How to Edit and sign Task Name Risk Score Calculator 1 1 1 1 1 1 2 2 2 2 2 Online
Read the following instructions to use CocoDoc to start editing and filling out your Task Name Risk Score Calculator 1 1 1 1 1 1 2 2 2 2 2:
- In the beginning, seek the “Get Form” button and click on it.
- Wait until Task Name Risk Score Calculator 1 1 1 1 1 1 2 2 2 2 2 is shown.
- Customize your document by using the toolbar on the top.
- Download your customized form and share it as you needed.
An Easy Editing Tool for Modifying Task Name Risk Score Calculator 1 1 1 1 1 1 2 2 2 2 2 on Your Way
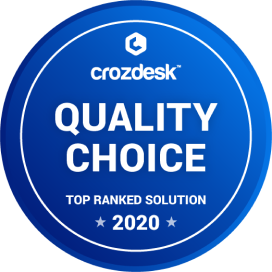
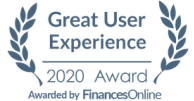
Open Your Task Name Risk Score Calculator 1 1 1 1 1 1 2 2 2 2 2 Immediately
Get FormHow to Edit Your PDF Task Name Risk Score Calculator 1 1 1 1 1 1 2 2 2 2 2 Online
Editing your form online is quite effortless. You don't have to install any software through your computer or phone to use this feature. CocoDoc offers an easy tool to edit your document directly through any web browser you use. The entire interface is well-organized.
Follow the step-by-step guide below to eidt your PDF files online:
- Find CocoDoc official website from any web browser of the device where you have your file.
- Seek the ‘Edit PDF Online’ icon and click on it.
- Then you will visit this awesome tool page. Just drag and drop the form, or select the file through the ‘Choose File’ option.
- Once the document is uploaded, you can edit it using the toolbar as you needed.
- When the modification is done, tap the ‘Download’ button to save the file.
How to Edit Task Name Risk Score Calculator 1 1 1 1 1 1 2 2 2 2 2 on Windows
Windows is the most widespread operating system. However, Windows does not contain any default application that can directly edit document. In this case, you can install CocoDoc's desktop software for Windows, which can help you to work on documents productively.
All you have to do is follow the guidelines below:
- Get CocoDoc software from your Windows Store.
- Open the software and then choose your PDF document.
- You can also choose the PDF file from Google Drive.
- After that, edit the document as you needed by using the diverse tools on the top.
- Once done, you can now save the customized paper to your computer. You can also check more details about how to edit a pdf PDF.
How to Edit Task Name Risk Score Calculator 1 1 1 1 1 1 2 2 2 2 2 on Mac
macOS comes with a default feature - Preview, to open PDF files. Although Mac users can view PDF files and even mark text on it, it does not support editing. Thanks to CocoDoc, you can edit your document on Mac without hassle.
Follow the effortless guidelines below to start editing:
- To start with, install CocoDoc desktop app on your Mac computer.
- Then, choose your PDF file through the app.
- You can attach the document from any cloud storage, such as Dropbox, Google Drive, or OneDrive.
- Edit, fill and sign your paper by utilizing this help tool from CocoDoc.
- Lastly, download the document to save it on your device.
How to Edit PDF Task Name Risk Score Calculator 1 1 1 1 1 1 2 2 2 2 2 with G Suite
G Suite is a widespread Google's suite of intelligent apps, which is designed to make your job easier and increase collaboration with each other. Integrating CocoDoc's PDF file editor with G Suite can help to accomplish work effectively.
Here are the guidelines to do it:
- Open Google WorkPlace Marketplace on your laptop.
- Seek for CocoDoc PDF Editor and get the add-on.
- Attach the document that you want to edit and find CocoDoc PDF Editor by choosing "Open with" in Drive.
- Edit and sign your paper using the toolbar.
- Save the customized PDF file on your cloud storage.
PDF Editor FAQ
What is the right way to AI interaction?
A range of Human-AI Interaction Guidelines have recently been released based on decade of study and validated by strict consumer studies in a range of AI products to help practitioners to develop better user-facing AI-based systems. This guidance encompasses a wide variety of different experiences: from the initial integration of an AI system by the user, changes and refinements of the AI model and management of AI errors to ongoing interactions.1- Initially Set the Right GoalsRule 1: Obvious what the program is able to do.Rule 2: Clear the system 's willingness to do what it should.It is essential for any user experience software to clear the capabilities and limitations of a program. In IA-based systems this can be especially relevant because people often have unreasonable expectations regarding their capabilities. The user manuals, comprehensive documentation and contextual assistance are standard techniques for transmitting this knowledge. We really don't know exactly how to create such things for the software AI today, sadly. For example, use your chosen personal assistant (e.g., Alexa, Cortana, Google Assistant, Siri) as a question answering device. Could we define precisely for what domains questions he can or can't answer ?If so, do we still know how specific every domain is? If the employee is able to answer historical questions, can he answer geographical questions as well? Can a computer research engineer confidently identify these facets? Inflated user expectations combined with a lack of clarification about the capabilities of the AI system could lead to unhappiness, mistrust or abandonment of the product in the best case and injury, unfairness and damage in the worst.2- In order to test AI model strengths and shortcomings rather than just aggregate, one-score testsThe explanation of AI systems' strengths and weaknesses begins with a more detailed understanding of the possible actions of an AI. It includes a rethought of existing appraisal methods focused on aggregated results numbers for one score.It is popular to evaluate model performance using statements like "90 per cent accurate Model X," for a specific measurement of machine learning evaluation. This aggregate number does not tell us too much whether we should expect consistent results over the entire benchmark or if this benchmark includes pools of data that are much less reliable. For fact, the latter happens much more frequently because of bias for training data or because it may be harder to understand certain concepts than others.The GenderShades research, which shows that the performance of gender recognition algorithms in women's detection with a darker skin tone is substantially reduced than that for other demographic groups, is a well known example of such behavior. It is definitely difficult to clarify these differences to end-users and consumers if the machine engineers themselves do not learn them.Multifaceted, systematic error analysis will help us respond to questions like: Is the model equally valid for all groups of people? Will the model perform substantially better or worse in certain environmental or input contexts? So poor is the percentile output of the 99th error ?Study Failure Rates at Various Granularity LevelsPandora is a process which can lead to a common explanation of failure. Pandora offers a range of performance views that run at various abstraction levels: global views (for overall device performance), cluster views (for individual data pockets) and instance views. It can be seen with every view how one or more input features are connected to model output in combination.Turning these views back and forth allows developers in various contexts to grasp errors better by slicing and dicing the data in ways guided by the likelihood of error. For example, the findings of this study have shown that the output can differ greatly in different regions for systems with rich and multi modal input spaces, and that differences can occur for quite various reasons.Examine failure patterns on different Data SlicesThe Errudite tool in the language domain enables developers to flexibly test input data and describe the output according to error levels. Errudite promotes data cutting by adding operators for data collection with semantical meaning. Furthermore, the tool also permits temporary edits that enable counter-factual analysis (i.e., what if a certain example had been slightly different?).3- Using Numerous & Practical Measurement MetricsTo determine an AI 's capacities and weaknesses using metrics, evaluating AI models on such data normally requires. The evaluation of AI models on established public metrics is often an excellent activity. When contrast with the other state of the art systems, it provides a quantitative viewpoint on how well the Program is performing. Nonetheless, for two reasons, it should not be the only form of assessment.Optimizing on one particular benchmark over and over and over again will lead to hidden overfitting in each model improvement process. For example, the induction distortion of modeling decision may have been driven to boost benchmark, even though the model is not trained or validated according to a benchmark. Sadly we have also promoted the practice in major competitions and scholarly papers by reporting and awarding, which raises the essential question of whether such processes can be rethunked in order to make true structures more comfortable.Second, our data can look very different from the distribution of the benchmark. A standard face detection dataset might not contain pictures with the same angle or lighting conditions as your application. In addition, these conditions can change over time as people use the system and modify their behaviour.In order to reduce