The Guide of finishing Concept Review Section Work Power And Machines Online
If you take an interest in Modify and create a Concept Review Section Work Power And Machines, here are the simple ways you need to follow:
- Hit the "Get Form" Button on this page.
- Wait in a petient way for the upload of your Concept Review Section Work Power And Machines.
- You can erase, text, sign or highlight as what you want.
- Click "Download" to preserver the documents.
A Revolutionary Tool to Edit and Create Concept Review Section Work Power And Machines
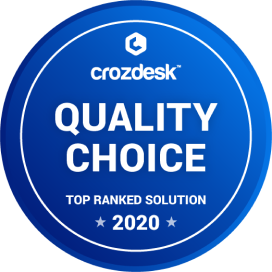
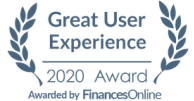
Edit or Convert Your Concept Review Section Work Power And Machines in Minutes
Get FormHow to Easily Edit Concept Review Section Work Power And Machines Online
CocoDoc has made it easier for people to Modify their important documents by online browser. They can easily Fill through their choices. To know the process of editing PDF document or application across the online platform, you need to follow these simple ways:
- Open the website of CocoDoc on their device's browser.
- Hit "Edit PDF Online" button and Upload the PDF file from the device without even logging in through an account.
- Edit the PDF file by using this toolbar.
- Once done, they can save the document from the platform.
Once the document is edited using the online platform, you can download the document easily through your choice. CocoDoc promises friendly environment for implementing the PDF documents.
How to Edit and Download Concept Review Section Work Power And Machines on Windows
Windows users are very common throughout the world. They have met lots of applications that have offered them services in editing PDF documents. However, they have always missed an important feature within these applications. CocoDoc intends to offer Windows users the ultimate experience of editing their documents across their online interface.
The way of editing a PDF document with CocoDoc is easy. You need to follow these steps.
- Select and Install CocoDoc from your Windows Store.
- Open the software to Select the PDF file from your Windows device and continue editing the document.
- Modify the PDF file with the appropriate toolkit presented at CocoDoc.
- Over completion, Hit "Download" to conserve the changes.
A Guide of Editing Concept Review Section Work Power And Machines on Mac
CocoDoc has brought an impressive solution for people who own a Mac. It has allowed them to have their documents edited quickly. Mac users can easily fill form with the help of the online platform provided by CocoDoc.
For understanding the process of editing document with CocoDoc, you should look across the steps presented as follows:
- Install CocoDoc on you Mac to get started.
- Once the tool is opened, the user can upload their PDF file from the Mac easily.
- Drag and Drop the file, or choose file by mouse-clicking "Choose File" button and start editing.
- save the file on your device.
Mac users can export their resulting files in various ways. They can download it across devices, add it to cloud storage and even share it with others via email. They are provided with the opportunity of editting file through various methods without downloading any tool within their device.
A Guide of Editing Concept Review Section Work Power And Machines on G Suite
Google Workplace is a powerful platform that has connected officials of a single workplace in a unique manner. While allowing users to share file across the platform, they are interconnected in covering all major tasks that can be carried out within a physical workplace.
follow the steps to eidt Concept Review Section Work Power And Machines on G Suite
- move toward Google Workspace Marketplace and Install CocoDoc add-on.
- Upload the file and Push "Open with" in Google Drive.
- Moving forward to edit the document with the CocoDoc present in the PDF editing window.
- When the file is edited at last, download it through the platform.
PDF Editor FAQ
I want to learn Artificial Intelligence and Machine learning. Where can I start?
