The Guide of modifying Umass W 9 Online
If you take an interest in Modify and create a Umass W 9, heare are the steps you need to follow:
- Hit the "Get Form" Button on this page.
- Wait in a petient way for the upload of your Umass W 9.
- You can erase, text, sign or highlight as what you want.
- Click "Download" to download the changes.
A Revolutionary Tool to Edit and Create Umass W 9
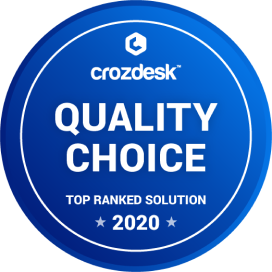
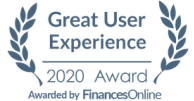
How to Easily Edit Umass W 9 Online
CocoDoc has made it easier for people to Modify their important documents by online website. They can easily Fill through their choices. To know the process of editing PDF document or application across the online platform, you need to follow these steps:
- Open the website of CocoDoc on their device's browser.
- Hit "Edit PDF Online" button and Upload the PDF file from the device without even logging in through an account.
- Edit your PDF file by using this toolbar.
- Once done, they can save the document from the platform.
Once the document is edited using the online platform, the user can export the form according to your ideas. CocoDoc provides a highly secure network environment for consummating the PDF documents.
How to Edit and Download Umass W 9 on Windows
Windows users are very common throughout the world. They have met millions of applications that have offered them services in managing PDF documents. However, they have always missed an important feature within these applications. CocoDoc wants to provide Windows users the ultimate experience of editing their documents across their online interface.
The procedure of editing a PDF document with CocoDoc is easy. You need to follow these steps.
- Select and Install CocoDoc from your Windows Store.
- Open the software to Select the PDF file from your Windows device and continue editing the document.
- Modify the PDF file with the appropriate toolkit appeared at CocoDoc.
- Over completion, Hit "Download" to conserve the changes.
A Guide of Editing Umass W 9 on Mac
CocoDoc has brought an impressive solution for people who own a Mac. It has allowed them to have their documents edited quickly. Mac users can fill PDF form with the help of the online platform provided by CocoDoc.
For understanding the process of editing document with CocoDoc, you should look across the steps presented as follows:
- Install CocoDoc on you Mac to get started.
- Once the tool is opened, the user can upload their PDF file from the Mac in minutes.
- Drag and Drop the file, or choose file by mouse-clicking "Choose File" button and start editing.
- save the file on your device.
Mac users can export their resulting files in various ways. Not only downloading and adding to cloud storage, but also sharing via email are also allowed by using CocoDoc.. They are provided with the opportunity of editting file through multiple ways without downloading any tool within their device.
A Guide of Editing Umass W 9 on G Suite
Google Workplace is a powerful platform that has connected officials of a single workplace in a unique manner. While allowing users to share file across the platform, they are interconnected in covering all major tasks that can be carried out within a physical workplace.
follow the steps to eidt Umass W 9 on G Suite
- move toward Google Workspace Marketplace and Install CocoDoc add-on.
- Upload the file and Push "Open with" in Google Drive.
- Moving forward to edit the document with the CocoDoc present in the PDF editing window.
- When the file is edited at last, share it through the platform.
PDF Editor FAQ
What colleges have you applied and been accepted into?
