A Quick Guide to Editing The Practice 2 3 Simplifying Variable Expression
Below you can get an idea about how to edit and complete a Practice 2 3 Simplifying Variable Expression in seconds. Get started now.
- Push the“Get Form” Button below . Here you would be introduced into a dashboard that enables you to carry out edits on the document.
- Pick a tool you need from the toolbar that pops up in the dashboard.
- After editing, double check and press the button Download.
- Don't hesistate to contact us via [email protected] regarding any issue.
The Most Powerful Tool to Edit and Complete The Practice 2 3 Simplifying Variable Expression
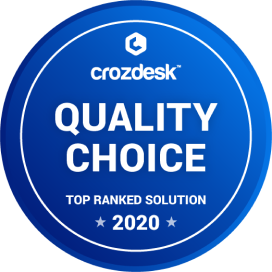
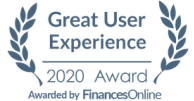
A Simple Manual to Edit Practice 2 3 Simplifying Variable Expression Online
Are you seeking to edit forms online? CocoDoc has got you covered with its comprehensive PDF toolset. You can get it simply by opening any web brower. The whole process is easy and quick. Check below to find out
- go to the free PDF Editor page.
- Drag or drop a document you want to edit by clicking Choose File or simply dragging or dropping.
- Conduct the desired edits on your document with the toolbar on the top of the dashboard.
- Download the file once it is finalized .
Steps in Editing Practice 2 3 Simplifying Variable Expression on Windows
It's to find a default application which is able to help conduct edits to a PDF document. Fortunately CocoDoc has come to your rescue. View the Manual below to form some basic understanding about possible methods to edit PDF on your Windows system.
- Begin by acquiring CocoDoc application into your PC.
- Drag or drop your PDF in the dashboard and make alterations on it with the toolbar listed above
- After double checking, download or save the document.
- There area also many other methods to edit PDF forms online, you can check it out here
A Quick Guide in Editing a Practice 2 3 Simplifying Variable Expression on Mac
Thinking about how to edit PDF documents with your Mac? CocoDoc has got you covered.. It empowers you to edit documents in multiple ways. Get started now
- Install CocoDoc onto your Mac device or go to the CocoDoc website with a Mac browser. Select PDF file from your Mac device. You can do so by clicking the tab Choose File, or by dropping or dragging. Edit the PDF document in the new dashboard which provides a full set of PDF tools. Save the paper by downloading.
A Complete Guide in Editing Practice 2 3 Simplifying Variable Expression on G Suite
Intergating G Suite with PDF services is marvellous progess in technology, a blessing for you simplify your PDF editing process, making it quicker and more cost-effective. Make use of CocoDoc's G Suite integration now.
Editing PDF on G Suite is as easy as it can be
- Visit Google WorkPlace Marketplace and search for CocoDoc
- set up the CocoDoc add-on into your Google account. Now you are all set to edit documents.
- Select a file desired by hitting the tab Choose File and start editing.
- After making all necessary edits, download it into your device.
PDF Editor FAQ
Why are people so confused about PEMDAS?
“Why are people so confused about PEMDAS?”There are many reasons that people are confused about PEMDAS.Being written as PEMDAS makes it appear as a sequence of individual letters, so there is a tendency to think that the corresponding operations are intended to be processed in the same sequential order. However, multiplication and divisions are intended to be done together, and additions and subtractions are intended to be done together. It would be more accurately written as PE[MD][AS], but that now looks more complicated and, therefore, confusing to keep straight which two pairs there are and which two letters are not paired. It turns out that the intent is more accurately expressed as PEDMSA and work sequentially through the individual letters, but that would make an acronym that is not easily pronounced like a word, which some educrats think is a bad thing.The concept of P for parentheses is too restrictive and many people do not realize what all comes under that categorization besides parentheses(): brackets[], braces{}, vinculum (horizontal bar that is used to indicate what is grouped together in a numerator and grouped together in a denominator along with indication of the operation being division as in [math]\frac{25-16}{5+4}[/math] so that the subtraction and addition are each to be done before the division, and it is also used to extend a radical sign to indicate what is being grouped together before extracting a root as in [math]\sqrt{9+16}[/math] to indicate the addition is to be done before the square root [exponentiation]). and superscripting as in 2¹⁺³ so that the sum is to be done before the exponentiation even though there are no explicit parentheses for the addition. Also, absolute value bars serve like parentheses along with directing the evaluation of an absolute value, such as |4 − 5| = 1; there are other operations whose notation similarly serves a dual purpose, such as floor and ceiling.People tend to be told that MD and AS are to proceed as pairs left-to-right, but they are usually not told what to do with a compound exponentiation though it should be clear when written with vertical stacking as opposed to linearized format common in texting and blogs. For example, [math]4^{3^2}[/math] is to be done top down, that is the [math]3^2[/math] first and then [math]4^9[/math], but that can be viewed also as right-to-left; left-to right is incorrect, so do not assume that the left-to-right of MD and of AS works for E. Writing 4^3^2 is actually bad syntax. Converting from [math]4^{3^2}[/math] to 4^3^2 loses information that the hierarchy of superscripts actually is a form of parentheses (see point 2 above), and linearizing the text causes those “parentheses” to be lost and they must be recovered by using explicit parentheses in the linearized text to convey properly the intended meaning. Thus [math]4^{3^2}[/math] is equivalent to 4^(3^2) and not to (4^3)^2. Writing 4^3^2 is bad practice because it does not give at all a clear indication as to which of the two distinct forms is really intended.The relationship between fractions and division is not conveyed well in elementary school. Both the fraction 3/4 divided by 5 and the number 3 divided by the fraction 4/5 are typically naturally written by students as 3/4/5 and yet the original two problems have two quite different answers: 3/20 versus 15/4, respectively. Related to this, students are taught that [math]\frac{1}{2}[/math] and 1/2 are equivalent when used as part of a larger expression, and such is not the case. This situation shows up in some interpretations of a set of rules called BODMAS in some countries nominally equivalent to PEMDAS. The B is appropriately more general sounding as brackets instead of what is implied by the P for parentheses. The O is variously referred to as orders, which are equivalent to exponentiation and roots, or as of, referring to fractions (2/3 of 12 instead of 2/3 times 12 being 8)—the orders meaning is fine but the of meaning throws a serious kink in the works. (Note: the third and fourth letter are to be paired, so MD and DM are equivalent.)PEMDAS is an oversimplified system because it is incomplete, contrary to what teachers tend to convey in primary and secondary school. It does not tell you the sequence of steps to evaluate every mathematical expression no matter how complicated, though many teachers try to convey that impression. The most egregious incompleteness is where unary operators fit into the scheme—they are not mentioned at all in PEMDAS. The most common unary operator is the unary −, such as in −x + y, which has different meaning than −(x + y), with the former meaning to negate (take the additive opposite) the x and then add y, whereas the latter means to add x and y first, then negate the sum. The expression −x can be thought of as meaning either 0 − x or as (−1) · x; at an intuitive level, the former is probably easier to comprehend, but we will see from a technical, manipulative standpoint the latter is really more appropriate. [From a practical, everyday standpoint for simple arithmetic it does not matter which viewpoint you take—the same answer results.] Now, if we get a little more complicated, like −3², is this to be interpreted as squaring the quantity −3 (performing the unary − first) to get 9 or as the negative of the square of 3 (exponentiating first) to get −9? Standard practice by professional mathematicians is the latter: perform the square first and then the unary −. Some people object that the − is intimately connected to and part of the number −3 (and Excel does this, evaluating =-3^2 as 9), but for consistency with other elements of algebra that cannot be the case. The notation −3 is describing a negative real number but by using notation that is actually a unary − operator applied to the number 3. This becomes more clear when we use variables rather than specific numeric values as in −x² where we clearly have two operators—squaring (and exponentiation) and a unary −, the latter of which we cannot say is intimately coupled with the variable x. Consistency demands that we treat −3 like we treat −x. An even better example is with multiplication of complex numbers, such as (3 + 4i)(5 − 2i), which we carry out exactly in parallel with (a + bi)(c − di); in both cases we treat the + and the − as binary operators and each of the 4, 2, b, and d each being multiplied with i. Based on the discussion so far, unary operators need to be done after exponentiation and somewhere around multiplication, division, addition, and subtraction, but can we pin it down more specifically? Yes. There are numerous other unary operators. The absolute value, floor, and ceiling operations mentioned in point 2 are unary operators. However, there are others that surprise some people and these unary operators have some very key consequences. Many people are used to expressions with trigonometric functions, such as sin(2u). Now an operator is actually nothing more than a function with a special syntax. For example, the addition operation a + b can be expressed as an addition function +(a, b); it is just a matter of commonness of usage that we are much more used to the operator form in this case. However, it often works the other way round as well. The functional notation sin(2u) can be written in unary operator form sin 2u as well, and commonly is by professional mathematicians. Conventional practice among professional mathematicians is to use sin 2u to mean that u is first to be multiplied by 2 and the sin applied to the product. Does that mean that multiplications (and, therefore, divisions) are to be done before unary operations? Let’s look at a more extensive example before jumping to conclusions. The first step in expanding sin 4u would commonly be written by professional mathematicians as:sin 4u = 2 sin 2u cos 2u.In order for this to work correctly, we cannot do all of the multiplications before we do all of the unary operations. The multiplication of the 2, the sin 2u, and the cos 2u cannot be done until the sin and the cos are applied to the 2u; in turn, the sin and cos cannot be applied to the 2u until the multiplication 2u is done. That makes it seem that unary operations have to join the MD pair and all are done together. However, that would imply left-to-right, but the 2u on the right must be done before the sin and the cos on the left. Contradictions are abounding. There is, nevertheless, a simple remedy. Note that the factors in the multiplications that are to be done early are concatenated together like a single entity, whereas the factors in the multiplications are to be done later are separated by spaces. This is not a coincidence—mathematicians try to develop notation that is not merely individual symbols meaning something but also the form helps guide the eye more quickly to the correct interpretation. The eye couples things more quickly the closer they are together. Thus, juxtaposition is used to indicate products to be done earlier, while spacing or explicit multiplication symbols (× or ·) indicate delayed multiplications. Mathematicians also like consistency. If we need to do this when unary operations are involved, we should use the same rules when unary operations are not involved. This means that there are two levels of multiplication—the level with products indicated using juxtaposition of factors to be done before the level with products indicated with spacing or with explicit multiplication operators. This is contrary to PEMDAS, in which all multiplications are to be done at the same level and, not only that, but all divisions are to be done at that same level as well, in a left-to-right sequence. In fact, divisions are generally regarded, with some caveats, as being at the same level as non-juxtaposed multiplications. As a result, some of the “trick” arithmetic problems posted on the Internet have the correct answers depending very keenly on how they are written:6/2(3) = 1 because all juxtaposed multiplications , and 2(3) is considered juxtaposed as there is no space and no explicit multiplication symbol, are to be done before all divisions, so 2(3) = 6 is divided into 6 to yield 1;6/2 × 3 = 9 because 2 × 3 is an explicit multiplication, and such multiplications are to be done at the same level as all divisions left-to-right and here the division 6/2 is on the left and to be done first, yielding 3 that is to be multiplied by 3 to yield 9.For those who object based on 2(3) meaning 2 × 3, that applies only as a standalone expression, not to be substituted as part of a larger expression. Blindly applying standalone substitutions in situations that involve parts of larger expressions usually results in error: For example, it is true that 7 = 3 + 4, but it is invalid to substitute 3 + 4 for 7 in the expression 7²: 7² does not equal 3 + 4²; instead one needs to parenthesize the 3 + 4 as part of the substitution. Similarly, one needs to parenthesize 2 × 3 as (2 × 3) when replacing 2(3).The biggest confusion by far is why are secondary school teachers conveying PEMDAS as the one correct way to evaluate all mathematical expressions when professional mathematicians use a different approach that does not always yield the same outcome. Professional mathematicians do NOT use PEMDAS. PEMDAS requires interpreting 1/2a as (1/2)a; professional mathematicians interpret 1/2a as 1/(2a), which is different except when a = ±1—remember all juxtaposed multiplications, which 2a is, are to be done before all divisions. My expectation is that secondary school teachers will not convey material in a subject that is contrary to how professionals in that subject do business. I am confused as to why they would teach PEMDAS as the end-all mathematics rule when professional mathematicians do not use it. For younger students who do not have the foundations nor the level of maturity to handle the full-up rules of actual practice, I understand oversimplification but only with letting the students know that as they get to more advanced courses the rules will get tweaked, but the simplified rules will do fine for now—especially do not claim this is the final and complete set of rules. Sadly, this is far from being the only mathematics topic that is taught in secondary schools contrary to professional practice.It should be noted that some major scientific and technical organizations have been so disturbed by the adverse influence of PEMDAS on interpretation of expressions like 1/2x, that they have further altered the rules so that expressions such as a/bc and a/b/c have become regarded as unacceptable syntax due to ambiguity resulting from conflicts of PEMDAS and standard professional practice. In other words, the meaning of such expressions is officially considered to be undefined, and the expressions must be rewritten using the vinculum or parentheses to indicate explicitly what is intended to be in the numerator and what is intended to be in the denominator, or treat everything as factors in a product with an exponent of −1 where division is intended—whatever it takes to avoid having a multiplication or another / to the right of a / in a term unless parentheses explicitly indicate the intended order.
