How to Edit Your An Application Of Probabilistic Matching Online Lightning Fast
Follow these steps to get your An Application Of Probabilistic Matching edited for the perfect workflow:
- Click the Get Form button on this page.
- You will be forwarded to our PDF editor.
- Try to edit your document, like adding text, inserting images, and other tools in the top toolbar.
- Hit the Download button and download your all-set document for the signing purpose.
We Are Proud of Letting You Edit An Application Of Probabilistic Matching Seamlessly
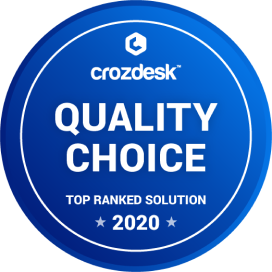
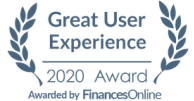
How to Edit Your An Application Of Probabilistic Matching Online
When dealing with a form, you may need to add text, Add the date, and do other editing. CocoDoc makes it very easy to edit your form into a form. Let's see the simple steps to go.
- Click the Get Form button on this page.
- You will be forwarded to this PDF file editor web app.
- In the the editor window, click the tool icon in the top toolbar to edit your form, like checking and highlighting.
- To add date, click the Date icon, hold and drag the generated date to the field to fill out.
- Change the default date by modifying the date as needed in the box.
- Click OK to ensure you successfully add a date and click the Download button for the different purpose.
How to Edit Text for Your An Application Of Probabilistic Matching with Adobe DC on Windows
Adobe DC on Windows is a must-have tool to edit your file on a PC. This is especially useful when you have need about file edit in the offline mode. So, let'get started.
- Click and open the Adobe DC app on Windows.
- Find and click the Edit PDF tool.
- Click the Select a File button and select a file to be edited.
- Click a text box to optimize the text font, size, and other formats.
- Select File > Save or File > Save As to keep your change updated for An Application Of Probabilistic Matching.
How to Edit Your An Application Of Probabilistic Matching With Adobe Dc on Mac
- Browser through a form and Open it with the Adobe DC for Mac.
- Navigate to and click Edit PDF from the right position.
- Edit your form as needed by selecting the tool from the top toolbar.
- Click the Fill & Sign tool and select the Sign icon in the top toolbar to make a signature for the signing purpose.
- Select File > Save to save all the changes.
How to Edit your An Application Of Probabilistic Matching from G Suite with CocoDoc
Like using G Suite for your work to finish a form? You can integrate your PDF editing work in Google Drive with CocoDoc, so you can fill out your PDF to get job done in a minute.
- Integrate CocoDoc for Google Drive add-on.
- Find the file needed to edit in your Drive and right click it and select Open With.
- Select the CocoDoc PDF option, and allow your Google account to integrate into CocoDoc in the popup windows.
- Choose the PDF Editor option to move forward with next step.
- Click the tool in the top toolbar to edit your An Application Of Probabilistic Matching on the target field, like signing and adding text.
- Click the Download button to keep the updated copy of the form.
PDF Editor FAQ
What are some real life applications of dynamic programming?
WASP (Winning and Score Predictor)Those who follow the game of cricket may have seen it in Sky Sports telecast of matches taken place in New Zealand. Invented in the University of Canterbury, it's a probabilistic tool to predict score and outcome of a match based on various factors.From Wikipedia:The WASP system is grounded in the theory of dynamic programming. It looks at data from past matches and estimates the probability of runs and wickets in each game situation, and works backwards to calculate the total runs or probability of winning in any situation.This is how Dr Seamus Hogan – one of the creators of WASP – described the system:Let V(b,w) be the expected additional runs for the rest of the innings when b (legitimate) balls have been bowled and w wickets have been lost, and let r(b,w) and p(b,w) be, respectively, the estimated expected runs and the probability of a wicket on the next ball in that situation.We can then write,Since V(b*,w)=0 where b* equals the maximum number of legitimate deliveries allowed in the innings (300 in a 50 over game), we can solve the model backwards.This means that the estimates for V(b,w) in rare situations depends only slightly on the estimated runs and probability of a wicket on that ball, and mostly on the values of V(b + 1,w) and V(b + 1,w + 1), which will be mostly determined by thick data points.The second innings model is a bit more complicated, but uses essentially the same logic.
What are some of the must-have features in an Master Data Management (MDM) product?
Based on Industry requirements, some of the must-have features in MDM product are -Flexible data model - to map varied master data across business unitsProbabilistic Match & Merge algorithm to create Golden Record of master dataIntuitive Workflow based Data Stewardship User InterfaceStandard communication interfaces (Web Services, APIs) - to integrate with Governance tools, ETL tools, Middleware tools (ESB and MQ) and Analytical toolsExpose pluggable points - to insert custom business rules and validationsStrong support to Audit requirements - in terms of who accessed what and when, version historySecurity - in terms of user authentication at application level and transaction based authorization, which user can access what data attribute and recordsEasy to deploy incremental changes without the necessity to stop MDM application
What are the most significant machine learning advances in 2017?