Answering as a competitive, but not top level student since I feel a lot of answers are top, top level, and I wanted to document my own journey, and show that you can go to a great school even if you aren’t top of your class!Background: I am from the south and I desperately did not want to go to college in the south. It’s just not my place. Luckily, my parents were very supportive of this, and helped me visit out of state and research colleges with me. I applied to the local public university as a safety, got in, but I had no intention of going so I’m not including it here.Stats: 3.5 UW, 4.1 W, 9 AP classes (One 3, rest 4s and 5s), top 25% of my class (large, super competitive public school). I think I had good recs, and a really good essay.Accepted:Augsburg University: Recommended by my dad through his research, strong psychology program which was going to be my major but I changed my mind. Got a presidential scholarship which was cool.Clark University: One of my favorites and the tour convinced me to apply. My friend’s grandparents met there and a fantastic psych program, but like I said I changed my mind. Got the second largest scholarship.DePaul University: The first college I visited and the first I was accepted to (and got a Presidential scholarship). I was convinced for a while this was where I was gonna end up, I still view the college fondly.Emmanuel College: I loved Boston and I really liked the Fenway consortium. Large scholarship.Mills College: Really liked the idea of a women’s college. Didn’t get a huge scholarship and decided against going to a CA school, but I wish it the best, it’s a darling school.Roosevelt University: Safety Chicago school, large scholarship, but I never visited and had been accepted by DePaul already.Simmons University: Another Fenway school. Got a really large scholarship but was rejected from to honors program. (I’m forever bitter about that.)SUNY Albany: Really amazing campus (here) and good psych program. I got a scholarship and I was pretty sure I’d get in.Umass Boston: I really wanted to go to school in Boston and this was a safety + scholarship.University of San Francisco: I at first really wanted to go to school in CA, and I loved SF. This was a nice surprise to get in considering it’s competitive, but I got a Presidential scholarship too!Waitlisted, Withdrew:Occidental College: I loved the campus and it’s a great school, was expecting rejection but hey waitlisted is better. I withdrew because I didn’t want to risk past the May 1 deadline and I was pretty set on another college by then.Rejected:Smith College: I was disappointed but not surprised. I did an interview but never visited, but it was my dream reach school. My mom consoled me with “I had coworker who went there and was a b*tch, so you’re better off,” which was sweet.Final Decision:Clark University! I absolutely loved everything about this quirky little school, and am excited to start in the fall! I learned a lot through this experience and my advice is to apply where you want, but be smart about it. There were schools I had never heard of before the research, and didn’t think I was a shoe-in, that gave me Presidential scholarships. I knew someone who underestimated the selective-ness of certain top liberal arts schools, is stuck at the local public university. I also knew a lot of people who got off waitlists and attending their dream schools. It’s all a guessing game and hard to know, but please do some research! Know that Stanford isn’t going to accept you, and that there are plenty of schools out there that you can be happy going to. If you can’t see yourself going there, don’t apply. I’m so happy with my choice, but I also know if I had gotten rejected, I could’ve been happy elsewhere.Good luck class of 2024!
What's the most effective way to get started with deep learning?