Can you explain to non-coders the most impressive code you've seen?
Don’t be surprised if understanding this answer would take you many days, weeks or even months. But if you value intellectual development for the sake of itself, I think you should be satisfied. Frankly speaking, I’ve found most other answers here completely disappointing.Also, if you find something unclear, or if you think that some parts could be explained better, feel free to leave a comment.IntroductionThe most impressive code that I have seen comes from Daniel Friedman and William Byrd, who came up with the idea of “running the evaluator backwards”.This phrase, however short, might be impenetrable to non-programmers, because it refers to some notion of “evaluator” and uses a rather unclear metaphor of “running things backwards”, both of which I'm going to explain.Historical and conceptual backgroundBut, to get a clearer picture, we should go back to the times before computers were invented. In those times, people were doing a lot to improve the language of mathematics, in particular to clarify the basic ideas and to refine the mathematical notation. They managed to develop some systems — called formal systems — which were precise and which could encode all the prior mathematical knowledge.These formalisms changed the perspective of mathematical inquiries: since mathematical theorems and questions are just sequences of symbols, perhaps we could forget about the meanings of those symbols, and just look at their form, and still arrive at interesting conclusions?This idea was put to an extreme by a great and famous mathematician known as David Hilbert. (Since the targetted audience of this answer consists of “non-coders”, I suppose that pasting his picture here might be a good idea.)He suggested, in 1928, that there might exis a systematic procedure, which takes a formal representation of a mathematical “yes-no” question, and solves it in a way that only requires applying deterministic rules, and does not require thinking or understanding the subject matter, and produces an answer (obviously, either “yes” or “no”).This question, known as Entscheidungsproblem (which is German for “decision problem”), was a direct inspiration for the invention of computers.In 1936, a young mathematician called Alan Turing invented a model of computation known today as “Turing machine”, which was used to show that Hilbert’s problem does not have a general solution: that if we encode some questions in a formal language, then every systematic attempt to answer them will result in a process that will never terminate.Since you’re a non-coder, and you’re accustomed to wasting your miserable life on Instagram, you’re probably interested how Turing looked like. There you go:A Turing machine has been described as a machine that can read symbols from a (potentially infinite) tape of “memory cells” and write symbols to that (or some other) tape. Each cell must be able to store at least one bit of information.The machine can roll the tape one cell left or one cell right. An important part of the description of a machine is its state transition function: there is a finite amount of states that a machine can be in.Designing Turing machines which perform basic aritmetic operations such as addition or multiplication is a common exercise among Computer Science students. I don’t want to get too technical with this yet, but I want you to embrace one critical idea that Turing had, which is that you candesign a Turing machine which reads a description of another Turing machine and an input for that machine, and which simulates the operations of the machine whose description it read.If you’ve ever used a Nintendo emulator on your smartphone, this probably shouldn’t be very surprising to you. But this simple idea is actually at the core of computing. Your operating system is a process which manages descriptions of other processes. Obviously, the authors of your operating systems had to form a description of this process.I want to turn your attention to this interplay between “description” and “process”. The process generated from a given description will depend on how the hosting process “understands” that description.But where does this “understanding” in the hosting process come from?It might come from another hosting process (if it happens to be “simulated” on some other machine), or it might come from the laws of physics (when a physical device such as a microprocessor “interprets” that description). Or it might come from you, from your understanding, if you’re, for example, simulating a Turing machine in your head (which is something that humans are perfectly capable of, at least as long the machines aren’t too complex).The evaluatorLet’s now move some two decades ahead, to the late 1950s. Some real computers already existed at that time, and — in addition to performing physical simulations and engineering computations, some people were trying to do some more ambitious things with them. In particular, they wanted to provide those real computers with human-like intelligence.Among them was John McCarthy. Here’s the obligatory picture:The thing that McCarthy is famous for is the invention of the LISP programming language.The fundamental problem that LISP aimed to solve was to conceive a system (or a notation) that could be used as general knowledge representation that would be easy to work with for both humans and machines.Initially LISP was many things, but the thing that is mostly associated today with is the notation that McCarthy invented for representing data. It is called “s-expressions”. An s-expression is either atomic expression (sequence of characters that do not contain whitespaces or parentheses and do not consist of a single dot), or a sequence of s-expressions separated by whitespaces and surrounded by a pair of matching parentheses. (If there’s more than one element, then the last element can be preceded with a dot.)Here are some examples (each line contains one s-expression, possibly compound):2 + (+ 2 2) (a . b) (a b . c) (a . (b . c)) () define (+ (* 2 3) (/ 4 5)) (define (abs n) (if (< n 0) (- n) n)) The dot has a special meaning because of another design goal of LISP: it was intended as a system for LISt Processing (hence its name).LISP was built around the following idea: a list can either be empty, or it can be something consisting of a first element (head) attached to a list containing the remaining elements (tail).This explains how we can build complex structures (by prepending elements to some existing simpler structures) but also how we can decompose those structures.Consequently, every list is built from elementary structures called pairs. A pair consisting of some elements b and c can be written down as (b . c). If we construct a new pair whose head is a and whose tail is that previous pair, we get (a . (b . c)). But in LISP this can also be written down more conveniently as (a b . c). Moreover, if c (more generally called “last tail”) is an empty list (), then the face of the expression is further simplified to (a b).The things that I’ve described so far may seem unfamiliar, abstract or even weird, but they aren’t difficult. Actually, they reflect a necessary property of every action: either we're already done, or there is still something left to do.Lists in LISP can be nested arbitrarily, which makes the LISP notation suitable to represent trees with any number of branches in a very simple and straight-forward manner.Why is this important?It turns out that, for some reason, trees appear a lot in language processing. Expressions in many diffent languages, such as English, the language of mathematics or most programming languages can be analyzed and visualized in the form of trees. For example, the parse treecan be written down using the notation invented by McCarthy as(S (N John) (VP (V hit) (NP (D the) (N ball)))) I don’t really know why trees are so ubiquitous. Probably because we can only understand complex things by analyzing them in simpler and more familiar terms (and, similarly, we can only build complex things from simpler ones).LISP provided a way of expressing things that was easy to analyze and process to both humans and computers, which is why it has been the main work horse of Artificial Intelligence for a few decades.But the above description doesn’t explain everything there is to be explained about LISP. Except the weird but simple notation, LISP brought with itself a particular idea of how to perceive computations, namely — by reducing complex expressions to simplest possible terms.This idea wasn’t original in the sense that it was already widespread in mathematics. For example, under the common rules of arithmetics, the expression(+ 2 2) should be reduced to 4.What was perhaps more novel was that, in addition to numbers, LISP was also able to process symbols (one of its first applications was symbolic differentiation) and functions.A function is something that, when receives an argument (or a few arguments), produces a value. For example, the expression(function (x) (* x x)) denotes a function which takes one argument, and multiplies it by itself. We could apply this function to some argument, say, 5:((function (x) (* x x)) 5) As you could expect, this expression should reduce to 25.Expressions like this quickly get rather hairy, and to simplify them, we often name some intermediate terms. For example, a common name for a function which multiplies its argument by itself is ‘square’.(define square (function (x) (* x x))) This allows us to write an expression equivalent to the above one as:(square 5) which is much easier to read.But readability isn't the sole purpose of naming things. One powerful feature of LISP was that it allowed do express ideas using recursion or a self-reference. But, if you know anything about recursive definitions, you should realize that in order to make them work, we need to be able to specify at least one non-self-referential case (otherwise the joke that “in order to understand recursion, you only need to understand recursion” wouldn’t be funny. Oh, wait a minute!). LISP provides the if form, which can be used precisely for that.A simple example usage of the if form is the definition of absolute value, which, when given a negative argument, gives a negated argument (to make it positive), and otherwise it just returns its argument left intact:(define absolute-value (function (x) (if (< x 0) (- x) x))) As you can see, if has the following form:(if <condition> <consequent> <alternative>) It first evaluates <condition>, and when its result is true, the value of the whole expression becomes the value of <consequent>, and otherwise it becomes the value of <alternative>.So for example,(absolute-value -5) can be replaced with(if (< -5 0) (- -5) -5) Now the value of the condition (< -5 0) is true (because -5 is smaller than 0), so the value of the whole expression becomes the value of the consequent (- -5), which is 5.On the other hand, if we wanted to know the value of(absolute-value 6) then we know that it's equivalent to(if (< 6 0) (- 6) 6) Now the value of the condition (< 6 0) is false (because 6 isn’t smaller than 0), so the value of the whole expression becomes the alternative 6.I hope that you find this obvious to the point of being boring. The if form can be used to define common logical connectors like conjunction (and) and disjunction (or). Those are typically special ‘syntactic forms', such that(and <a> <b>) is interpreted as(if <a> <b> #false) and(or <a> <b>) is interpreted as(if <a> #true <b>) (The names for logical values true and false begin with the # character to make sure that no one could redefine them).In case you ever wondered, you can define logical negation as a function:(define not (function (condition) (if condition #false #true))) I hope that you didn't find the examples that I used so far, very off-putting. They relied on some mathematical knowledge of things like numbers and some operations on them (multiplying, subtracting and comparing). I did that, because I thought that many people are familiar with things like “squaring” or “absolute values”, and so I thought that it should be easier to get to them by leveraging the things they already know.But, while this can help some people, it can also be a barrier to other people. So while there are many well known recursive functions that operate on numbers, before I get on to recursion, we shall elaborate a language for talking about symbolic expressions.We already said something about them when we were discussing the s-expressions. We said that a list can either be empty or it can consist of a head and a tail (where the tail is also a list).We also said that you can construct a list by prepending an element to the front of some existing list.The basic operation of prepending an element to the front of a list is traditionally called cons. In particular, if y is the list (1 2 3), then(cons 0 y) will produce the list(0 1 2 3) Or, speaking more broadly, the operation(cons a b) produces a pair(a . b) for any two values a and b.It should therefore be clear that, for example, the expression(cons 1 (cons 2 (cons 3 4))) would evaluate to(1 2 3 . 