The Guide of finalizing Blue Sky Application Online
If you are curious about Alter and create a Blue Sky Application, here are the simple steps you need to follow:
- Hit the "Get Form" Button on this page.
- Wait in a petient way for the upload of your Blue Sky Application.
- You can erase, text, sign or highlight of your choice.
- Click "Download" to download the files.
A Revolutionary Tool to Edit and Create Blue Sky Application
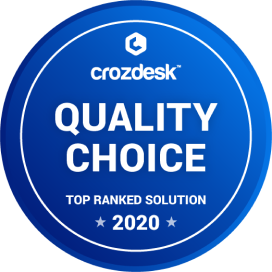
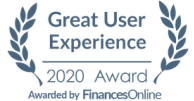
How to Easily Edit Blue Sky Application Online
CocoDoc has made it easier for people to Customize their important documents with the online platform. They can easily Customize according to their ideas. To know the process of editing PDF document or application across the online platform, you need to follow these steps:
- Open the official website of CocoDoc on their device's browser.
- Hit "Edit PDF Online" button and Append the PDF file from the device without even logging in through an account.
- Edit your PDF for free by using this toolbar.
- Once done, they can save the document from the platform.
Once the document is edited using online website, you can download or share the file as what you want. CocoDoc ensures that you are provided with the best environment for fulfiling the PDF documents.
How to Edit and Download Blue Sky Application on Windows
Windows users are very common throughout the world. They have met millions of applications that have offered them services in modifying PDF documents. However, they have always missed an important feature within these applications. CocoDoc wants to provide Windows users the ultimate experience of editing their documents across their online interface.
The steps of modifying a PDF document with CocoDoc is very simple. You need to follow these steps.
- Choose and Install CocoDoc from your Windows Store.
- Open the software to Select the PDF file from your Windows device and move on editing the document.
- Customize the PDF file with the appropriate toolkit appeared at CocoDoc.
- Over completion, Hit "Download" to conserve the changes.
A Guide of Editing Blue Sky Application on Mac
CocoDoc has brought an impressive solution for people who own a Mac. It has allowed them to have their documents edited quickly. Mac users can make a PDF fillable with the help of the online platform provided by CocoDoc.
In order to learn the process of editing form with CocoDoc, you should look across the steps presented as follows:
- Install CocoDoc on you Mac firstly.
- Once the tool is opened, the user can upload their PDF file from the Mac hasslefree.
- Drag and Drop the file, or choose file by mouse-clicking "Choose File" button and start editing.
- save the file on your device.
Mac users can export their resulting files in various ways. Not only downloading and adding to cloud storage, but also sharing via email are also allowed by using CocoDoc.. They are provided with the opportunity of editting file through different ways without downloading any tool within their device.
A Guide of Editing Blue Sky Application on G Suite
Google Workplace is a powerful platform that has connected officials of a single workplace in a unique manner. When allowing users to share file across the platform, they are interconnected in covering all major tasks that can be carried out within a physical workplace.
follow the steps to eidt Blue Sky Application on G Suite
- move toward Google Workspace Marketplace and Install CocoDoc add-on.
- Select the file and Hit "Open with" in Google Drive.
- Moving forward to edit the document with the CocoDoc present in the PDF editing window.
- When the file is edited completely, share it through the platform.
PDF Editor FAQ
Why did Rami Sivan convert to Hinduism?
Conversion is a process whereby one rejects and leaves one BS to join another BS (Belief System). It is only applicable to the monotheisms.Monotheism is essential a club with exclusive membership and is a closed intellectual system, hedged in and delimited by dogmatic borders and creeds. Conversion is therefore taking out membership in the group and subscribing to the BS and dogmas and rejecting all other forms of spirituality.In Hinduism there is no “conversion” there is only adoption. So when one adopts Hinduism one doesn’t leave one club to join another - one achieves liberation and freedom from all limits and constraints of thought, all borders are broken.As a Hindu one can dance with the dervishes, chant with the Krishnas, bow with the Muslims or take ayahuasca with Peruvian Shamans or meditate with the Theravadins. One is free to explore every form of spirituality without guilt, and one is free to join and associate with all sincere seekers and practitioners.Instead of gobbling with the turkeys in one pen or the other, one soars into the blue sky with the eagles. This why Garuda is emblematic of the Dharma.
Is the Delhi government's decision to allow private vehicles with even/odd number plates to run on alternative days (to control pollution) correct?