Here are some resources to start learning Deep Learning:Free Online BooksDeep Learning by Yoshua Bengio, Ian Goodfellow and Aaron Courville (05/07/2015)Neural Networks and Deep Learning by Michael Nielsen (Dec 2014)Deep Learning by Microsoft Research (2013)Deep Learning Tutorial by LISA lab, University of Montreal (Jan 6 2015)neuraltalk by Andrej Karpathy : numpy-based RNN/LSTM implementationAn introduction to genetic algorithmsArtificial Intelligence: A Modern ApproachDeep Learning in Neural Networks: An OverviewCoursesMachine Learning - Stanford by Andrew Ng in Coursera (2010-2014)Machine Learning - Caltech by Yaser Abu-Mostafa (2012-2014)Machine Learning - Carnegie Mellon by Tom Mitchell (Spring 2011)Neural Networks for Machine Learning by Geoffrey Hinton in Coursera (2012)Neural networks class by Hugo Larochelle from Université de Sherbrooke (2013)Deep Learning Course by CILVR lab @ NYU (2014)A.I - Berkeley by Dan Klein and Pieter Abbeel (2013)A.I - MIT by Patrick Henry Winston (2010)Vision and learning - computers and brains by Shimon Ullman, Tomaso Poggio, Ethan Meyers @ MIT (2013)Convolutional Neural Networks for Visual Recognition - Stanford by Fei-Fei Li, Andrej Karpathy (2015)Deep Learning for Natural Language Processing - StanfordNeural Networks - usherbrookeMachine Learning - Oxford (2014-2015)Deep Learning - Nvidia (2015)Videos and LecturesHow To Create A Mind By Ray KurzweilDeep Learning, Self-Taught Learning and Unsupervised Feature Learning By Andrew NgRecent Developments in Deep Learning By Geoff HintonThe Unreasonable Effectiveness of Deep Learning by Yann LeCunDeep Learning of Representations by Yoshua bengioPrinciples of Hierarchical Temporal Memory by Jeff HawkinsMachine Learning Discussion Group - Deep Learning w/ Stanford AI Lab by Adam CoatesMaking Sense of the World with Deep Learning By Adam CoatesDemystifying Unsupervised Feature Learning By Adam CoatesVisual Perception with Deep Learning By Yann LeCunThe Next Generation of Neural Networks By Geoffrey Hinton at GoogleTechTalksThe wonderful and terrifying implications of computers that can learn By Jeremy Howard at TEDxBrusselsUnsupervised Deep Learning - Stanford by Andrew Ng in Stanford (2011)Natural Language Processing By Chris Manning in StanfordPapersImageNet Classification with Deep Convolutional Neural NetworksUsing Very Deep Autoencoders for Content Based Image RetrievalLearning Deep Architectures for AICMU’s list of papersNeural Networks for Named Entity Recognition zipTraining tricks by YBGeoff Hinton's reading list (all papers)Supervised Sequence Labelling with Recurrent Neural NetworksStatistical Language Models based on Neural NetworksTraining Recurrent Neural NetworksRecursive Deep Learning for Natural Language Processing and Computer VisionBi-directional RNNLSTMGRU - Gated Recurrent UnitGFRNN . .LSTM: A Search Space OdysseyA Critical Review of Recurrent Neural Networks for Sequence LearningVisualizing and Understanding Recurrent NetworksWojciech Zaremba, Ilya Sutskever, An Empirical Exploration of Recurrent Network ArchitecturesRecurrent Neural Network based Language ModelExtensions of Recurrent Neural Network Language ModelRecurrent Neural Network based Language Modeling in Meeting RecognitionDeep Neural Networks for Acoustic Modeling in Speech RecognitionSpeech Recognition with Deep Recurrent Neural NetworksReinforcement Learning Neural Turing MachinesLearning Phrase Representations using RNN Encoder-Decoder for Statistical Machine TranslationGoogle - Sequence to Sequence Learning with Nneural NetworksMemory NetworksPolicy Learning with Continuous Memory States for Partially Observed Robotic ControlMicrosoft - Jointly Modeling Embedding and Translation to Bridge Video and LanguageNeural Turing MachinesAsk Me Anything: Dynamic Memory Networks for Natural Language ProcessingTutorialsUFLDL Tutorial 1UFLDL Tutorial 2Deep Learning for NLP (without Magic)A Deep Learning Tutorial: From Perceptrons to Deep NetworksDeep Learning from the Bottom upTheano TutorialNeural Networks for MatlabUsing convolutional neural nets to detect facial keypoints tutorialTorch7 TutorialsThe Best Machine Learning Tutorials On The WebVGG Convolutional Neural Networks PracticalDatasetsMNIST Handwritten digitsGoogle House Numbers from street viewCIFAR-10 and CIFAR-1004.IMAGENETTiny Images 80 Million tiny images6.Flickr Data 100 Million Yahoo datasetBerkeley Segmentation Dataset 500UC Irvine Machine Learning RepositoryFlickr 8kFlickr 30kMicrosoft COCOVQAImage QAAT&T Laboratories Cambridge face databaseAVHRR PathfinderAir Freight - The Air Freight data set is a ray-traced image sequence along with ground truth segmentation based on textural characteristics. (455 images + GT, each 160x120 pixels). (Formats: PNG)Amsterdam Library of Object Images - ALOI is a color image collection of one-thousand small