How to Edit Your Mapping Nominal Values To Numbers For Effective Visualization Online Easily and Quickly
Follow these steps to get your Mapping Nominal Values To Numbers For Effective Visualization edited with efficiency and effectiveness:
- Select the Get Form button on this page.
- You will enter into our PDF editor.
- Edit your file with our easy-to-use features, like adding checkmark, erasing, and other tools in the top toolbar.
- Hit the Download button and download your all-set document for reference in the future.
We Are Proud of Letting You Edit Mapping Nominal Values To Numbers For Effective Visualization In the Most Efficient Way
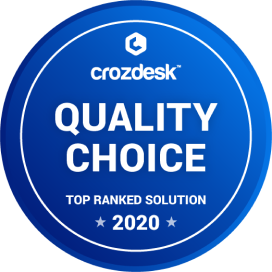
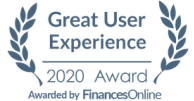
Explore More Features Of Our Best PDF Editor for Mapping Nominal Values To Numbers For Effective Visualization
Get FormHow to Edit Your Mapping Nominal Values To Numbers For Effective Visualization Online
When you edit your document, you may need to add text, give the date, and do other editing. CocoDoc makes it very easy to edit your form with the handy design. Let's see the easy steps.
- Select the Get Form button on this page.
- You will enter into our online PDF editor page.
- Once you enter into our editor, click the tool icon in the top toolbar to edit your form, like signing and erasing.
- To add date, click the Date icon, hold and drag the generated date to the field you need to fill in.
- Change the default date by deleting the default and inserting a desired date in the box.
- Click OK to verify your added date and click the Download button once the form is ready.
How to Edit Text for Your Mapping Nominal Values To Numbers For Effective Visualization with Adobe DC on Windows
Adobe DC on Windows is a popular tool to edit your file on a PC. This is especially useful when you like doing work about file edit without using a browser. So, let'get started.
- Find and open the Adobe DC app on Windows.
- Find and click the Edit PDF tool.
- Click the Select a File button and upload a file for editing.
- Click a text box to change the text font, size, and other formats.
- Select File > Save or File > Save As to verify your change to Mapping Nominal Values To Numbers For Effective Visualization.
How to Edit Your Mapping Nominal Values To Numbers For Effective Visualization With Adobe Dc on Mac
- Find the intended file to be edited and Open it with the Adobe DC for Mac.
- Navigate to and click Edit PDF from the right position.
- Edit your form as needed by selecting the tool from the top toolbar.
- Click the Fill & Sign tool and select the Sign icon in the top toolbar to make you own signature.
- Select File > Save save all editing.
How to Edit your Mapping Nominal Values To Numbers For Effective Visualization from G Suite with CocoDoc
Like using G Suite for your work to sign a form? You can edit your form in Google Drive with CocoDoc, so you can fill out your PDF without worrying about the increased workload.
- Add CocoDoc for Google Drive add-on.
- In the Drive, browse through a form to be filed and right click it and select Open With.
- Select the CocoDoc PDF option, and allow your Google account to integrate into CocoDoc in the popup windows.
- Choose the PDF Editor option to begin your filling process.
- Click the tool in the top toolbar to edit your Mapping Nominal Values To Numbers For Effective Visualization on the field to be filled, like signing and adding text.
- Click the Download button in the case you may lost the change.
PDF Editor FAQ
Why is the Henan province in China underdeveloped?
Q. Why is the Henan province in China underdeveloped?A. Reasons convergence has slowed, inland versus coastal economies (gap between rich coastal provinces and inland provinces not decreasing):Commodity boom is over.Policy mistakes (wasteful spending on physical assets as share of provincial GDP).Network effect (business stays in coastal cities because of better skills and education, more reliable legal institutions)Inland economic reliance on agriculture, natural resources/commodities, semi-industrialized, weak service sectorPopulation: 94 millions, 5th largest economy, 19th per capita GDP.Coastal provinces rely on export markets. Inland provinces rely on central government.Rich province, poor province (economist.com)Print edition | China Oct 1st 2016 BEIJINGEARLY in the summer Xi Jinping, China’s president, toured one of the country’s poorest provinces, Ningxia in the west. “No region or ethnic group can be left behind,” he insisted, echoing an egalitarian view to which the Communist Party claims to be wedded. In the 1990s, as China’s economy boomed, inland provinces such as Ningxia fell far behind the prosperous coast, but Mr Xi said there had since been a “gradual reversal” of this trend. He failed to mention that this is no longer happening. As China’s economy slows, convergence between rich and poor provinces is stalling. One of the party’s much-vaunted goals for the country’s development, “common prosperity”, is looking far harder to attain.This matters to Mr Xi (pictured, in Ningxia). In recent years the party’s leaders have placed considerable emphasis on the need to narrow regional income gaps. They say China will be a “moderately prosperous society” by the end of the decade. It will only be partly so if growth fails to pick up again inland. Debate has started to emerge in China about whether the party has been using the right methods to bring prosperity to backward provinces.China is very unequal. Shanghai, which is counted as a province, is five times wealthier than the poorest one, Gansu, which has a similar-sized population (see map). That is a wider spread than in notoriously unequal Brazil, where the richest state, São Paulo, is four times richer than the poorest, Piauí (these comparisons exclude the special cases of Hong Kong and Brasília).To iron out living standards, the government has used numerous strategies. They include a “Go West” plan involving the building of roads, railways, pipelines and other investment inland; Mr Xi’s signature “Belt and Road” policy aimed partly at boosting economic ties with Central Asia and South-East Asia and thereby stimulating the economies of provinces adjoining those areas; a twinning arrangement whereby provinces and cities in rich coastal areas dole out aid and advice to inland counterparts; and a project to beef up China’s rustbelt provinces in the north-east bordering Russia and North Korea. The central government also gives extra money to poorer provinces. Ten out of China’s 33 provinces get more than half their budgets from the centre’s coffers. Prosperous Guangdong on the coast gets only 10%.The number, range and cost of these policies suggest the party sees its legitimacy rooted not only in the creation of wealth but the ability to spread it around. Deng Xiaoping’s economic reforms, launched in the late 1970s, helped seaboard provinces, which were then poorer than inland ones, to catch up by making things and shipping them abroad. (Mao had discouraged investment in coastal areas, fearing they were vulnerable to attack.) In the 1990s the coast pulled ahead. Then, after 2000, the gap began to narrow again as the worldwide commodity boom—a product of China’s rapid growth—increased demand for raw materials produced in the interior (see chart). That was a blessing for Mr Xi’s predecessor Hu Jintao, who made “rebalancing” a priority after he became party chief in 2002. It also boosted many economists’ optimism about China’s ability to sustain rapid growth. Even if richer provinces were to slow down, they reckoned, the high growth potential of inland regions would compensate for that.But convergence is ending. GDP growth slowed across the country last year, but especially in poorer regions. Seven inland provinces had nominal growth below 2%, a recession by Chinese standards (in 2014 only one province reported growth below that level). In contrast, the rich provincial-level municipalities of Shanghai, Beijing and Tianjin, plus a clutch of other coastal provinces including Guangdong, grew between 5% and 8%. Though there were exceptions, the rule of thumb in 2015 was that the poorer the region, the slower the growth. Most of the provinces with below-average growth were poor.Of course, 2015 was just one year. But a longer period confirms the pattern. Of 31 provinces, 21 had an income below 40,000 yuan ($6,200) per person in 2011. Andrew Batson of Gavekal Dragonomics, a research firm, says that of these 21, 13 (almost two-thirds) saw their real GDP growth slow down by more than 4 points between 2011 and 2014. In contrast, only three of the ten richer provinces (those with income per person above the 40,000 yuan mark) slowed that much. In 2007 all of China’s provinces were narrowing their income gap with Shanghai. In 2015 barely a third of them were. In other words, China’s slowdown has been much sharper in poorer areas than richer ones.There are three reasons why convergence has stalled. The main one is that the commodity boom is over. Both coal and steel prices fell by two-thirds between 2011 and the end of 2015, before recovering somewhat this year. Commodity-producing provinces have been hammered. Gansu produces 90% of the country’s nickel. Inner Mongolia and