The most significant advance for me is some clarity on the limitations of deep learning.Other than that, I am primarily interested in applications of machine learning, which is what colors my preferences in the following list.Domain knowledgeThe breakthrough of the decade which changed the face of machine learning happened 5 years ago with Alex Krizhevsky’s NIPS 2012 paper [0], reviving deep learning.Over the past 5 years, most advances involved grabbing the low hanging fruits that Krizhevsky’s paper exposed: applying DL to problem X**.With performance on such problems saturating, the most significant trend in 2017 for me is the slow return of domain knowledge [1,2,3,4,5,6,26]. We have examined how far entirely data-driven approaches could take us, and its becoming clear that data alone won’t take us all the way [7] to solving many of the relevant problems. So the next step is to incorporate strong priors into networks. This is challenging because unlike probabilistic graphical models, we need major new ideas to bake priors into convolutional networks.DL vs. (some) well-engineered systemsAnother, perhaps related, significant discovery for me is the clarity that DL may not even be able to beat some hand-engineered systems. Specifically, we saw multiple papers [8,9] this year that indicated that CNN learnt features for keypoint matching don’t outperform handcrafted ones. We also saw that end-to-end trained SLAM systems [10] don’t beat standard pipelines.Even a problem of visual recognition i.e. specific object instance detection so far doesn’t seem amenable to deep learning, and the state-of-the-art on the problem is still traditional pipelines [11,12].Memorization in deep neural networksOne major advance or confirmation in our understanding of how neural networks work came from [22] which showed that CNNs can even achieve zero training error on randomly labeled datasets indicating the massive memorization capability of these networks and raised questions on whether they are anything more than large locality sensitive hash tables! Fortunately, within the same year, and thanks to arXiv, we also got to see [23] which finds that in fact NN training is more sensible than that, and when actual patterns exist they are learnt first in a generalizable way before memorization happens.Convolutional LSTMs for navigationOne work I loved explicitly incorporates a map into a neural network is [21]. Obviously this is related to the earlier heading of incorporating domain knowledge into NNs. It eliminates the need for SLAM in navigation, and also seems biologically realistic - although it would be more impressive practically if it didn’t need ground truth odometry. While this paper [21] uses supervised learning, there is another work [28] that employs reinforcement learning to train a similar model.Conditional GANsI also found myself fascinated by applications of conditional GANs [13,14,15]. I think these models will be responsible for great things in the coming few years.3D scene datasetsAnother significant advance is recently proposed large scale datasets of 3D scenes [16,17,18]. ImageNet is still the largest computer vision dataset the community has, 7 years after its release; and its the dataset that had a big part in making Krizhevsky’s [0] paper succeed.But the real world isn’t just 2D internet images. These datasets are going to enable a host of new capabilities [19].CNNs for languageAnother significant advance is [20], which introduces a convolutional network that can compete with RNNs for language processing.RNNs have disappointed me in computer vision, and with this work it seems that in language too, they will get beaten thoroughly by CNNs.CNNs for oddly-structured dataTo my knowledge before 2017, CNNs were exclusively applied to volumetric representations when processing 3D data. [24,27] introduce an approach to interpreting point clouds directly.Finally, I also think graph convolutional networks[25] that allow applying all this deep learning machinery to graphs are going to be important.** Consequently, we saw researchers (i) approximate RL value functions using neural networks, (ii) replace traditional visual object detection, semantic segmentation, even low level tasks such as optical flow and stereo with CNNs, (iii) invent image captioning with LSTMs and CNNs, (iv) get language translation to exploit LSTMs and recently even CNNs, (v) get speech recognition deployed in large scale products amongst other applications.[1] https://arxiv.org/pdf/1705.00754.pdf[2] https://arxiv.org/pdf/1612.00380.pdf[3] https://arxiv.org/pdf/1612.02699.pdf[4] https://arxiv.org/pdf/1710.00489.pdf[5] https://arxiv.org/pdf/1703.06211.pdf[6] https://arxiv.org/pdf/1612.00404.pdf[7] [1707.02968] Revisiting Unreasonable Effectiveness of Data in Deep Learning Era[8] https://arxiv.org/pdf/1704.05939.pdf[9] https://www.researchgate.net/profile/Johannes_Schoenberger/publication/315788135_Comparative_Evaluation_of_Hand-Crafted_and_Learned_Local_Features/links/58e4f20145851547e17d4dee/Comparative-Evaluation-of-Hand-Crafted-and-Learned-Local-Features.pdf[10] https://arxiv.org/pdf/1709.08429.pdf[11] https://www.cv-foundation.org/openaccess/content_cvpr_2016/papers/Brachmann_Uncertainty-Driven_6D_Pose_CVPR_2016_paper.pdf[12] https://arxiv.org/pdf/1703.10896.pdf[13] https://arxiv.org/pdf/1611.07004.pdf[14] https://arxiv.org/pdf/1703.05192.pdf[15] https://arxiv.org/pdf/1710.10196.pdf[16] https://arxiv.org/pdf/1702.04405.pdf[17] https://arxiv.org/pdf/1709.06158.pdf[18] https://arxiv.org/pdf/1612.07429.pdf[19] https://arxiv.org/pdf/1611.08974.pdf[20] https://arxiv.org/pdf/1705.03122.pdf[21] https://arxiv.org/pdf/1702.03920.pdf[22] https://arxiv.org/pdf/1611.03530.pdf[23] https://arxiv.org/pdf/1710.05468.pdf[24] https://arxiv.org/pdf/1612.00593.pdf[25] https://arxiv.org/pdf/1609.02907.pdf[26] https://arxiv.org/pdf/1703.00443.pdf[27] https://arxiv.org/pdf/1704.01222.pdf[28] https://arxiv.org/pdf/1702.08360.pdf
- Home >
- Catalog >
- Business >
- Certificate Template >
- Death Certificate Template >
- Cause Of Death And The Death Certificate Form >
- death certificate cause of death examples >
- An Application Of Probabilistic Matching