these issues, you can:Track the model with more than one benchmark. In this way, you can test whether model improvements and modifications are generalized over different benchmarks.Cut metrics into a number of cases and track their performance to allow them to link back to the type of case when generalization is failing.Include in the assessment data from your real world app. If you are concerned that the application use data is not adequate, the good news is that you do not need as much information for assessment as you do for testing. Only small amounts of real information will expose failures which would be obscured otherwise.Enhance the assessment data by data increase (for example , visual transformations), testing under simulated adversarial distributions and the use of red teaming notions to assess errors that can not be found in established benchmarks.It must also be remembered that any improvement or review of metrics involves careful attention to privacy issues in order to avoid exposing user-friendly data in the evaluation process itself.4- Use Tests Based on Humans in the Evaluation of an AI 's BehaviorTo strengthen compliance with our AI, we need to incorporate human-centered indicators in our assessments in order for end-users to be able to trust us to live up to their beliefs. The model accuracy is one of the most widely used metrics. Nonetheless, precision can not always be used to satisfy the customer and to perform effectively.Work in the field of metric design shows that there are secret dimensions of model efficiency, which affect people but can not reflect existing metrics in domains like machine translation and image captioning. Similarly , the way people interpret precision may vary considerably from calculated accuracy. The difference depends on several factors related to the kind of errors and the reason for end-users for device accuracy.Human-centered AI assessment metrics are continuing to be developed, and are closer to human concepts and quality expectations. These metrics are especially important if a model is used to help individuals, such as decision-making or mixed initiative structures. Several metrics need to be considered:InteroperabilityWhat can a person understand how the model decides?FairnessWas the model working in different population groups comparable? Will the framework provide these sub-groups with equivalent toolsTeam utilityWhat is the functionality of man and machine? Is it better than any team effort alone?Performance Explainability –Should humans expect the program to make a mistake in advance?ComplementaryDoes the system actually substitute the human being or is it more oriented on the examples and tasks that people need ?These measures rely on the context and sometimes differ on the interpretation best for an application. The exact and formal description of these metrics depends These discussions however have led to numerous open source inputs in the form of software libraries which are often tailored for such human-centered metrics: InterpretML, FairLearn, AI Explainability 360.Remember that none of these metrics will substitute the assessment by real users such as user studies at the end of the day. If human assessment is too resource intensive for your case , at least consider using human annotators to look at smaller data divisions and see if your choice criteria fit with human-like conceptions of quality in your scenario.5- Using Models That are Easier to Explain to People about Their Results.We can have several hypotheses of equivalent or comparable accuracy during model optimization and hyperparameter search. Consider explainability of results as well as precision when determining which pattern to use. The explanability of results makes the model more human-centered, as it helps people to better understand and predict how errors the model will take over when required. In a recent study focusing on human beings, we found that the way people perceive and predict a model's error limit is important when working with an ML model for decision-making.Consider the following in order to find a model with greater explainability of performance:You what pick models with high parsimony (i.e., when the method is incorrect, and how complex the error explanation is?) and low stochasticity (i.e., to what degree is error separation possible by error explaining)?To assess the perceived parsimony and inventory attempt to approximate human mental models of error borders by training basic theories based on laws such as decision trees or rule lists based on past experiences (i.e. what humans have known about the error boundary). Naturally, only approximations or simulations can be learning models, but if they are easy enough, we can make sure that they do not contain false assumptions about the way people learn.More research is hoped for as part of model optimization and training to encourage better model selection through either through loss functions or through restricted reduction with the goal of developing model training for which humans can establish an informed and justified trust.6- Take into Consideration the Expense and Probability of Errors when Tuning Model ParametersWhile a specific program may make several types of errors, the probability will not be identical and different application-related costs will also occur. Many medical applications may, for example, be much more costly for a false negative mistake than a false positive, especially if the implications of non-treatment are more life-threatening for the patient than their related side effects when the condition is present. As may already have been expected, it is particularly important for high stakes decision-making to quantify these costs and risks directly.To order to evaluate model parameters the cost estimates should be used. Today, however, most models are usually trained and are not adapted to costs associated with a market , primarily because developers often do not understand these costs and they can vary between individual customers. Many models are therefore trained on the simplistic 0/1 loss norm strategies, which ideally serve the general applications.As costs differ between users, the long-term usage, orchestration and maintenance of the models is additionally complex. Nevertheless, the job has become more approachable due to ongoing improvement in Cloud deployment tools (e.g. MLOps on AzureML). Typically these services include and represent consumers in various endpoints of the different models.7- Uncertainty in Calibrate & ExplainThe methods proposed to date are more suitable for global or instance definition and measurement of device efficiency. Nevertheless, as model performance varies from case to instance, it may also help to set reasonable expectations for end-users (e.g. transmit model incertitudes on individual instances) by voicing model output at individual instances during the interaction. Model calibration aims to match learning forecasts with carefully calibrated confidence values that reflect the distribution of probability of error. This means that if a model recognizes a 95 % confidence traffic light in an picture, the probability of failure is 5 percent (over a large quantity of samples) when considering model prediction precision as a random variable.Some of the out-of-the-box algorithms for learning today don't come with normal property balanced uncertainties. The Naïve Bayes, SVMs and even neural networks have a few examples. For ambiguity testing, some methods you may use:Post-hoc Techniques (e.g. platform-scaling) that do not change how the model is equipped, but post phase the estimates of the model 's uncertainty to the exit likelihood.In-built Techniques mostly tailor-made to such model groups, but often in broader contexts ( e.g. bootstrapping or drop off for an uncertainty estimation).Uncertainty Explanation – Uncertainties used in manufacturing, in particular for systems with high dimensional and high outputs, can not always be easy to understand. For example, using an image subscribed program that gives a visually impaired user scene definition. The program has given the name "A group of people sitting around a table and having dinner" to the consumer and is 80% positive. When does the consumer know this trust? Does this mean there is nobody on the scene at all? And does it mean they don't have dinner but they're doing something else? For these instances, the semántics of the performance value are potentially important for users.Training Data vs. Real-world Distributions – When using the above-mentioned methods, it is important to be mindful that trust values would only be as good as training data as any other question. If, given our greatest calibration efforts, there are significant discrepancies between the actual data from the experiment and what the experiment has actually observed, confidence levels that still be unrelated to accuracy.8- ConclusionThis section presents techniques that engineering professionals should take advantage of to set the correct user standards about what and how well an AI system can do. Because fictional advertising is often difficult to discern from real functionalities, it is responsible for describing the desired product quality to the fullest extent possible. While it may still be difficult for data-intensive learning systems, machine learning and engineering practice such as this, and hopefully other activities that emerge in the future, will enable us to communicate the right message and build good faith.