4) Given a value, we can ask whether it is a pair. The pair? function does that, so for example the value of the expression(pair? (cons 1 2)) is true, whereas the value of the expression(pair? 5) is false. (It is a common convention among some programmers to use names that end with question mark for functions which answer yes-no questions.)Having a list resulting from evaluation of expression (cons a b), you can obtain the head and the tail of that list using the head and tail functions:(head (cons a b)) produces a, and(tail (cons a b)) produces b.These four functions, cons, pair?, head and tail, are sufficient for working with arbitrarily complex tree structures. But it is also convenient to be able to refer directly to the first few elements of a list:(define first (function (list) (head list))) (define second (function (list) (head (tail list)))) (define third (function (list) (head (tail (tail list))))) (define fourth (function (list) (head (tail (tail (tail list)))))) You can imagine this going on as long as you like (but if you have the urge to talk about a larger number of elements, there are usually better ways to achieve that).LISP also provides a convenience function called list, such that, say(list 1 2 3) produces the list (1 2 3). Of course, you could build the same list using the cons operator, so the list function doesn’t add anything to the expressive power of our language. If we wanted to, we could leverage the way LISP handles many arguments to define it ourselves (but it would feel like cheating):(define list (function args args)) (Note the lack of parentheses around the first occurrence of args)I mentioned before that LISP can deal with symbolic expressions. Since expressions are essentially tree structures, we have the second part of the phrase “symbolic expressions” covered. But what about the “symbolic” part?We have already seen some symbols in the programs that we wrote, like +, function, x, *, define, square, cons and so on. Some of those symbols had their meanings. Others received some meaning from us: we used the define form to assign some meanings to symbols like square or absolute-value.But we never talked about symbols themselves — only about their meanings.LISP provides a special operator called quote, which allows to refer to symbols and symbolic expressions, rather than their meanings.For example, the value of the expression(quote x) is the symbol x. There is a built-in function symbol? which says whether a given object is a symbol or not.But, in addition to quoting symbols, you can also quote whole expressions. The value of the expression(quote (+ 2 2)) is a list of three elements, whose first element is the symbol +, and whose second and third element are instances of the number 2.The expression (quote x) can be abbreviated as 'x (which makes the notation more succinct).One of the most important properties of a symbol is its identity. Roughly speaking, two objects are considered the same symbol if they are both symbols and they “look the same”. So for example the symbol x is the same as the symbol x, and the symbol y is the same as the symbol y, but the symbol x is not the same as the symbol y.This may sound like an obvious thing to say, but actually the notion of identity is extremely confusing, and almost every programming language gets it somehow wrong. The particular primitive notion of identity in LISP is associated with the eq? predicate. Two LISP objects are considered eq? if they are located in the same place of computer memory — and once a symbol is read into memory, it becomes identified with its location in that memory. (When a symbol is read, the system checks whether it has already seen a symbol like that)But this isn't the case for pairs. Each time you call the cons function, it may return a new pair from some free area of memory. For this reason, it may not be the case (and generally isn't), that, say, (eq? '(1 2 3) '(1 2 3)).The only list which is guaranteed to be eq? to every other list with the same content is the empty list: (eq? '() '()) is always true.But we can use this primitive notion of identity to implement a more intuitive one, where the lists that contain the same elements in the same order would be considered the same.(define equal? (function (a b) (or (eq? a b) (and (and (pair? a) (pair? b)) (and (equal? (head a) (head b)) (equal? (tail a) (tail b)))) ) ) ) As you can see, we have referred to the term being defined in the body of this definition. But that's ok, because we have one non-recursive case, and the size of arguments will shrink towards that case with each recursive call.Another example of recursion can be found in the definition of the append function, which takes two lists and appends them together, so for example (append '(a b) '(c d)) would produce the list (a b c d).The base case of the recursion is when one of the lists is empty — in such case, we'd just need to return the other list.As to the recursive step, let'd recall that the cons operation is only able to prepend a new element to the front of some existing list — so it should make sense to prepend elements from the first list to the second.Obviously, (append '() b) should be b. If we wanted to prepend a single element list, then we'd just need to cons this element to the second list:(append '(a) b) is the same as(cons 'a b) It may take some insight to observe that b is the same as (append '() b), and that '() happens to be the tail of '(a).This prompts us with the following definition:(define append (function (a b) (if (pair? a) (cons (head a) (append (tail a) b)) b))) The heavily parenthesized syntax of LISP may not be particularly readable. In languages with richer syntax, such as Haskell, you could express the same idea in two lines of code:append (h:t) b = h:(append t b) append [] b = b Here, the : operator served the purpose of cons. We don't need the head and tail functions, because using that operator on the left hand side of the = operator allows us to name the head and the tail of a list however we like (here we used the names h and t).An even simpler and more natural example of a recursive definition is a function that takes a list of elements and a unary function, and produces a new list, resulting from applying the function to each element. It is customarily called map, so you can expect that(map square '(1 2 3)) would produce(1 4 9) The definition should follow this reasoning: if the list of elements is empty, then the result must also be empty. If it's non-empty, then we need to apply the function to the first element, and cons it with the result of map on the tail of the list.The definition of map in Haskell would look like this:map f (h:t) = (f h):(map f t) map f [] = [] In LISP, an equivalent definition would be:(define map (function (f l) (if (pair? l) (cons (f (head l)) (map f (tail l))) '()))) Those examples should give us some taste of recursive thinking. It may seem that, since Haskell version is probably easier to grasp, it would make no sense to stick to this pile of nested parentheses.But the spartan syntax of LISP has a virtue that no other syntax I know has: the expressions of the language, which is suited for processing lists of symbols, are themselves just lists of symbols.So far we have learned about the following four core forms of the LISP language, namely: define, function, if and quote (we also learned about some built-in functions such as *, -, cons or eq?, but that’s not important at this moment).I have (somewhat) explained — using English prose — how those forms should be understood.Now here's the fun part: LISP is also a language, and it can itself be used to explain the meaning of the core forms of LISP. This is a very similar idea to that of Universal Turing Machines that I mentioned earlier.Normally, a programming language comes with a bunch of built-in functions (like number multiplication) that are associated with some names (like the * symbol). This association can be captured in a structure called environment.An environment can be represented by a list of pairs whose heads are names, and whose tails are values. For example, a structure that would hold some built-in LISP functions discussed here could be defined as:(define initial-environment (list (cons '* *) (cons '+ +) (cons '- -) (cons '= =) (cons '< <) (cons 'list list) (cons 'cons cons) (cons 'head head) (cons 'tail tail) (cons 'pair? pair?) (cons 'symbol? symbol?) (cons 'eq? eq?))) In order to use those functions, we need to be able to look them up by name. Assuming that an association is present in our list, it can either be the first element of the list, or it can be somewhere on the rest of the list:(define lookup (function (key dictionary) (if (eq? (head (head dictionary)) key) (tail (head dictionary)) (lookup key (tail dictionary))))) For the purpose of presentation, we can assume that a LISP program can be seen as a sequence of definitions followed by a single expression. Then, each definition wiill augment the environment with a proper binding. Running a program will mean reading all the definitions and then evaluating the final expression:(define run (function (prog env) (if (is? (head prog) 'define) (run (tail prog) (cons (cons (second (head prog)) (third (head prog))) env)) (value (head prog) env)))) Where is? is a simple utility function that checks whether the head of an expression is eq? to a particular symbol:(define is? (function (exp type) (and (pair? exp) (eq? (head exp) type)))) The actual point of the run function is just to collect the values from definition into environment, so(run '((define x1 v1) ... (define xN vN) expr) env) is equivalent to running(value 'expr (append (list (cons 'xN 'vN) ... (cons 'x1 'v1)) env)) The heart of the evaluator is the value function, which takes an expression and an environment and returns the value of that expression. Since there are a few different types of expressions — quote, function, if, and, or, application, symbol and numeric or boolean value, the function will need to check the type and act accordingly. The following definition of value is scattered with my explanations(define value (function (exp env) If the expression is a symbol, then we need to look up its value in the environment (to make the recursion work, we also calculate the value after the lookup): (if (symbol? exp) (value (lookup exp env) env) In case of quote, we simply return the quoted expression: (if (is? exp 'quote) (second exp) In case of if, we first evaluate the condition (second subexpression). If it succeeds, we return the value of the consequent (third subexpression), and otherwise we return the value of alternative (fourth subexpression): (if (is? exp 'if) (if (value (second exp) env) (value (third exp) env) (value (fourth exp) env)) In case of and and or, we transform them to use the if form, and then evaluate: (if (is? exp 'and) (value (list 'if (second exp) (third exp) #false) env) (if (is? exp 'or) (value (list 'if (second exp) #true (third exp)) env) We can consider function to be self-evaluating: (if (is? exp 'function) exp The case of function application will be explained shortly. Note that we apply the value of the head of a function to the values of arguments: (if (pair? exp) (applied (value (head exp) env) (map (function (arg) (value arg env)) (tail exp)) env) If the expression does not belong to any of the aforementioned types (e.g. it is a number or a boolean value), then we do not reduce it any further. exp))))))))) This ends our definition of value. (Aren't all those closing parentheses beautiful?)In case of function application, we need to consider two cases. If we are applying a function provided by a host implementation, then we need to delegate the application to the host. Otherwise we shouldextract the argument list from function (that’s second element, right after the function keyword)extend the environment with new bindings which map argument names to the valuesrun the body of the function (that is, tail of tail) in this extended environment (we use run rather than value, because this allows to use the define form inside the function form)This is how this might be done in practice:(define applied (function (operator arguments env) (if (host-function? operator) (host-apply operator arguments) (run (tail (tail operator)) (extended env (second operator) arguments))))) We assume that the host provides the functions host-function? and host-apply for us. This assumption is convenient for testing, but if you find this confusing, you can ignore it and assume that there are no built-in functions and that the initial environment is empty.The only thing that’s left to explain is how to extend the environment with bindings from argument names to argument values. We have essentially two cases to consider here: if the list of arguments is empty, then we are done. Otherwise we take the first argument, bind it with the first value, and extend the