!)This is why Fisher insisted that the null should be our default position, the thing that we already believe and would keep believing if the data didn’t convince us otherwise: we would implicitly be making decisions in a setting in which the null was likely true, thus making the [math]p[/math]-value a more meaningful quantity to look at.A different kind of issue with the coverage guarantees is related to the fact that they only hold if the null distribution — that is, the distribution of the test statistic that is implied by the null hypothesis — is compatible with reality. But this is almost never the case. For instance, coming back to our COVID-19 example, it would be standard to assume that the distribution of the difference in recovery times between the treatment and the control groups is a Gaussian. However, since biology is complicated, this is almost certainly not the case. Now, if we run our experiment and find a statistically significant answer, it might mean that the treatment works — or it might mean that we had a large-enough sample to detect that the distribution is not normal.The forgotten alternative hypothesisWhen trying to decide between two hypotheses, the null and the alternative, you can make two kinds of errors. A Type I error is when you reject the null hypothesis even though it’s true. A Type II error is when you fail to reject it even though it’s false. The coverage guarantee for NHST tells you that you can’t have more than [math]\alpha[/math] rate of Type I errors; but it says nothing at all about Type II.Unfortunately, significance testing is often used in cases where Type II errors are common. Part of the reason is the approach I described above: often scientists are taught to set the alternative hypothesis as the hypothesis that they most believe is true, and have the null be a straw man that they proceed to reject using the data. In this case, Type I errors are less likely — after all, we built the null so that it’s unlikely to be true! — and Type II errors should be considered. But the typical methods only report a [math]p[/math]-value and say nothing at all about Type II errors.(xkcd: Error Types)How can you estimate Type II errors? By taking the alternative hypothesis seriously. The rate of Type II errors is essentially the [math]p[/math]-value calculated for the alternative. Of course, to be able to calculate that, we need to have a precise statement, so that we can calculate the distribution of the test statistic under the alternative hypothesis. We can then take the ratio of the [math]p[/math]-values calculated under the two hypotheses to help us decide whether or not to reject the null in favor of the alternative. This more symmetric approach to significance testing was advocated by Neyman and Pearson and generally has better properties than the Fisherian version. However, it is harder to use (since we need to specify a precise alternative), so it’s rarely used in practice.Continuous parameters, effect size, and actual significanceNull-hypothesis significance testing handles a discrete set of hypotheses: we’re trying to reject one hypothesis, perhaps in (weak) favor of another. In doing so, it suffers from a lot of poorly chosen language. For instance, when the data is deemed strong enough to reject a null hypothesis (e.g., [math]p<0.05[/math]), it is said that the result is “statistically significant”. Unfortunately, this is often shortened to “significant”, which takes on a very different meaning.And this leads us to the concept of effect size. You see, typically there isn’t a sharp distinction between “no effect” and “some effect”. Instead, there is a continuum of possibilities, parameterized by an effect size. Think about the speed of light. At one point, people thought light travelled instantaneously. Then we found out that it doesn’t — but not only that, we measured the speed at which it does travel. So this was never really a binary question (“is the speed of light finite”), but rather an estimation question (“how fast does light travel”).A different way to think of this is that there is not one alternative, but infinitely many: one for each value of the speed of light. It should be clear that NHST is not particularly well-suited in such cases — after all, imagine if we measured the speed of light by binary search. Project #1: is it larger than 1m/s? Yes. Project #2: is it larger than 2m/s? Yes. Project #3: etc. Does that seem reasonable?…Now, the nice thing about the size of an effect is that it can have meaning beyond just the statistical question of “is it different from zero”. For instance, if a study looked at the diets of hundreds of thousands of people, it might conclude that drinking a glass of wine every day increases their life expectancy by 1 day, [math]p=10^{-4}[/math]. (Such studies do in fact exist, but I’m making up more extreme numbers to make a point.) This is a statistically significant result — but it’s not actually significant in the usual sense of the word, is it? It’s not like you’d be convinced to drink wine if you didn’t before just to gain that extra one day. Statistical significance in this case does not translate to real-life significance; instead it’s more of a measure of how large the sample size was in the study.