It is a long process and one need to spend few years over 2000 hours in AI and another 2000 hours in Deep Learning to get good at it. It is highly mathematical oriented and parts are driven by biology and those who do not know foundations of Physiology will find great difficulty in relating real world problems in mechanisation.Artificial Intelligence is a broad subject and an old one with research has been done over 60 years based on machines, based on brain it over 100 years old. Operations of a machine mimic some skills of humans have been defined by Artificial Intelligence as first proposed by John McCarthy in 1956. His published a paper called “SOME PHILOSOPHICAL PROBLEMS FROM THE STANDPOINT OF ARTIFICIAL INTELLIGENCE” in 1969, while working in computer science dept., of famous Standford University in Silicon Valley, CA, USA. Source: https://www.csee.umbc.edu/courses/771/spring03/papers/mcchay69.pdfHe said, “ We may regard the subject of AI as beginning with Turing's article Computing Machinery and Intelligence (Turing 1950) and with Shannon's (1950) discussion of howa machine might be programmed to playchess.”Basic view of intelligence is epistemological and the heuristic way according to Macarthy. The epistemological part is the representation of the world in such a form that the solution of problems follows from the facts expressed in the representation. The heuristic part is the mechanism that on the basis of the information solves the problem and decides what to do. Most of the work in articial intelligence so far can be regarded as devoted to the heuristic part of the problem.Source: Minsky, M. (1961), “Steps towards Artificial Intelligence”, Proceedings of the I.R.E., 49, 8-30.Alan Turing created a machine that just can plug in a electrical socket run forever off course doing some really came later but Turning test are critcial in AI. Source: Turing, A.M. (1950), “Computing machinery and intelligence”, Mind, 59, 433-60.First question is how to create an algorithm for an intelligent process, the basis of AI.Next how to formulate a problem or a process. Propose a solution and develop a prototype and test itHow does an eye work lot more complex than an camera. How do photos process in a smart phone is also complex?Storing in a creative way and process thru a microprocessor is the focus now. But select a specific photo is not still there but get photos by date or by group is only possibility now. In Brian, think an incident and close eyes you pictures, HOW? Hypothalamus and Neocortex work synchronizely in CNS to produce is what baffling many scientists. There “ thousands of processes we do how” is the study of AI.There are 7 million rods and more than a million cones in each besides Ganglion Cells, Bipolar cells etc to decide our view into retina eventually store in cortex.The process of getting information, process and transmit is done in any nerve called “Synapse” with Dendrite (with small wires) , Nucleus and Axon (a long part)Lynn Conway, a famous mainframe designer at IBM, also worked at XEROX at Palo Alto and Carver Mead a professor at Cal Tech, a top five research universities i. USA, created AI based eye in latest 1980s but it can only less than 20 percent of our eye. There are the “First” authors VLSI, especially Conway preached MIT, Berkeley, UCLA, etc to teach VLSI. Then came expert systems, neural networks, fuzzy logic etc.Introduction to VLSI Systems by Carver Mead & Lynn Conway, Addison-Wesley Longman Publishing Co., Inc. Boston, MA, USA ©1979ISBN:0201043580This book was cited over 4400 in Research.How you make a machine to bring coffee from a flask. Open the top cup by rotating clockwise, get a mug, bend flask, pour Coffee, close lid, navigates where you are? All thus done now so called Robotics but not cheap.How do we understand this simply process but a complex system is THE foundation of AI.There are variety coffee machines made in USA, while I was there for different dose if coffee or sugar or temperatures of for Indians mix milk. How can AI can be used, a simple way is Fuzzy logic (FL). At a machine, a person takes a coffee he can say coffee need darker or more temp or less sugar. The machine with processor and memory can create new set of values for three variables of coffee, sugar, temperature, a fuzzy way of doing AI. In 1964, Lotfi Zadeh developed FL.Honey Bees collect honey through flowers using earth magnetic field. But how do they find, get back, communicate and establish a nest just like a group of human develop a family. Few algorithms were developed on this and Artificial Neural Networkes were created.Read about Marvin Lee Minsky (August 9, 1927 – January 24, 2016) was an American cognitive scientist concerned largely with research of artificial intelligence (AI)A great book by Gerald M. Edelman, “A Remembered Present” who won a Noble Prize in Medicine.Ex: “Creating and simulating neural networks in the honeybee brain using a graphical toolchai”, http://greenbrain.group.shef.ac.uk/wp-content/uploads/2013/11/SFN_2013_GB.pdfDeep Blue, a super computer made by IBM, almost beat any Grand Master, Vishwanth Anand played many times, list only few times, a great example of a billion dollar AI investment on research.