Shanxi account for half of coal production. In all but four of the 21 inland provinces, mining and metals account for a higher share of GDP than the national average.Commodity-influenced slowdowns are often made worse by policy mistakes. This is the second reason for the halt in convergence. Inland provinces built a housing boom on the back of the commodity one, creating what seemed at the time like a perpetual-motion machine: high raw-material prices financed construction which increased demand for raw materials. When commodity prices fell, the boom began to look unsustainable.The pace of inland growth was evident in dizzying levels of investment in physical assets such as buildings and roads. Between 2008 and last year, as a share of provincial GDP, it rose from 48% to 73% in Shanxi, 64% to 78% in Inner Mongolia, and from 54% to an astonishing 104% in Xinjiang. In the country as a whole, investment as a share of GDP rose only slightly in that period, to 43%. In Shanghai it fell.This would be fine if the investments were productive, but provinces in the west are notorious for waste. In the coal-rich city of Ordos in Inner Mongolia, on the edge of the Gobi desert, a new district was built, designed for 1m people. It stood empty for years, a symbol of ill-planned extravagance (people are at last moving in).Investment by the government is keeping some places afloat. Tibet, for example, logged 10.6% growth in the first half of this year, thanks to net fiscal transfers from the central government amounting to a stunning 112% of GDP last year. Given the region’s political significance and strategic location, such handouts will continue—Tibet’s planners admit there is no chance of the region getting by without them for the foreseeable future.Tibet is an extreme example of the third reason why convergence is ending. Despite oodles of aid, both it and other poor provinces cannot compete with rich coastal ones. In theory, poorer places should eventually converge with rich areas because they will attract businesses with their cheaper labour and land. But it turns out that in China (as elsewhere) these advantages are outweighed by the assets of richer places: better skills and education, more reliable legal institutions, and so-called “network effects”—that is, the clustering of similar businesses in one place, which then benefit from the swapping of ideas and people. A recent study by Ryan Monarch, an economist at America’s Federal Reserve Board, showed that American importers of Chinese goods were very reluctant to change suppliers. When they do, they usually switch to another company in the same city. This makes it hard for inland competitors to break into export markets.There are exceptions. The south-western region of Chongqing has emerged as the world’s largest exporter of laptops. Chengdu, the capital of neighbouring Sichuan province, is becoming a financial hub. But by and large China’s export industry is not migrating inland. In 2002 six big coastal provinces accounted for 80% of manufactured exports. They still do.This contrast is worrying. Though income gaps did narrow after 2000 and only stopped doing so recently, provinces have not become alike in other respects. Rich ones continue to depend on world markets and foreign investment. Poor provinces increasingly depend on support from the central government.A divergence of viewsOfficials bicker about this. Mr Xi asserted the Robin-Hood view in Ningxia that regional gaps matter and that redistribution is needed. “The first to prosper,” he said, “should help the latecomers.” But three months earlier, an anonymous “authoritative person” (widely believed to be Mr Xi’s own adviser, Liu He) took a more relaxed view, telling the party’s mouthpiece, the People’s Daily, that “divergence is a necessity of economic development,” and “the faster divergence happens, the better.”It is unclear how this difference will be resolved, though the money must surely be on Mr Xi. Economically, though, Mr Liu is right. Regional-aid programmes have had little impact on the narrowing of income gaps. More of them will not stop those gaps widening. Socially, a slowdown in poorer provinces should not be a problem so long as jobs are still being created in richer ones, enabling migrants from inland to find work there and send money home. But politically the end of convergence is a challenge to Mr Xi, who has been trying to appeal to traditionalists in the party who extol Mao as a champion of equality. Wasteful and ineffective measures to achieve it will remain in place.Henan - WikipediaHenan (Chinese: 河南) is a province of the People's Republic of China, located in the central part of the country. Henan is often referred to as Zhongyuan or Zhongzhou (中州) which literally means "central plain land" or "midland", although the name is also applied to the entirety of China proper. Henan is the birthplace of Chinese civilization with over 3,000 years of recorded history, and remained China's cultural, economical, and political center until approximately 1,000 years ago.Henan province is a home to a large number of heritage sites which have been left behind including the ruins of Shang dynasty capital city Yin and the Shaolin Temple. Four of the Eight Great Ancient Capitals of China, Luoyang, Anyang, Kaifeng, and Zhengzhou are located in Henan.Although the name of the province (河南) means "south of the [Yellow] river", approximately a quarter of the province lies north of the Yellow River, also known as the Huang He. With an area of 167,000 km (64,479 sq mi), Henan covers a large part of the fertile and densely populated North China Plain. Its neighbouring provinces are Shaanxi, Shanxi, Hebei, Shandong, Anhui and Hubei. Henan is China's third most populous province with a population of over 94 million. If it were a country by itself, Henan would be the 14th most populous country in the world, ahead of Egypt and Vietnam.Henan is the 5th largest provincial economy of China and the largest among inland provinces. However, per capita GDP is low compared to other eastern and central provinces.Henan is considered to be one of the less developed areas in China. The economy continues to grow based on aluminum and coal prices, as well as agriculture, heavy industry, tourism, and retail, and high tech industries and service sector is underdeveloped and is concentrated around Zhengzhou and Luoyang.EconomyHenan has seen rapid development in its economy over the past two decades, and its economy has expanded at an even faster rate than the national average of 10%. This rapid growth has transformed Henan from one of the poorest provinces to one that matches other central provinces, though still relatively impoverished on a national scale. In 2011, Henan's nominal GDP was 3.20 trillion RMB (US$427 billion), making it the fifth largest economy in China, although it ranks nineteenth in terms of GDP per capita.Henan is a semi-industrialized economy with an underdeveloped service sector. In 2009, Henan's primary, secondary, and tertiary industries were worth 277 billion RMB (US$40 billion), 1.097 trillion RMB (US$160 billion), and 563 billion RMB (US$82 billion), respectively. Agriculture has traditionally been a pillar of its economy, with the nation's highest wheat and sesame output and second highest rice output, earning its reputation as the breadbasket of China. Henan is also an important producer of beef, cotton, maize, pork, animal oil, and corn. Food production and processing makes up more than 14% of the output from the province's secondary industry, and it is said that 90% of Chinese McDonald's and KFC ingredients comes from Henan.Although Henan's industry has traditionally been based on light textiles and food processing, recent developments have diversified the industry sector to metallurgy, petrol, cement, chemical industry, machinery and electronics. Henan has the second largest molybdenum reserves in the world. Coal, aluminum, alkaline metals and tungsten are also present in large amounts in western Henan. Export and processing of these materials is one of the main sources of revenues.Henan is actively trying to build its economy around the provincial capital of Zhengzhou, and it is hoped that the province may become an important transportation and manufacturing hub in the years to come. In 2008, the total trade volume (import and export) was US$17.5 billion, including US$10.7 billion for exports. Since 2002, 7,111 foreign enterprises have been approved, and foreign funds (FDI) of US$10.64 billion have been used in contracts with a realized FDI of US$5.3 billion. Foreign exchanges are increasing continuously. Friendly provincial relationships have been established with 16 states (districts) in the United States, Japan, Russia, France, Germany, and others. Some cities of Henan have established friendly relationships (sister city) with thirty-two foreign cities.Henan's service sector is rather small and underdeveloped. Finance and commerce are largely concentrated in urban centers such as Zhengzhou and Luoyang, where the economy is fueled by a large and relatively affluent consumer base. In order to make the economy more knowledge- and technology-based, the government established a number of development zones in all of