(xkcd: Boyfriend)Significance testing answers the wrong questionWhen faced with interpreting the [math]p[/math]-value, people are often tempted to think that, e.g., [math]p=0.01[/math] means that with 99% probability, the null hypothesis is wrong. This is not true, for a few different reasons (see below). However, this fact underlines an important point: most of the times the actual question we’re trying to ask is very different from the question that significance testing answers. We don’t really care about the probability of the data given the hypothesis; but rather we’re interested in the converse, how much to believe the hypothesis given the data. Bayes’ theorem,[math]P(\text{hypothesis} \vert \text{data}) = \frac {\displaystyle P(\text{data}\vert \text{hypothesis}) P(\text{hypothesis})} {\displaystyle P(\text{data})}\,,[/math]allows us to connect the two, and it shows some of the ways in which these quantities can differ.Prior probabilities(xkcd: Seashell)The [math]p[/math]-value is, roughly, the probability of the data given the hypothesis. The term in Bayes’ theorem that multiplies this probability, [math]P(\text{hypothesis})[/math], is called the prior probability of the hypothesis. It formalizes the notion that I mentioned earlier that if we have reasons to believe that something is unlikely, we should need stronger evidence to convince ourselves that it happened (“extraordinary claims require extraordinary evidence”).How do prior probabilities affect our understanding of data? Here’s a standard example. Suppose there’s a rare disease that affects about 1 in 1000 people, and there’s a test that is 99% accurate, in the sense that 99 out of 100 people who are sick are identified as such, while one sick person is missed by the test; and also 99 out of 100 people who are healthy are identified as such, while one is incorrectly diagnosed as sick. Now suppose you take the test and it comes out positive. How likely do you think it is that you are actually sick?It’s very tempting to say that the probability is high. Maybe you wouldn’t say 99%, but 90% certainly comes to mind. Yet, that’s completely off — the actual probability is lower than 10%! How?!Well, imagine that 100,000 randomly chosen people get tested. You would expect about 100 of them to be sick, since the incidence of the disease is 1 in 1000. The test is 99% accurate, so out of the 100 sick ones, the test will flag 99 as sick and 1 will (wrongly) be identified as healthy. But here’s the kicker: out of the remaining 99,900, who are all healthy, the test will mistakenly claim that 999 are sick! That means that we have almost 1100 sick diagnoses out of which only about 100 are correct! That’s less than 10%!By ignoring prior knowledge, significance testing implicitly assumes that the hypotheses that are being contrasted are approximately equally likely — and that’s often not true. That’s especially the case when the null is something like “the effect is zero” and the alternative is “the effect is literally anything else” — how likely do you think it is for an effect to be precisely zero and not some small number, say, [math]10^{-23}[/math]?Proof by approximate contradiction doesn’t workYou might be familiar with proof by contradiction from school. It works like this: you start with an assumption that is the logical negation of the statement you’re trying to prove. You then work out its consequences, and find that you run into a logical contradiction. You thus conclude that the initial assumption must have been wrong, and it follows that the statement you were trying to prove is true. This is a perfectly valid logical argument.(xkcd: Principle of Explosion)Null-hypothesis significance testing often has a very similar flavor. In order to find evidence for a hypothesis, [math]H_1[/math], one generates a “null” hypothesis [math]H_0[/math] that’s the negation of the first. Then one runs an experiment and finds a result that is unlikely given the null. One then concludes that the null is likely false.This is almost identical to proof by contradiction, right, so it must work? Except for one key flaw: the contradiction is not exact — it’s merely unlikely. And that makes all the difference.Paraphrasing an example from an old-ish paper (The earth is round (p<.05)), imagine someone trying to investigate the hypothesis that Barack Obama is not an American citizen. He reasons like this: let’s make the “null” hypothesis that Obama is a citizen. Our intrepid scientist researches Obama and finds that he was president. He calculates the [math]p[/math]-value for this observation and finds [math]p<10^{-7} [/math] — after all, there are only 5 living presidents of the US in a population of over 300 million. So he feels justified to reject the null hypothesis, and declare that Obama is not a citizen.This doesn’t make any sense, does it? The reason is that, while it’s unlikely for a citizen to have been president, it’s even more unlikely for a non-citizen to have been one — in fact, the latter is not allowed. This goes to show one of the ways in which proof by approximate contradiction doesn’t work: unlikely things happen all the time. Only impossible ones don’t.Focus on rejectionAnother harmful side effect of the obsession with [math]p[/math]-values is the pervasive belief that one can only reject a hypothesis, but never prove it.