objects, recorded for scientific purposes. In order to capture the sensory variation in object recordings, we systematically varied viewing angle, illumination angle, and illumination color for each object, and additionally captured wide-baseline stereo images. We recorded over a hundred images of each object, yielding a total of 110,250 images for the collection. (Formats: png)Annotated face, hand, cardiac & meat images - Most images & annotations are supplemented by various ASM/AAM analyses using the AAM-API. (Formats: bmp,asf)Image Analysis and Computer GraphicsBrown University Stimuli - A variety of datasets including geons, objects, and "greebles". Good for testing recognition algorithms. (Formats: pict)CAVIAR video sequences of mall and public space behavior - 90K video frames in 90 sequences of various human activities, with XML ground truth of detection and behavior classification (Formats: MPEG2 & JPEG)Machine Vision UnitCCITT Fax standard images - 8 images (Formats: gif)CMU CIL's Stereo Data with Ground Truth - 3 sets of 11 images, including color tiff images with spectroradiometry (Formats: gif, tiff)CMU PIE Database - A database of 41,368 face images of 68 people captured under 13 poses, 43 illuminations conditions, and with 4 different expressions.CMU VASC Image Database - Images, sequences, stereo pairs (thousands of images) (Formats: Sun Rasterimage)Caltech Image Database - about 20 images - mostly top-down views of small objects and toys. (Formats: GIF)Columbia-Utrecht Reflectance and Texture Database - Texture and reflectance measurements for over 60 samples of 3D texture, observed with over 200 different combinations of viewing and illumination directions. (Formats: bmp)Computational Colour Constancy Data - A dataset oriented towards computational color constancy, but useful for computer vision in general. It includes synthetic data, camera sensor data, and over 700 images. (Formats: tiff)Computational Vision LabContent-based image retrieval database - 11 sets of color images for testing algorithms for content-based retrieval. Most sets have a description file with names of objects in each image. (Formats: jpg)Efficient Content-based Retrieval GroupDensely Sampled View Spheres - Densely sampled view spheres - upper half of the view sphere of two toy objects with 2500 images each. (Formats: tiff)Computer Science VII (Graphical Systems)Digital Embryos - Digital embryos are novel objects which may be used to develop and test object recognition systems. They have an organic appearance. (Formats: various formats are available on request)Univerity of Minnesota Vision LabEl Salvador Atlas of Gastrointestinal VideoEndoscopy - Images and Videos of his-res of studies taken from Gastrointestinal Video endoscopy. (Formats: jpg, mpg, gif)FG-NET Facial Aging Database - Database contains 1002 face images showing subjects at different ages. (Formats: jpg)FVC2000 Fingerprint Databases - FVC2000 is the First International Competition for Fingerprint Verification Algorithms. Four fingerprint databases constitute the FVC2000 benchmark (3520 fingerprints in all).Biometric Systems Lab - University of BolognaFace and Gesture images and image sequences - Several image datasets of faces and gestures that are ground truth annotated for benchmarkingGerman Fingerspelling Database - The database contains 35 gestures and consists of 1400 image sequences that contain gestures of 20 different persons recorded under non-uniform daylight lighting conditions. (Formats: mpg,jpg)Language Processing and Pattern RecognitionGroningen Natural Image Database - 4000+ 1536x1024 (16 bit) calibrated outdoor images (Formats: homebrew)ICG Testhouse sequence - 2 turntable sequences from ifferent viewing heights, 36 images each, resolution 1000x750, color (Formats: PPM)Institute of Computer Graphics and VisionIEN Image Library - 1000+ images, mostly outdoor sequences (Formats: raw, ppm)INRIA's Syntim images database - 15 color image of simple objects (Formats: gif)INRIAINRIA's Syntim stereo databases - 34 calibrated color stereo pairs (Formats: gif)Image Analysis Laboratory - Images obtained from a variety of imaging modalities -- raw CFA images, range images and a host of "medical images". (Formats: homebrew)Image Analysis LaboratoryImage Database - An image database including some texturesJAFFE Facial