A Comprehensive Guide to Editing The Sample Workshop - Team-Based Learning Collaborative
Below you can get an idea about how to edit and complete a Sample Workshop - Team-Based Learning Collaborative in seconds. Get started now.
- Push the“Get Form” Button below . Here you would be taken into a webpage that allows you to make edits on the document.
- Select a tool you need from the toolbar that appears in the dashboard.
- After editing, double check and press the button Download.
- Don't hesistate to contact us via [email protected] if you need some help.
The Most Powerful Tool to Edit and Complete The Sample Workshop - Team-Based Learning Collaborative
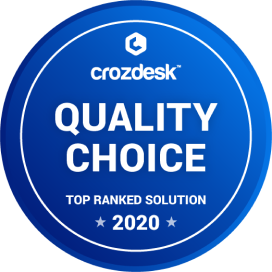
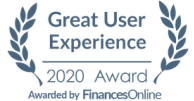
A Simple Manual to Edit Sample Workshop - Team-Based Learning Collaborative Online
Are you seeking to edit forms online? CocoDoc has got you covered with its detailed PDF toolset. You can accessIt simply by opening any web brower. The whole process is easy and quick. Check below to find out
- go to the free PDF Editor Page of CocoDoc.
- Import a document you want to edit by clicking Choose File or simply dragging or dropping.
- Conduct the desired edits on your document with the toolbar on the top of the dashboard.
- Download the file once it is finalized .
Steps in Editing Sample Workshop - Team-Based Learning Collaborative on Windows
It's to find a default application capable of making edits to a PDF document. Luckily CocoDoc has come to your rescue. Take a look at the Manual below to know how to edit PDF on your Windows system.
- Begin by adding CocoDoc application into your PC.
- Import your PDF in the dashboard and make edits on it with the toolbar listed above
- After double checking, download or save the document.
- There area also many other methods to edit PDF for free, you can read this article
A Comprehensive Handbook in Editing a Sample Workshop - Team-Based Learning Collaborative on Mac
Thinking about how to edit PDF documents with your Mac? CocoDoc can help.. It enables you to edit documents in multiple ways. Get started now
- Install CocoDoc onto your Mac device or go to the CocoDoc website with a Mac browser. Select PDF file from your Mac device. You can do so by hitting the tab Choose File, or by dropping or dragging. Edit the PDF document in the new dashboard which encampasses a full set of PDF tools. Save the content by downloading.
A Complete Guide in Editing Sample Workshop - Team-Based Learning Collaborative on G Suite
Intergating G Suite with PDF services is marvellous progess in technology, with the potential to cut your PDF editing process, making it easier and more cost-effective. Make use of CocoDoc's G Suite integration now.
Editing PDF on G Suite is as easy as it can be
- Visit Google WorkPlace Marketplace and search for CocoDoc
- establish the CocoDoc add-on into your Google account. Now you are in a good position to edit documents.
- Select a file desired by pressing the tab Choose File and start editing.
- After making all necessary edits, download it into your device.
PDF Editor FAQ
How can I become a data scientist?
