How to Edit The Rasch Scaling Of Different Forms Of Thought And Different Logical conviniently Online
Start on editing, signing and sharing your Rasch Scaling Of Different Forms Of Thought And Different Logical online refering to these easy steps:
- Click on the Get Form or Get Form Now button on the current page to direct to the PDF editor.
- Give it a little time before the Rasch Scaling Of Different Forms Of Thought And Different Logical is loaded
- Use the tools in the top toolbar to edit the file, and the added content will be saved automatically
- Download your edited file.
The best-reviewed Tool to Edit and Sign the Rasch Scaling Of Different Forms Of Thought And Different Logical
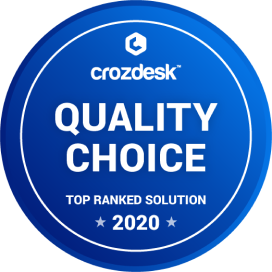
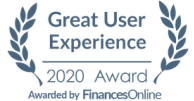
Start editing a Rasch Scaling Of Different Forms Of Thought And Different Logical now
Get FormA simple direction on editing Rasch Scaling Of Different Forms Of Thought And Different Logical Online
It has become really easy nowadays to edit your PDF files online, and CocoDoc is the best tool you have ever used to make some changes to your file and save it. Follow our simple tutorial to start!
- Click the Get Form or Get Form Now button on the current page to start modifying your PDF
- Create or modify your text using the editing tools on the top toolbar.
- Affter changing your content, add the date and make a signature to complete it.
- Go over it agian your form before you click and download it
How to add a signature on your Rasch Scaling Of Different Forms Of Thought And Different Logical
Though most people are accustomed to signing paper documents by handwriting, electronic signatures are becoming more accepted, follow these steps to sign documents online for free!
- Click the Get Form or Get Form Now button to begin editing on Rasch Scaling Of Different Forms Of Thought And Different Logical in CocoDoc PDF editor.
- Click on Sign in the tool box on the top
- A popup will open, click Add new signature button and you'll be given three choices—Type, Draw, and Upload. Once you're done, click the Save button.
- Drag, resize and position the signature inside your PDF file
How to add a textbox on your Rasch Scaling Of Different Forms Of Thought And Different Logical
If you have the need to add a text box on your PDF for making your special content, follow these steps to accomplish it.
- Open the PDF file in CocoDoc PDF editor.
- Click Text Box on the top toolbar and move your mouse to drag it wherever you want to put it.
- Write down the text you need to insert. After you’ve filled in the text, you can actively use the text editing tools to resize, color or bold the text.
- When you're done, click OK to save it. If you’re not satisfied with the text, click on the trash can icon to delete it and start over.
A simple guide to Edit Your Rasch Scaling Of Different Forms Of Thought And Different Logical on G Suite
If you are finding a solution for PDF editing on G suite, CocoDoc PDF editor is a recommended tool that can be used directly from Google Drive to create or edit files.
- Find CocoDoc PDF editor and establish the add-on for google drive.
- Right-click on a PDF file in your Google Drive and click Open With.
- Select CocoDoc PDF on the popup list to open your file with and allow access to your google account for CocoDoc.
- Edit PDF documents, adding text, images, editing existing text, highlight important part, retouch on the text up in CocoDoc PDF editor and click the Download button.
PDF Editor FAQ
How do I convert an interval scale to a ratio scale?
The difference between interval and ratio scales comes from their ability to dip below zero. Interval scales hold no true zero and can represent values below zero. For example, you can measure temperature below 0 degrees Celsius, such as -10 degrees. Ratio variables, on the other hand, never fall below zero.One way to transform ordinal level data into interval scale is to use some kind of Item Responsemodel. A well-known example is the Rasch model, which extends the idea of the parallel test model from classical test theory to cope with binary-scored items through a generalized (with logit link) mixed-effect linear model (in some of the 'modern' software implementation), where the probability of endorsing a given item is a function of 'item difficulty' and 'person ability' (assuming there's no interaction between one's location on the latent trait being measured and item location on the same logit scale--which could be captured through an additional item discrimination parameter, or interaction with individual-specific characteristics--which is called differential item functioning). The underlying construct is assumed to be unidimensional, and the logic of the Rasch model is just that the respondent has a certain 'amount of the construct'--let's talk about subject's liability (his/her 'ability'), and call it θθ, as does any item that defines this construct (their 'difficulty'). What is of interest is the difference between respondent location and item location on the measurement scale, θθ. To give a concrete example, consider the following question: "I found it hard to focus on anything other than my anxiety" (yes/no). A person suffering from anxiety disorders is more likely to answer positively to this question compared to a random individual taken from the general population and having no past history of depression or anxiety-related disorder.An illustration of 29 item response curves derived from a large-scale US study that aims to build a calibrated item bank assessing anxiety-related disorders(1,2) is shown below. The sample size is N=766N=766; exploratory factor analysis confirmed the unidimensionality of the scale (with first eigenvalue largely above the second eigenvalue (by a 17-fold amount), and unreliable 2nd factor axis (eigenvalue juste above 1) as confirmed by parallel analysis), and this scale shows reliability index in the acceptable range, as assessed by Cronbach's alpha (α=0.971α=0.971, with 95% bootstrap CI [0.967;0.975][0.967;0.975]). Initially, five response categories were proposed (1 = 'Never', 2 = 'Rarely', 3 = 'Sometimes', 4 = 'Often', and 5 = 'Always') for each item. We will here only consider binary-scored responses.