the major cities, promoting industries such as software, information technologies, new materials, bio-pharmaceutical and photo-machinery-electronics. Henan is a major destination for tourists, with places such as Shaolin Temple and Longmen Grottoes attracting millions of tourists each year.How China’s poorest regions are going to save its growth rateChina is undergoing a dramatic shift in its economic geography. In 2001, Chinese coastal residents on average had an income that was 2.4 times that of their inland brethren. By 2011 this ratio fell to only 1.9 times. Analyzing this collapsing income gap between the interior and the coast paints a much more optimistic picture of the sustainability of China’s investment and growth.From 2001 to 2011, the Chinese economy grew at an average annual rate of 11.6% percent, whereas the US economy grew at only 1.8%. Does this mean that the Chinese system is “better” at delivering growth? Not really. The primary reason for this difference is because China is still so poor. As former World Bank chief economist Justin Lin has noted, China’s relative backwardness in technology means it can grow through “imitation, import, and/or integration of existing technologies and industries.” At this stage, the Chinese economy does not need to invent—it needs only to build. As a result, China is enjoying a period of accelerated growth as it catches up with the West—a process that economists call convergence.But what is true for China relative to the world is also true for Chinese provinces relative to each other. As seen in the maps above, the highest provincial growth rates from 2001 to 2011 have occurred among the inland Chinese provinces, which were also the poorest. As an example of this phenomenon, consider the decision by many manufacturing companies to move production inland. “Henan and Sichuan have always been the largest sources of migrant workers. That was why we moved to both of these provinces to tap their labor pool,” says Foxconn spokesman Louis Woo. As a result of this inland growth, in the span of 10 years, inland Chinese residents have on average seen their incomes rise by a factor of 3.2, whereas coastal residents have had their incomes rise by only a factor of 2.6. Therefore income levels in inland provinces are catching up to those on the coast.Another way of visualizing this convergence is to consider the relationship between a province’s initial income level in 2001 and its growth rate over the subsequent decade. As seen in the plot below, there was a significant negative correlation between the two. In fact, it explains over 50% of the variation in growth rates in this sample. The negative relationship means that poorer provinces have tended to grow faster than rich ones. On average, a doubling of a province’s initial per capita income in 2001 lowered its average growth rate in the subsequent decade by about 2%. So even as the coast slows down, inland China picks up and incomes converge.This change in Chinese economic geography has a wide range of implications for both the short and long term.First, regional convergence makes a sudden stop in Chinese growth implausible. If growth were to fall to 4%, that would demand that growth in inland provinces be substantially worse than the growth rates of coastal China 10 years prior. But why should the Hubei of 2011 be unable to attain growth rates comparable to Guangdong when it was at the same income level 10 years ago? As discussed above, manufacturing, the previous engine of coastal growth, is moving inland. This suggests that the coastal model of growth may still be applicable for inland China.Others have raised the argument that inland China’s institutions are worse than those of coastal China (sign up required), and therefore inland China’s growth will fail to be as fast. But even if inland China suffers from more extractive institutions right now, there’s nothing intrinsic to those provinces that should keep them stay that way. In fact, strong growth can spark a virtuous cycle of institutional reform and help inland China follow the growth path previously blazed by the coast.Second, any worries about impending social unrest as the result of slowing growth are likely overblown. Because social unrest in China is typically local, focusing on national GDP growth is very misleading. Instead, it’s provincial level growth that matters. On this point, the data is quite optimistic. From 2011 to 2012, the average per capita income growth rate in inland provinces was around 11%, whereas the average for coastal provinces was only 7%. Although the coastal provinces may have suffered lower rates of growth, they already have relatively high incomes. Therefore no matter what, income levels and growth rates should be high enough to placate any mass scale social unrest.Third, regional convergence means that China’s long-run growth rates will still be quite high for the next decade. Because provinces such as Anhui, Hubei, and Sichuan are still very poor, there is room for them to catch up. So with the assumption that growth in the provinces for the next 10 years follows the same relationship between income and growth observed from 2001 to 2011, then China will still be able to maintain a growth rate of 7.7%. This makes the recent 7.5% target set by Lin (paywall) seem quite moderate and grounded on long term fundamentals.While this growth prediction may seem high in light of recent bearishness towards China, it is actually more likely to be an underestimate of China’s real growth potential. If the provinces were to follow the same convergence path as in 2001 to 2011, that would imply that the only remaining factor in Chinese economic growth is for provinces to accumulate capital, and that advances in technology will no longer play a role. But as discussed above, China is still very far from the frontier of technology development. This means that by adopting more western technology, the Chinese government still has the potential to further propel growth beyond 7.7%.Moreover, as China’s economic center of gravity moves inland, the inland provinces will be able to contribute more to GDP growth. Just as emerging markets are starting to make a larger contribution to global growth, inland provinces are starting to make a larger contribution to Chinese growth. From 2001 to 2011, inland China contributed to 46% of the growth in real GDP, compared to 40% over the previous 10 year period. If Chinese provinces converge as described above, this proportion will rise to 54% by 2021. Because inland provinces now make up a larger share of GDP, their relatively high growth rates will take up a larger proportion of total Chinese growth. Therefore so long as the inland provinces can maintain their current growth path, China’s national growth rate will not collapse.Fourth, convergence makes claims of excessive investment in inland provinces (pdf), much less credible. Recently, there has been concern that China’s real estate investment, particularly in the inland provinces, has created too much excess capacity. But since China’s inland population of over 700 million will roughly double its income over the next 10 years, growth will create the demand for much of the new housing being built in those areas. Therefore the much maligned ghost cities may soon be crowded by a rising Chinese middle class.Similarly, the increase in wealth will put transportation infrastructure under greater pressure and the “over invested” white elephants in the inland provinces may turn brown in the dust of growth. A recent video of a Beijing subway transfer line during rush hour serves as a reminder of just how much infrastructure will still be needed in the inland provinces as their incomes converge to those on the coast. The bottom line is that China, with its high growth rates, can “grow into” its capital stock. While this policy may have hurt Chinese households by denying them many years of more consumption, strong growth trends mean that the foundations for growth are still there and there is no reason for all of the accumulated investment and capacity to go to waste.So when Paul Krugman boldly declares that China will suffer from a sudden drop in growth, the first question that should be asked is “which China?” Because economic conditions differ so widely across provinces, to speak of a unified level of Chinese development obscures the underlying regional patterns of growth. These patterns lead to a different understanding of the economic data and a much brighter outlook for the Chinese economy. Even if growth in developing countries must eventually slow down, a look inland shows that the mini-countries we call Chinese provinces still have a long way to go.(Note: Nominal GDP numbers for each province are from the University of Michigan China Data Center. To get real GDP values, the nominal GDP levels were deflated by the China national level GDP deflator as provided by the World Bank World Development Indicators.)Follow Yichuan on Twitter at @yichuanw. His blog is Synthenomics. We welcome your comments at [email protected] | province, China (brittanica.com)Why are people from Dongbei and Henan despised in China (Quora)Blue is exporting, Red is importing
How do you handle categorical data?