Become a Data Scientist by Doing Data ScienceThe best way to become a data scientist is to learn - and do - data science. There are a many excellent courses and tools available online that can help you get there.Here is an incredible list of resources compiled by Jonathan Dinu, Co-founder of Zipfian Academy, which trains data scientists and data engineers in San Francisco via immersive programs, fellowships, and workshops.EDIT: I've had several requests for a permalink to this answer. See here: A Practical Intro to Data Science from Zipfian AcademyEDIT2: See also: "How to Become a Data Scientist" on SlideShare: http://www.slideshare.net/ryanorban/how-to-become-a-data-scientistEnvironmentPython is a great programming language of choice for aspiring data scientists due to its general purpose applicability, a gentle (or firm) learning curve, and — perhaps the most compelling reason — the rich ecosystem of resources and libraries actively used by the scientific community.DevelopmentWhen learning a new language in a new domain, it helps immensely to have an interactive environment to explore and to receive immediate feedback. IPython provides an interactive REPL which also allows you to integrate a wide variety of frameworks (including R) into your Python programs.STATISTICSData scientists are better at software engineering than statisticians and better at statistics than any software engineer. As such, statistical inference underpins much of the theory behind data analysis and a solid foundation of statistical methods and probability serves as a stepping stone into the world of data science.CoursesedX: Introduction to Statistics: Descriptive Statistics: A basic introductory statistics course.Coursera Statistics, Making Sense of Data: A applied Statistics course that teaches the complete pipeline of statistical analysisMIT: Statistical Thinking and Data Analysis: Introduction to probability, sampling, regression, common distributions, and inference.While R is the de facto standard for performing statistical analysis, it has quite a high learning curve and there are other areas of data science for which it is not well suited. To avoid learning a new language for a specific problem domain, we recommend trying to perform the exercises of these courses with Python and its numerous statistical libraries. You will find that much of the functionality of R can be replicated with NumPy, @SciPy, @Matplotlib, and @Python Data Analysis LibraryBooksWell-written books can be a great reference (and supplement) to these courses, and also provide a more independent learning experience. These may be useful if you already have some knowledge of the subject or just need to fill in some gaps in your understanding:O'Reilly Think Stats: An Introduction to Probability and Statistics for Python programmersIntroduction to Probability: Textbook for Berkeley’s Stats 134 class, an introductory treatment of probability with complementary exercises.Berkeley Lecture Notes, Introduction to Probability: Compiled lecture notes of above textbook, complete with exercises.OpenIntro: Statistics: Introductory text book with supplementary exercises and labs in an online portal.Think Bayes: An simple introduction to Bayesian Statistics with Python code examples.MACHINE LEARNING/ALGORITHMSA solid base of Computer Science and algorithms is essential for an aspiring data scientist. Luckily there are a wealth of great resources online, and machine learning is one of the more lucrative (and advanced) skills of a data scientist.CoursesCoursera Machine Learning: Stanford’s famous machine learning course taught by Andrew Ng.Coursera: Computational Methods for Data Analysis: Statistical methods and data analysis applied to physical, engineering, and biological sciences.MIT Data Mining: An introduction to the techniques of data mining and how to apply ML algorithms to garner insights.Edx: Introduction to Artificial Intelligence: Introduction to Artificial Intelligence: The first half of Berkeley’s popular AI course that teaches you to build autonomous agents to efficiently make decisions in stochastic and adversarial settings.Introduction to Computer Science and Programming: MIT’s introductory course to the theory and application of Computer Science.BooksUCI: A First Encounter with Machine Learning: An introduction to machine learning concepts focusing on the intuition and explanation behind why they work.A Programmer's Guide to Data Mining: A web based book complete with code samples (in Python) and exercises.Data Structures and Algorithms with Object-Oriented Design Patterns in Python: An introduction to computer science with code examples in Python — covers algorithm analysis, data structures, sorting algorithms, and object oriented design.An Introduction to Data Mining: An interactive Decision Tree guide (with hyperlinked lectures) to learning data mining and ML.Elements of Statistical Learning: One of the most comprehensive treatments of data mining