I won't say that this is a correct decision or not.. I will just share what i found and you as a rational person can decide yourself !Beijing Tried The #OddEvenFormula For Two Weeks And Here’s What Happened Next.............It took just two weeks.On August 20th, Beijing put restrictions on factory production and car use. Five million cars were forced to drive on alternating days (the odd even formula that you have been hearing so much about) leading up to the 70th anniversary of Japan’s WWII defeat on September 3 so that the city’s usually smoggy skies would be a picture-perfect blue -- the things we do for the sake of propaganda.This photo shows two images of the view from CNN's Beijing Bureau, one of a blue sky day from a week before the military parade and one of a hazy sky the day after the parade. Image credit: CNN/Twitter.Beijing needed to look good for the world and perhaps not even the authorities could predict what would happened next. The authorities had also shut down hundreds of factories during this time.And what do you know... it worked.On the morning of the parade, the air quality index (AQI) -- an international standard for measuring the severity of air pollution -- dipped to a pristine 17 out of 500, signifying very healthy air. And the skies were crystal clear.Image credit: CNN/Twitter.Then, they lifted the restrictions. And almost immediately, the smog returned. The blue skies nicknamed “parade blue” by netizens disappeared.Toward the end of November, the air quality worsened to an official reading of 611 parts per million, which is 111 points greater than the maximum number on the scale of measurement.The World Health Organization’s scale doesn’t go above 500ppm, and they say that any reading over 300ppm is hazardous. Delhi is also above 300ppm on an average -- not as bad as Beijing but bad enough to make us all worry and very sick.Tiananmen Square during a heavily polluted day on December 1, 2015 (LOWER) and two days later when the smog cleared on December 3 (TOP) in Beijing. AFPSo before we start criticising Kejriwal and the AAP government's decision to impose the #OddEvenFormula, let's take a moment to just think what it could mean for the children and the citizens of the city.It isn't that difficult -- we just have to travel by bus or metro (are we too elite to do that?) -- and as Beijing has shown, we too can see the blue skies.Maybe a glimpse of a smog, pollution free Delhi will change how we think for the better. At least, we need to give the #OddEvenFormula a chance.Is this how you want Delhi to look? Image credit: AFP
How practically relevant are classical machine learning algorithms like SVMs or decision trees as compared to deep learning?
Your question is extremely important, and vital to answer and clarify. As someone who has spent 30+ years doing ML and AI, this is the one question I am constantly dealing with, especially from students, who sometimes think we can now throw away all the hard earned knowledge of the past 50–100 years, in the mistaken belief that deep learning is the one “magic” solution that we’ve all been waiting or, and now that it’s here, we can ignore the previous work.I mean, who wants to work hard to understand difficult material, like linear algebra, multivariate statistics, convex optimization, and more esoteric math. Just collect data, run TensorFlow or some other package, and presto, you’ve solved any problem in ML. If you believe that, I have some real estate in Florida I’d like to sell you :-).I’ll let you on a “secret” that should be more widely known. For all the “hoopla” over the “successes” of deep learning, when it comes down to the “bread and butter” projects in the tech industry — I mean core revenue generating businesses that actually make money, and fund the rest of the “escapades” into deep (reinforcement) learning on Atari video games and similar Blue Sky projects — what gets used each and every day at most tech companies, to the best of my knowledge, from talking to engineers who actually work on such projects, is good old fashioned statistics and ML, not deep learning.Bandit algorithms, decision trees, logistic regression, linear programming, random forests, linear statistical models, and SVMs, in short, are the foundations for revenue generation. Take these away, and the tech industry would probably collapse overnight, since the revenue from the ad generation business would come to a halt. The reasons should not be surprising to anyone. As an analogy, I think the Nobel-prize winning physicist and director of Fermi Labs in Illinois once said that if the laws of physics stopped working for one microsecond, the US economy would collapse instantly.As the inventor of SVMs, Vapnik, said most notably, “There’s nothing more practical than a good theory”. Or, as the late Nobel prize winning physicist Richard Feynman, concluded in his appendix to the Challenger disaster report commissioned by the U.S. Congress: “Nature cannot be fooled”, and public relations must eventually give way to solid engineering and physics. Classical methods, like bandit algorithms, nearest neighbor, decision trees, logistic regression, random forests and ensemble methods like boosting, are based on a solid foundation of statistical theory. They work! Consequently, they get used in the nerve center projects in the tech industry, i.e. where the real dollars come in. No one wants to ultimately mess with the “goose that’s laying the golden eggs”, to use a metaphor.As for deep learning, it is certainly an extremely promising area, and has shown some remarkable performance in selected tasks. As further research continues, it will get better understood, and eventually will morph into some theoretically well understood approach based on nonlinear optimization and classical statistics. Such insights are already flowing in on models like GANs, where the initial approach was very general, but as anyone who has worked on GANs knows, they don’t converge, and in fact, theoretically can be shown to be divergent. But, special cases are emerging, where some proofs of convergence can be established. My PhD student Ian Gemp just published such a study on Arxiv, a long and difficult 50+ paper showing perhaps for the first time, a global convergence result on a subclass of GANs.