(Here, responses to Likert-type items have been recoded as binary responses (1/2=0, 3-5=1), and we consider that each item is equally discriminative across individuals, hence the parallelism between item curve slopes (Rasch model).)As can be seen, people located to the right of the xx-axis, which reflects the latent trait (anxiety), who are thought to express more of this trait are more likely to answer positively to questions like "I felt terrified" (terrific) or "I had sudden feelings of panic" (panic) than people located to the left (normal population, unlikely to be considered as cases); on the other hand, it is not unlikely than someone from the general population would report having trouble to get asleep (sleeping): for someone located at an intermediate range of the latent trait, say 0 logits, his/her probability of scoring 3 or higher is about 0.5 (which is the item difficulty).For polytomous items with ordered categories, there are several choices: the partial credit model, the rating scale model, or the graded response model, to name but a few that are mostly used in applied research. The first two belong to the so-called "Rasch family" of IRT models and share the following properties: (a) monotonicity of the response probability function (item/category response curve), (b)sufficiency of the total individual score (with latent parameter considered as fixed), (c) local independence meaning that responses to items are independent, conditional on the latent trait, and (d) absence of differential item functioning meaning that, conditional on the latent trait, responses are independent of external individual-specific variables (e.g., gender, age, ethnicity, SES).Extending the previous example to the case where the five response categories are effectively accounted for, a patient will have a higher probability of choosing response category 3 to 5, compared to someone sampled from the general population, without any antecedent of anxiety-related disorders. Compared to the modelling of dichotomous item described above, these models consider either cumulative (e.g., odds of answering 3 vs. 2 or less) or adjacent-category threshold (odds of answering 3 vs. 2), which is also discussed in Agresti's Categorical Data Analysis (chapter 12). The main difference between the aforementioned models lies in the way transitions from one response category to the other are handled: the partial credit model does not assume that difference between any given threshold location and the mean of the threshold locations on the latent trait is equal or uniform across items, contrary to the rating scale model. Another subtle difference between those models is that some of them (like the unconstrained graded response or partial credit model) allows for unequal discrimination parameters between item. See Applying item response theory modelling for evaluating questionnaire item and scale properties, by Reeve and Fayers, or The basis of item response theory, by Frank B. Baker, for more details.Because in the preceding case we discussed the interpretation of responses probability curves for dichotomously scored items, let's look at item response curves derived from a graded response model, highlighting the same target items:(Unconstrained graded response model, allowing for unequal discrimination among items.)Here, the following observations deserve some consideration:Response categories for the 'sleeping' item are less discriminative than, say, the ones attached to 'terrific': in the case of 'sleeping', for two persons located at the two extrema of the interval [2;2.5][2;2.5] on the latent trait (in logit units), their probability of choosing the fourth response ("often had difficulty sleeping") goes from approx. 0.35 to 0.4; with 'terrific', that probability goes from less than 0.1 to about 0.25 (dashed blue line). If you want to discriminate between two patients showing signs of anxiety, the latter item is more informative.There is an overall shift, from the left to the right, between item assessing sleep quality and those assessing more severe conditions, although sleeping disorders are not uncommon. This is expected: after all, even people in the general population might experience some difficulty falling asleep, independent of their health state, and people severely depressed or anxious are likely to exhibit such problems. However, 'normal persons' (if this ever had any meaning) are unlikely to show some signs of panic disorder (the probability they choose the highest response category is zero for people located up to the intermediate-range or more of the latent trait, [0;1]).In both cases discussed above, this θθ scale which reflects individual liability on the assumed latent trait has the property of an interval scale.Besides being thought of as truly measurement models, what makes Rasch models attractive is that sum scores, as a sufficient statistic, can be used as surrogates for the latest scores. Moreover, the sufficiency property readily implies the separability of model (persons and items) parameters (in the case of polytomous items, one should not forget that everything applies at the level of item response category), hence conjoint additivity.A good review of IRT model hierarchy, with R implementation, is available in Mair and Hatzinger's article published in the Journal of Statistical Software: Extended Rasch Modeling: The eRm Package for the Application of IRT Models in R. Other models include log-linear models, non-parametric model, like the Mokken model, or graphical models.Apart from R, I am not aware of Excel implementations, but several statistical packages were proposed on this thread: How to get started with applying item response theory and what software to use?Finally, if you want to study the relationships between a set of items and a response variable without resorting on a measurement model, some form of variable quantization through optimal scaling can be interesting too. Apart from R implementations discussed in those threads, SPSS solutions were also proposed on related threads
- Home >
- Catalog >
- Life >
- Musical Instrument Chart >
- Piano Notes Chart >
- Piano Course Notes >
- ker notes >
- Rasch Scaling Of Different Forms Of Thought And Different Logical