“Artificial Intelligence and Human Thinking “ by Robert Kowalski, Imperial College London United Kingdom [email protected]. According to Kowalski, “Research in AI has built upon the tools and techniques of many different disciplines, including formal logic, probability theory, decision theory, management science, linguistics and philosophy. However, the application of these disciplines in AI has necessitated the development of many enhancements and extensions. Among the most powerful of these are the methods of computational logic. I will argue that computational logic, embedded in an agent cycle, combines and improves upon both traditional logic and classical decision theory. I will also argue that many of its methods can be used, not only in AI, but also in ordinary life, to help people improve their own human intelligence without the assistance of computers.” According him that Abductive Logic Programming form of compututational Logic embeds agent cycle shows in the following figure.A basic paper in AI can be easily accessed throughj Reasearch Gate: Artificial Intelligence by Mariam Khaled Alsedrah, The American University of the Middle East, December 2017. She told that AI works based on several models such as: Ant Colony Algorithm, Immune Algorithm, Fuzzy Algorithm, Decision Tree, Genetic Algorithm, Particle Swarm Algorithm, Neural Network, and Deep Learning.Neural networks deal with higher millions neurons that process information in CNS if Brain so called nerves. They are an essential part of AI nowadays. From a synaptic weight to summation, to back propagation to networking is the complex maze of ANN. I worked on two ANN projects at Motorola in CMOS VLSI. First chip has 1,15,200 logic gates and 2nd chip had over 1 million logic gates and fabricated 1994.A Hopfield network is a form of recurrent artificial neural network popularized by John Hopfield in 1982 and serve as content-addressable ("associative") memory systems with binary threshold nodes.Areas of of AI1. Language understanding: The ability to "know and understand" while responding to the natural language, in English by SIRI in Apple iPhone. AI has a significant research and applications in processing spoken language or written language or mother tounge: Language Translation , Semantics Processing, Vocabulary building of specific individual, Information Retrieval, etc.2. Problem solving: Formulate a problem in a specific situation, develope a solution meeting with a criteria, identify what new information is needed to formulate and the barriers to be identified to obtain it. Inductive and Deductive logic, Resolution-Based Theorem Proving and Heuristic Search.3. Perception: he Pattern recognistion is critical and models have to use and need to develop further to analyze a sensed scene and how accurately it represents the process of living mind.4. Learning and adaptive systems: The ability to adapt behavior based on previous experience, and to develop general rules concerning the world based on such experience.5. Modeling: Identify a representation and a set of rules to predict the behavior and relationship between real objects or entities.6. Robots: A machine with intellengent abilities at some level with HUMAN (currenlty 1 to 2 percent) to move around but in defense over a terrain and capture data on specific objects of exploration, they deal with transportation and navigation. A specific area of Robots growing with AI is Industrial Automation (e.g., Honda uses Robots to paint all two wheeler, used in process control across many sectors, Heavily used in assembly. It is in many defense or aerospace for security and authentification.7. Games: Chess and Checkers are the first products of games to use AI methods. It allows learning abilities of Chess or Bridge. Performance is monitored, errores are corrected. Lot programming has been involved besides tough algorithms.See “One Hundred Year Study on Artificial Intelligence (AI100),” Stanford University, accessed August 1, 2016, One Hundred Year Study on Artificial Intelligence (AI100) |.Deep LearningDEEP LEARNING: A REVIEW (PDF Available) by Rocio Vargas, Ramon Ruiz and Amir Mosavi in Advances in Intelligent Systems and Computing 5(2) · August 2017, Deep learning is an emerging area of machine learning (ML) research. It comprises multiple hidden layers of artificial neural networks. The deep learning methodology applies nonlinear transformations and model abstractions of high level in large databases. The recent advancements in deep learning architec-tures within numerous fields have already provided significant contributions in artificial intelligence. This article presents a state of the art survey on the contributions and the novel applications of deep learning. The following review chronologically presents how and in what major applications deep learning algorithms have been utilized. Furthermore, the superior and beneficial of the deep learning methodology and its hierarchy in layers and nonlinear operations are presented and compared with the more conventional algorithms in the common applications. The state of the art survey further provides a general overview on the novel concept and the ever-increasing advantages and popularity of deep learning.1. Spherical CNNs Researchers at the University of Amsterdam have developed a variation of convolution neural networks (CNN) known as Spherical CNNs. These CNNs work with images which are spherical in shape (3D). For example, images from drones and autonomous cars generally cover many directions and are three-dimensional. Regular CNNs are applicable only to two-dimensional images, and imposing 3D features from images mentioned in this example may literally fail in a DL model. This is where Spherical CNNs were envisioned. In the paper, the