[1808.01531] Global Convergence to the Equilibrium of GANs using Variational InequalitiesWork like this will eventually lead to GANs or some replacement of them being based on a solid foundation of math and engineering, and such a transformed model might become as much of a workhorse in the land of statistical generative models, as for example, mixture models currently are.However, it is extremely important to develop a realistic picture of where deep learning currently is, and be aware of its failures. As a simple example, take the latest version of MATLAB R2018b, which comes with a good deep learning package, advertised most prominently along with a short 5 line MATLAB program that “solves” the computer vision problem. Hook up a webcam to your computer, run the program, presto, it outputs a label that describes the visual scene from the webcam. I made the mistake of trying to demo this to my wife — a classically trained statistician — on how “promising” deep learning was in solving hard problems like computer vision. Well, guess what? I pointed the webcam to my living room, ran the program, and the deep learning program said “Barbershop”! Well, you can guess what my wife’s reaction was. If you tried to market a home robot that used such software, I can imagine the chaos that might result. Such a robot would serve as a nice entertainment device, when it constantly mislabels objects and, as one deep learning study showed, mislabeled a dog as a desktop PC. But, it would not be a reliable approach based on solid engineering.To me, this failure is not surprising at all, since the MATLAB deep learning program simply calls an Imagenet trained network, like Alexnet or VGG, and there’s absolutely no way that such a program can work as a universal visual recognition device. Further tests showed the program utterly failed to recognize most objects, and only with great care — showing it a coffee cup in a canonical orientation and good lighting — would it produce the right label.Software like MATLAB’s package are showing up all over the place — Mathematica has a similar feature — and in my experience, they all have the same high failure rate. Indeed, from all the 30+ years of training I have in ML, including having read hundreds of deep learning papers, programmed extensively using several deep learning packages, built 5+ GPU machines by hand from scratch, I am not surprised by this outcome. It’s what I would expect. If you train on one dataset, using deep learning, you are almost certainly overfitting to that dataset, and there’s no way it’s going to work in the real world on data that has a very different distribution from the training dataset. In short, transfer learning is the hard problem here, and standard deep learning architectures don’t address this problem. There is, of course, deep learning approaches that try to address this, but they are still a work in progress.The problem of computer vision is a challenging one, and it will not get solved so easily. As the saying goes, “a problem worthy of attack proves its worth by hitting back”. Human vision took millions of years of evolution to perfect, and a huge amount of neural hardware — practically half your cortex or 50 billions neurons by some accounts — is devoted to vision. It’s not going to get solved by rigging up a 1000+ layer deep learning network, when we don’t understand in a fundamental way what the submodules of this network are doing. There is a great deal of knowledge about how vision is implemented in the brain. It’s the one area where amazing progress has been made (e.g., the brain uses different pathways for computing “what” and “where”, for motion and color, and so on), and few of these insights are actually reflected in the deep learning solutions I have seen.Now, I’m sure that deep learning does get used in some areas, e.g. to drive speech recognition by Alexa or Siri, and for some visual content search and related problems. So, it’s not that deep learning is not used — it surely is. But, the hysteria that is created around this field, that somehow we can forget all the hard work that statisticians have done for over a century, and ML’ers have worked hard over the past 40 years to perfect, is pure propaganda, sadly encouraged by overzealous media reporting of selective blue sky projects by some tech industry research divisions, where in fact no real work on applications of ML takes place.These blue sky tech industry research divisions, I’m sad to say, are a temporary phenomenon. No one has the deep pockets to endlessly fund research in industry that does not pay off at some point. There were the heady days of Bell Telephone Laboratories and IBM Research, where basic discoveries like the invention of the transistor, or background radiation from the Big Bang, were made, resulting in many Nobel prizes being awarded to Bell Lab or IBM scientists. These projects were based on a solid foundation of physics and math, but were pure curiosity driven research. There’s absolutely a need for such research, but for better or worse, such projects are getting harder to do in academia. Having written many proposals to the National Science Foundation, basic research in AI or ML that does not address some real-world challenge, is very hard to get funded.Ultimately, the solution to challenging AI problems will have to be based on solid advances in engineering and math and physics, and I for one am confident that eventually deep learning will become transformed into a solid scientific field. That day, however, is not here yet.
- Home >
- Catalog >
- Finance >
- Application Form >
- Credit Card Application Template >
- credit application form pdf >
- Blue Sky Application