Expression Image Database - The JAFFE database consists of 213 images of Japanese female subjects posing 6 basic facial expressions as well as a neutral pose. Ratings on emotion adjectives are also available, free of charge, for research purposes. (Formats: TIFF Grayscale images.)ATR Research, Kyoto, JapanJISCT Stereo Evaluation - 44 image pairs. These data have been used in an evaluation of stereo analysis, as described in the April 1993 ARPA Image Understanding Workshop paper ``The JISCT Stereo Evaluation'' by R.C.Bolles, H.H.Baker, and M.J.Hannah, 263--274 (Formats: SSI)MIT Vision Texture - Image archive (100+ images) (Formats: ppm)MIT face images and more - hundreds of images (Formats: homebrew)Machine Vision - Images from the textbook by Jain, Kasturi, Schunck (20+ images) (Formats: GIF TIFF)Mammography Image Databases - 100 or more images of mammograms with ground truth. Additional images available by request, and links to several other mammography databases are provided. (Formats: homebrew)ftp://ftp.cps.msu.edu/pub/prip - many images (Formats: unknown)Middlebury Stereo Data Sets with Ground Truth - Six multi-frame stereo data sets of scenes containing planar regions. Each data set contains 9 color images and subpixel-accuracy ground-truth data. (Formats: ppm)Middlebury Stereo Vision Research Page - Middlebury CollegeModis Airborne simulator, Gallery and data set - High Altitude Imagery from around the world for environmental modeling in support of NASA EOS program (Formats: JPG and HDF)NIST Fingerprint and handwriting - datasets - thousands of images (Formats: unknown)NIST Fingerprint data - compressed multipart uuencoded tar fileNLM HyperDoc Visible Human Project - Color, CAT and MRI image samples - over 30 images (Formats: jpeg)National Design Repository - Over 55,000 3D CAD and solid models of (mostly) mechanical/machined engineerign designs. (Formats: gif,vrml,wrl,stp,sat)Geometric & Intelligent Computing LaboratoryOSU (MSU) 3D Object Model Database - several sets of 3D object models collected over several years to use in object recognition research (Formats: homebrew, vrml)OSU (MSU/WSU) Range Image Database - Hundreds of real and synthetic images (Formats: gif, homebrew)OSU/SAMPL Database: Range Images, 3D Models, Stills, Motion Sequences - Over 1000 range images, 3D object models, still images and motion sequences (Formats: gif, ppm, vrml, homebrew)Signal Analysis and Machine Perception LaboratoryOtago Optical Flow Evaluation Sequences - Synthetic and real sequences with machine-readable ground truth optical flow fields, plus tools to generate ground truth for new sequences. (Formats: ppm,tif,homebrew)Vision Research Groupftp://ftp.limsi.fr/pub/quenot/opflow/testdata/piv/ - Real and synthetic image sequences used for testing a Particle Image Velocimetry application. These images may be used for the test of optical flow and image matching algorithms. (Formats: pgm (raw))LIMSI-CNRS/CHM/IMM/visionLIMSI-CNRSPhotometric 3D Surface Texture Database - This is the first 3D texture database which provides both full real surface rotations and registered photometric stereo data (30 textures, 1680 images). (Formats: TIFF)SEQUENCES FOR OPTICAL FLOW ANALYSIS (SOFA) - 9 synthetic sequences designed for testing motion analysis applications, including full ground truth of motion and camera parameters. (Formats: gif)Computer Vision GroupSequences for Flow Based Reconstruction - synthetic sequence for testing structure from motion algorithms (Formats: pgm)Stereo Images with Ground Truth Disparity and Occlusion - a small set of synthetic images of a hallway with varying amounts of noise added. Use these images to benchmark your stereo algorithm. (Formats: raw, viff (khoros), or tiff)Stuttgart Range Image Database - A collection of synthetic range images taken from high-resolution polygonal models available on the web (Formats: homebrew)Department Image UnderstandingThe AR Face Database - Contains over 4,000 color images corresponding to 126 people's faces (70 men and 56 women). Frontal views with variations in facial expressions, illumination, and occlusions. (Formats: RAW (RGB 24-bit))Purdue Robot Vision LabThe MIT-CSAIL Database of Objects and Scenes - Database for testing multiclass object detection and scene recognition algorithms. Over 72,000 images with 2873 annotated frames. More