environment with the tails of argument names and values.In order to handle functions with variable number of arguments (such as list), we can also consider a third case: if the tail of argument names is a symbol, then we bind this symbol with the whole list of values. In order to prevent the values from being evaluated too many times, we quote them:(define extended (function (env names vals) (if (pair? names) (extended (cons (cons (head names) (list 'quote (head vals))) env) (tail names) (tail vals)) (if (symbol? names) (cons (cons names (list 'quote vals)) env) env)))) That’s it. This program, whose entry point is — in our case — the run function — is called “metacircular evaluator” (the “metacircular” adjective is just a fancy name of saying that it implements the language that it is itself implemented in. In a sense, you can think of it as a program whose purpose is to explain itself).It isn’t very useful by itself — after all, the only thing it can do is slow down the execution of your program (assuming that you already have a running LISP interpreter on your machine — otherwise it just won’t work). It wasn’t intended to be useful, though — John McCarthy, who conceived it, intended it as a program for reading.However, one of his students, Steve Russell, took this program and translated it to the machine language of the IBM-704 mainframe computer, which made LISP a practical programming system. This happened in 1958, and this is how the computers looked like back then:So we now know what an evaluator is: it is a program (or a device) which attributes interpretations to other programs. We managed to fit it in a few dozen lines of code, using a handful of definitions.At least we understand one word from the phrase “running the evaluator backwards” — what’s left is to explain what we mean by the phrase:Running things backwardsSo far, we have presented a model of programming where computation was modelled as a reduction of complex expressions to the simplest terms. This isn’t the only conceivable model, and we have already mentioned a different one (Turing machines).Now we’re going to see a yet different model, which resembles the work of a detective.What detectives do is they gather evidence and build possible images of some situation. Their goal is to settle facts, to synthesize knowledge from different sources and to spot possible inconsistencies between different testimonies. The ideal that they try to pursue is the truth.So far, the notion of truth manifested itself in our programs in that we could ask some yes-no questions (like “is this number smaller than 0?”) and act differently depending on the answer. But sometimes we perform the reasoning in the different direction: we assume that some sentence is true, and then we ask what conditions need to be satisifed for that to be the case.In this model, we can imagine that a program consists of some facts and rules that are scattered around and contain some scraps of knowledge, and our task is to synthesize a consistent world view (or to report that different scraps of information are contradictory and that no such world view can exist).One question is how to represent this knowledge. When I was introducing LISP, I suggested that — in addition to being a programming model — it provided a notation for representing knowledge (which essentially was a format for representing trees as sequences of symbols and parentheses). The idea of representing knowledge as trees seems to be a good one, but we should also be able to represent our “lack of knowledge”, or the fact that our knowledge is often incomplete — for example, we might know that “someone stole the car”, but we don’t know who it was.The structures that represent incomplete knowledge are also useful for representing questions. Broadly speaking, you can divide questions into two groups: “yes-no” questions (“did someone steal the car?”, “did John steal the car?”) and questions containing pronouns (“who stole the car?”, “what was stolen?”).Of course, we understand that the role of pronouns like who, what or some, and nouns like something or somebody or everything, is different than the role of most nouns and proper names: usually, nouns are used to refer to some known things (like “plants are green” or “it was John who stole the car”).There is also a very significant difference between general sentences like “all plants are green”, and particular sentences like “John stole the car”. In the case of general sentences, we ought to understand them as rules:whatever is a plant, must also be greenor equivalentlyfor any X, if X is a plant, then X is greenOn the other hand, particular sentences are just unique facts about the world. The sentence “John stole this car” only says something about a single particular event that occured once, and doesn't allow us to conclude that “John steals every car” or anything like that.To summarize, we'd like our programming system to be able to represent (at least) the following:factsrulesincomplete knowledge/questionsIf you look at the examples that I gave so far, you can observe that they either stated that some objects have some properties (plants are green), or that some relation between some set of objects holds (John stole the car).Since sentences in natural language such as English tend to be ambiguous, we are going to use LISP's s-expressions to represent our relations. We are going to write them down as follows:(<relation> <object-1> <object-2> ...) (where properties are just relations with one argument). For example(stole 'John 'the-car) (green 'this-tree) We use quotation to represent individual names — remember that we also want to be able to represent things like pronouns (who, what, someone, everything etc.). Since — in more complex situations — using pronouns could be ambiguous, we're going to allow arbitrary (unquoted) symbols to be used in this role.So for example if we ask our system(stole 'John what) (or: what did John steal?), we expect that one of the possible answers would be something like((what . the-car)) Now that we chose some (rather straightforward) representation for relations, we need to come up with a way of representing rules and facts.People who developed this style of programming suggest to write the consequence of the rule before the conditions that trigger that rule, so we could write them as:(conclude <consequence> from <conditions> ...) where <consequence> is a relation, and <conditions> is zero or more relations. The interpretation is that all of the <conditions> need to be satisfied to be able to derive the <consequence>.If there are zero <conditions>, then the <consequence> is assumed to be true — in such case we are dealing with a fact, and we could use a slightly simplified notation for such situations, i.e. skip the from keyword:(conclude <fact>) We could therefore write our examples as:(conclude (stole 'John 'the-car)) (conclude (green X) from (plant X)) Now we have an idea how to augment our model with facts and rules. The only thing that’s left to design is how to ask our model questions.We need to be careful here, because in general there can be more than one answer to our question (John could have stolen more than one thing, for instance). Moreover, in some situations there could even be infinitely many answers to our question, and in such cases, the program could consume all the available memory and never finish anyway.So our interface for asking questions should take this fact into account, and enable us to limit the number of answers that we get. So for example, we could write(some 5 (stole 'John what)) to get an answer which could look like(((what . the-car)) ((what . the-necklace)) ((what . the-money)) ((what . the-bracelet)) ((what . Janes-heart))) This should give us some idea how to talk to our system.We are now going to demonstrate how this relational model of computation should be able to express some things that were expressible in the functional model from LISP. Then we are going to embed this model in the LISP model, that is, have our conclude and some forms processed by some LISP functions.Let’s recall the definition of append (for brevity, we’re going to use the Haskell version here):append (h:t) b = h:(append t b) append [] b = b In the relational model, there are no “function arguments” and “function values”. All we have is relations that hold (or “are true”). But we can emulate the behavior of functions by adding extra argument. In the relational model, that’s going to represent function’s value (or output, if you will). Consequently, we can represent the base case of the recursion as the following rule (one thing I didn’t mention about our language was that relation objects can be lists — but this shouldn’t be too surprising, as we really don’t have many other ways to represent complex objects):(conclude (appending () b b)) The recursive step can be expressed using the following rule:(conclude (appending (h . t) b (h . l)) from (appending t b l)) For example, we can expect that the following query should be true:(some 1 (appending (1 2) (3 4) result)) Let’s think for a second how this should work. Our reasoning engine is going to search through all the applicable rules for the appending relation.The first rule (the base case) is not going to be useful here, because the lists () and (1 2) differ.But the second rule is going to match with h having the value 1, t having the value (2), b having the value (3 4) and result having the value (1 . l), provided that the condition (appending (2) (3 4) l) succeeds.To answer this question, we need to search our rules again, this time for the relation (appending (2) (3 4) l).The first rule fails, because the lists () and (2) differ. The second rule matches, with the h’ variable having the value 2, t’ having the value (), b’ having the value (3 4) and l having the value (2 . l’), provided that the condition (appending () (3 4) l’) succeeds.To answer this question, we need to search our rules again, this time for the relation (appending () (3 4) l’).Now the first rule succeeds, because the first argument is the empty list (). This allows us to conclude that l’ is the list (3 4). We can step back to see that l is (2 . l’), so we can conclude that it’s (2 3 4). Making a similar step leads us to the conclusion that result, which is (1 . l), must be (1 2 3 4).But we can now do things that the functional model wasn’t capable of doing easily. We called the last argument result to suggest that it stores the “result” of what was meant to be a “function”.But from the perspective of our programming model this argument isn’t any different from any other argument. So we could ask, for example, what list needs to be appended to the list (3 4) to obtain the list (1 2 3 4).(some 1 (appending what (3 4) (1 2 3 4))) The base rule doesn’t match because (3 4) and (1 2 3 4) differ. Therefore we look for the second rule, which assigns to what the value (h . t), to h the value 1, and to l — the value (2 3 4), and then tries to agree on (appending t (3 4) (2 3 4)). This time t receives the value (h’ . t’) and h’ receives the value 2, and we further ask about (appending t’ (3 4) (3 4)). We now see that the base rule matches with t’ being equal to (). This allows us to conclude that — since what is (h . t), t is (h’ . t’), h is 1, h’ is 2 and t’ is () — what must be (1 2).If we imagine that the direction “from arguments to the result” was “forward”, then the direction “form the result to arguments” could be called “backward”. This should be a hint for you how to interpret the phrase about “running programs backwards”: rather than getting a “result” (or “output”), we can obtain sets of arguments (or “inputs”) that would produce the desired result.Before be dare to interpret what would the phrase “running the evaluator backwards” mean, let’s try to be a bit more precise in describing how our reasoning system works.By now, we should be able to see that there are essentially two things going on here: searching through the available rules and matching variables with values, synthesizing and expanding our knowledge (or assumptions) along the way.One thing that we should be concerned with is how to deal with potentially infinite amount of results, and this is something that we’ll actually begin with.Let’s consider the sequence of natural numbers:[math]0, 1, [/math][math]2[/math][math], [/math][math]3[/math][math], 4, [/math][math][/math][math]...