and ML, often used as a university textbook.Stanford: An Introduction to Information Retrieval: Textbook from a Stanford course on NLP and information retrieval with sections on text classification, clustering, indexing, and web crawling.DATA INGESTION AND CLEANINGOne of the most under-appreciated aspects of data science is the cleaning and munging of data that often represents the most significant time sink during analysis. While there is never a silver bullet for such a problem, knowing the right tools, techniques, and approaches can help minimize time spent wrangling data.CoursesSchool of Data: A Gentle Introduction to Cleaning Data: A hands on approach to learning to clean data, with plenty of exercises and web resources.TutorialsPredictive Analytics: Data Preparation: An introduction to the concepts and techniques of sampling data, accounting for erroneous values, and manipulating the data to transform it into acceptable formats.ToolsOpenRefine (formerly Google Refine): A powerful tool for working with messy data, cleaning, transforming, extending it with web services, and linking to databases. Think Excel on steroids.Data Wrangler: Stanford research project that provides an interactive tool for data cleaning and transformation.sed - an Introduction and Tutorial: “The ultimate stream editor,” used to process files with regular expressions often used for substitution.awk - An Introduction and Tutorial: “Another cornerstone of UNIX shell programming” — used for processing rows and columns of information.VISUALIZATIONThe most insightful data analysis is useless unless you can effectively communicate your results. The art of visualization has a long history, and while being one of the most qualitative aspects of data science its methods and tools are well documented.CoursesUC Berkeley Visualization: Graduate class on the techniques and algorithms for creating effective visualizations.Rice University Data Visualization: A treatment of data visualization and how to meaningfully present information from the perspective of Statistics.Harvard University Introduction to Computing, Modeling, and Visualization: Connects the concepts of computing with data to the process of interactively visualizing results.BooksTufte: The Visual Display of Quantitative Information: Not freely available, but perhaps the most influential text for the subject of data visualization. A classic that defined the field.TutorialsSchool of Data: From Data to Diagrams: A gentle introduction to plotting and charting data, with exercises.Predictive Analytics: Overview and Data Visualization: An introduction to the process of predictive modeling, and a treatment of the visualization of its results.ToolsD3.js: Data-Driven Documents — Declarative manipulation of DOM elements with data dependent functions (with Python port).Vega: A visualization grammar built on top of D3 for declarative visualizations in JSON. Released by the dream team at Trifacta, it provides a higher level abstraction than D3 for creating “ or SVG based graphics.Rickshaw: A charting library built on top of D3 with a focus on interactive time series graphs.Modest Maps: A lightweight library with a simple interface for working with maps in the browser (with ports to multiple languages).Chart.js: Very simple (only six charts) HTML5 “ based plotting library with beautiful styling and animation.COMPUTING AT SCALEWhen you start operating with data at the scale of the web (or greater), the fundamental approach and process of analysis must change. To combat the ever increasing amount of data, Google developed the MapReduce paradigm. This programming model has become the de facto standard for large scale batch processing since the release of Apache Hadoop in 2007, the open-source MapReduce framework.CoursesUC Berkeley: Analyzing Big Data with Twitter: A course — taught in close collaboration with Twitter — that focuses on the tools and algorithms for data analysis as applied to Twitter microblog data (with project based curriculum).Coursera: Web Intelligence and Big Data: An introduction to dealing with large quantities of data from the web; how the tools and techniques for acquiring, manipulating, querying, and analyzing data change at scale.CMU: Machine Learning with Large Datasets: A course on scaling machine learning algorithms on Hadoop to handle massive datasets.U of Chicago: Large Scale Learning: A treatment of handling large datasets through dimensionality reduction, classification, feature parametrization, and efficient data structures.UC Berkeley: Scalable Machine Learning: A broad introduction to the systems, algorithms, models, and optimizations necessary at scale.BooksMining Massive Datasets: Stanford course resources on large scale machine learning and MapReduce with accompanying book.Data-Intensive Text Processing with MapReduce: An introduction to algorithms for the indexing and processing of text that teaches you to “think in MapReduce.”Hadoop: The Definitive