What can be the unit of knowledge if we want to measure it?
Basic Concepts of MeasurementBefore you can use statistics to analyze a problem, you must convert information about the problem into data. That is, you must establish or adopt a system of assigning values, most often numbers, to the objects or concepts that are central to the problem in question. This is not an esoteric process but something people do every day. For instance, when you buy something at the store, the price you pay is a measurement: it assigns a number signifying the amount of money that you must pay to buy the item. Similarly, when you step on the bathroom scale in the morning, the number you see is a measurement of your body weight. Depending on where you live, this number may be expressed in either pounds or kilograms, but the principle of assigning a number to a physical quantity (weight) holds true in either case.Data need not be inherently numeric to be useful in an analysis. For instance, the categories male and female are commonly used in both science and everyday life to classify people, and there is nothing inherently numeric about these two categories. Similarly, we often speak of the colors of objects in broad classes such as red and blue, and there is nothing inherently numeric about these categories either. (Although you could make an argument about different wavelengths of light, it’s not necessary to have this knowledge to classify objects by color.)This kind of thinking in categories is a completely ordinary, everyday experience, and we are seldom bothered by the fact that different categories may be applied in different situations. For instance, an artist might differentiate among colors such as carmine, crimson, and garnet, whereas a layperson would be satisfied to refer to all of them as red. Similarly, a social scientist might be interested in collecting information about a person’s marital status in terms such as single—never married, single—divorced, and single—widowed, whereas to someone else, a person in any of those three categories could simply be considered single. The point is that the level of detail used in a system of classification should be appropriate, based on the reasons for making the classification and the uses to which the information will be put.MeasurementMeasurement is the process of systematically assigning numbers to objects and their properties to facilitate the use of mathematics in studying and describing objects and their relationships. Some types of measurement are fairly concrete: for instance, measuring a person’s weight in pounds or kilograms or his height in feet and inches or in meters. Note that the particular system of measurement used is not as important as the fact that we apply a consistent set of rules: we can easily convert a weight expressed in kilograms to the equivalent weight in pounds, for instance. Although any system of units may seem arbitrary (try defending feet and inches to someone who grew up with the metric system!), as long as the system has a consistent relationship with the property being measured, we can use the results in calculations.Measurement is not limited to physical qualities such as height and weight. Tests to measure abstract constructs such as intelligence or scholastic aptitude are commonly used in education and psychology, and the field of psychometrics is largely concerned with the development and refinement of methods to study these types of constructs. Establishing that a particular measurement is accurate and meaningful is more difficult when it can’t be observed directly. Although you can test the accuracy of one scale by comparing results with those obtained from another scale known to be accurate, and you can see the obvious use of knowing the weight of an object, the situation is more complex if you are interested in measuring a construct such as intelligence. In this case, not only are there no universally accepted measures of intelligence against which you can compare a new measure, there is not even common agreement about what “intelligence” means. To put it another way, it’s difficult to say with confidence what someone’s actual intelligence is because there is no certain way to measure it, and in fact, there might not even be common agreement on what it is. These issues are particularly relevant to the social sciences and education, where a great deal of research focuses on just such abstract concepts.Levels of MeasurementStatisticians commonly distinguish four types or levels of measurement, and the same terms can refer to data measured at each level. The levels of measurement differ both in terms of the meaning of the numbers used in the measurement system and in the types of statistical procedures that can be applied appropriately to data measured at each level.Nominal DataWith nominal data, as the name implies, the numbers function as a name or label and do not have numeric meaning. For instance, you might create a variable for gender, which takes the value 1 if the person is male and 0 if the person is female. The 0 and 1 have no numeric meaning but function simply as labels in the same way that you might record the values as M or F. However, researchers often prefer numeric coding systems for several reasons. First, it can simplify analyzing the data because some statistical packages will not accept nonnumeric values for use in certain procedures. (Hence, any data coded nonnumerically would have to be recoded before analysis.) Second, coding with numbers bypasses some issues in data entry, such as the conflict between upper- and lowercase letters (to a computer, M is a different value than m, but a person doing data entry might treat the two characters as equivalent).Nominal data is not limited to two categories. For instance, if you were studying the relationship between years of experience and salary in baseball players, you might classify the players according to their primary position by using the traditional system whereby 1 is assigned to the pitchers, 2 to the catchers, 3 to first basemen, and so on.If you can’t decide whether your data is nominal or some other level of measurement, ask yourself this question: do the numbers assigned to this data represent some quality such that a higher value indicates that the object has more of that quality than a lower value? Consider the example of coding gender so 0 signifies a female and 1 signifies a male. Is there some quality of gender-ness of which men have more than women? Clearly not, and the coding scheme would work as well if women were coded as 1 and men as 0. The same principle applies in the baseball example: there is no quality of baseball-ness of which outfielders have more than pitchers. The numbers are merely a convenient way to label subjects in the study, and the most important point is that every position is assigned a distinct value. Another name for nominal data is categorical data, referring to the fact that the measurements place objects into categories (male or female, catcher or first baseman) rather than measuring some intrinsic quality in them. Chapter 5 discusses methods of analysis appropriate for this type of data, and some of the techniques covered in Chapter 13 on nonparametric statistics are also appropriate for categorical data.When data can take on only two values, as in the male/female example, it can also be called binary data. This type of data is so common that special techniques have been developed to study it, including logistic regression (discussed in Chapter 11), which has applications in many fields. Many medical statistics, such as the odds ratio and the risk ratio (discussed in Chapter 15), were developed to describe the relationship between two binary variables because binary variables occur so frequently in medical research.Ordinal DataOrdinal data refers to data that has some meaningful order, so that higher values represent more of some characteristic than lower values. For instance, in medical practice, burns are commonly described by their degree, which describes the amount of tissue damage caused by the burn. A first-degree burn is characterized by redness of the skin, minor pain, and damage to the epidermis (outer layer of skin) only. A second-degree burn includes blistering and involves the superficial layer of the dermis (the layer of skin between the epidermis and the subcutaneous tissues), and a third-degree burn extends through the dermis and is characterized by charring of the skin and possibly destruction of nerve endings. These categories may be ranked in a logical order: first-degree burns are the least serious in terms of tissue damage, second-degree burns more serious, and third-degree burns the most serious. However, there is no metric analogous to a ruler or scale to quantify how great the distance between categories is, nor is it possible to determine whether the difference between first- and second-degree burns is the same as the difference between second- and third-degree burns.Many ordinal scales involve ranks. For instance, candidates applying for a job may be ranked by the personnel department in order of desirability as a new hire. This ranking tells you who is the preferred candidate, the second most preferred, and so on, but does not tell you whether the first and second candidates are in fact very similar to each other or the first-ranked candidate is much more preferable than the second. You could also rank countries of the