[/math]We can see a clear pattern here: each number can be constructed by adding 1 to the previous number. We could grasp this pattern in the following definitions:(define numbers-from (function (n) (cons n (numbers-from (+ n 1))))) (define numbers (numbers-from 0)) Suppose now that we’d like to learn the value of(first numbers) According to the rules specified in the value function, to learn the value of function application, we must first learn the values of all arguments, which in this case means numbers.numbers are, by definition, the result of applyting the numbers-from function to the value 0, which is the value of (cons 0 (numbers-from 1)). To learn the value of that expression, we must first evaluate all the arguments. In particular, we need to learn the value of (numbers-from 1). By definition, it’s (cons 1 (numbers-from 2)). To learn the value of that expression, we must first evaluate all the arguments…See the problem? We will never learn the first element of the list, because the evaluation will go on forever (or at least until some computer runs out of memory, or someone pulls the plug).Although we could modify the evaluator to delay the evaluation of arguments until their value is actually needed (this strategy, known as “lazy evaluation”, is supported by default by some programming languages such as Haskell), we could also use a certain programming trick to represent infinite sequences: rather than returning a list, we could return a recipe for creating a list:(define $numbers-from (function (n) (function () (cons n ($numbers-from (+ n 1)))))) (define $numbers ($numbers-from 0)) You may have noticed that we use the $ character as a prefix to the names of our objects. It suggests that these objects are potentially infinite sequences (or rather — recipes for generating sequences). They are slightly different to work with than lists — if we wish to get the first element of such sequence, we must first “follow the recipe”:(first ($numbers)) Note the additional pair of parentheses around the argument to first: we need them, because the $numbers object is not a list, but a function. It is a rather peculiar function, because it doesn’t take any arguments — its only purpose is to delay the computation. Applying “no arguments” to that function forces the delayed computation.Getting the second element of such infinite sequence is also a bit tricky. First we need to force the object to get a pair. Then, we need to force the tail of that pair, which will give us a new pair. Then it should suffice to take the head of that pair:(head ((tail ($numbers)))) You can see that it’s rather easy to make a mistake in the number of parentheses that you need. It may therefore be convenient to have a function that would convert some amount of initial elements of a stream to a list.(define take (function (n stream) (if (or (= n 0) (eq? stream '())) '() (if (pair? stream) (cons (head stream) (take (- n 1) (tail stream))) (take n (stream)))))) For example, (take 3 $numbers) would produce the list (0 1 2).There’s a lot of examples of beautiful programs that make use of infinite sequences, but since they have little to do with the merit of my answer, I won’t be showing them here.Now that we know how to deal with infinite sequences, we can get back to our implementation. As we noted before, the two essential things that our inference engine will be doing, are:searching through the available rulesmatching variables with values (synthesizing knowledge along the way)Probably the most intriguing part of the above description is this idea of “synthesizing knowledge”, so this is something that we’re going to begin with.Recall that we decided to represent our knowledge as “potentially incomplete trees”, i.e. trees that may contain holes (or variables). We can imagine a situation when there are two sources of information about the same thing, and the information from those two sources is partially overlapping. For example, one person might have heard that the car has been stolen, and other person might have heard that John stole something. Therefore we might deal with the following two bits of knowledge:(stole X 'the-car) (stole 'John Y) If we synthesize those two bits of information, we might conclude that(stole 'John 'the-car) (Of course intuitively this might be an invalid reasoning, but the point of this example is to show the process of knowledge integration, rather than the process of valid reasoning).It can also be the case that two bits of knowledge are contradictory or disjoint and cannot be integrated. For example, you cannot integrate those two pieces of knowledge:(stole 'John X) (broke 'John Y) The process of knowledge integration is, in some ways, similar to checking whether two LISP objects are equal?: if two pieces of knowledge are different symbols (or numbers), they cannot be integrated. If they are different variables (holes), they can be integrated only if those variables wouldn’t be bound to different symbols (or numbers). If one of them is a variable, and another is not, then we should be able to bind that variable to the value (which means that the variable must either be unbound, or it must be bound to something that can be integrated with the value). Otherwise, if they are both pairs, then we need to be able to integrate their heads and their tails.The historical name for this kind of knowledge integration is unification. Compared to equal?, it needs to take an additional argument that is going to represent bindings from variables to values or to other variables. Also, the return value of the function will either be false if the objects can’t be unified, or a list of bindings for variables that would allow to obtain a form that would integrate the knowledge from the two terms that are being unified.The bindings are going to be represented in the same structure that we used for representing environments in our evaluator — as a list of pairs. This allows us to re-use some functions that we’ve defined previously, like lookup. However, since we now consider a possibility that a symbol may be unbound, we need a way to know it:(define bound? (function (symbol bindings) (and (pair? bindings) (or (eq? symbol (head (head bindings))) (bound? symbol (tail bindings)))))) Now we can translate the logic described above to LISP:(define unify (function (x y bindings) (if (eq? bindings #false) #false (if (equal? x y) bindings (if (symbol? x) (if (bound? x bindings) (unify (lookup x bindings) y bindings) (cons (cons x y) bindings)) (if (symbol? y) (unify y x bindings) (if (and (pair? x) (pair? y)) (if (or (is? x 'quote) (is? y 'quote)) #false (unify (tail x) (tail y) (unify (head x) (head y) bindings))) #false))))))) We can now check that, say,(unify '('stole 'John X) '('stole Y 'the-car) '()) results with((X . 'the-car) (Y . 'John)) (if you ever try to run this code in some actual LISP system, you might get something like ((X quote the-car) (Y quote John)), but note that — given what we said earlier about quote and the . — those are just two ways of writing the same thing)This should give us some idea how to synthesize knowledge. What’s left is the strategy for applying the rules to our query.Recall that, in our evaluator, we considered a program to be a sequence of definitions followed by a single expression. Here, similarly, we can imagine a program to be a sequence of rules followed by a single query (compare it with the definition of run).(define answer (function (question assumptions) (if (is? (head question) 'conclude) (answer (tail question) (cons (conclusion (head question)) assumptions)) (take (second (head question)) (solutions (quote-head (third (head question))) assumptions))))) Similarly to run, the answer function simply converts the human-readable representation of a program into representation some internal representation that is easier to operate on. So for example, the expression(answer '((conclude (apd () b b)) (conclude (apd (h . t) b (h . l)) from (apd t b l)) (some N (apd (1 2) (3 4) X))) '()) is equivalent to(take N (solutions '('apd (1 2) (3 4) X) '((('apd (h . t) b (h . l)) ('apd t b l)) (('apd () b b))))) The conclusion function drops the from symbol from conclude forms (if present), which allows to transform the human readable (conclude <consequence> from <conditions> …) to a more compact form (<consequence> <conditions> …) that will be used for internal processing. It also makes sure that the first symbol, which is used for denoting a relation, gets quoted, so that forms like (stole 'John X) become ('stole 'John X):(define conclusion (function (form) (cons (quote-head (second form)) (if (pair? (tail (tail form))) (map quote-head (tail (tail (tail form)))) '())))) (define quote-head (function (relation) (cons (list 'quote (head relation)) (tail relation)))) The actual work is delegated to the solutions function. It needs to do the following:starting with empty knowledge, search through the rules to find the ones that can be helpful in answering our questionfor each such rule, unify the question with the consequence of that rule, getting a set of bindings (“pending knowledge”); subsequently, for each condition of the considered rule, solve it recursively, augmenting the knowledgeThe second part is actually a bit tricky: when we invoke solutions recursively, we get a (potentially infinite) sequence of bindings, which represent alternative conclusions (or “knowledges”, if you please). For example, suppose that we have a rule with three conditions. Unification with the consequence of that rule produces one initial candidate for solution (one set of bindings). Suppose that applying this candidate recursively to the first condition gives us two updated candidates for solutions. So then, if we want to apply the second condition, we need to apply it to both new candidates. As a result, we’ll get two lists of candidates for solutions. Before applying them to the third condition, we need to merge them (or “append”, if it rings the bell) into a single list.It could be tempting to use the append function that we’ve defined before. However, it won't work, because it is only capable of working with lists, and not lazy streams. But there is also a more subtle issue here: imagine that you have two streams, and you want to combine them. They are both potentially infinite, so if you want to be able to access elements of each, you cannot “first enumerate all the elements of the first stream, and then all the elements of the second”, because you'll never be done with enumerating the first stream (it's infinite!).What you need to do instead is interleave the elements from both streams.This is how it could be done:(define interleave (function (stream-a stream-b) (if (eq? stream-a '()) stream-b (if (pair? stream-a) (cons (head stream-a) (interleave (tail stream-a) stream-b)) (function () (interleave stream-b (stream-a))))))) The interleave function isn’t the whole story, though. Suppose that, having some set of partial solutions, we wish to expand them further, i.e. apply them recursively and merge (interleave) the results. Of course, if the set is empty, we have nothing left to do (and hence also return an empty set).Otherwise the set can either be a stream or a list. If it is a list, we interleave the result of applying the function to the first element of the list with the expansion of the function on the tail of the list.I admit that I don’t fully understand why streams are handled the way they are here, but the idea here is that we return a new stream which — when evaluated — produces the expansion of the elements of the original stream:(define expand (function (f elements) (if (eq? elements '()) '() (if (pair? elements) (interleave (f (head elements)) (expand f (tail elements))) (function () (expand f (elements))))))) (If you’re somewhat familiar with functional programming, you may think of expand as a variant of flat-map function. If you’re not, suppose that you have a function f that takes a natural number and returns a list with that number repeated that number times, for example (f 0) produces (), (f 1) — (1), (f 2) — (2 2), (f 3) — (3 3 3) and so on. Then, (flat-map f ‘(1 2 3)) would produce the list (1 2 2 3 3 3). The expand function is similar, but it can operate on infinite streams and the order of the elements in the resulting list can be thought of as “unspecified”.)We now know (hopefully) how to merge the results of a recursive call on a condition of a rule to update those results. We’re going to repeat this process for all conditions of a rule.This pattern of repeating some operation over some set of data is very common in programming, even though in this presentation it may seem as appearing out of the blue. Some programmers call it “reduction” or “folding”. Speaking algebraically, if [math]\circ[/math] is a binary function, then[math]fold \circ, [x_1,x_2,…,x_n] = x_1 \circ x_2 \circ … \circ x_n[/math].The problem with this formulation is that it does not specify the order in which the [math]\circ[/math] function is applied, and that the value for empty list is unspecified. We could be more specific about the order of operations — the two most obvious candidates are “left-to-right” and “right-to-left”. We can also add additional argument to serve as the default value for the empty list. This leaves us with the following formulations:[math]fold_{left} \circ, e, [x_1,x_2,…,x_n] = (…((e \circ x_1) \circ x_2)\circ … \circ x_n)[/math][math]fold_{right} \circ, e, [x_1,x_2,…,x_n] = (x_1 \circ (x_2 \circ … \circ (x_n \circ e)…))[/math]Here I’m only going to define the left-to-right variant of [math]fold[/math], although the order in which we’ll be processing the conditions for rules shouldn’t actually matter (if you want, you can try to define the right-to-left variant as an exercise):(define fold-left (function (op e l) (if (pair? l) (fold-left op (op e (head l)) (tail l)) e))) If you're familiar with some popular programming languages like Python, you can think of(fold-left f init items) as an equivalent of a simple for loop wit the following structure:def fold_left(f, init, items): for x in items: init = f(init, x) return init (if you don't have any experience with programming languages like this, you can safely ignore this remark)In our logic engine, we’re also going to need to be able to consider only those rules, whose consequence matches the query being processed. Selecting only those elements of a list which satisfies some desired criterion is also a very common pattern in programming:(define only (function (satisfying? elements) (if (pair? elements) (if (satisfying? (head elements)) (cons (head elements) (only satisfying? (tail elements))) (only satisfying? (tail elements))) '()))) Reading those definitions may not be a very pleasant experience for you. It can give you some insight into the process of programming, though. Probably every advanced programmer would be able to write these definitions by heart. They may seem very abstract, but they are also very convenient. You can’t deduce their convenience from the definitions alone, though, which is why it is often more instructive to see examples.So let’s suppose that even? is a function which evaluates to true if its argument is an even number (divisible by 2), and to false otherwise. Even if you didn’t understand the definition of only, you could probably guess that(only even? '(1 2 3 4 5 6)) produces the list (2 4 6).