Guide: The most thorough treatment of the Hadoop framework, a great tutorial and reference alike.Programming Pig: An introduction to the Pig framework for programming data flows on Hadoop.PUTTING IT ALL TOGETHERData Science is an inherently multidisciplinary field that requires a myriad of skills to be a proficient practitioner. The necessary curriculum has not fit into traditional course offerings, but as awareness of the need for individuals who have such abilities is growing, we are seeing universities and private companies creating custom classes.CoursesUC Berkeley: Introduction to Data Science: A course taught by Jeff Hammerbacher and Mike Franklin that highlights each of the varied skills that a Data Scientist must be proficient with.How to Process, Analyze, and Visualize Data: A lab oriented course that teaches you the entire pipeline of data science; from acquiring datasets and analyzing them at scale to effectively visualizing the results.Coursera: Introduction to Data Science: A tour of the basic techniques for Data Science including SQL and NoSQL databases, MapReduce on Hadoop, ML algorithms, and data visualization.Columbia: Introduction to Data Science: A very comprehensive course that covers all aspects of data science, with an humanistic treatment of the field.Columbia: Applied Data Science (with book): Another Columbia course — teaches applied software development fundamentals using real data, targeted towards people with mathematical backgrounds.Coursera: Data Analysis (with notes and lectures): An applied statistics course that covers algorithms and techniques for analyzing data and interpreting the results to communicate your findings.BooksAn Introduction to Data Science: The companion textbook to Syracuse University’s flagship course for their new Data Science program.TutorialsKaggle: Getting Started With Python For Data Science: A guided tour of setting up a development environment, an introduction to making your first competition submission, and validating your results.CONCLUSIONData science is infinitely complex field and this is just the beginning.If you want to get your hands dirty and gain experience working with these tools in a collaborative environment, check out our programs at http://zipfianacademy.com.There's also a great SlideShare summarizing these skills: How to Become a Data ScientistYou're also invited to connect with us on Twitter @zipfianacademy and let us know if you want to learn more about any of these topics.
What are some good institutes in Bangalore for Business Analyst course which provides placement as well?
In the world of internet, location is not a constraint.Keeping up with ever-evolving learning trends can be hard, as technology continuously evolves; online training is one way of ensuring you stay ahead of the game. Online training is one of the top learning trends and brings many benefits like-1) Relief from the exhaustion of commuting & the inconvenience of packed up batches.2) It will also provide you with the best & expert industry trainers from the global pool of industry experts.3) Collaborative sessions, sitting at your convenient place.Adaptive US is one of the world’s leading business analysis solutions organization based in the US which helps participants and organizations succeed in their quest for prestigious certifications from IIBA, Canada.Our training is Webex based online training where candidates from any part of the world can participate.Adaptive US is the only training organization to offer success guarantee and all-inclusive plans for its workshops.Our trainers are part of BABoK V3 team http://adaptiveus.com/our-experts/Go through our session feedback: - FeedbackSome facts of our training-1) The classes are virtual instructor led-live sessions over webex with classes being recorded.2) The classes are live and interactive, you can ask questions to the coach, see what he shares like presentation, templates and articles etc and listen to him.3) We provide reference materials like question banks, study guides, simulators, flashcards, audio books, techniques book, mind map, digital learning packs etc.We are proud to say that till now 99% of participants, who have appeared for CBAP after attending our CBAP Preparatory workshops have cleared the certification at the first attempt. We have certified 240+ CBAP professionals on BABoK V3 as of now.https://adaptiveus.com/cbap-certified-professionals/Register free for a trial account on our learning portal and access our sample study materials and exam tips from our recent certified candidates– AdaptiveUS.com/SuxeedWe are an IIBA Endorsed Certification Training Agency and we don’t provide placement services. Business Analysis certification is the fastest growing career today. Hence IIBA certifications will take you to greater heights in your career as these certifications are recognized worldwide.
Why is it that United States is so far behind in AI technology implementation compared to China?