Identifying Categorical Data: Nominal, Ordinal and ContinuousCategorical features can only take on a limited, and usually fixed, number of possible values. For example, if a dataset is about information related to users, then you will typically find features like country, gender, age group, etc. Alternatively, if the data you're working with is related to products, you will find features like product type, manufacturer, seller and so on.These are all categorical features in your dataset. These features are typically stored as text values which represent various traits of the observations. For example, gender is described as Male (M) or Female (F), product type could be described as electronics, apparels, food etc.Note that these type of features where the categories are only labeled without any order of precedence are called nominal features.Features which have some order associated with them are called ordinal features. For example, a feature like economic status, with three categories: low, medium and high, which have an order associated with them.There are also continuous features. These are numeric variables that have an infinite number of values between any two values. A continuous variable can be numeric or a date/time.Regardless of what the value is used for, the challenge is determining how to use this data in the analysis because of the following constraints:Categorical features may have a very large number of levels, known as high cardinality, (for example, cities or URLs), where most of the levels appear in a relatively small number of instances.Many machine learning models, such as regression or SVM, are algebraic. This means that their input must be numerical. To use these models, categories must be transformed into numbers first, before you can apply the learning algorithm on them.While some ML packages or libraries might transform categorical data to numeric automatically based on some default embedding method, many other ML packages don’t support such inputs.For the machine, categorical data doesn’t contain the same context or information that humans can easily associate and understand. For example, when looking at a feature called City with three cities New York, New Jersey and New Delhi, humans can infer that New York is closely related to New Jersey as they are from same country, while New York and New Delhi are much different. But for the model, New York, New Jersey and New Delhi, are just three different levels (possible values) of the same feature City. If you don’t specify the additional contextual information, it will be impossible for the model to differentiate between highly different levels.You therefore are faced with the challenge of figuring out how to turn these text values into numerical values for further processing and unmask lots of interesting information which these features might hide. Typically, any standard work-flow in feature engineering involves some form of transformation of these categorical values into numeric labels and then applying some encoding scheme on these values.General Exploration steps for Categorical DataIn this section, you'll focus on dealing with categorical features in the pnwflights14 dataset, but you can apply the same procedure to all kinds of datasets. pnwflights14 is a modified version of Hadley Wickham's nycflights13 dataset and contains information about all flights that departed from the two major airports of the Pacific Northwest (PNW), SEA in Seattle and PDX in Portland, in 2014: 162,049 flights in total.To help understand what causes delays, it also includes a number of other useful datasets:weather: the hourly meterological data for each airportplanes: constructor information about each planeairports: airport names and locationsairlines: translation between two letter carrier codes and namesThe datasets can be found here.Since it's always a good idea to understand before starting working on it, you'll briefly explore the data! To do this, you will first import the basic libraries that you will be using throughout the tutorial, namely pandas, numpy and copy.Also make sure that you set Matplotlib to plot inline, which means that the outputted plot will appear immediately under each code cell.import pandas as pd import numpy as np import copy %matplotlib inline Next you will read the flights dataset in a pandas DataFrame with read_csv() and check the contents with the .head() method.df_flights = pd.read_csv('https://raw.githubusercontent.com/ismayc/pnwflights14/master/data/flights.csv') df_flights.head() As you will probably notice, the DataFrame above contains all kinds of information about flights like year, departure delay, arrival time, carrier, destination, etc.Note if you are reading the RDS file formats you can do so by installing rpy2 library. Checkout this link to install the library on your system. The simplest way to install the library is using pip install rpy2 command on command line terminal.Running the following code would read the flights.RDS file and load it in a pandas DataFrame. Remember that you already imported pandas earlier.import rpy2.robjects as robjects from rpy2.robjects import pandas2ri pandas2ri.activate() readRDS = robjects.r['readRDS'] RDSlocation = 'Downloads/datasets/nyc_flights/flights.RDS' #location of the file df_rds = readRDS(RDSlocation) df_rds = pandas2ri.ri2py(df_rds) df_rds.head(2) The same rpy2 library can also be used to read rda file formats. The code below reads and loads flights.rda into a pandas DataFrame:from rpy2.robjects import r import rpy2.robjects.pandas2ri as pandas2ri file="~/Downloads/datasets/nyc_flights/flights.rda" #location of the file rf=r['load'](file) df_rda=pandas2ri.ri2py_dataframe(r[rf[0]]) df_rda.head(2) The next step is to gather some information about different column in your DataFrame. You can do so by using .info(), which basically gives you information about the number of rows, columns, column data types, memory usage, etc.print(df_flights.info()) <class 'pandas.core.frame.DataFrame'> RangeIndex: 162049 entries, 0 to 162048 Data columns (total 16 columns): year 162049 non-null int64 month 162049 non-null int64 day 162049 non-null int64 dep_time 161192 non-null float64 dep_delay 161192 non-null float64 arr_time 161061 non-null float64 arr_delay 160748 non-null float64 carrier 162049 non-null object tailnum 161801 non-null object flight 162049 non-null int64 origin 162049 non-null object dest 162049 non-null object air_time 160748 non-null float64 distance 162049 non-null int64 hour 161192 non-null float64 minute 161192 non-null float64 dtypes: float64(7), int64(5), object(4) memory usage: 19.8+ MB None As you can see, columns like year, month and day are read as integers, and dep_time, dep_delay etc. are read as floats.The columns with object dtype are the possible categorical features in your dataset.The reason why you would say that these categorical features are 'possible' is because you shouldn't not completely rely on .info() to get the real data type of the values of a feature, as some missing values that are represented as strings in a continuous feature can coerce it to read them as object dtypes.That's why it's always a good idea to investigate your raw dataset thoroughly and then think about cleaning it.One of the most common ways to analyze the relationship between a categorical feature and a continuous feature is to plot a boxplot. The boxplot is a simple way of representing statistical data on a plot in which a rectangle is drawn to represent the second and third quartiles, usually with a vertical line inside to indicate the median value. The lower and upper quartiles are shown as horizontal lines at either side of the rectangle.You can plot a boxplot by invoking .boxplot() on your DataFrame. Here, you will plot a boxplot of the dep_time column with respect to the two origin of the flights from PDX and SEA.df_flights.boxplot('dep_time','origin',rot = 30,figsize=(5,6)) <matplotlib.axes._subplots.AxesSubplot at 0x7f32ee10f550> As you will only be dealing with categorical features in this tutorial, it's better to filter them out. You can create a separate DataFrame consisting of only these features by running the following command. The method .copy() is used here so that any changes made in new DataFrame