(Good programmers make a habit of inventing such names for things that facilitate making good guesses. The traditional name for only is filter, but — other than being traditional — it's a rather bad name. The name map used before is also traditional, and it’s also a bad name, but I didn't manage to come up with anything better so far. Maybe you will.)Before we move on to the actual implementation of our logic engine, there’s still one issue waiting to be resolved, namely — that of the identity of holes.Suppose that we have the following rule:(conclude (same X X)) Here there are two instances of the X variable in the rule’s consequence — and clearly they are meant to refer to the same object.If we consider another rule,(conclude (green X) from (plant X)) then the X variable from the conclusion is meant to be the same as the one in the condition. But we don’t want this variable to be (in principle) the same as the one used in the previous rule. Even though in both cases we used the name X, we could have used, say variable X1 in the first rule, and variable X2 in the second rule (ore something like that).Moreover, if — during our question answering process — we’ll need to use the same rule more than one time, then we don’t want the same variable to bind two different objects (because that wouldn’t unify!).For this reason, prior to applying a rule, we want to rename all the variables that appear within that rule, using a name which is guaranteed to be unique. For this purpose, let’s assume that we have a primitive function — fresh-symbol — which, each time it’s evaluated, would generate a new symbol.(Actually calling such thing “a function” might be considered an abuse by some. We consider it “primitive”, because we wouldn’t be able to define such operation using the LISP language that we’ve described before.)In order to guarantee uniqueness, we might restrict our language, for example, to forbid identifiers which contain the ~ character in variable names. Then, subsequent invocations of, say, (fresh-symbol ‘x) could produce values like x~1, x~2, x~3 and so on.If we want to preserve the identity of symbols, we must make sure that all instances of a particular symbol are substituted with the same fresh symbol. To do so, we can apply the following procedure:extract all the variables that appear within a rulegenerate fresh names for each of those variablessubstitute variables with fresh names throughout the ruleTo be able to extract variables, we need to be able to treat lists as sets, rather than sequences.A set is something that either has some element, or hasn't. That's all that a set is. For example, the lists (a b c) and (c a b) can represent the same set. Of course, the essential relation is that of having an element.An empty set does not have any element. A non-empty set has some particular element either if that element is its head, or if it is in its tail:(define in? (function (element set) (and (pair? set) (or (eq? element (head set)) (in? element (tail set)))))) (compare it with the definition of bound?)Given two sets, you can construct their union (a set containing all the elements from both these sets) and their intersection (a set containing elements that are in both these sets). For our purpose, the union operation should be sufficient (you can think how you'd implement intersection by yourself).The code for calculating union is very similar to append. The only difference is that, before appending, we check whether a particular element is already in the list being appended to:(define union (function (a b) (if (pair? a) (if (in? (head a) b) (union (tail a) b) (cons (head a) (union (tail a) b))) b))) Equipped with union, we can extract variables from an expression rather easily — recall that in our system a variable is a symbol that is not quoted:(define variables (function (expression) (if (symbol? expression) (list expression) (if (or (not (pair? expression)) (is? expression 'quote)) '() (union (variables (head expression)) (variables (tail expression))) )))) In order to reuse functions that operate on bindings, such as lookup or bound?, we can generate fresh names wrapped in our familiar binding structure (list of pairs):(define fresh-names (function (variables) (map (function (variable) (cons variable (fresh-symbol variable))) variables))) This makes variable renaming rather straightforward:(define substitute (function (expression bindings) (if (and (symbol? expression) (bound? expression bindings)) (lookup expression bindings) (if (or (is? expression 'quote) (not (pair? expression))) expression (cons (substitute (head expression) bindings) (substitute (tail expression) bindings)))))) Making variables that appear in rules independent from other variables appearing in other rules (or other instances of the same rule) is now rather easy:(define independent (function (rule) (substitute rule (fresh-names (variables rule))))) Now that we have all the pieces of the puzzle (or, as I prefer to think, sufficiently elaborate vocabulary), we can put them together.Let’s recall what we wrote above:The actual work is delegated to the solutions function. It needs to do the following:starting with empty knowledge, search through the rules to find the ones that can be helpful in answering our questionfor each such rule, unify the question with the consequence of that rule, getting a set of bindings (“pending knowledge”); subsequently, for each condition of the considered rule, solve it recursively, augmenting the knowledgeIf your impression is that we’re repeating the same thing over and over again, you’re absolutely right. Programming requires focus, and to attain focus, programmers need to recall themselves what they are actually doing. Moreover, programming is repeating the same thing over and over again, albeit in a different language.So let’s now try to translate this logic using the notions that we managed to elaborate:(define solutions* (function (query rules knowledge) (if (eq? knowledge #false) '() (expand (function (rule) (fold-left (function (knowledges condition) (expand (function (knowledge) (function () (solutions* condition rules knowledge))) knowledges)) (list (unify query (head rule) knowledge)) (tail rule))) (map independent (only (function (rule) (unify query (independent (head rule)) knowledge)) rules)))))) Admittedly, this code can be a bit difficult to read — the things that appear last should actually be read first (call of the only function). This is not how I would normally write this code.But it’s not how the code is written that is the main source of difficulty. It takes time to get familiar with functions like fold; the way of composing recursive solutions also requires getting used to. I made a lot of mistakes on the way of arriving at this function. I have been testing it on my computer along the way, and I eventually made it work.I believe that similifying this one function, and understanding its different aspects, could form a whole research field on its own, so don’t feel intimidated if you find it difficult to understand.The form of the results from the solutions* function is far from satisfactory, though, and can be simplified rather easily. We can see this if we run it for some example data:(take 1 (solutions* '('appending P Q (1 2 3 4)) '((('appending () Y Y)) (('appending (X . T) Y (X . L)) ('appending T Y L))) '())) The results are rather cryptic:(((Y~52 . (1 2 3 4)) (Q . Y~52) (P . ()))) We see that a part of our result is some transient symbol Y~52, even though we only asked for P and Q. Therefore we need to process the result further, and extract the final values from the chain of bindings that were constructed during repeated unification.Our extraction routine must account for the case when some unified object is a list containing some variables. In such case we want to extract final value of some variable (if there are cyclic references in bindings, we’re screwed). A value is final when it is not bound:(define extract (function (value bindings) (if (and (symbol? value) (bound? value bindings)) (extract (lookup value bindings) bindings) (if (pair? value) (cons (extract (head value) bindings) (extract (tail value) bindings)) value)))) In our final result, we’re only interested in the values of variables that were present in the original query. So we can wrap the call to solutions* to perform such extraction:(define solutions (function (query rules) ($map (lambda (result) (map (lambda (variable) (cons variable (extract variable result))) (variables query))) (solutions* query rules '())))) where $map is a variant of map which is able to work with streams:(define $map (function (f $) (if (eq? $ '()) '() (if (pair? $) (cons (f (head $)) ($map f (tail $))) (function () ($map f ($))))))) Now the result is much easier to decode:(take 5 (solutions '('appending P Q (1 2 3 4)) '((('appending () Y Y)) (('appending (X . T) Y (X . L)) ('appending T Y L))))) produces(((P . ()) (Q . (1 2 3 4))) ((P . (1)) (Q . (2 3 4))) ((P . (1 2)) (Q . (3 4))) ((P . (1 2 3)) (Q . (4))) ((P . (1 2 3 4)) (Q . ()))) This gives us all the possible arguments to the append function that would produce the list (1 2 3 4). From the perspective of LISP, this means that we managed to reverse the order of execution — rather than calculating the result, we treat the result as given and “run our program in the opposite direction”.But the possibilities of our language are even bigger: we can write queries like:(some 3 (appending x y z)) which could be interpreted as: “what are some possible relations between argments to appending and its result?”