Like Russia, China views AI as its future, which is to revolutionize everything, how we live, work, learn, think, discover, communicate, etc.Both put all the national resources on its development as the winning geopolitical super-weapon.“Whoever leads the global AI race rules the world”.American AI of machine learning is mostly a commercial profit-making technology spread among the G-MAFIA and speculative AI startups.In China, the BAT-Triada has all support of the public administration, which is to come with most advanced National Strategy of AI.Look at Trump’s Executive Order on Maintaining American Leadership in Artificial IntelligenceExecutive Order on Maintaining American Leadership in Artificial Intelligence | The White HouseAnd just compare the US AI strategies with the China’s AI strategies, as given below, differing as the sky and the earth.Strategy 1: Make long-term investments in AI research. Prioritize investments in the next generation of AI that will drive discovery and insight and enable the United States to remain a world leader in AI.Strategy 2: Develop effective methods for human-AI collaboration. Rather than replace humans, most AI systems will collaborate with humans to achieve optimal performance. Research is needed to create effective interactions between humans and AI systems.Strategy 3: Understand and address the ethical, legal, and societal implications of AI. We expect AI technologies to behave according to the formal and informal norms to which we hold our fellow humans. Research is needed to understand the ethical, legal, and social implications of AI, and to develop methods for designing AI systems that align with ethical, legal, and societal goals.Strategy 4: Ensure the safety and security of AI systems. Before AI systems are in widespread use, assurance is needed that the systems will operate safely and securely, in a controlled, well-defined, and well-understood manner. Further progress in research is needed to address this challenge of creating AI systems that are reliable, dependable, and trustworthy.Strategy 5: Develop shared public datasets and environments for AI training and testing.The depth, quality, and accuracy of training datasets and resources significantly affect AI performance. Researchers need to develop high quality datasets and environments and enable responsible access to high-quality datasets as well as to testing and training resources.Strategy 6: Measure and evaluate AI technologies through standards and benchmarks. . Essential to advancements in AI are standards, benchmarks, testbeds, and community engagement that guide and evaluate progress in AI. Additional research is needed to develop a broad spectrum of evaluative techniques.Strategy 7: Better understand the national AI R&D workforce needs. Advances in AI will require a strong community of AI researchers. An improved understanding of current and future R&D workforce demands in AI is needed to help ensure that sufficient AI experts are available to address the strategic R&D areas outlined in this plan.National Science and Technology Council Networking and Information Technology Research and Development Subcommitteehttps://www.nitrd.gov/PUBS/national_ai_rd_strategic_plan.pdfState Council Notice on the Issuance of the Next Generation Artificial Intelligence Development PlanReleased: July 20, 2017A Next Generation Artificial Intelligence Development PlanThe rapid development of artificial intelligence (AI) will profoundly change human society and life and change the world. To seize the major strategic opportunity for the development of AI, to build China’s first-mover advantage in the development of AI, to accelerate the construction of an innovative nation and global power in science and technology, in accordance with the requirements of the CCP Central Committee and the State Council, thisplan has been formulated.(3) Strategic ObjectivesThese are divided into the following three steps:First, by 2020, the overall technology and application of AI will be in step with globally advanced levels, the AI industry will have become a new important economic growth point, and AI technology applications will have become a new way to improve people’s livelihoods, strongly supporting [China’s] entrance into the ranks of innovative nations and comprehensively achieving the struggle toward the goal of a moderately prosperous society.● By 2020 China will have achieved important progress in a new generation of AI theories and technologies. It will have actualized important progress in big data intelligence, cross-medium intelligence, swarm intelligence, hybrid enhanced intelligence, and autonomous intelligence systems, and will have achieved important progress in other foundational theories and core technologies; the country will have achieved iconic advances in AI models and methods, core devices, high-end equipment, and foundational software.● The AI industry’s competitiveness will have entered the first echelon internationally.China will have established initial AI technology standards, service systems, and industrial ecological system chains. It will have cultivated a number of the world's leading AI backbone enterprises, with the scale of AI’s core industry exceeding 150 billion RMB, and exceeding 1 trillion RMB as driven by the scale of related industries.