researchers conceptualise spherical features with the help of the Fourier Theorem, as well as an algorithm called Fast Fourier Transform. Once developed, they test the CNNs with a 3D model and check for accuracy and effectiveness.The concept of Spherical CNNs is still at a nascent stage. With this study, it will definitely propel the way CNNs are perceived and used. You can read the paper here.2. Can Recurrent Neural Networks Warp Time? Not just ML and AI researchers, even sci-fi enthusiasts can quench their curiosity about time travel, if they possess a strong grasp of concepts like neural networks. In a research paper published by Corentin Tallec, researcher at University of Paris-Sud, and Yann Ollivier, researcher at Facebook AI, they explore the possibility of time warping through recurrent neural networks such as Gated Recurrent Units (GRUs) and Long Short Term Memory (LSTM) networks. The self-learning capabilities present in these models are analysed. The authors have come up with a new concept called ‘Chrono Initialisation’ that derives information from gate biases of LSTM and GRUs. This interesting paper can be read here.3. Learning How To Explain Neural Networks: PatternNet And PatternAttribution We are yet to fully understand why neural networks work exactly in a particular way. Complex ML systems have intricate details which sometimes astonish researchers. Even though there are systems which decode neural networks, it is difficult at times to establish relationships in DL models. In this paper, scholars at Technical University in association with researchers at Google Brain, present two techniques called PatternNet and PatternAttribution which explain linear models. The paper discusses a host of previously established factors such as signal estimators, gradients and saliency maps among others. You can read the paper here.4. Lifelong Learning With Dynamically Expandable Networks: Lifelong learning was a concept first conceived by Sebastian Thrun in his book Learning to Learn. He offered a different perspective of the conventional ML. Instead of ML algorithms learning one single task, he emphasises on machines taking a lifelong approach wherein they learn a variety of tasks over time. Based on this, researchers from KAIST and Ulsan National Institute of Science and Technology developed a novel deep network architecture called Dynamically Expandable Network (DEN) which can dynamically adjust its network capacity for a series of tasks along with requisite knowledge-sharing between them. DEN has been tested on public datasets such as MNIST, CIFAR-100 and AWA for accuracy and efficiency. It was evaluated for factors including selective retraining, network expansion and network timestamping (split/duplication). This novel technique can be read here.5. Wasserstein Auto-Encoders: Autoencoders are neural networks which are used for dimensionality reduction and are popularly used for generative learning models. One particular type of autoencoder which has found most applications in image and text recognition space is variational autoencoder (VAE). Now, scholars from Max Planck Institute for Intelligent Systems, Germany, in collaboration with scientists from Google Brain have come up with the Wasserstein Autoencoder (WAE) which utilises Wasserstein distance in any generative model. In the study, the aim was to reduce optimal transport cost function in the model distribution all along the formulation of this autoencoder. After testing, WAE proved to be more stable than other autoencoders such as VAE with lesser architectural complexity. This is a great improvement in autoencoder architecture. Readers can go through the paper here.Endnote: All of these papers present a unique perspective in the advancements in deep learning. The novel methods also provide a diverse avenue for DL research. Machine learning and artificial intelligence enthusiasts can gain a lot from them when it comes to latest techniques developed in research. By the research of Abhishek Sharma in data science. Source: Top 5 Deep Learning Research Papers You Must Read In 2018Most cited deep learning papers (since 2012) posted by Terry Taewoong Um.Following figure shows deep learning with it’s nature of workinghe repository is broken down into the following categories:Understanding / Generalization / TransferOptimization / Training TechniquesUnsupervised / Generative ModelsConvolutional Network ModelsImage Segmentation / Object DetectionImage / Video / EtcRecurrent Neural Network ModelsNatural Language ProcessSpeech / Other DomainReinforcement Learning / RoboticsMore Papers from 2016For instance, the first category contains the following articles:Distilling the knowledge in a neural network (2015), G. Hinton et al. [pdf]Deep neural networks are easily fooled: High confidence predictions for unrecognizable images (2015), A. Nguyen et al. [pdf]How transferable are features in deep neural networks? (2014), J. Yosinski et al. [pdf]CNN features off-the-Shelf: An astounding baseline for recognition (2014), A. Razavian et al. [pdf]Learning and transferring mid-Level image representations using convolutional neural networks (2014), M. Oquab et al. [pdf]Visualizing and understanding convolutional networks (2014), M. Zeiler and R. Fergus [pdf]Decaf: A deep convolutional activation feature for generic visual recognition (2014), J. Donahue et al. [pdf]Deep learning (Book, 2016), Goodfellow et al. (Bengio) [html]Deep learning (2015), Y. LeCun, Y. Bengio and G. Hinton [html]Deep learning in neural networks: An overview (2015), J. Schmidhuber [pdf]SEE this site: Awesome - Most Cited Deep Learning PapersTop DSC ResourcesArticle: Difference between Machine Learning, Data Science, AI, Deep Learnin...Article: What is Data Science? 