don't get reflected in the original one.cat_df_flights = df_flights.select_dtypes(include=['object']).copy() Again, use the .head() method to check if you have filtered the required columns.cat_df_flights.head() One of the most common data pre-processing steps is to check for null values in the dataset. You can get the total number of missing values in the DataFrame by the following one liner code:print(cat_df_flights.isnull().values.sum()) 248 Let's also check the column-wise distribution of null values:print(cat_df_flights.isnull().sum()) carrier 0 tailnum 248 origin 0 dest 0 dtype: int64 It seems that only the tailnum column has null values. You can do a mode imputation for those null values. The function fillna() is handy for such operations.Note the chaining of method .value_counts() in the code below. This returns the frequency distribution of each category in the feature, and then selecting the top category, which is the mode, with the .index attribute.cat_df_flights = cat_df_flights.fillna(cat_df_flights['tailnum'].value_counts().index[0]) Tip: read more about method chaining with pandas here.Let's check the number of null values after imputation should result in a zero count.print(cat_df_flights.isnull().values.sum()) 0 Another Exploratory Data Analysis (EDA) step that you might want to do on categorical features is the frequency distribution of categories within the feature, which can be done with the .value_counts() method as described earlier.print(cat_df_flights['carrier'].value_counts()) AS 62460 WN 23355 OO 18710 DL 16716 UA 16671 AA 7586 US 5946 B6 3540 VX 3272 F9 2698 HA 1095 Name: carrier, dtype: int64 To know the count of distinct categories within the feature you can chain the previous code with the .count() method:print(cat_df_flights['carrier'].value_counts().count()) 11 Visual exploration is the most effective way to extract information between variables.Below is a basic template to plot a barplot of the frequency distribution of a categorical feature using the seaborn package, which shows the frequency distribution of the carrier column. You can play with different arguments to change the look of the plot. If you want to learn more about seaborn, you can take a look at this tutorial.%matplotlib inline import seaborn as sns import matplotlib.pyplot as plt carrier_count = cat_df_flights['carrier'].value_counts() sns.set(style="darkgrid") sns.barplot(carrier_count.index, carrier_count.values, alpha=0.9) plt.title('Frequency Distribution of Carriers') plt.ylabel('Number of Occurrences', fontsize=12) plt.xlabel('Carrier', fontsize=12) plt.show() Similarly, you could plot a pie chart with the matplotlib library to get the same information. The labels list below holds the category names from the carrier column:labels = cat_df_flights['carrier'].astype('category').cat.categories.tolist() counts = cat_df_flights['carrier'].value_counts() sizes = [counts[var_cat] for var_cat in labels] fig1, ax1 = plt.subplots() ax1.pie(sizes, labels=labels, autopct='%1.1f%%', shadow=True) #autopct is show the % on plot ax1.axis('equal') plt.show() Encoding Categorical DataYou will now learn different techniques to encode the categorical features to numeric quantities. To keep it simple, you will apply these encoding methods only on the carrier column. However, the same approach can be extended to all columns.The techniques that you'll cover are the following:Replacing valuesEncoding labelsOne-Hot encodingBinary encodingBackward difference encodingMiscellaneous featuresReplace ValuesLet's start with the most basic method, which is just replacing the categories with the desired numbers. This can be achieved with the help of the replace() function in pandas. The idea is that you have the liberty to choose whatever numbers you want to assign to the categories according to the business use case.You will now create a dictionary which contains mapping numbers for each category in the carrier column:replace_map = {'carrier': {'AA': 1, 'AS': 2, 'B6': 3, 'DL': 4, 'F9': 5, 'HA': 6, 'OO': 7 , 'UA': 8 , 'US': 9,'VX': 10,'WN': 11}} Note that defining a mapping via a hard coded dictionary is easy when the number of categories is low, like in this case which is 11. You can achieve the same mapping with the help of dictionary comprehensions as shown below. This will be useful when the categories count is high and you don't want to type out each mapping. You will store the category names in a list called labels and then zip it to a seqeunce of numbers and iterate over it.labels = cat_df_flights['carrier'].astype('category').cat.categories.tolist() replace_map_comp = {'carrier' : {k: v for k,v in zip(labels,list(range(1,len(labels)+1)))}} print(replace_map_comp) {'carrier': {'AA': 1, 'OO': 7, 'DL': 4, 'F9': 5, 'B6': 3, 'US': 9, 'AS': 2, 'WN': 11, 'VX': 10, 'HA': 6, 'UA': 8}} Throughout this tutorial, you will be making a copy of the dataset via the .copy() method to practice each encoding technique to ensure that the original DataFrame stays intact and whatever changes you are doing happen only in the copied one.cat_df_flights_replace = cat_df_flights.copy() Use the replace() function on the DataFrame by passing the mapping dictionary as argument:cat_df_flights_replace.replace(replace_map_comp, inplace=True) print(cat_df_flights_replace.head()) As you can observe, you have encoded the categories with the mapped numbers in your DataFrame.You can also check the dtype of the newly encoded column, which is now converted to integers.print(cat_df_flights_replace['carrier'].dtypes) int64 Tip: in Python, it's a good practice to typecast categorical features to a category dtype because they make the operations on such columns much faster than the object dtype. You can do the typecasting by using .astype() method on your columns like shown below:cat_df_flights_lc = cat_df_flights.copy() cat_df_flights_lc['carrier'] = cat_df_flights_lc['carrier'].astype('category') cat_df_flights_lc['origin'] = cat_df_flights_lc['origin'].astype('category') print(cat_df_flights_lc.dtypes) carrier category tailnum object origin category dest object dtype: object You can validate the faster operation of the category dtype by timing the execution time of the same operation done on a DataFrame with columns as category dtype and object dtype by using the time library.Let's say you want to calculate the number of flights for each carrier from each origin places, you can use the .groupby() and .count() methods on your DataFrame to do so.import time %timeit cat_df_flights.groupby(['origin','carrier']).count() #DataFrame with object dtype columns 10 loops, best of 3: 28.6 ms per loop %timeit cat_df_flights_lc.groupby(['origin','carrier']).count() #DataFrame with category dtype columns 10 loops, best of 3: 20.1 ms per loop Note that the DataFrame with category dtype is much faster.Label EncodingAnother approach is to encode categorical values with a technique called "label encoding", which allows you to convert each value in a column to a number. Numerical labels are always between 0 and n_categories-1.You can do label encoding via attributes .cat.codes on your DataFrame's column.cat_df_flights_lc['carrier'] = cat_df_flights_lc['carrier'].cat.codes cat_df_flights_lc.head() #alphabetically labeled from 0 to 10 Sometimes, you might just want to encode a bunch of categories within a feature to some numeric value and encode all the other categories to some other numeric value.You could do this by using numpy's where() function like shown below. You will encode all the US carrier flights to value 1 and other carriers to value 0. This will create a new column in your DataFrame with the encodings. Later, if you want to drop the original column, you can do so by using the drop() function in pandas.cat_df_flights_specific = cat_df_flights.copy() cat_df_flights_specific['US_code'] = np.where(cat_df_flights_specific['carrier'].str.contains('US'), 1, 0) cat_df_flights_specific.head() You can achieve the same label encoding using scikit-learn's LabelEncoder:cat_df_flights_sklearn = cat_df_flights.copy() from sklearn.preprocessing import LabelEncoder lb_make = LabelEncoder() cat_df_flights_sklearn['carrier_code'] = lb_make.fit_transform(cat_df_flights['carrier']) cat_df_flights_sklearn.head() #Results in appending a new