● The AI development environment will be further optimized, opening up new applications in important domains, gathering a number of high-level personnel and innovation teams, and initially establishing AI ethical norms, policies, and regulations in some areas.Second, by 2025, China will achieve major breakthroughs in basic theories for AI, such that some technologies and applications achieve a world-leading level and AI becomes the main driving force for China’s industrial upgrading and economic transformation, while intelligent social construction has made positive progress.● By 2025, a new generation of AI theory and technology system will be initially established, as AI with autonomous learning ability achieves breakthroughs in many areas to obtain leading research results.● The AI industry will enter into the global high-end value chain. This new-generation AI will be widely used in intelligent manufacturing, intelligent medicine, intelligent city, intelligent agriculture, national defense construction, and other fields, while the scale of AI’s core industry will be more than 400 billion RMB, and the scale of related industries will exceed 5 trillion RMB.● By 2025 China will have seen the initial establishment of AI laws and regulations, ethical norms and policy systems, and the formation of AI security assessment and control capabilities.Third, by 2030, China’s AI theories, technologies, and applications should achieve world leading levels, making China the world’s primary AI innovation center, achieving visible results in intelligent economy and intelligent society applications, and laying an important foundation for becoming a leading innovation-style nation and an economic power.● China will have formed a more mature new-generation AI theory and technology system. The country will achieve major breakthroughs in brain-inspired intelligence, autonomous intelligence, hybrid intelligence, swarm intelligence, and other areas, having important impact in the domain of international AI research and occupying the commanding heights of AI technology.● AI industry competitiveness will reach the world-leading level. AI should be expansively deepened and greatly expanded into production and livelihood, social governance, national defense construction, and in all aspects of applications, will become an expansive core technology for key systems, support platforms, and the intelligent application of a complete industrial chain and high-end industrial clusters, with AI core industry scale exceeding 1 trillion RMB, and with the scale of related industries exceeding 10 trillion RMB.● China will have established a number of world-leading AI technology innovation and personnel training centers (or bases), and will have constructed more comprehensive AI laws and regulations, and an ethical norms and policy system.III. Focus Tasks(1) Build open and coordinated AI science and technology innovation systemsFocus on increasing the supply of AI innovation sources; strengthen deployments in areas such as advanced basic theory, key general technologies, basic platforms, talent teams, etc.; stimulate open-source sharing; systematically enhance sustained innovation capabilities; ensure that our country's AI science and technology levels ascend to the leading global ranks; and make ever more contributions to the development of global AI.1. Establish basic theory systems for a new generation of AIBox 1: Basic Theories1. Big data intelligence theory. Research new data-driven and knowledge-driven AI methods, theories and methods for sensing computing theory with natural language understanding, images and figures at the core, comprehensive deep reasoning and creative AI theories and methods, basic theories and frameworks on smart decisionmaking with incomplete information, data-driven common AI data models and theories, etc.2. Cross-media sensing and computing theory. Research sensing that exceeds human visual abilities, active visual sensing and computing aimed at the real world, auditory sensing and computing of natural acoustic scenes, language sensing and computing in an environment of natural interaction, human sensing and computing aimed at asynchronous orders, autonomous learning aimed at smart media sensing, and urban omnidimensional smart sensing and reasoning engines.3. Hybrid and enhanced intelligence theory. Research hybridization and convergence where “the human is in the loop,” behavioral strengthening through human machine smart symbiosis and brain-machine coordination, intuitive machine reasoning and causal models, associative recall models and knowledge evolution methods, complex data and task blended and enhanced intelligence learning methods, cloud robotics coordination computing methods, and situational comprehension and human-machine group coordination in real-world environments.4. Swarm intelligence theory. Research swarm intelligence structural theory and organizational methods, swarm intelligence incentive mechanisms and emergence mechanisms, swarm intelligence learning theories and methods, common swarm intelligence computing paradigms and models.5. Autonomous coordination and control, and optimized decision-making theory.Research coordination sensing and interaction aimed at autonomous unmanned systems, coordination, control and optimized decision-making aimed at autonomous and unmanned systems, knowledge-driven human-machine-object triangular coordination and interoperability theories.6. High-level machine learning theory. Research basic statistical learning theories, reasoning and decision-making under uncertainty, distributed learning and interaction, learning while protecting privacy, small-sample learning, deep intensive learning, unsupervised