24 Fundamental Articles Answering This QuestionArticle: Hitchhiker's Guide to Data Science, Machine Learning, R, PythonTutorial: Data Science Cheat SheetTutorial: How to Become a Data Scientist - On Your OwnTutorial: State-of-the-Art Machine Learning Automation with HDTCategories: Data Science - Machine Learning - AI - IoT - Deep LearningTools: Hadoop - DataViZ - Python - R - SQL - ExcelTechniques: Clustering - Regression - SVM - Neural Nets - Ensembles - Decision TreesLinks: Cheat Sheets - Books - Events - Webinars - Tutorials - Training - News - JobsLinks: Announcements - Salary Surveys - Data Sets - Certification - RSS Feeds - About UsNewsletter: Sign-up - Past Editions - Members-Only Section - Content Search - For BloggersDSC on: Ning - Twitter - LinkedIn - Facebook - GooglePlusFollow us on Twitter: @DataScienceCtrl | @AnalyticBridge
How do I prepare for a data scientist interview?
I recently wrote a blog post that aims precisely to answer this question. Cross-posting here from How to Ace a Data Science Interview.As I mentioned in my first post, I have just finished an extensive tech job search, which featured eight on-sites, along with countless phone screens and informal chats. I was interviewing for a combination of data science and software engineering (machine learning) positions, and I got a pretty good sense of what those interviews are like. In this post, I give an overview of what you should expect in a data science interview, and some suggestions for how to prepare.An interview is not a pop quiz. You should know what to expect going in, and you can take the time to prepare for it. During the interview phase of the process, your recruiter is on your side and can usually tell you what types of interviews you’ll have. Even if the recruiter is reluctant to share that, common practices in the industry are a good guide to what you’re likely to see.In this post, I’ll go over the types of data science interviews I’ve encountered, and offer my advice on how to prepare for them. Data science roles generally fall into two broad ares of focus: statistics and machine learning. I only applied to the latter category, so that’s the type of position discussed in this post. My experience is also limited to tech companies, so I can’t offer guidance for data science in finance, biotech, etc..Here are the types of interviews (or parts of interviews) I’ve come across.Always:Coding (usually whiteboard)Applied machine learningYour backgroundOften:Culture fitMachine learning theoryDataset analysisStatsYou will encounter a similar set of interviews for a machine learning software engineering position, though more of the questions will fall in the coding category.Coding (usually whiteboard)This is the same type of interview you’d have for any software engineering position, though the expectations may be less stringent. There are lots of websites and books that will tell you how to prepare. Practice your coding skills if they’re rusty. Don’t forget to practice coding away from the computer (e.g. on paper), which is surely a skill that’s rusty. Review the data structures you may never have used outside of school — binary search trees, linked lists, heaps. Be comfortable with recursion. Know how to reason about algorithm running times. You can generally use any “real” language you want in an interview (Matlab doesn’t count, unfortunately); Python’s succinct syntax makes it a great language for coding interviews.Prep tips:If you get nervous in interviews, try doing some practice problems under time pressure.If you don’t have much software engineering experience, see if you can get a friend to look over your practice code and provide feedback.During the interview:Make sure you understand exactly what problem you’re trying to solve. Ask the interviewer questions if anything is unclear or underspecified.Make sure you explain your plan to the interviewer before you start writing any code, so that they can help you avoid spending time going down less-than-ideal paths.If you can’t think of a good way to do something, it often helps to start by talking through a dumb way to do it.Mention what invalid inputs you’d want to check for (e.g. input variable type check). Don’t bother writing the code to do so unless the interviewer asks. In all my interviews, nobody has ever asked.Before declaring that your code is finished, think about variable initialization, end conditions, and boundary cases (e.g. empty inputs). If it seems helpful, run through an example. You’ll score points by catching your bugs yourself, rather than having the interviewer point them out.Applied machine learningAll the applied machine learning interviews I’ve had focused on supervised learning. The interviewer will present you with a prediction problem, and ask you to explain how you would set up an algorithm to make that prediction. The problem selected is often relevant to the company you’re interviewing at (e.g. figuring out which product to recommend to a user, which users are going to stop using the site, which ad to display, etc.), but can also be a toy example (e.g. recommending board games to a friend). This type of interview doesn’t depend on much background knowledge, other than having a general understanding