world in order of their population, creating a meaningful order without saying anything about whether, say, the difference between the 30th and 31st countries was similar to that between the 31st and 32nd countries. The numbers used for measurement with ordinal data carry more meaning than those used in nominal data, and many statistical techniques have been developed to make full use of the information carried in the ordering while not assuming any further properties of the scales. For instance, it is appropriate to calculate the median (central value) of ordinal data but not the mean because it assumes equal intervals and requires division, which requires ratio-level data.Interval DataInterval data has a meaningful order and has the quality of equal intervals between measurements, representing equal changes in the quantity of whatever is being measured. The most common example of the interval level of measurement is the Fahrenheit temperature scale. If you describe temperature using the Fahrenheit scale, the difference between 10 degrees and 25 degrees (a difference of 15 degrees) represents the same amount of temperature change as the difference between 60 and 75 degrees. Addition and subtraction are appropriate with interval scales because a difference of 10 degrees represents the same amount of change in temperature over the entire scale. However, the Fahrenheit scale has no natural zero point because 0 on the Fahrenheit scale does not represent an absence of temperature but simply a location relative to other temperatures. Multiplication and division are not appropriate with interval data: there is no mathematical sense in the statement that 80 degrees is twice as hot as 40 degrees, for instance (although it is valid to say that 80 degrees is 40 degrees hotter than 40 degrees). Interval scales are a rarity, and it’s difficult to think of a common example other than the Fahrenheit scale. For this reason, the term “interval data” is sometimes used to describe both interval and ratio data (discussed in the next section).Ratio DataRatio data has all the qualities of interval data (meaningful order, equal intervals) and a natural zero point. Many physical measurements are ratio data: for instance, height, weight, and age all qualify. So does income: you can certainly earn 0 dollars in a year or have 0 dollars in your bank account, and this signifies an absence of money. With ratio-level data, it is appropriate to multiply and divide as well as add and subtract; it makes sense to say that someone with $100 has twice as much money as someone with $50 or that a person who is 30 years old is 3 times as old as someone who is 10.It should be noted that although many physical measurements are ratio-level, most psychological measurements are ordinal. This is particularly true of measures of value or preference, which are often measured by a Likert scale. For instance, a person might be presented with a statement (e.g., “The federal government should increase aid to education”) and asked to choose from an ordered set of responses (e.g., strongly agree, agree, no opinion, disagree, strongly disagree). These choices are sometimes assigned numbers (e.g., 1—strongly agree, 2—agree, etc.), and this sometimes gives people the impression that it is appropriate to apply interval or ratio techniques (e.g., computation of means, which involves division and is therefore a ratio technique) to such data. Is this correct? Not from the point of view of a statistician, but sometimes you do have to go with what the boss wants rather than what you believe to be true in absolute terms.Continuous and Discrete DataAnother important distinction is that between continuous and discrete data. Continuous data can take any value or any value within a range. Most data measured by interval and ratio scales, other than that based on counting, is continuous: for instance, weight, height, distance, and income are all continuous.In the course of data analysis and model building, researchers sometimes recode continuous data in categories or larger units. For instance, weight may be recorded in pounds but analyzed in 10-pound increments, or age recorded in years but analyzed in terms of the categories of 0–17, 18–65, and over 65. From a statistical point of view, there is no absolute point at which data becomes continuous or discrete for the purposes of using particular analytic techniques (and it’s worth remembering that if you record age in years, you are still imposing discrete categories on a continuous variable). Various rules of thumb have been proposed. For instance, some researchers say that when a variable has 10 or more categories (or, alternatively, 16 or more categories), it can safely be analyzed as continuous. This is a decision to be made based on the context, informed by the usual standards and practices of your particular discipline and the type of analysis proposed.Discrete variables can take on only particular values, and there are clear boundaries between those values. As the old joke goes, you can have 2 children or 3 children but not 2.37 children, so “number of children” is a discrete variable. In fact, any variable based on counting is discrete, whether you are counting the number of books purchased in a year or the number of prenatal care visits made during a pregnancy. Data measured on the nominal scale is always discrete, as is binary and rank-ordered data.OperationalizationPeople just starting out in a field of study often think that the difficulties of research rest primarily in statistical analysis, so they focus their efforts on learning mathematical formulas and computer programming techniques to carry out statistical calculations. However, one major problem in research has very little to do with either mathematics or statistics and everything to do with knowing your field of study and thinking carefully through practical problems of measurement. This is the problem of operationalization, which means the process of specifying how a concept will be defined and measured.Operationalization is always necessary when a quality of interest cannot be measured directly. An obvious example is intelligence. There is no way to measure intelligence directly, so in the place of such a direct measurement, we accept something that we can measure, such as the score on an IQ test. Similarly, there is no direct way to measure “disaster preparedness” for a city, but we can operationalize the concept by creating a checklist of tasks that should be performed and giving each city a disaster-preparedness score based on the number of tasks completed and the quality or thoroughness of completion. For a third example, suppose you wish to measure the amount of physical activity performed by individual subjects in a study. If you do not have the capacity to monitor their exercise behavior directly, you can operationalize “amount of physical activity” as the amount indicated on a self-reported questionnaire or recorded in a diary.Because many of the qualities studied in the social sciences are abstract, operationalization is a common topic of discussion in those fields. However, it is applicable to many other fields as well. For instance, the ultimate goals of the medical profession include reducing mortality (death) and reducing the burden of disease and suffering. Mortality is easily verified and quantified but is frequently too blunt an instrument to be useful since it is a thankfully rare outcome for most diseases. “Burden of disease” and “suffering,” on the other hand, are concepts that could be used to define appropriate outcomes for many studies but that have no direct means of measurement and must therefore be operationalized. Examples of operationalization of burden of disease include measurement of viral levels in the bloodstream for patients with AIDS and measurement of tumor size for people with cancer. Decreased levels of suffering or improved quality of life may be operationalized as a higher self-reported health state, a higher score on a survey instrument designed to measure quality of life, an improved mood state as measured through a personal interview, or reduction in the amount of morphine requested for pain relief.Some argue that measurement of even physical quantities such as length require operationalization because there are different ways to measure even concrete properties such as length. (A ruler might be the appropriate instrument in some circumstances, a micrometer in others.) Even if you concede this point, it seems clear that the problem of operationalization is much greater in the human sciences, when the objects or qualities of interest often cannot be measured directly.Proxy MeasurementThe term proxy measurement refers to the process of substituting one measurement for another. Although deciding on proxy measurements can be considered as a subclass of operationalization, this book will consider it as a separate topic. The most common use of proxy measurement is that of substituting a measurement that is inexpensive and easily obtainable for a different measurement that would be more difficult or costly, if not impossible, to collect. Another example is collecting information about one person by asking another, for instance, by asking a parent to rate her child’s mood state.For a simple example of proxy measurement, consider some of the methods police officers use to evaluate the sobriety of individuals while in the field. Lacking a portable