learning, semi-supervised learning, active learning and other such learning theories and efficient models.7. Brain-inspired intelligence computing theory. Research theories and methods on brain-inspired sensing, brain-inspired learning, and brain-inspired recall mechanisms and computing blends, brain-inspired complex systems, brain-inspired control, etc.8. Quantum intelligent computing theory. Explore cognitive quantum models and intrinsic mechanisms, research efficient quantum intelligence models and algorithms, high-performance and high-bitrate quantum AI processors, real-time quantum AI systems that can exchange information with the outside world, etc.2. Build a next-generation AI key general technology systemBox 2: Key General Technologies1. Knowledge computing engines and knowledge service technology. Researching knowledge computing and visual interaction engines; researching innovative design, digital creation, and commercial intelligence with visual media at the core; developing large-scale organic data knowledge discovery.2. Cross-medium analytic reasoning technology. Researching cross-medium unified indicators, connected understanding and knowledge mining, knowledge map building and learning, knowledge evolution and inference, intelligent description and generation, etc., technology; developing cross-medium analytic reasoning engine and verification systems.3. Key swarm intelligence technology. Developing swarm intelligence's activeperception and discovery, knowledge gain and generation, cooperation and sharing, evaluation and evolution, human-machine integration and enhancement, selfpreservation and mutual security, etc., key technology studies; building service system architecture for the crowd intelligence space; researching mobile crowd intelligent coordinated decision making and control technologies.4. Hybrid enhanced intelligent new architectures and technologies. Researching hybrid enhanced intelligent core technology and cognitive computing frameworks; new model hybrid computing architectures, human-machine collective driving, online intelligent learning technology, and hybrid enhanced frameworks for simultaneous management and control.5. Autonomous unmanned systems intelligent technology. Researching unmanned autonomous control intelligent technology for automobiles, ships, traffic, automatic driving, etc.; service, space, maritime, and polar robot technology; unmanned workshop/intelligent factory intelligent technology; high-end intelligent control technology and autonomous unmanned operating systems. Researching positioning, navigation, recognition, etc., robotic and mechanical arm autonomous control technology for visual sensing in complex environments.6. Virtual reality intelligent modeling technology.Researching mathematical expression and modeling methods for virtual counterpart intelligent behavior; problems such as natural, persistent, and deep exchange between users and virtual counterparts and virtual environments; intelligent counterpart modeling technology and method systems.7. Intelligent computing chips and systems. Researching neural network processors, as well as high-energy efficiency, reconfigurable brain-inspired computing chips, etc.; new-model perception chips and systems, intelligent computing system structure and systems, and AI operating systems. Researching architectures suitable for AI hybrid architectures, etc.8. Natural language processing technology. Researching short text computing and analysis technology, cross-language text mining technology and turning toward semantic comprehension technology for machine cognitive intelligence, and human-machine interaction systems for multimedia information comprehension.3. Coordinate the layout of AI innovation platformsBox 3: Basic Support Platforms1. AI Open-Source Hardware and Software Infrastructure and Platforms. Establish big data and AI open-source software platforms, terminal, and cloud collaborative AI cloud service platforms, new multi-intelligent sensor and integrated platforms, new product design platforms based on AI hardware, and future network, big data intelligent service platforms.2. Group Intelligent Service Platforms. Establish group knowledge-based computing and support platforms, science and technology public service systems, group intelligent software development and verification automation systems, group intelligent software learning and innovation systems, open environment cluster decision-making systems, and group-sharing economic service systems.3. Hybrid Enhanced Intelligent Support Platforms. Establish AI supercomputing centers, large-scale super intelligent computing support environments, online intelligent education platforms, “human-in-the-loop” driving brains, intelligent platforms for complexity analyses and risk assessment in industrial development, intelligent security platforms to support nuclear power security operations, and research and development and testing platforms for human-machine joint driving technology.4. Autonomous Unmanned System Support Platforms. Establish common core technology and support platforms, independent unmanned systems, independent control of unmanned aerial vehicles, and automatic driving support platforms for auto, ship and rail traffic, service robots, space robots, marine robots, polar robot support platforms, technical support platforms for intelligent factory and intelligent control equipment, etc.5. AI Basic Data and Security Detection Platforms. Construct artificial data-oriented