(xkcd: Popper)This is wrong not because you can prove hypotheses — you can’t prove anything with 100% certainty — but because generically it’s just as hard to reject them. This should be obvious from a logical standpoint: rejecting a hypothesis is the same as accepting its negation, so if you can do one, you can clearly do the other. It should also be clear from intuition: rejecting the hypothesis “there are bacteria in this fridge” is no easier than accepting the hypothesis “there no apples in this fridge”:Indeed, one of the ideas hammered into science students’ heads in introductory statistics courses is that failing to reject the null hypothesis doesn’t provide evidence in its favor. But this isn’t always true, and it’s in fact contrary to how the method is applied: whether or not we make this explicit in our papers, we take repeated failures to reject an idea as evidence in favor of it. For instance, we believe the theory of evolution because we’ve tried many times to find evidence against it, and failed. Clearly, failure to reject does imply evidence in the hypothesis’ favor, at least sometimes. The problem, of course, is that many times failure to reject the null hypothesis does not provide strong evidence in its favor — and the [math]p[/math]-value gives us no clue which case is which.AlternativesSo, let’s pretend that I convinced you that significance testing isn’t so great… What can we do instead?I want to first reiterate this: the most important thing to do is to stop thinking that statistics can deliver certainty, and to avoid assigning too much importance to any single measure of “statistical significance”. If we use the [math]p[/math]-value as one of several tools to help us understand our data, and we keep in mind that, no matter how careful we are, only further research can solidify our findings, then we’re on a good path.Next: use parameter estimation instead of significance testing (but also remember the point above: no method will give you perfection!). For virtually every question, the size of the effect is important. We don’t just care whether a gene contributes to breast cancer; we care how much. Moreover, we can use the confidence interval on the parameter to perform the same task as the [math]p[/math]-value — e.g., if the [math]p <0.05[/math], then 0 is outside the 95% confidence interval. Parameter estimation answers both the significance question and a more useful, quantitative, question, it employs precise alternatives rather than the vague ones implied by rejecting the null, and — if done in the form of Bayesian credible intervals — can easily take advantage of prior knowledge.Remember: statistical tests can’t give us certainty. Nothing beats replication. If an effect is true, we should see it in many different ways, in many different experiments, from many different groups. No amount of statistics can compete with that.Tailor statistical methods to the problem at hand instead of using cookbook-style recipes for statistical analysis. People keep using [math]p[/math]-values and forcing every research question into the NHST framework because that’s the hammer they have, and so they want everything to be a nail. Not surprisingly, you can get better results by using better tools.Use Bayesian stats. In most scientific applications, we want statistics to answer the question “how much should I believe in this idea after seeing the data”. The Bayesian approach is a mathematically consistent framework for performing belief updates (in some sense, the only one: Cox's theorem - Wikipedia). There are numerous advantages to this method: it automatically includes base-rate information, it forces you to grapple with the implications of prior knowledge, it makes it relatively straightforward to incorporate arbitrary details of your models. This often comes with a steep computational price, but it’s becoming less of an issue with better algorithms, better software, and better hardware.If you do use binary tests, formulate both a precise null and a precise alternative hypothesis, and use something like Neyman-Pearson’s likelihood ratio. Or use Bayes. The fact that it’s hard to formulate a precise alternative is no excuse — without an alternative it is literally impossible to reject anything.Use more than one of these alternatives. No one method does it all!If you’ve read through and not just scrolled down here, congratulations — I’m impressed!Here’s a short summary: [math]p[/math]-values answer a very specific question (how likely is this data [or something “more extreme”] to have been seen if the null hypothesis is true), and does so very well (i.e., we have coverage guarantees telling us how often we’ll be wrong, in a certain sense). Unfortunately, this is typically not the question we want answered, and it’s easy to get confused; this ignores prior knowledge; it misleadingly downplays the importance of the alternative hypothesis; and it encourages the mindless application of standard statistical techniques when more nuanced approaches would work better. We can instead focus on parameter estimation, which can be done either in a frequentist or Bayesian setting, and addresses all of the downsides of the [math]p[/math]-value without missing much, if anything.
If humans manage not to destroy themselves, what do you think might be achievable technologically in space travel 500 years from now?