medical lab, an officer can’t measure a driver’s blood alcohol content directly to determine whether the driver is legally drunk. Instead, the officer might rely on observable signs associated with drunkenness, simple field tests that are believed to correlate well with blood alcohol content, a breath alcohol test, or all of these. Observational signs of alcohol intoxication include breath smelling of alcohol, slurred speech, and flushed skin. Field tests used to evaluate alcohol intoxication quickly generally require the subjects to perform tasks such as standing on one leg or tracking a moving object with their eyes. A Breathalyzer test measures the amount of alcohol in the breath. None of these evaluation methods provides a direct test of the amount of alcohol in the blood, but they are accepted as reasonable approximations that are quick and easy to administer in the field.To look at another common use of proxy measurement, consider the various methods used in the United States to evaluate the quality of health care provided by hospitals and physicians. It is difficult to think of a direct way to measure quality of care, short of perhaps directly observing the care provided and evaluating it in relation to accepted standards (although you could also argue that the measurement involved in such an evaluation process would still be an operationalization of the abstract concept of “quality of care”). Implementing such an evaluation method would be prohibitively expensive, would rely on training a large crew of evaluators and relying on their consistency, and would be an invasion of patients’ right to privacy. A solution commonly adopted instead is to measure processes that are assumed to reflect higher quality of care: for instance, whether anti-tobacco counseling was appropriately provided in an office visit or whether appropriate medications were administered promptly after a patient was admitted to the hospital.Proxy measurements are most useful if, in addition to being relatively easy to obtain, they are good indicators of the true focus of interest. For instance, if correct execution of prescribed processes of medical care for a particular treatment is closely related to good patient outcomes for that condition, and if poor or nonexistent execution of those processes is closely related to poor patient outcomes, then execution of these processes may be a useful proxy for quality. If that close relationship does not exist, then the usefulness of the proxy measurements is less certain. No mathematical test will tell you whether one measure is a good proxy for another, although computing statistics such as correlations or chi-squares between the measures might help evaluate this issue. In addition, proxy measurements can pose their own difficulties. To take the example of evaluating medical care in terms of procedures performed, this method assumes that it is possible to determine, without knowledge of individual cases, what constitutes appropriate treatment and that records are available that contain the information needed to determine what procedures were performed. Like many measurement issues, choosing good proxy measurements is a matter of judgment informed by knowledge of the subject area, usual practices in the field in question, and common sense.Surrogate EndpointsA surrogate endpoint is a type of proxy measurement sometimes used in clinical trials as a substitute for a true clinical endpoint. For instance, a treatment might be intended to prevent death (a true clinical endpoint), but because death from the condition being treated might be rare, a surrogate endpoint may be used to accrue evidence more quickly about the treatment’s effectiveness. A surrogate endpoint is usually a biomarker that is correlated with a true clinical endpoint. For instance, if a drug is intended to prevent death from prostate cancer, a surrogate endpoint might be tumor shrinkage or reduction in levels of prostate-specific antigens.The problem with using surrogate endpoints is that although a treatment might be effective in producing improvement in these endpoints, it does not necessarily mean that it will be successful in achieving the clinical outcome of interest. For instance, a meta-analysis by Stefan Michiels and colleagues (listed in Appendix C) found that for locally advanced head and neck squamous-cell carcinoma, the correlation between locoregional control (a surrogate endpoint) and overall survival (the true clinical endpoint) ranged from 0.65 to 0.76 (if results had been identical for both endpoints, the correlation would have been 1.00), whereas the correlation between event-free survival (a surrogate endpoint) and overall survival ranged from 0.82 to 0.90.Surrogate endpoints are sometimes misused by being added after the fact to a clinical trial, being used as substitutes for outcomes defined before the trial begins, or both. Because a surrogate endpoint might be easier to achieve (e.g., improvement in progression-free survival in the trial for an anti-cancer drug rather than improvement in overall survival), this can lead to a new drug being approved on the basis of effectiveness when it might have little effect on the true endpoint or even have a deleterious effect. For further general discussion of issues relating to surrogate endpoints, see the article by Thomas R. Fleming cited in Appendix C.True and Error ScoresWe can safely assume that few, if any, measurements are completely accurate. This is true not only because measurements are made and recorded by human beings but also because the process of measurement often involves assigning discrete numbers to a continuous world. One concern of measurement theory is conceptualizing and quantifying the degree of error present in a particular set of measurements and evaluating the sources and consequences of that error.Classical measurement theory conceives of any measurement or observed score as consisting of two parts: true score (T) and error (E). This is expressed in the following formula:X = T + Ewhere X is the observed measurement, T is the true score, and E is the error. For instance, a bathroom scale might measure someone’s weight as 120 pounds when that person’s true weight is 118 pounds, and the error of 2 pounds is due to the inaccuracy of the scale. This would be expressed, using the preceding formula, as:120 = 118 + 2which is simply a mathematical equality expressing the relationship among the three components. However, both T and E are hypothetical constructs. In the real world, we seldom know the precise value of the true score and therefore cannot know the exact value of the error score either. Much of the process of measurement involves estimating both quantities and maximizing the true component while minimizing error. For instance, if you took a number of measurements of one person’s body weight in a short period (so that his true weight could be assumed to have remained constant), using a recently calibrated scale, you might accept the average of all those measurements as a good estimate of that individual’s true weight. You could then consider the variance between this average and each individual measurement as the error due to the measurement process, such as slight malfunctioning in the scale or the technician’s imprecision in reading and recording the results.Random and Systematic ErrorBecause we live in the real world rather than a Platonic universe, we assume that all measurements contain some error. However, not all error is created equal, and we can learn to live with random error while doing whatever we can to avoid systematic error. Random error is error due to chance: it has no particular pattern and is assumed to cancel itself out over repeated measurements. For instance, the error scores over a number of measurements of the same object are assumed to have a mean of zero. Therefore, if someone is weighed 10 times in succession on the same scale, you may observe slight differences in the number returned to you: some will be higher than the true value, and some will be lower. Assuming the true weight is 120 pounds, perhaps the first measurement will return an observed weight of 119 pounds (including an error of −1 pound), the second an observed weight of 122 pounds (for an error of +2 pounds), the third an observed weight of 118.5 pounds (an error of −1.5 pounds), and so on. If the scale is accurate and the only error is random, the average error over many trials will be 0, and the average observed weight will be 120 pounds. You can strive to reduce the amount of random error by using more accurate instruments, training your technicians to use them correctly, and so on, but you cannot expect to eliminate random error entirely.Two other conditions are assumed to apply to random error: it is unrelated to the true score, and the error component of one measurement is unrelated to the error component of any other measurement. The first condition means that the value of the error component of any measurement is not related to the value of the true score for that measurement. For instance, if you measure the weights of a number of individuals whose true weights differ, you would not expect the error component of each measurement to have any relationship to each individual’s true weight. This means that, for example, the error component should not systematically be larger when the true score (the individual’s actual weight) is larger. The second condition means