public data resource libraries, standard test data sets, and cloud service platforms.Establish test models and evaluation models for the security of AI algorithms and platforms. Research and develop security evaluation tools for AI algorithms and platforms.4. Accelerate the training and gathering of high-end AI talentBox 4: Intelligentized Infrastructure1. Network Infrastructure. Speed up the layout of real-time collaborative AI 5G enhanced technology research and the development and application of space oriented collaborative AI for the construction of high-precision navigation and positioning networks to strengthen the core of intelligent sensing technology research and key facilities. Develop intelligent industrial support, driving networks, etc., to study the intelligent network security architecture. Speed up the construction of integrated information network for space and earth, promoting a space-based information network, the future of the Internet, mobile communication network of the full integration.2. Big Data Infrastructure. Rely on a national data sharing exchange platform, open data platform and other public infrastructure. Construct governance, public services, industrial development, technology research and development, and other fields of big data information databases Support the implementation of national governance data applications. Integrate various types of social data platforms and data center resources. Create nationwide integrated service capabilities with reasonable layout and linkages.3. High-performance computing infrastructure. Continue to strengthen thesupercomputing infrastructure, distributed computing infrastructure and cloud computing center construction. Build sustainable development of highperformance computing application for the ecological environment. Promote the next generation of supercomputer research and development and applications.(6) Plan a new generation of AI major science and technology projectsFor the development of China’s AI needs and weak links, establish of a new generation of AI major scientific and technological projects. Strengthen the overall co-ordination, clear the boundaries of the tasks and the focus of research and development. Form a new generation of AI major scientific and technological projects as the core, and use existing R&D layout to support the “1 + N” AI program.“1” refers to a new generation of AI scientific and technological mega-projects, focusing on forward-looking layout for basic theories and key common technologies, including the study of big data intelligence, cross-media perception and computing, hybrid enhanced intelligence, group intelligence, autonomous collaborative control, and decision-making theory. Research knowledge computing engines and knowledge service technologies,cross-medium analysis reasoning technology, key swarm intelligence technologies, new architecture and new technology for hybrid enhanced intelligent, autonomous unmanned control technology, and basic theory and common technology for open-source shared AI.Continue to carry out the development of AI prediction and research, strengthening the economic and social impact of and countermeasures for AI.“N” refers to the national planning and deployment of AI research and development projects. Focusing on strengthening the new generation of AI with the convergence major scientific and technological projects, collaborative impetus for research, technological breakthroughs and product development applications.Strengthen the convergence of major national science and technology projects.Support AI hardware and software development in the “Hegaoji” Megaproject,1 integrated circuit equipment and other national science and technology major projects.Strengthen mutual support for AI and other “Technological Innovation 2030 - Mega-Projects.” Accelerate the use of AI to provide support for major technical breakthroughs in brain science and brain computing, quantum information and quantum computing, intelligent manufacturing and robotics, and big data research.The National Key Research and Development Plan will continue to promote high performance computing and other key special applications, while increasing support for AI related technology research and development and application; the National Natural Science Foundation will strengthen cross-disciplinary research and support for free exploration in the field of AI. Focus on special deployment and strengthen the application of AI technology demonstrations to the deep sea space station, health protection, and other major projects, smart cities, intelligent agricultural equipment and other Key National R&D Projects. Support the openness and sharing of research results on basic theory of AI and common technology through other basic science and technology plans.Innovate in the organization and implementation of models for new generation AI major scientific and technological projects. Adhere to focus on doing things, focusing on the principle of breakthrough. Give full play to the role of market mechanisms to mobilize departments, local, business and social forces to promote the implementation of all aspects.Pursue clear management responsibility, regular assessments, to strengthen the dynamic adjustments and improve management efficiency...A Next Generation Artificial Intelligence Development Plan
- Home >
- Catalog >
- Miscellaneous >
- Evaluation Form >
- Peer Evaluation >
- peer evaluation examples >
- Sample Workshop - Team-Based Learning Collaborative