of machine learning concepts (see below). However, it definitely helps to prepare by brainstorming the types of problems a particular company might ask you to solve. Even if you miss the mark, the brainstorming session will help with the culture fit interview (also see below).When answering this type of question, I’ve found it helpful to start by laying out the setup of the problem. What are the inputs? What are the labels you’re trying to predict? What machine learning algorithms could you run on the data? Sometimes the setup will be obvious from the question, but sometimes you’ll need to figure out how to define the problem. In the latter case, you’ll generally have a discussion with the interviewer about some plausible definitions (e.g., what does it mean for a user to “stop using the site”?).The main component of your answer will be feature engineering. There is nothing magical about brainstorming features. Think about what might be predictive of the variable you are trying to predict, and what information you would actually have available. I’ve found it helpful to give context around what I’m trying to capture, and to what extent the features I’m proposing reflect that information.For the sake of concreteness, here’s an example. Suppose Amazon is trying to figure out what books to recommend to you. (Note: I did not interview at Amazon, and have no idea what they actually ask in their interviews.) To predict what books you’re likely to buy, Amazon can look for books that are similar to your past Amazon purchases. But maybe some purchases were mistakes, and you vowed to never buy a book like that again. Well, Amazon knows how you’ve interacted with your Kindle books. If there’s a book you started but never finished, it might be a positive signal for general areas you’re interested in, but a negative signal for the particular author. Or maybe some categories of books deserve different treatment. For example, if a year ago you were buying books targeted at one-year-olds, Amazon could deduce that nowadays you’re looking for books for two-year-olds. It’s easy to see how you can spend a while exploring the space between what you’d like to know and what you can actually find out.Your backgroundYou should be prepared to give a high-level summary of your career, as well as to do a deep-dive into a project you’ve worked on. The project doesn’t have to be directly related to the position you’re interviewing for (though it can’t hurt), but it needs to be the kind of work you can have an in-depth technical discussion about.To prepare:Review any papers/presentations that came out of your projects to refresh your mind on the technical details.Practice explaining your project to a friend in order to make sure you are telling a coherent story. Keep in mind that you’ll probably be talking to someone who’s smart but doesn’t have expertise in your particular field.Be prepared to answer questions as to why you chose the approach that you did, and about your individual contribution to the project.Culture fitHere are some culture fit questions your interviewers are likely to be interested in. These questions might come up as part of other interviews, and will likely be asked indirectly. It helps to keep what the interviewer is looking for in the back of your mind.Are you specifically interested in the product/company/space you’d be working in?It helps to prepare by thinking about the problems the company is trying to solve, and how you and the team you’d be part of could make a difference.Do you care about impact? Even in a research-oriented corporate environment, I wouldn’t recommend saying that you don’t care about company metrics, and that you’d love to just play with data and write papers.Will you work well with other people? I know it’s a cliché, but most work is collaborative, and companies are trying to assess this as best they can. Avoid bad-mouthing former colleagues, and show appreciation for their contributions to your projects.Are you willing to get your hands dirty? If there’s annoying work that needs to be done (e.g. cleaning up messy data), will you take care of it?Are you someone the team will be happy to have around on a personal level? Even though you might be stressed, try to be friendly, positive, enthusiastic and genuine throughout the interview process.You may also get broad questions about what kinds of work you enjoy and what motivates you. It’s useful to have an answer ready, but there may not be a “right” answer the interviewer is looking for.Machine learning theoryThis type of interview will test your understanding of basic machine learning concepts, generally with a focus on supervised learning. You should understand:The general setup for a supervised learning systemWhy you want to split data into training and test setsThe idea that models that aren’t powerful enough can’t capture the right generalizations about the data, and ways to address this (e.g. different model or projection into a higher-dimensional space)The idea that models that are too powerful suffer from overfitting, and ways to address this (e.g. regularization)You don’t need to know a lot of machine learning algorithms, but you definitely need to understand logistic regression, which seems to be what most companies are using. I also had some in-depth discussions of SVMs, but that may just be because I brought them up.Dataset analysisIn this type of interview, you will be given a data set, and asked to write a script to pull out features for some