I love this question, because it allows the coverage of many key space topics.So ok, let’s imagine this is year 2520.We have sent humans onto pretty much all bodies in the solar system where a human presence is reasonably feasible. After the Moon in 1969, Mars came in 2030. In 2095, we managed to land an astronaut on Ceres, the biggest asteroid of the main Belt. The following three decades we repeated our exploration unto Vesta, Pallas and Hygiea.In the years 2200-2270, we managed to set foot on Jupiter’s main moons: Io, Europa, Ganymede and Callisto. The same century, we managed to send an astronaut down unto Venus’s surface for a couple of hours in a special cabin. Of course, he could not technically set foot on the ground, but it was as good as we could do.Finally, in the years 2300-2360, we managed to conquer Saturn’s main moons in the following order: Titan, Mimas, Enceladus, Tethys, Dione and Rhea.All these missions were driven mainly by prestige and glory. Once a destination was reached, the public quickly lost interest and there was no funding to repeat further manned missions. A few human colonies have been started here and there. Although, as they were all lacking a sustainable business model, they all went bankrupt after a while. Space is now only populated with Sophisticated Robots that are operating semi-autonomously all around our solar system. Those robots conduct mostly scientific missions, plus a few mining and energy projects.The only place we still maintain a permanent human presence is Mars and the Moon. Even there, most big discoveries are long behind us, and from a scientific perspective, we are experiencing diminishing returns. Today, those bases are mainly used as tourism attractions. However, given how hostile the Moon and Mars environments are, they are difficult to develop and it remains a small niche market.On Earth, after a lot of trials and errors, we eventually found a way to manage our limited planet resources in a sustainable way. The consequence was that the world GDP has stopped growing in the middle of the XXIII century. It’s a reasonable price to pay to maintain our standard of living for future generations.Remember, this is year 2520. There is an ambitious terraforming project for Mars that has been going on for more than a century now. After the initial excitement, we got stuck in some hard engineering roadblocks.The project got back on track, but it still remains a long shot. At best, it will take another 200 years to get the atmosphere to the desired level of pressure. And this is assuming funding will not be reduced, which is not a given in our stagnant economy. For plants to grow, and the air to be somewhat breathable, the most optimistic forecast puts this at least a couple of thousand years from now.Given the uncertainties remaining on the project, it’s hard to sell any real estate to investors and the project has to rely mostly on government funding.The last frontier remains interstellar colonization. In 2028, we identified a potentially habitable planet only 8 light years from us that we called New Earth. By the year 2080, our huge space telescopes had conducted full spectral analysis of its atmosphere composition. Its air is not completely breathable, but with a light mask it should work just fine.In the year 2100, we managed to send a small probe, at 2% of light speed, using an innovative fusion reactor. It arrived around New Earth 400 years later and by the year 2508, we started to get detailed coverage of the planet.Key parameters like pressure, temperature, gravity, and the magnetosphere are all confirmed to be ok. This New Earth hosts some basic unicellular life, but the probe found no trace of any multicellular organisms. A perfect place to start a new home!This New Earth seems indeed a much better place for humans than Mars, (or any other spot in our solar system), even if we assume the ongoing Martian terraforming efforts will be successful, (which is still a big if).So now again, it’s the year 2520. How do we proceed to colonize this New Earth?In our stagnant economy, there is only that much we can dedicate to interstellar travel, a project that generates no substantial benefits to politicians on Earth who control the funding. Still, they managed to secure $80 billion financing per year, (2020 equivalent), for this exciting project. Quite an impressive achievement for something which is seen by a large part of the world population as a white elephant without purpose.Faster than light travel is obviously out of the question. Worse, our space transportation technology is stuck at 4% of light speed. Any attempt to go beyond this speed limit generates huge issues and exponential costs beyond the ones reasonably proposed. This means that to reach this New Earth, we must deal with a 200-year trip.In the last 500 years, AI has made tremendous progress. Well, to be honest most progress came in the first 100 years. After 2120, we started to face diminishing returns. Even if our AI are today capable of super impressive feats, we never quite reached the holy grail of Artificial General Intelligence. In particular, we realized that consciousness required a biological human body.Our robots would always remain sophisticated machines although they would have no purpose of their own. In a way, it’s a relief. Who wants its washing machine to be self-aware? For the same reasons, downloading human minds into a computer has never worked. Which is sadly another shortcut we cannot use for interstellar travel. Whether we like it or not, we