that the error component of each score is independent and unrelated to the error component for any other score. For instance, in a series of measurements, a pattern of the size of the error component should not be increasing over time so that later measurements have larger errors, or errors in a consistent direction, relative to earlier measurements. The first requirement is sometimes expressed by saying that the correlation of true and error scores is 0, whereas the second is sometimes expressed by saying that the correlation of the error components is 0 (correlation is discussed in more detail in Chapter 7).In contrast, systematic error has an observable pattern, is not due to chance, and often has a cause or causes that can be identified and remedied. For instance, a scale might be incorrectly calibrated to show a result that is 5 pounds over the true weight, so the average of multiple measurements of a person whose true weight is 120 pounds would be 125 pounds, not 120. Systematic error can also be due to human factors: perhaps the technician is reading the scale’s display at an angle so that she sees the needle as registering higher than it is truly indicating. If a pattern is detected with systematic error, for instance, measurements drifting higher over time (so the error components are random at the beginning of the experiment, but later on are consistently high), this is useful information because we can intervene and recalibrate the scale. A great deal of effort has been expended to identify sources of systematic error and devise methods to identify and eliminate them: this is discussed further in the upcoming section Measurement Bias.Reliability and ValidityThere are many ways to assign numbers or categories to data, and not all are equally useful. Two standards we commonly use to evaluate methods of measurement (for instance, a survey or a test) are reliability and validity. Ideally, we would like every method we use to be both reliable and valid. In reality, these qualities are not absolutes but are matters of degree and often specific to circumstance. For instance, a survey that is highly reliable when used with demographic groups might be unreliable when used with a different group. For this reason, rather than discussing reliability and validity as absolutes, it is often more useful to evaluate how valid and reliable a method of measurement is for a particular purpose and whether particular levels of reliability and validity are acceptable in a specific context. Reliability and validity are also discussed in Chapter 18 in the context of research design, and in Chapter 16 in the context of educational and psychological testing.ReliabilityReliability refers to how consistent or repeatable measurements are. For instance, if we give the same person the same test on two occasions, will the scores be similar on both occasions? If we train three people to use a rating scale designed to measure the quality of social interaction among individuals, then show each of them the same film of a group of people interacting and ask them to evaluate the social interaction exhibited, will their ratings be similar? If we have a technician weigh the same part 10 times using the same instrument, will the measurements be similar each time? In each case, if the answer is yes, we can say the test, scale, or rater is reliable.Much of the theory of reliability was developed in the field of educational psychology, and for this reason, measures of reliability are often described in terms of evaluating the reliability of tests. However, considerations of reliability are not limited to educational testing; the same concepts apply to many other types of measurements, including polling, surveys, and behavioral ratings.The discussion in this chapter will remain at a basic level. Information about calculating specific measures of reliability is discussed in more detail in Chapter 16 in the context of test theory. Many of the measures of reliability draw on the correlation coefficient (also called simply the correlation), which is discussed in detail in Chapter 7, so beginning statisticians might want to concentrate on the logic of reliability and validity and leave the details of evaluating them until after they have mastered the concept of the correlation coefficient.There are three primary approaches to measuring reliability, each useful in particular contexts and each having particular advantages and disadvantages:Multiple-occasions reliabilityMultiple-forms reliabilityInternal consistency reliabilityMultiple-occasions reliability, sometimes called test-retest reliability, refers to how similarly a test or scale performs over repeated administration. For this reason, it is sometimes referred to as an index of temporal stability, meaning stability over time. For instance, you might have the same person do two psychological assessments of a patient based on a videotaped interview, with the assessments performed two weeks apart, and compare the results. For this type of reliability to make sense, you must assume that the quantity being measured has not changed, hence the use of the same videotaped interview rather than separate live interviews with a patient whose psychological state might have changed over the two-week period. Multiple-occasions reliability is not a suitable measure for volatile qualities, such as mood state, or if the quality or quantity being measured could have changed in the time between the two measurements (for instance, a student’s knowledge of a subject she is actively studying). A common technique for assessing multiple-occasions reliability is to compute the correlation coefficient between the scores from each occasion of testing; this is called the coefficient of stability.Multiple-forms reliability (also called parallel-forms reliability) refers to howsimilarly different versions of a test or questionnaire perform in measuring the same entity. A common type of multiple-forms reliability is split-half reliability in which a pool of items believed to be homogeneous is created, then half the items are allocated to form A and half to form B. If the two (or more) forms of the test are administered to the same people on the same occasion, the correlation between the scores received on each form is an estimate of multiple-forms reliability. This correlation is sometimes called the coefficient of equivalence. Multiple-forms reliability is particularly important for standardized tests that exist in multiple versions. For instance, different forms of the SAT (Scholastic Aptitude Test, used to measure academic ability among students applying to American colleges and universities) are calibrated so the scores achieved are equivalent no matter which form a particular student takes.Internal consistency reliability refers to how well the items that make up an instrument (for instance, a test or survey) reflect the same construct. To put it another way, internal consistency reliability measures how much the items on an instrument are measuring the same thing. Unlike multiple-forms and multiple-occasions reliability, internal consistency reliability can be assessed by administering a single instrument on a single occasion. Internal consistency reliability is a more complex quantity to measure than multiple-occasions or parallel-forms reliability, and several methods have been developed to evaluate it; these are further discussed in Chapter 16. However, all these techniques depend primarily on the inter-item correlation, that is, the correlation of each item on a scale or a test with each other item. If such correlations are high, that is interpreted as evidence that the items are measuring the same thing, and the various statistics used to measure internal consistency reliability will all be high. If the inter-item correlations are low or inconsistent, the internal consistency reliability statistics will be lower, and this is interpreted as evidence that the items are not measuring the same thing.Two simple measures of internal consistency are most useful for tests made up of multiple items covering the same topic, of similar difficulty, and that will be scored as a composite: the average inter-item correlation and the average item-total correlation. To calculate the average inter-item correlation, you find the correlation between each pair of items and take the average of all these correlations. To calculate the average item-total correlation, you create a total score by adding up scores on each individual item on the scale and then compute the correlation of each item with the total. The average item-total correlation is the average of those individual item-total correlations.Split-half reliability, described previously, is another method of determining internal consistency. This method has the disadvantage that, if the items are not truly homogeneous, different splits will create forms of disparate difficulty, and the reliability coefficient will be different for each pair of forms. A method that overcomes this difficulty is Cronbach’s alpha (also called coefficient alpha), which is equivalent to the average of all possible split-half estimates. For more about Cronbach’s alpha, including a demonstration of how to compute it, see Chapter 16.ValidityValidity refers to how well a test or rating scale measures what it is supposed to measure. Some researchers describe validation as the process of gathering evidence to support the types of