.The detective can respond to this query in the following way:(((x . ()) (y . b~49) (z . b~49)) ((x . (h~50)) (y . b~102) (z . (h~50 . b~102))) ((x . (h~50 h~103)) (y . b~155) (z . (h~50 h~103 . b~155)))) As you can see, there are some weird symbols appearing in the result, like b~49 or h~103. These symbols aren’t quoted, so they are variables. They stand for any value, so in a sense, such result represents infinite amount of results. Some people say that these values are unreified (because “to reify” means “to make a thing out of something”, from the Latin word “re”, which means “a thing”). You should be impressed that our language is capable of expressing such concept.Running the evaluator backwards — the first attemptBy now we should have a fairly good understanding of what an evaluator is, and what it means to run a function backwards. It should therefore be fairly straightforward to guess what the phrase “running the evaluator backwards” might mean: just as we managed to translate the append function so that it became understandable to our “detective”, we are now also going to try to translate the value function and its friends in a similar fashion.Now, from the course of my presentation this might seem like an obvious idea. As I wrote earlier, the evaluator was invented in the late 1950s (if we discount Turing’s ideas). The “detective-style programming” was developed in the early 1970s. Both ideas were very well known to programmers who were dealing with the field called “Artificial Intelligence” — which is a field that tends to attract great minds. They were both presented in MIT’s introductory course to Computer Science for almost three decades since the mid 1980s.Yet — to my knowledge — it wasnt’t until the second decade of the XXI century that someone (namely: Daniel Friedman and William Byrd) actually merged those two ideas to obtain a working program and started exploring its consequences (the first presentation of this idea that I know appeared around 2013).Let's recall the code of our evaluator.Its heart consisted of the value function, which dispatched on the possible type of the expression being evaluated (to make the code simpler, I removed the parts responsible for processing the and and or forms):(define value (function (exp env) (if (symbol? exp) (value (lookup exp env) env) (if (is? exp 'quote) (second exp) (if (is? exp 'if) (if (value (second exp) env) (value (third exp) env) (value (fourth exp) env)) (if (is? exp 'function) exp (if (pair? exp) (applied (value (head exp) env) (map (function (arg) (value arg env)) (tail exp)) env) exp))))))) We'd like to translate this program to our ‘detective language’. However, you can observe a few problems with this endaevour. First, it uses the symbol? function, which cannot be expressed in the detective language, which only allows us to pattern-match, either on literals (like particular symbols or numbers) or on structures. So while we can easily convert the transformation of quote, if and function, namely(conclude (valuation ('quote x) env x)) (conclude (evaluates ('if test then else) env result) from (evaluates test env #true) (evaluates then env result)) (conclude (evaluates ('if test then else) env result) from (evaluates test env #false) (evaluates else env result)) (conclude (evaluates ('function args . body) env ('function args . body))) there is no way for us write a rule that would only apply if a given argument was only a symbol.A similar problem concerns the application. While we can emulate the pair? function in our language rather easily (by unifying an object with a (head . tail) pattern), this is insufficient for our purpose: the rule for application doesn’t only check whether a given expression is a pair, but it does so in the circumstances which rule out the head of that pair being any of the symbols quote, if and function (as well as and and or in the original evaluator).But, while we can write rules that are satisfied if a given subexpression is a particular symbol, we have no way of saying that a rule should be satisfied if a given variable is not a particular symbol (or set of symbols).The art of saying “no”It would therefore be desirable to extend our language with a capability for expressing such restrictions, so that we would be able to write down our rule as:(conclude (valuation (operator . operands) env result) from (valuation operator env procedure) (valuations operands env arguments) (application procedure arguments env result) (differ operator 'quote) (differ operator 'function) (differ operator 'if)) where differ is a special predicate which succeeds only if its arguments do not unify.Likewise, we would like to be able to verify whether a given argument is a symbol, regardless of its actual value:(conclude (valuation variable ((variable . value) . _) value) from (symbol variable)) (conclude (valuation variable ((another-variable . _) . env) value) from (valuation variable env value) (symbol variable) (differ variable another-variable)) Let’s now think for a minute how we could implement such extension.We would like to be able to extend our set of rules with a bunch of “magic rules” like differ or symbol. These rules would either succeed or fail (or they could be unresolved in that we could have insufficient information to know whether they succeed or fail).It should be easy to see that there are actually two questions here:How should the general mechanism for writing such magic rules be organized?How should the particular magic rules (i.e. differ and symbol) be implemented?Let’s begin with the first question. We’re going to need to modify the solutions* and its representation of knowledge: so far, we have only been using bindings, but now we’d also like to include a list of constraints.The most straightforward way of doing that is to store a pair whose head is a list of bindings, and whose tail is a list of constraints.Technically, we could just use the cons function to construct that pair. However, this would obscure our intent. Moreover, if bindings are #false, there's no need to construct the pair, because there are no values to be constrained. So, instead of cons, we're going to use the make-knowledge function:(define make-knowledge (function (bindings constraints) (if (eq? bindings #false) #false (cons bindings constraints)))) Accordingly, we need to be able to access both bindings and constraints from our knowledge representation:(define knowledge-bindings (function (knowledge) (if (eq? knowledge #false) #false (head knowledge)))) (define knowledge-constraints (function (knowledge) (if (eq? knowledge #false) #false (tail knowledge)))) Of course, it could be the case that some piece of knowledge (or rather hypothesis) is inconsistent, in the sense that the bindings do not satisfy some of the constraints, and therefore we’d like to be able to check for this consistency.As we said before, a constraint is a function whose value could either be #true, which would mean that a constraint is satisfied, #false, which means that a constraint is violated, or some other value, which means that we don’t yet have sufficient information to decide.The last case is easy to handle — we just keep the constraint intact. If a constraint is violated, then we can immediately say that our hypothesis is false. But if the result is #true, then we know that the constraint is satisfied, and therefore we can remove it from our set of constraints.Therefore, we need a function that takes a constraint, the outcome of applying this constraint on a given set of bindings, and the pair expressing the knowledge being verified. Then, the above logic could be translated as:(define verified (function (constraint outcome knowledge) (if (eq? outcome #false) #false (if (eq? outcome #true) (make-knowledge (knowledge-bindings knowledge) (only (function (c) (not (eq? c constraint))) knowledge)) knowledge)))) This allows us check for consistency of knowledge in the following way:(define consistent (function (knowledge) (fold-left (function (knowledge constraint) (if (eq? knowledge #false) #false (verified constraint (satisified knowledge constraint) knowledge))) knowledge (knowledge-bindings knowledge)))) where the satisfied function applies the constraint to knowledge. Its exact definition would depend on how we decide to represent constraints.Let’s recall that we have decided to represent regular rules as a list of predicates (with quoted heads), for example(('appending () Y Y)) or(('appending (X . T) Y (X . L)) ('appending T Y L)) or in general,(<consequence> <conditions> ...) where <conditions> is a (possibly empty) list.We could represent constraints as pairs:(<consequence> . <verifier>) where <verifier> is a LISP function that verifies the constraint. It is safe to assume that it takes two arguments: the <consequence> form, which allows to specify which arguments are of concern to us, and a set of bindings that are meant to be tested against the constraint.Given this representation, the satisfied function should just take the <verifier> and apply it to the <consequence> and to the bindings from a given knowledge:(define satisfied (function (constraint knowledge) ((tail constraint) (head constraint) (knowledge-bindings knowledge)))) (If you find this definition puzzling, you should give it a thought. However, it might be helpful to read on, until some actual definitions of constraints appear — it’s usually easier to think about concrete examples than abstract definitions.)Now that we have an idea how to represent and use constraints, we need to integrate this idea into our inference engine.Let’s recall the definition of the solutions* function:(define solutions* (function (query rules knowledge) (if (eq? knowledge #false) '() (expand (function (rule) (fold-left (function (knowledges condition) (expand (function (knowledge) (function () (solutions* condition rules knowledge))) knowledges)) (list (unify query (head rule) knowledge)) (tail rule))) (map independent (only (function (rule) (unify query (independent (head rule)) knowledge)) rules)))))) We’re going to modify it to account for constraints (the modified version will be called solutions&, because the & character looks like a bond, which is something that constraints).There will be the following differences:we need to treat magic rules/constraints differently than regular rulesupon recursive call, we want to filter out all the solutions that are inconsistentin many places where we used knowledge before (which previously meant “bindings”), we’re now going to use (knowledge-bindings knowledge) or something even more complicated, and where we have returned bindings, we’re going to need to make-knowledgeThere’s one remark concerning the “filtering-out” (p. 2). The list of answers obtained from the recursive call is potentially infinite, so we’d need a variant of the only function that would be able to operate on streams. However, even the only function wouldn’t be satisfactory to us, because the consistent function might remove some of the constraints (the ones for which the verifier function returned #true). Therefore we need to combine the capabilities of the only function and the map function, as well as the ability to operate on infinite streams.It turns out that we already have such function — we have called it expand. The only difference is that it doesn’t accept a function that could return #false, but it accepts functions that produce lists. Therefore, we could take the result of the consistent function, and if it’s #false, return the empty list, and otherwise return a single-element list containing the result:(define listed (function (value) (if (eq? value #false) '() (list value)))) So, here’s the definition of solutions&:(define solutions& (function (query rules knowledge) (if (not knowledge) '() (expand (function (rule) (if (procedure? (tail rule)) (constrained query knowledge rule) (fold-left (function (knowledges condition) (expand (function (knowledge) (lambda () (solutions& condition rules knowledge))) knowledges)) (listed (consistent (make-knowledge (unify query (head rule) (knowledge-bindings knowledge)) (knowledge-constraints knowledge)))) (tail rule)))) (map independent (only (function (rule) (unify query (independent (head rule)) (knowledge-bindings knowledge))) rules)))))) As in the case of solutions*, I arrived at this definition by the way of trial and error. I’d like to turn your attention to the following subexpression: (if (procedure? (tail rule)) (constrained query knowledge rule) (fold-left ...) It replaces the direct call to fold-left from solutions*. The constrained function is defined as:(define constrained (function (query knowledge rule) (consistent (make-knowledge (unify query (head rule) (knowledge-bindings knowledge)) (cons rule (knowledge-constraints knowledge)))))) which I hope is at this point straight-forward enough that I don’t need to explain it.Now that we have some idea about the general mechanism of using constraints, we can answer the second question that we asked at the beginning of this section, namely — how to implement the differ and symbol constraints.Let’s begin with symbol. The rule itself could be obtained from evaluation of the expression:(cons '('symbol X) (function (pattern bindings) ???)) where the ??? is to be filled by us shortly.The pattern argument is going to have a value like ('symbol X~567) during function application. Of course, the 'symbol element isn't particularly interesting to us, and we care more about the second element of pattern.We should obtain its value (using the extract function that we wrote before) and see if it is:a symbol (variable), which means that the value is not reified yet, so we can’t decide whether the constraint is satisfied or violateda list of two elements, whose first element is the quote symbol, and whose second element is a symbol, which means that the constraint is satisfiedanything else means that the constraint is violatedTranslating this logic to Lisp, we could write it as:(define check-symbol (function (value) (if (symbol? value) 'unknown (if (and (pair? value) (pair? (tail value)) (eq? (tail (tail value)) '()) (eq? (head value) 'quote)) #true #false)))) This definition allows us to complete our constraint:(cons '('symbol X) (function (pattern bindings) (check-symbol (extract (second pattern) bindings)))) The implementation of disequality constraint is going to have a similar form. The rule for our constraint might be obtained from evaluation of the expression(cons '('differ X Y) (function (pattern bindings) ???)) It should be easy to see that this time we’ll be interested in the second and the third element of pattern.However, the way we are going to check for equality of those elements may not be obvious at first: we’re going to use the unify function, but this time, we’re going to interpret the results