column to df Label encoding is pretty much intuitive and straight-forward and may give you a good performance from your learning algorithm, but it has as disadvantage that the numerical values can be misinterpreted by the algorithm. Should the carrier US (encoded to 8) be given 8x more weight than the carrier AS (encoded to 1) ?To solve this issue there is another popular way to encode the categories via something called one-hot encoding.One-Hot encodingThe basic strategy is to convert each category value into a new column and assign a 1 or 0 (True/False) value to the column. This has the benefit of not weighting a value improperly.There are many libraries out there that support one-hot encoding but the simplest one is using pandas' .get_dummies() method.This function is named this way because it creates dummy/indicator variables (1 or 0). There are mainly three arguments important here, the first one is the DataFrame you want to encode on, second being the columns argument which lets you specify the columns you want to do encoding on, and third, the prefix argument which lets you specify the prefix for the new columns that will be created after encoding.cat_df_flights_onehot = cat_df_flights.copy() cat_df_flights_onehot = pd.get_dummies(cat_df_flights_onehot, columns=['carrier'], prefix = ['carrier']) print(cat_df_flights_onehot.head()) As you can see, the column carrier_AS gets value 1 at the 0th and 4th observation points as those points had the AS category labeled in the original DataFrame. Likewise for other columns also.scikit-learn also supports one hot encoding via LabelBinarizer and OneHotEncoder in its preprocessing module (check out the details here). Just for the sake of practicing you will do the same encoding via LabelBinarizer:cat_df_flights_onehot_sklearn = cat_df_flights.copy() from sklearn.preprocessing import LabelBinarizer lb = LabelBinarizer() lb_results = lb.fit_transform(cat_df_flights_onehot_sklearn['carrier']) lb_results_df = pd.DataFrame(lb_results, columns=lb.classes_) print(lb_results_df.head()) Note that this lb_results_df resulted in a new DataFrame with only the one hot encodings for the feature carrier. This needs to be concatenated back with the original DataFrame, which can be done via pandas' .concat() method. The axis argument is set to 1 as you want to merge on columns.result_df = pd.concat([cat_df_flights_onehot_sklearn, lb_results_df], axis=1) print(result_df.head()) While one-hot encoding solves the problem of unequal weights given to categories within a feature, it is not very useful when there are many categories, as that will result in formation of as many new columns, which can result in the curse of dimensionality. The concept of the “curse of dimensionality” discusses that in high-dimensional spaces some things just stop working properly.Binary EncodingThis technique is not as intuitive as the previous ones. In this technique, first the categories are encoded as ordinal, then those integers are converted into binary code, then the digits from that binary string are split into separate columns. This encodes the data in fewer dimensions than one-hot.You can do binary encoding via a number of ways but the simplest one is using the category_encoders library. You can install category_encoders via pip install category_encoders on cmd or just download and extract the .tar.gz file from the site.You have to first import the category_encoders library after installing it. Invoke the BinaryEncoder function by specifying the columns you want to encode and then call the .fit_transform() method on it with the DataFrame as the argument.cat_df_flights_ce = cat_df_flights.copy() import category_encoders as ce encoder = ce.BinaryEncoder(cols=['carrier']) df_binary = encoder.fit_transform(cat_df_flights_ce) df_binary.head() Notice that four new columns are created in place of the carrier column with binary encoding for each category in the feature.Note that category_encoders is a very useful library for encoding categorical columns. Not only does it support one-hot, binary and label encoding, but also other advanced encoding methods like Helmert contrast, polynomial contrast, backward difference, etc.5. Backward Difference EncodingThis technique falls under the contrast coding system for categorical features. A feature of K categories, or levels, usually enters a regression as a sequence of K-1 dummy variables. In backward difference coding, the mean of the dependent variable for a level is compared with the mean of the dependent variable for the prior level. This type of coding may be useful for a nominal or an ordinal variable.If you want to learn other contrast coding methods you can check out this resource.The code structure is pretty much the same as any method in the category_encoders library, just this time you will call BackwardDifferenceEncoder from it:encoder = ce.BackwardDifferenceEncoder(cols=['carrier']) df_bd = encoder.fit_transform(cat_df_flights_ce) df_bd.head() The interesting thing here is that you can see that the results are not the standard 1’s and 0’s you saw in the dummy encoding examples but rather regressed continuous values.Miscellaneous FeaturesSometimes you may encounter categorical feature columns which specify the ranges of values for observation points, for example, the age column might be described in the form of categories like 0-20, 20-40 and so on.While there can be a lot of ways to deal with such features, the most common ones are either split these ranges into two separate columns or replace them with some measure like the mean of that range.You will first create a dummy DataFrame which has just one feature age with ranges specified using the pandas DataFrame function. Then you will split the column on the delimeter - into two columns start and end using split() with a lambda() function. If you want to learn more about lambda functions, check out this tutorial.dummy_df_age = pd.DataFrame({'age': ['0-20', '20-40', '40-60','60-80']}) dummy_df_age['start'], dummy_df_age['end'] = zip(*dummy_df_age['age'].map(lambda x: x.split('-'))) dummy_df_age.head() To replace the range with its mean, you will write a split_mean() function which basically takes one range at a time, splits it, then calculates the mean and returns it. To apply a certain function to all the entities of a column you will use the .apply() method:dummy_df_age = pd.DataFrame({'age': ['0-20', '20-40', '40-60','60-80']}) def split_mean(x): split_list = x.split('-') mean = (float(split_list[0])+float(split_list[1]))/2 return mean dummy_df_age['age_mean'] = dummy_df_age['age'].apply(lambda x: split_mean(x)) dummy_df_age.head() Dealing with Categorical Features in Big Data with SparkNow you will learn how to read a dataset in Spark and encode categorical variables in Apache Spark's Python API, Pyspark. But before that it's good to brush up on some basic knowledge about Spark.Spark is a platform for cluster computing. It lets you spread data and computations over clusters with multiple nodes. Splitting up your data makes it easier to work with very large datasets because each node only works with a small amount of data.As each node works on its own subset of the total data, it also carries out a part of the total calculations required, so that both data processing and computations are performed in parallel over the nodes in the cluster.Deciding whether or not Spark is the best solution for your problem takes some experience, but you can consider questions like:Is my data too big to work with on a single machine?Can my calculations be easily parallelized?The first step in using Spark is connecting to a cluster. In practice, the cluster will be hosted on a remote machine that's connected to all other nodes. There will be one computer, called the master that manages splitting up the data and the computations. The master is connected to the rest of the computers in the cluster, which are called slaves. The master sends the slaves data and calculations to run, and they send their results back to the master.When you're just getting started with Spark, it's simpler to just run a cluster locally. If you wish to run Spark on a cluster and use Jupyter Notebook, you can check out this blog.If you wish to learn more about Spark, check out this great