inferences intended to be drawn from the measurements in question. Researchers disagree about how many types of validity there are, and scholarly consensus has varied over the years as different types of validity are subsumed under a single heading one year and then separated and treated as distinct the next. To keep things simple, this book will adhere to a commonly accepted categorization of validity that recognizes four types: content validity, construct validity, concurrent validity, and predictive validity. The face validity, which is closely related to content validity, will also be discussed. These types of validity are discussed further in the context of research design in Chapter 18.Content validity refers to how well the process of measurement reflects the important content of the domain of interest and is of particular concern when the purpose of the measurement is to draw inferences about a larger domain of interest. For instance, potential employees seeking jobs as computer programmers might be asked to complete an examination that requires them to write or interpret programs in the languages they would use on the job if hired. Due to time restrictions, only limited content and programming competencies may be included on such an examination, relative to what might actually be required for a professional programming job. However, if the subset of content and competencies is well chosen, the score on such an exam can be a good indication of the individual’s ability on all the important types of programming required by the job. If this is the case, we may say the examination has content validity.A closely related concept to content validity is known as face validity. A measure with good face validity appears (to a member of the general public or a typical person who may be evaluated by the measure) to be a fair assessment of the qualities under study. For instance, if a high school geometry test is judged by parents of the students taking the test to be a fair test of algebra, the test has good face validity. Face validity is important in establishing credibility; if you claim to be measuring students’ geometry achievement but the parents of your students do not agree, they might be inclined to ignore your statements about their children’s levels of achievement in this subject. In addition, if students are told they are taking a geometry test that appears to them to be something else entirely, they might not be motivated to cooperate and put forth their best efforts, so their answers might not be a true reflection of their abilities.Concurrent validity refers to how well inferences drawn from a measurement can be used to predict some other behavior or performance that is measured at approximately the same time. For instance, if an achievement test score is highly related to contemporaneous school performance or to scores on similar tests, it has high concurrent validity. Predictive validity is similar but concerns the ability to draw inferences about some event in the future. To continue with the previous example, if the score on an achievement test is highly related to school performance the following year or to success on a job undertaken in the future, it has high predictive validity.TriangulationBecause every system of measurement has its flaws, researchers often use several approaches to measure the same thing. For instance, American universities often use multiple types of information to evaluate high school seniors’ scholastic ability and the likelihood that they will do well in university studies. Measurements used for this purpose can include scores on standardized exams such as the SAT, high school grades, a personal statement or essay, and recommendations from teachers. In a similar vein, hiring decisions in a company are usually made after consideration of several types of information, including an evaluation of each applicant’s work experience, his education, the impression he makes during an interview, and possibly a work sample and one or more competency or personality tests.This process of combining information from multiple sources to arrive at a true or at least more accurate value is called triangulation, a loose analogy to the process in geometry of determining the location of a point in terms of its relationship to two other known points. The key idea behind triangulation is that, although a single measurement of a concept might contain too much error (of either known or unknown types) to be either reliable or valid by itself, by combining information from several types of measurements, at least some of whose characteristics are already known, we can arrive at an acceptable measurement of the unknown quantity. We expect that each measurement contains error, but we hope it does not include the same type of error, so that through multiple types of measurement, we can get a reasonable estimate of the quantity or quality of interest.Establishing a method for triangulation is not a simple matter. One historical attempt to do this is the multitrait, multimethod matrix (MTMM) developed by Campbell and Fiske (1959). Their particular concern was to separate the part of a measurement due to the quality of interest from that part due to the method of measurement used. Although their specific methodology is used less today and full discussion of the MTMM technique is beyond the scope of a beginning text, the concept remains useful as an example of one way to think about measurement error and validity.The MTMM is a matrix of correlations among measures of several concepts (the traits), each measured in several ways (the methods). Ideally, the same several methods will be used for each trait. Within this matrix, we expect different measures of the same trait to be highly related; for instance, scores of intelligence measured by several methods, such as a pencil-and-paper test, practical problem solving, and a structured interview, should all be highly correlated. By the same logic, scores reflecting different constructs that are measured in the same way should not be highly related; for instance, scores on intelligence, deportment, and sociability as measured by pencil-and-paper questionnaires should not be highly correlated.Measurement BiasConsideration of measurement bias is important in almost every field, but it is a particular concern in the human sciences. Many specific types of bias have been identified and defined. They won’t all be named here, but a few common types will be discussed. Most research design textbooks treat measurement bias in great detail and can be consulted for further discussion of this topic. The most important point is that the researcher must always be alert to the possibility of bias because failure to consider and deal with issues related to bias can invalidate the results of an otherwise exemplary study.Bias can enter studies in two primary ways: during the selection and retention of the subjects of study or in the way information is collected about the subjects. In either case, the defining feature of bias is that it is a source of systematic rather than random error. The result of bias is that the data analyzed in a study is incorrect in a systematic fashion, which can lead to false conclusions despite the application of correct statistical procedures and techniques. The next two sections discuss some of the more common types of bias, organized into two major categories: bias in sample selection and retention and bias resulting from information collection and recording.Bias in Sample Selection and RetentionMost studies take place on samples of subjects, whether patients with leukemia or widgets produced by a factory, because it would be prohibitively expensive if not entirely impossible to study the entire population of interest. The sample needs to be a good representation of the study population (the population to which the results are meant to apply) for the researcher to be comfortable using the results from the sample to describe the population. If the sample is biased, meaning it is not representative of the study population, conclusions drawn from the study sample might not apply to the study population.Selection bias exists if some potential subjects are more likely than others to be selected for the study sample. This term is usually reserved for bias that occurs due to the process of sampling. For instance, telephone surveys conducted using numbers from published directories by design remove from the pool of potential respondents people with unpublished numbers or those who have changed phone numbers since the directory was published. Random-digit-dialing (RDD) techniques overcome these problems but still fail to include people living in households without telephones or who have only a cell (mobile) phone. This is a problem for a research study because if the people excluded differ systematically on a characteristic of interest (and this is a very common occurrence), the results of the survey will be biased. For instance, people living in households with no telephone service tend to be poorer than those who have a telephone, and people who have only a cell phone (i.e., no land line) tend to be younger than those who have residential phone service. If poverty or youth are related to the subject being studied, excluding these individuals from the sample will introduce bias into the study.Volunteer bias refers to the fact that people who volunteer to be in studies are usually