prediction task. You may be asked to then plug the features into a machine learning algorithm. This interview essentially adds an implementation component to the applied machine learning interview (see above). Of course, your features may now be inspired by what you see in the data. Do the distributions for each feature you’re considering differ between the labels you’re trying to predict?I found these interviews hardest to prepare for, because the recruiter often wouldn’t tell me what format the data would be in, and what exactly I’d need to do with it. (For example, do I need to review Python’s csv import module? Should I look over the syntax for training a model in scikit-learn?) I also had one recruiter tell me I’d be analyzing “big data”, which was a bit intimidating (am I going to be working with distributed databases or something?) until I discovered at the interview that the “big data” set had all of 11,000 examples. I encourage you to push for as much info as possible about what you’ll actually be doing.If you plan to use Python, working through the scikit-learn tutorial is a good way to prepare.StatsI have a decent intuitive understanding of statistics, but very little formal knowledge. Most of the time, this sufficed, though I’m sure knowing more wouldn’t have hurt. You should understand how to set up an A/B test, including random sampling, confounding variables, summary statistics (e.g. mean), and measuring statistical significance.Preparation Checklist & ResourcesHere is a summary list of tips for preparing for data science interviews, along with a few helpful resources.Coding (usually whiteboard)Get comfortable with basic algorithms, data structures and figuring out algorithm complexity.Practice writing code away from the computer in your programming language of choice.Resources:Pretty exhaustive list of what you might encounter in an interviewMany interview prep books, e.g. Cracking the Coding InterviewApplied machine learningThink about the machine learning problems that are relevant for each company you’re interviewing at. Use these problems as practice questions.Your backgroundThink through how to summarize your experience.Prepare to give an in-depth technical explanation of a project you’ve worked on. Try it out on a friend.Culture fitThink about the problems each company is trying to solve, and how you and the team you’d be part of could make a difference.Be prepared to answer broad questions about what kind of work you enjoy and what motivates you.Machine learning theoryUnderstand machine learning concepts on an intuitive level, focusing especially on supervised learning.Learn the math behind logistic regression.Resources:The Shape of Data blog provides a nice intuitive overview.A Few Useful Things to Know about Machine LearningTo really go in depth, check out Andrew Ng’s Stanford machine learning course on Coursera or OpenClassroom.Dataset analysisGet comfortable with a set of technical tools for working with data.Resources:If you plan to use Python, work through the scikit-learn tutorial (you could skip section 2.4).StatsGet familiar with how to set up an A/B test.Resources:Quora answer about how to prepare for interview questions about A/B testingHow not to run an A/B testSample size calculator, which you can use to get some intuition about sample sizes required based on the sensitivity (i.e. minimal detectable effect) and statistical significance you’re looking for
Which book is the best book for deep learning, AI and IOT?
Here is a shortlist that reflects my collective recommendations, but I’ve highlighted who I think should find the particular book most interesting so that you can zero in on the one that’s best for you.Introduction to Artificial Intelligence by Philip C JacksonOriginally written over 40 years ago, and released as a second edition in 1985, this classic provides an introduction to the science of reasoning processes in computers, as well as the approaches and results of more than two decades of research. Subjects such as, proving predicate-calculus theorem, machine architecture, psychological simulation, automatic programming, novel software techniques, industrial automation, have been enhanced by diagrams and clear illustrations.Who would find this book most interesting:Anyone who is entering the Artificial Intelligence space and would like to have a much deeper understanding of the field. Especially if you would like explore new topics and develop a broad understanding of different areas, so that you will know what to learn next.Deep Learning (Adaptive Computation and Machine Learning series) by Ian Goodfellow, Yoshua Bengio, & Aaron CourvilleAfter two and a half years in the making, Deep Learning (Adaptive Computation and Machine Learning series) was released in late 2016 and has quickly become a groundbreaking resource on the subject of deep learning. Written by three of the top academics in the subject of deep learning, this book has been created for both graduate-level university students studying computer science, and software engineers alike. The authors have tackled the subject head-on, while also providing a necessary framework for understanding such highly technical subjects as convolution, generative models, and hidden layers.Who would find this book most interesting:Experienced engineers who want to get serious about Deep Learning. This is a great resource before you start coding with any framework so that it is easier to really understand and get going faster.The