tutorial which covers almost everything about it, or DataCamp's Introduction to PySpark course.The first step in Spark programming is to create a SparkContext. SparkContext is required when you want to execute operations in a cluster. SparkContext tells Spark how and where to access a cluster. You'll start by importing SparkContext.from pyspark import SparkContext sc = SparkContext() Note that if you are working on Spark's interactive shell then you don't have to import SparkContext as it will already be in your environment as sc.To start working with Spark DataFrames, you first have to create a SparkSession object from your SparkContext. You can think of the SparkContext as your connection to the cluster and the SparkSession as your interface with that connection.Note that if you are working in Spark's interactive shell you'll have a SparkSession called spark available in your workspace!from pyspark.sql import SparkSession as spark Once you've created a SparkSession, you can start poking around to see what data is in your cluster.Your SparkSession has an attribute called catalog which lists all the data inside the cluster. This attribute has a few methods for extracting different pieces of information.One of the most useful is the .listTables() method, which returns the names of all the tables in your cluster as a list.print(spark.catalog.listTables()) [] Your catalog is currently empty!You will now load the flights dataset in the Spark DataFrame.To read a .csv file and create a Spark DataFrame you can use the .read attribute of your SparkSession object. Here, apart from reading the csv file, you have to additionally specify the headers option to be True, since you have column names in the dataset. Also, the inferSchema argument is set to True, which basically peeks at the first row of the data to determine the fields' names and types.spark_flights = spark.read.format("csv").option('header',True).load('Downloads/datasets/nyc_flights/flights.csv',inferSchema=True) To check the contents of your DataFrame you can run the .show() method on the DataFrame.spark_flights.show(3) +----+-----+---+--------+---------+--------+---------+-------+-------+------+------+----+--------+--------+----+------+ |year|month|day|dep_time|dep_delay|arr_time|arr_delay|carrier|tailnum|flight|origin|dest|air_time|distance|hour|minute| +----+-----+---+--------+---------+--------+---------+-------+-------+------+------+----+--------+--------+----+------+ |2014| 1| 1| 1| 96| 235| 70| AS| N508AS| 145| PDX| ANC| 194| 1542| 0| 1| |2014| 1| 1| 4| -6| 738| -23| US| N195UW| 1830| SEA| CLT| 252| 2279| 0| 4| |2014| 1| 1| 8| 13| 548| -4| UA| N37422| 1609| PDX| IAH| 201| 1825| 0| 8| +----+-----+---+--------+---------+--------+---------+-------+-------+------+------+----+--------+--------+----+------+ only showing top 3 rows If you wish to convert a pandas DataFrame to a Spark DataFrame, use the .createDataFrame() method on your SparkSession object with the DataFrame's name as argument.To have a look at the schema of the DataFrame you can invoke .printSchema() as follows:spark_flights.printSchema() root |-- year: integer (nullable = true) |-- month: integer (nullable = true) |-- day: integer (nullable = true) |-- dep_time: string (nullable = true) |-- dep_delay: string (nullable = true) |-- arr_time: string (nullable = true) |-- arr_delay: string (nullable = true) |-- carrier: string (nullable = true) |-- tailnum: string (nullable = true) |-- flight: integer (nullable = true) |-- origin: string (nullable = true) |-- dest: string (nullable = true) |-- air_time: string (nullable = true) |-- distance: integer (nullable = true) |-- hour: string (nullable = true) |-- minute: string (nullable = true) Note that Spark doesn't always guess the data type of the columns right and you can see that some of the columns (arr_delay, air_time, etc.) which seem to have numeric values are read as strings rather than integers or floats, due to the presence of missing values.At this point, if you check the data in your cluster using the .catalog attribute and the .listTables() method like you did before, you will find it's still empty. This is because you DataFrame is currently stored locally, not in the SparkSession catalog.To access the data in this way, you have to save it as a temporary table. You can do so by using the .createOrReplaceTempView() method. This method registers the DataFrame as a table in the catalog, but as this table is temporary, it can only be accessed from the specific SparkSession used to create the Spark DataFrame.spark_flights.createOrReplaceTempView("flights_temp") Print the tables in catalog again:print(spark.catalog.listTables()) [Table(name=u'flights_temp', database=None, description=None, tableType=u'TEMPORARY', isTemporary=True)] Now you have registered the flight_temp table as a temporary table in your catalog.Now that you have gotten your hands dirty with a little bit of PySpark code, it's time to see how to encode categorical features. To keep things neat, you will create a new DataFrame which consists of only the carrier column by using the .select() method.carrier_df = spark_flights.select("carrier") carrier_df.show(5) +-------+ |carrier| +-------+ | AS| | US| | UA| | US| | AS| +-------+ only showing top 5 rows The two most common ways to encode categorical features in Spark are using StringIndexer and OneHotEncoder.StringIndexer encodes a string column of labels to a column of label indices. The indices are in [0, numLabels] ordered by label frequencies, so the most frequent label gets index 0. This is similar to label encoding in pandas.You will start by importing the StringIndexer class from the pyspark.ml.feature module. The main arguments inside StringIndexer are inputCol and outputCol, which are self-explanatory. After you create the StringIndex object you call the .fit() and .transform() methods with the DataFrame as the argument passed as shown:from pyspark.ml.feature import StringIndexer carr_indexer = StringIndexer(inputCol="carrier",outputCol="carrier_index") carr_indexed = carr_indexer.fit(carrier_df).transform(carrier_df) carr_indexed.show(7) +-------+-------------+ |carrier|carrier_index| +-------+-------------+ | AS| 0.0| | US| 6.0| | UA| 4.0| | US| 6.0| | AS| 0.0| | DL| 3.0| | UA| 4.0| +-------+-------------+ only showing top 7 rows Since AS was the most frequent category in the carrier column, it got the index 0.0.OneHotEncoder: as you already read before, one-hot encoding maps a categorical feature, represented as a label index, to a binary vector with at most a single one-value indicating the presence of a specific feature value from among the set of all feature values.For example, with 5 categories, an input value of 2.0 would map to an output vector of [0.0, 0.0, 1.0, 0.0]. The last category is not included by default (configurable via OneHotEncoder .dropLast because it makes the vector entries sum up to one, and hence linearly dependent. That means that an input value of 4.0 would map to [0.0, 0.0, 0.0, 0.0].Note that this is different from scikit-learn's OneHotEncoder, which keeps all categories. The output vectors are sparse.For a string type like in this case, it is common to encode features using StringIndexer first, here carrier_index. Then pass that column to the OneHotEncoder class.The code is shown below.carrier_df_onehot = spark_flights.select("carrier") from pyspark.ml.feature import OneHotEncoder, StringIndexer stringIndexer = StringIndexer(inputCol="carrier", outputCol="carrier_index") model = stringIndexer.fit(carrier_df_onehot) indexed = model.transform(carrier_df_onehot) encoder = OneHotEncoder(dropLast=False, inputCol="carrier_index", outputCol="carrier_vec") encoded = encoder.transform(indexed) encoded.show(7) +-------+-------------+--------------+ |carrier|carrier_index| carrier_vec| +-------+-------------+--------------+ | AS| 0.0|(11,[0],[1.0])| | US| 6.0|(11,[6],[1.0])| | UA| 4.0|(11,[4],[1.0])| | US| 6.0|(11,[6],[1.0])| | AS| 0.0|(11,[0],[1.0])| | DL| 3.0|(11,[3],[1.0])| | UA| 4.0|(11,[4],[1.0])| +-------+-------------+--------------+ only showing top 7 rows Note that OneHotEncoder has created a vector for each category which can then be processed further by your machine learning pipeline.
What is the most successful animation studio?