not representative of the population as a whole. For this reason, results from entirely volunteer samples, such as the phone-in polls featured on some television programs, are not useful for scientific purposes (unless, of course, the population of interest is people who volunteer to participate in such polls). Multiple layers of nonrandom selection might be at work in this example. For instance, to respond, the person needs to be watching the television program in question. This means she is probably at home; hence, responses to polls conducted during the normal workday might draw an audience largely of retired people, housewives, and the unemployed. To respond, a person also needs to have ready access to a telephone and to have whatever personality traits would influence him to pick up the telephone and call a number he sees on the television screen. The problems with telephone polls have already been discussed, and the probability that personality traits are related to other qualities being studied is too high to ignore.Nonresponse bias refers to the other side of volunteer bias. Just as people who volunteer to take part in a study are likely to differ systematically from those who do not, so people who decline to participate in a study when invited to do so very likely differ from those who consent to participate. You probably know people who refuse to participate in any type of telephone survey. (I’m such a person myself.) Do they seem to be a random selection from the general population? Probably not; for instance, the Joint Canada/U.S. Survey of Health found not only different response rates for Canadians versus Americans but found nonresponse bias for nearly all major health status and health care access measures [results are summarized here].Informative censoring can create bias in any longitudinal study (a study in which subjects are followed over a period of time). Losing subjects during a long-term study is a common occurrence, but the real problem comes when subjects do not drop out at random but for reasons related to the study’s purpose. Suppose we are comparing two medical treatments for a chronic disease by conducting a clinical trial in which subjects are randomly assigned to one of several treatment groups and followed for five years to see how their disease progresses. Thanks to our use of a randomized design, we begin with a perfectly balanced pool of subjects. However, over time, subjects for whom the assigned treatment is not proving effective will be more likely to drop out of the study, possibly to seek treatment elsewhere, leading to bias. If the final sample of subjects we analyze consists only of those who remain in the trial until its conclusion, and if those who drop out of the study are not a random selection of those who began it, the sample we analyze will no longer be the nicely randomized sample we began with. Instead, if dropping out was related to treatment ineffectiveness, the final subject pool will be biased in favor of those who responded effectively to their assigned treatment.Information BiasEven if the perfect sample is selected and retained, bias can enter a study through the methods used to collect and record data. This type of bias is often called information bias because it affects the validity of the information upon which the study is based, which can in turn invalidate the results of the study.When data is collected using in-person or telephone interviews, a social relationship exists between the interviewer and the subject for the course of the interview. This relationship can adversely affect the quality of the data collected. When bias is introduced into the data collected because of the attitudes or behavior of the interviewer, this is known as interviewer bias. This type of bias might be created unintentionally when the interviewer knows the purpose of the study or the status of the individuals being interviewed. For instance, interviewers might ask more probing questions to encourage the subject to recall chemical exposures if they know the subject is suffering from a rare type of cancer related to chemical exposure. Interviewer bias might also be created if the interviewer displays personal attitudes or opinions that signal to the subject that she disapproves of the behaviors being studied, such as promiscuity or drug use, making the subject less likely to report those behaviors.Recall bias refers to the fact that people with a life experience such as suffering from a serious disease or injury are more likely to remember events that they believe are related to that experience. For instance, women who suffered a miscarriage are likely to have spent a great deal of time probing their memories for exposures or incidents that they believe could have caused the miscarriage. Women who had a normal birth may have had similar exposures but have not given them as much thought and thus will not recall them when asked on a survey.Detection bias refers to the fact that certain characteristics may be more likely to be detected or reported in some people than in others. For instance, athletes in some sports are subject to regular testing for performance-enhancing drugs, and test results are publicly reported. World-class swimmers are regularly tested for anabolic steroids, for instance, and positive tests are officially recorded and often released to the news media as well. Athletes competing at a lower level or in other sports may be using the same drugs but because they are not tested as regularly, or because the test results are not publicly reported, there is no record of their drug use. It would be incorrect to assume, for instance, that because reported anabolic steroid use is higher in swimming than in baseball, the actual rate of steroid use is higher in swimming than in baseball. The observed difference in steroid use could be due to more aggressive testing on the part of swimming officials and more public disclosure of the test results.Social desirability bias is caused by people’s desire to present themselves in a favorable light. This often motivates them to give responses that they believe will please the person asking the question. Note that this type of bias can operate even if the questioner is not actually present, for instance when subjects complete a pencil-and-paper survey. Social desirability bias is a particular problem in surveys that ask about behaviors or attitudes that are subject to societal disapproval, such as criminal behavior, or that are considered embarrassing, such as incontinence. Social desirability bias can also influence responses in surveys if questions are asked in a way that signals what the “right,” that is, socially desirable, answer is.ExercisesHere’s a review of the topics covered in this chapter.ProblemWhat potential types of bias should you be aware of in each of the following scenarios, and what is the likely effect on the results?A university reports the average annual salary of its graduates as $120,000, based on responses to a survey of contributors to the alumni fund.A program intended to improve scholastic achievement in high school students reports success because the 40 students who completed the year-long program (of the 100 who began it) all showed significant improvement in their grades and scores on standardized tests of achievement.A manager is concerned about the health of his employees, so he institutes a series of lunchtime lectures on topics such as healthy eating, the importance of exercise, and the deleterious health effects of smoking and drinking. He conducts an anonymous survey (using a paper-and-pencil questionnaire) of employees before and after the lecture series and finds that the series has been effective in increasing healthy behaviors and decreasing unhealthy behaviors.SolutionSelection bias and nonresponse bias, both of which affect the quality of the sample analyzed. The reported average annual salary is probably an overestimate of the true value because subscribers to the alumni magazine were probably among the more successful graduates, and people who felt embarrassed about their low salary were less likely to respond. One could also argue a type of social desirability bias that would result in calculating an overly high average annual salary because graduates might be tempted to report higher salaries than they really earn because it is desirable to have a high income.Informative censoring, which affects the quality of the sample analyzed. The estimate of the program’s effect on high school students is probably overestimated. The program certainly seems to have been successful for those who completed it, but because more than half the original participants dropped out, we can’t say how successful it would be for the average student. It might be that the students who completed the program were more intelligent or motivated than those who dropped out or that those who dropped out were not being helped by the program.Social desirability bias, which affects the quality of information collected. This will probably result in an overestimate of the effectiveness of the lecture program. Because the manager has made it clear that he cares about the health habits of his employees, they are likely to report making more improvements in their health behaviors than they have actually made to please the boss.
- Home >
- Catalog >
- Miscellaneous >
- Method Statement >
- Task Name Risk Score Calculator 1 1 1 1 1 1 2 2 2 2 2