differently:if the patterns fail to unify, then it means that they cannot be made equal under any circumstances, which means that the constraint is certainly satisfiedif the patterns unify, then the interpretation depends on the result:if the bindings resulting from the application of unify are exactly the same as the bindings passed to that function, then it means that the given bindings already violate the constraintotherwise, if they are different, it means that there are some extra conditions that would need to be satisfied in order to violate the constraint.Translating this logic to Lisp, we get:(define check-disequality (function (unified bindings) (if (eq? unified #false) #true (if (eq? unified bindings) #false 'unknown)))) So, we could write down the disequality constraint rule as:(cons '('differ X Y) (function (pattern bindings) (check-disequality (unify (second pattern) (third pattern) bindings)))) It should make sense to gather these rules in one place, and pass it to the answer function instead of empty assumptions:(define fundamental-assumptions (list (cons '('symbol X) (function (pattern bindings) (check-symbol (extract (second pattern) bindings)))) (cons '('differ X Y) (function (pattern bindings) (check-disequality (unify (second pattern) (third pattern) bindings)))))) We also need to adapt our interface, i.e. the solutions function, to be able to process our new representation of knowledge. It is a relatively simple task, even though the resulting code is rather lengthy — instead of a list of bindings, the result is now a list of pairs of bindings and constraints:(define solutions (function (query rules) ($map (lambda (result) (cons (map (lambda (variable) (cons variable (extract variable (knowledge-bindings result)))) (variables query)) (map (lambda (constraint) (cons (second (head (head constraint))) (map (lambda (variable) (extract variable (knowledge-bindings result))) (tail (head constraint))))) (knowledge-constraints result)))) (solutions& query rules (make-knowledge '() '()))))) I admit that this function is barely readable in this form. This isn’t very important from our point of view, because it is only responsible for displaying the results. (Perhaps it could be rewritten to a more digestible form.)We can test the result using some sample rules:(answer '((conclude (distinct-symbols X Y) from (differ X Y) (symbol X) (symbol Y)) (some 1 (distinct-symbols A B))) fundamental-assumptions) produces ((((A . X~215) (B . X~221)) (symbol X~221) (symbol X~215) (differ X~215 X~221))), whereas(answer '((conclude (distinct-symbols X Y) from (differ X Y) (symbol X) (symbol Y)) (some 1 (distinct-symbols 'A B))) fundamental-assumptions) yields ((((B . X~247)) (symbol X~247) (differ 'A X~247))), but(answer '((conclude (distinct-symbols X Y) from (differ X Y) (symbol X) (symbol Y)) (some 1 (distinct-symbols 'A 'B))) fundamental-assumptions) gives ((())), and(answer '((conclude (distinct-symbols X Y) from (differ X Y) (symbol X) (symbol Y)) (some 1 (distinct-symbols A A))) fundamental-assumptions) produces ().We have only defined two types of constraints, but it may turn out that we’re going to need some more. We are prepared for this situation, though, because our framework is flexible enough to allow us to define new constraints (even though they are extra-linguistic from the point of view of our detective).Running the evaluator backwards — the second attemptNow that our language is advanced enough to express various constraints, translating the meta-circular evaluator to our detective language should be a relatively straightforward task.Let’s begin by translating the run function. Our counterpart will be called reduces:(conclude (reduces (('define name value). rest) env result) from (reduces rest ((name . value) . env) result)) (conclude (reduces (last-expression) env result) from (evaluates last-expression env result)) The core of the evaluator is the evaluates function, which corresponds to the value function. We need to transform all possible types of expressions that we might have to deal with, i.e. quote, if, function, variables, function application and literals.The case of quote is very simple — we simply give back the quoted term:(conclude (evaluates ('quote literal) env literal)) The case of if is slightly more complicated: we need to evaluate the test of an expression, and if it evaluates to #false, then produce the value of the “else” branch, and otherwise — the value of the “then” branch:(conclude (evaluates ('if test then else) env result) from (evaluates test env #false) (evaluates else env result)) (conclude (evaluates ('if test then else) env result) from (evaluates test env test-result) (differ test-result #false) (evaluates then env result)) Functions are self-evaluating:(conclude (evaluates ('function args . body) env ('function args . body))) In case of variables, we need to look up the value of the variable in the environment:(conclude (evaluates key ((key . value) . _) value) from (symbol key)) (conclude (evaluates key ((other-key . _) . env) value) from (symbol key) (symbol other-key) (differ key other-key) (evaluates key env value)) The case of function application is a bit tricky: we need to evaluate operator and operands recursively and then apply the resulting function to the resulting arguments. But, we must make sure that operator is neither quote nor function nor if:(conclude (evaluates (operator . operands) env result) from (differ operator 'quote) (differ operator 'function) (differ operator 'if) (evaluates operator env function) (evaluate operands env arguments) (application function arguments env result)) where evaluate produces a list of evaluated arguments(conclude (evaluate (first . rest) env (first* . rest*)) from (evaluates first env first*) (evaluate rest env rest*)) (conclude (evaluate () env ())) and application extends the environment with the appropriate values and reduces the given sub-program:(conclude (application ('function (arg . args) . body) (val . vals) env result) from (application ('function args . body) vals ((arg . val) . env) result)) (conclude (application ('function () . body) () env result) from (reduces body env result)) (conclude (application ('function last-arg . body) vals env result) from (symbol last-arg) (reduces body ((last-arg . vals) . env) result)) Literals (like numbers) are self-evaluating, so they could be handled using the following rule:(conclude (evaluates value env value) from (literal value)) which would of course add another type of constraints that would hold if a given object is a literal value.So that’s it. This is how “the most impressive code I’ve seen” looks like. To be more specific, this is my attempt to rewrite this code. If you’re not sure how you’re supposed to feel after reading it, here’s a hint:Why’s that important?While the above code doesn’t itself offer too many capabilities, it could be extended (for example, with operations like cons, head and tail, or some operations on numbers) that would allow to generate programs which give some particular results, or have some particular properties.So, instead of using computers to execute our programs, we can use them to generate some programs that have some desired properties.I’ve learned about this technique from Dan Friedman and Will Byrd, who presented it in 2012 during a Clojure/Conj conference. The recorded talk is available on Youtube (you should watch at least the first 20 minutes to see the potential of that idea):Will Byrd has applied this technique to develop an experimental editor that is able to synthesize programs from some hints that programmers give to it, as he explained in this talk:The SummaryThe philosopher Ludwig Wittgenstein said once:the limits of my language mean the limits of my worldWhat I like about the example that I have presented above is that it shows the “perennial value” of Lisp, which makes it very easy to design new languages (like “the detective language”), which in turn is an amplifier for the mind that allows you to shift the limits of your world.
In C or C++, what are your favorite pointer tricks?
I’ll start with an important warning about aliasing, then cover my three favorite pointer tricks, which are the double pointer trick for linked list traversal, finding the length of an array without sizeof and division, and the Linux kernel’s container_of macro.Strict aliasing rulesBefore you start using pointer tricks, you should be sure to understand the language’s aliasing rules. From the C99 standard §6.5, Expressions:7. An object shall have its stored value accessed only by an lvalue expression that has one of the following types:a type compatible with the effective type of the object,a qualified version of a type compatible with the effective type of the object,a type that is the signed or unsigned type corresponding to the effective type of the object,a type that is the signed or unsigned type corresponding to a qualified version of the effective type of the object,an aggregate or union type that includes one of the aforementioned types among its members (including, recursively, a member of a subaggregate or contained union), ora character type.Historically, you could get away with violating these rules, and programmers would use pointer casting to access representations of the same data using different types. But modern optimizing compilers will often break that kind of code, because the rules allow them to assume that pointers of different types do not point to the same region of memory!See the GCC documentation and Understanding Strict Aliasing.Linked list manipulation with double pointersConsider the problem of inserting a number into a sorted linked singly-linked list.struct node { int data; struct node *next; } *head; A typical solution has two pointers and a few special cases to get right:void insert(struct node *new_node) { struct node *node = head, *prev = NULL; while (node != NULL && node->data < new_node->data) { prev = node; node = node->next; } new_node->next = node; if (prev == NULL) head = new_node; else prev->next = new_node; } But with clever use of a pointer to a pointer, you can do this with no extra cases:void insert(struct node *new_node) { struct node **link = &head; while (*link != NULL && (*link)->data < new_node->data) link = &(*link)->next; new_node->next = *link; *link = new_node; } The same trick applies to node deletion. It’s also useful to store the previous links this way in non-circular doubly-linked list implementations, such as the Linux kernel’s hlist (types, operations).(Of course, in C++, you don’t need to write your own linked lists, since the STL provides std::list and std::slist.)How long is that array?It’s typical to see loops likeint arr[] = {1, 1, 2, 3, 5, 8, 13}; for (int i = 0; i < sizeof(arr)/sizeof(arr[0]); i++) printf("%d\n", arr[i]); That sizeof nonsense is ugly, and it’s often hidden behind a macro. But we can simplify it using pointer arithmetic.&arr is a pointer to the array. It points at the same memory address as arr—which decays to a pointer to its first element—but with a different type, whose size is that of the entire array rather than of just one element.That means that &arr + 1 points at the address after the end of the array.Dereferencing to *(&arr + 1) gives the address after the end of the last element (which is again the same address with a different type).Finally, we can subtract the pointer to the first element to get the length of the array: *(&arr + 1) - arr.The equivalence between array indexing and pointer arithmetic simplifies this to (&arr)[1] - arr == 1[&arr] - arr, saving a pair of parens. This might be that equivalence’s only practical use outside the International Obfuscated C Code Contest.for (int i = 0; i < 1[&arr] - arr; i++) printf("%d\n", arr[i]); Having done that, it’s even simpler to make the iteration variable a pointer too:for (int *p = arr; p < 1[&arr]; p++) printf("%d\n", *p); (C++11 provides the wonderful new for (int n : arr) syntax for this.)The container_of macroThe Linux kernel source includes this useful macro that’s invaluable for writing object-oriented code in C. I’ve simplified its definition to remove GCC-specific extensions:#define container_of(ptr, type, member) \ (type *)((char *)(ptr) - offsetof(type, member)) It’s used as follows (example taken from linux/Documentation/kobject.txt). Imagine you have a structure containing another structure:struct uio_map { struct kobject kobj; struct uio_mem *mem; }; Then, given a struct kobject that you know is a member of a struct uio_map, you might be tempted to get the outer struct uio_map just by casting the pointer:struct kobject *kp = …; struct uio_map *u_map = (struct uio_map *)kp; But that leads to fragile code that would be broken by the addition of another member to the beginning of struct uio_map. Instead, write:struct uio_map *u_map = container_of(kp, struct uio_map, kobj);
- Home >
- Catalog >
- Miscellaneous >
- Checklist Template >
- Baby Shower Checklist >
- baby shower planning guide >
- Practice 2 3 Simplifying Variable Expression