Elements of Statistical Learning: Data Mining, Inference, and Prediction, Second Edition (Springer Series in Statistics) by Trevor Hastie, Robert Tibshirani & Jerome FriedmanThis book is an excellent resource for anyone looking for a better understanding of the concepts of data mining, machine learning, and bioinformatics through a statistical approach. It is quite comprehensive, with many topics including neural networks, support vector machines, classification trees and boosting. Concepts are well defined and clearly presented with vivid color illustrations throughout the book.Who would find this book most interesting:Our opinion is that this is advanced stuff. Of course academics and statisticians will dig it, as well as anyone technical that needs to beef up their knowledge of the topic. When you read the published reviews, it says that it is relevant for “anyone interested in the field…as an entry point to the area…” so what do we know?Python Machine Learning by Sebastian RaschkaThis very practical guide offers deep insights into machine learning, as well as a hands-on approach to the latest developments in predictive analytics. Python Machine Learning covers a wide range of powerful Python libraries, including scikit-learn, Theano, and Pylearn2, and features guidance and tips on everything from sentiment analysis to neural networks. Sebastian Raschka has provided a crucial resource that clearly demonstrates what makes Python one of the leading data science languages in the world.Who would find this book most interesting:Written for anyone looking to ask better questions of their data, or for those who need to improve and extend the capabilities of their machine learning systems. If you are a beginner in machine learning, this book is also for you, but every reader should at least have a solid foundation in Python.How to Create a Mind: The Secret of Human Thought Revealed by Ray KurzweilWritten by acclaimed futurist Ray Kurzweil, this book takes a deep dive into how future civilizations will be dominated by the interconnectedness of humans and machines. He describes the rise and development of intelligent machines through the process of reverse engineering the human brain. Ray describes this process through clear explanations of themes such as logical agents, the quantification of uncertainty, learning from example, the communication, perception, and action of natural language processing, and more. The book concludes with a discussion of the philosophical foundations of A.I., as well as an examination of what lies ahead in the years to come.Who would find this book most interesting:Ideal for those with an interest in the future of advanced machine learning, with a focus on the correlation between intelligent machines and humanity. If you are trying to calibrate yourself, don’t worry, this one is for everyone, the mere fact that you are reading this should tell you that this book is accessible, think of it as a philosophy treatise as opposed to a technical manual.Artificial Intelligence and Soft Computing: Behavioral and Cognitive Modeling of the Human Brain by Amit KonarWidely considered to be the bible of theoretical A.I.within the field of computer science, this book provides a comprehensive resource that is both conceptually advanced and accessible enough to enable the reader to both understand and apply modern and traditional A.I.concepts. The content is diverse, but complete, covering subjects ranging from the behavioral perspective of human cognition to non-monotonic and spatio-temporal reasoning. The text is clearly written, practical, and thorough.Who would find this book most interesting:This book should have broad appeal: it provides an excellent resource for anyone involved in computer science— from students to seasoned professionals.Reinforcement Learning: An Introduction by Richard S. Sutton & Andrew G. BartoReinforcement learning has quickly become one of the hottest topics in Artificial Intelligence research today. This book provides a comprehensive introduction to many of the key insights and algorithms associated with reinforcement learning. The text is intelligibly written into 3 sections with the first section dedicated to a deeper understanding of the Markov decision processes. The second section covers basic solution methods such as dynamic programming, Monte Carlo methods, and temporal-difference learning. Lastly, the third section provides a unified view of the solution methodology covering topics that range from artificial neural networks to eligibility traces, and planning. As a whole, Richard S. Sutton and Andrew G. Barto do an excellent job of covering both the conceptual foundations of reinforcement learning, as well as its latest developments and applications.Who would find this book most interesting:It’s an introductory book to a new field of Artificial Intelligence. Engineers who are looking to stay on top of the latest trends in artificial intelligence, including a thorough understanding of reinforcement learning, should find this book helpful.The Second Machine Age: Work, Progress and Prosperity in a Time of Brilliant TechnologiesGetting started with Internet of ThingsThe Silent IntelligenceIoT Disruptions: The Internet of Things – Innovation & JobsEveryware: The dawning age of ubiquitous computing
- Home >
- Catalog >
- Business >
- Report Template >
- Report Writing Format >
- Format Of Report Writing >
- report writing sample pdf >
- Concept Review Section Work Power And Machines