than 50 annotated object classes. (Formats: jpg)The RVL SPEC-DB (SPECularity DataBase) - A collection of over 300 real images of 100 objects taken under three different illuminaiton conditions (Diffuse/Ambient/Directed). -- Use these images to test algorithms for detecting and compensating specular highlights in color images. (Formats: TIFF )Robot Vision LaboratoryThe Xm2vts database - The XM2VTSDB contains four digital recordings of 295 people taken over a period of four months. This database contains both image and video data of faces.Centre for Vision, Speech and Signal ProcessingTraffic Image Sequences and 'Marbled Block' Sequence - thousands of frames of digitized traffic image sequences as well as the 'Marbled Block' sequence (grayscale images) (Formats: GIF)IAKS/KOGSU Bern Face images - hundreds of images (Formats: Sun rasterfile)U Michigan textures (Formats: compressed raw)U Oulu wood and knots database - Includes classifications - 1000+ color images (Formats: ppm)UCID - an Uncompressed Colour Image Database - a benchmark database for image retrieval with predefined ground truth. (Formats: tiff)UMass Vision Image Archive - Large image database with aerial, space, stereo, medical images and more. (Formats: homebrew)UNC's 3D image database - many images (Formats: GIF)USF Range Image Data with Segmentation Ground Truth - 80 image sets (Formats: Sun rasterimage)University of Oulu Physics-based Face Database - contains color images of faces under different illuminants and camera calibration conditions as well as skin spectral reflectance measurements of each person.Machine Vision and Media Processing UnitUniversity of Oulu Texture Database - Database of 320 surface textures, each captured under three illuminants, six spatial resolutions and nine rotation angles. A set of test suites is also provided so that texture segmentation, classification, and retrieval algorithms can be tested in a standard manner. (Formats: bmp, ras, xv)Machine Vision GroupUsenix face database - Thousands of face images from many different sites (circa 994)View Sphere Database - Images of 8 objects seen from many different view points. The view sphere is sampled using a geodesic with 172 images/sphere. Two sets for training and testing are available. (Formats: ppm)PRIMA, GRAVIRVision-list Imagery Archive - Many images, many formatsWiry Object Recognition Database - Thousands of images of a cart, ladder, stool, bicycle, chairs, and cluttered scenes with ground truth labelings of edges and regions. (Formats: jpg)3D Vision GroupYale Face Database - 165 images (15 individuals) with different lighting, expression, and occlusion configurations.Yale Face Database B - 5760 single light source images of 10 subjects each seen under 576 viewing conditions (9 poses x 64 illumination conditions). (Formats: PGM)Center for Computational Vision and ControlFrameworksCaffeTorch7Theanocuda-convnetconvetjsCcvNuPICDeepLearning4JBrainDeepLearnToolboxDeepnetDeeppyJavaNNhebelMocha.jlOpenDLcuDNNMGLKUnet.jlNvidia DIGITS - a web app based on CaffeNeon - Python based Deep Learning FrameworkKeras - Theano based Deep Learning LibraryChainer - A flexible framework of neural networks for deep learningRNNLM ToolkitRNNLIB - A recurrent neural network librarychar-rnnMatConvNet: CNNs for MATLABMinerva - a fast and flexible tool for deep learning on multi-GPUMiscellaneousGoogle Plus - Deep Learning CommunityCaffe Webinar100 Best Github Resources in Github for DLWord2VecCaffe DockerFileTorontoDeepLEarning convnetgfx.jsTorch7 Cheat sheetMisc from MIT's 'Advanced Natural Language Processing' courseMisc from MIT's 'Machine Learning' courseMisc from MIT's 'Networks for Learning: Regression and Classification' courseMisc from MIT's 'Neural Coding and Perception of Sound' courseImplementing a Distributed Deep Learning Network over SparkA chess AI that learns to play chess using deep learning.Reproducing the results of "Playing Atari with Deep Reinforcement Learning" by DeepMindWiki2Vec. Getting Word2vec vectors for entities and word from Wikipedia DumpsThe original code from the DeepMind article + tweaksGoogle deepdream - Neural Network artAn efficient, batched LSTM.A recurrent neural network designed to generate classical music.
- Home >
- Catalog >
- Miscellaneous >
- Individual Tax Form >
- Form W-9 >
- w-9 instructions >
- Umass W 9