are stuck in our biological bodies.Medicine has also achieved impressive progress within the last centuries. We now routinely live healthy lives until 110 years old. Which is great! However, beyond that age, the body breaks down. This age limit is hard coded in our DNA and there is not much we can do. Eternal life remains an elusive dream. In a way, it’s also a relief, as immortality could have overstressed social fabric.There has been many attempts to put the human body into cryogenic sleep. In the years 2350, the most ambitious experiment included a dozen volunteers and lasted 10 years. Unfortunately, it did not go too well. Only 3 of them awoke in relatively good shape and after a few days, they were all diagnosed with some form of schizophrenia. Two of them committed suicide in the following year. Given this setback, no one was willing to maintain funding on the project. Unfortunately, for interstellar travel, this area of research has been abandoned.On the other hand, an area where we have made a lot of progress is artificial wombs. We now routinely carry full extracorporeal pregnancy. Even if, thanks to modern medicine, women can get pregnant at up to 60 years old, artificial wombs have become quite popular among the wealthy. It still remains a delicate technology though. To avoid any issues with the immune system of the baby, the process requires serious medical attention during the whole pregnancy.In theory, this gives us the option to send an interstellar ship with a large set of frozen fertilized eggs with the right genetic diversity. This set would be combined with an artificial womb that would be activated a few decades before arriving at the destination. During the years 2180, there had been several experiments of raising babies in closed environments with just robotic nannies.Unfortunately, the results had been catastrophic. All children experienced severe psychological trauma beyond repair. For obvious ethical reasons, those experiments have been shut down since then.For our 200-year trip, it leaves us with the only remaining option, of a generation ship. To stay within the budget allowed for this interstellar mission, it has been calculated that the size of the pressurized habitat of the ship has to be limited to 3600 cubic meters and 2000 tons. This is only 4 times the ISS, but remember it has to be accelerated to 4% of light speed, which requires a crazy amount of energy.The crew would be only females. This reason is that no manufacturer could guarantee that the delicate artificial womb would keep functioning properly during such a long duration. The backup plan was therefore to use the crew as surrogate mothers using in-vitro fertilization.There has been a lot of debate about how many people would join the ship. More people provide more expertise. Although, at the same time, the limited volume of the pressurized habitat is a key constraint. Eventually, it was decided that 3 people would be optimal.A simple calculation showed that with a couple of babies born after 20 years, and then every 50 years afterwards, the whole crew would always remain under 9 people, (assuming that people would be living, on average, up to 100 years). This ensures, at all times, a reasonable amount of living space for each crew member.The whole success of the mission relies on maintaining a strong and healthy culture among the crew members. This is especially critical among the 4 people that will be born respectively 20 and 70 years into the trip, though will never see the final arrival.To maximize the odds, it has been decided to send, at the same time, two twin ships, following the same course. Beyond obvious mission redundancy, this has another advantage. It provides each crew with continuous real time communications with someone within close range of the ship.On top of the psychological comfort, to know that others are facing a similar fate, this could prove useful to deal with any troubleshooting, as opposed to mission support from Earth, which due to very long transmission delays, would quickly prove to be useless in the case of any emergencies.Dealing with the arrival on this New Earth is a whole new challenge on its own. The success of the settlement will depend on how much technology regression the local ecosystem will allow. If we can live on the land with very low tech, the colony might be able to develop organically in a successful fashion.If on the other hand, the local ecosystem is hostile or just not practical, it means that survival requires some fancy technology, (like complex respiratory systems, or special chemicals to grow food…), then the chances of long term survival would shrink dramatically.Given the small population of the colony, it will indeed be impossible to maintain the right level of expertise over time. All mission critical material brought by the mission will slowly but certainly break. As no one will be capable of replacing it, (unless the technology has been cataloged into a library that would easily be accessible to the crew), the whole colony would collapse. Rescue missions sent from Earth would of course not be an option.After such a huge effort, this would be quite sad. So no need to rush. What’s key is to pick the perfect destination for our civilizations New Earth. Hopefully it exists in a 20 light year radius from us. Otherwise interstellar colonization will remain off the table for ever.
- Home >
- Catalog >
- Life >
- Score Sheet >
- Hockey Score Sheet >
- ice hockey scoresheet >
- Term And Coverage Guarantee Is In Place For 10 Years For