This in-depth report lists the 9 most influential and prominent animation studios in the world.This report will give you a detailed look at each of the animation studios: The history of the studio, its founders, the size and number of employees, notable staff members and highest grossing / most awarded films.Bloop Animation | Animation FilmmakingAnimation Studios:Pixar Animation StudiosLocation: Emeryville, California, USAAnimation style: CG AnimationFounded: February 3rd, 1986Founder(s): Steve Jobs, Edwin Catmull, and Alvy Ray SmithEmployees: 600Notable employees: John Lasseter, Brad Bird, Pete Docter, Andrew Stanton, Lee UnkrichStudio History:Even though Pixar Animation Studios was officially founded in 1986, you can trace the history of the studio as far back as 1974, when the founder of the New York Institute of Technology, Dr.Alexander Schure, established the Computer Graphics Lab. The purpose of this lab was to create the first fully CG animated feature film, The Works, but they never completed it.Edwin Catmull was part of the CGL team, but moved over to Lucasfilms in 1979 when NYIT was running low on funds to support their project. He launched the Computer Graphics department of Lucasfilms. Alvy Ray Smith, also a member of CGL, followed Edwin and together they started working on a 3D rendering program. It would later become the work horse for Pixar; Renderman.After working with Industrial Light and Magic on Star Trek II: Wrath of Kahn and Young Sherlock Holmes, Catmull and Smith worried that George Lucas would sell off the entire Computer Graphics Group as he was going through a messy divorce and losing revenue on the Star Wars license. Catmull and Smith convinced Lucas to turn the group into an independent company to prevent the loss of valuable employees. Steve Jobs became very interested in investing into Pixar. Lucas was uncertain as he was only offering a total of 10 Million Dollars.In the end, Lucas had no choice, no one else showed any interest in buying, so he sold it to Steve.Pixar first started off with hardware, creating the Pixar Image Computers. They sold it primarily to government agencies, as well as to scientific and medical research purposes. Walt Disney Studios eventually bought the computers for their Computer Animation Production System project.However, Pixar was losing money badly, despite the stream of funding Steve Jobs poured into the company. The studio had to lay off 30 employees. Even a 26 million dollar deal with Disney to produce three animated films couldn’t save the studio from constantly bleeding money and workforce.At some point Steve Jobs considered selling the studio to Microsoft, but changed his mind after finding out that Disney will distribute Pixar’s first film, Toy Story, during the Christmas season. Toy Story was a massive hit, earning 361 million dollars worldwide, cementing Pixar as the pioneer of CG animation and a powerhouse in the animation industry to even rival Disney.Pixar would develop something they called the Pixar Braintrust, a collective of creative minds who would critique each others projects to achieve maximum potential for every film. Going by the success of Toy Story, Pixar wanted to recreate the process by creating a studio run by filmmakers instead of executives.Disagreement rose between Disney and Pixar during the production of Toy Story 2. The film was meant for a straight-to-video release, which they thought would circumvent the three-picture deal. Disney protested and insisted that the film should have a theatrical release. Relations kept getting worse as Steve Jobs and Disney CEO Michael Eisner couldn’t agree on a new distribution contract. After ten months, the negotiations fell through and Pixar was then seeking a new distributorMichael Eisner was forced out of his position and replaced by Bob Iger, who led a deal to purchase Pixar for 7.4 Billion Dollars, and negotiated that Catmull and Lasseter would be president of both Walt Disney Animation Studio and Pixar. Steve Jobs owned 50.1% of Pixar stocks, and became the chairman at Walt Disney Studio’s board of directors once the purchase was complete. Catmull and Lasseter would become a hugely positive influence for Disney, as they brought along with them the very effective Pixar Braintrust production process.Highest awarded film:Inside Out (76 Awards)Highest grossing film:Toy Story 3 (1.063 Billion Dollars)Walt Disney Animation StudiosLocation: Burbank, California, USAAnimation style: 2D Animation & CG AnimationFounded: October 16th, 1923Founder(s): Walt Disney and Roy O. DisneyEmployees: 800+Notable employees: Ub Iwerks, Les Clark, Marc Davis, Ollie Johnston, Mitt Kahl, Ward Kimball, Eric Larson, John Lounsbery, Wolfgang Reitherman, Frank ThomasStudio History:Walt Disney Animation Studios was created as a division of Walt Disney Studios. Its purpose was to create animated feature films, short films, and television specials. The studio’s name was actually Disney Brothers Cartoon Studio at first, but was later incorporated into the Walt Disney Studio in 1929. It would not be the first time it changed its name. They changed it to Walt Disney Feature Animations in 1986, and then finally to Walt Disney Animation Studios in 2006 when Disney officially acquired Pixar Animation Studios.The first studio was tiny, based in their uncle’s garage in Los Angeles, but eventually they moved into a larger space, next to a real-estate agency office. Then, in 1926, the studio moved to Silver Lake, where they took on the Walt Disney Studio name.When the studio was founded in 1923, Walt Disney and Roy O. Disney got their start by producing several Alice Comedies shorts. A live-action girl interacted with an animated world. These shorts were distributed by Winkler Pictures. In 1927 ordered several animated shorts based on Disney’s original character Oswald the Lucky Rabbit. However, when the head of Winkler Pictures, Margaret J.Winkler, married Charles Mintz, he took over the company and tried to force Disney to reduce advance payment for each Oswald cartoon. Disney refused, which led to Mintz taking the Oswald character and making his own cartoons through Universal Pictures. However, Walt Disney and his lead animator Ub Iwerks had secretly developed a character who would become the face of Walt Disney Animation Studios; Mickey Mouse.In 1928, Walt Disney Studios premièred Steamboat Willie in New York, which was the first animation with synchronized sounds. It was a massive success and the Mickey Mouse series became the most popular cartoon series in the US. The success of Mickey Mouse lead to Disney creating another series of animation – Silly Symphonies.Silly Symphonies was distributed by Pat Powers through his company Celebrity Productions. However, in 1930 there was a financial dispute between Disney and Powers. It led to Disney signing a distribution contract with Columbia Pictures. This contract only lasted for two years, as Disney signed a new contract with United Artists in 1932, as well as signing an exclusive contract with Technicolor to use their new 3-strip color film process.In 1934 Walt Disney announced to his employees that they would create a feature-length animated film. Thus began the production of Snow White and the Seven Dwarfs. Critics considered this foolish and expected it would end up bankrupting the studio. In the end, however, they completed the film, which was a box-office hit. It was also the very first English-speaking animated film.As World War II raged on, Walt Disney decided to do his part for the cause by making a deal with the U.S Army to produce animated military training films, as well as propaganda films for civilians. The war proved to be quite a headache for Disney. Equipment and materials were difficult to acquire, and most people simply couldn’t afford to go to the cinema. They released Bambi in 1942 and it became the studio’s third major flop, following Pinocchio and Fantasia. This led to deep financial troubles for Walt Disney Studio. Prior to that, the animators had gone on strike. It led to many talented animators leaving the company before a final deal was set, and an animators union was established. The Studio stopped making animated feature films, and would instead focus on low-budget “package films”.Walt Disney Studio did make two live-action/animation hybrid films, Song of the South and So Dear To My Heart, but the studio wouldn’t start production on another feature-length animated film until 1948 with Cinderella. Being the highest budgeted film in Disney history at the time, much was hanging on this one being a success. And it was. The revenue from Cinderella would make sure that the studio can continue making animated feature films all throughout the 50’s. It led to classics like Alice in Wonderland, Sleeping Beauty, Lady and the Tramp, and Peter Pan. By the end of the 50’s, all production on animated shorts has ended. The dedicated team was then reassigned to television programs.In 1966 Walt Disney died, leaving behind a massive legacy of animated feature-films and shorts, as well as an entire park dedicated to his studio’s work; Disneyland. Throughout the 60’s, 70’s, and 80’s, the style of Disney animation was further defined with the use of Xerography. It allowed for colors to be photo-chemically transferred on paper, rather than having to trace every single cell. The results of this was a scratchier line-art that would become the staple of the Disney style until the 90’s.Things were calm at the studio until 1979, when Don Bluth and other animators felt dissatisfied with the stagnation of the production and left to form their own studio; Don Bluth Productions. The loss of so many talented animators delayed the production on The The Fox and The Hound and The Black Cauldron. Don Bluth would continue to be Walt Disney Studio’s biggest rival throughout the 80’s. Don Bluth wouldn’t be the only talented artist to leave Disney, as Disney fired John Lasseter for pushing the studio to explore computer animation, leading him to work for Pixar.The 80’s were also ripe with corporate drama. Roy E. Disney, Walt Disney’s nephew, launched a campaign called SaveDisney to convince the board to fire Ron Miller, Walt Disney’s son-in-law and CEO of Walt Disney Studio. Roy succeeded and brought in Michael Eisner as the new CEO. Eisner’s new appointment would end up coming at a really bad time. The studio’s most expensive animated film at the time, Black Cauldron, became a massive critical and financial flop.Eisner wanted to focus on live-action films and outsource all animation production. Roy managed to convince him otherwise and offered himself as the new head of animation production to help turn the tide. Fortune favors the brave as The Great Mouse Detective was released in 1986. The film was made in just over a year and cost as little as 14 Million Dollars to produce. It saved the animation department as it became a critical and financial success.The success kept on pouring in, with the release of Oliver and Company in 1988. It became the highest grossing animated film at the time, beating Don Bluth’s The Land Before Time.Walt Disney Studio then expanded its territory by buying up the Hannah Barbera Studio in Sydney, Australia, as well as opening up a new studio in London. It would animate portions of Who Framed Roger Rabbit, and three Roger Rabbit animated shorts. Another studio was established in the Disneyland theme park in Florida, allowing people to watch the animators at work.With the development of the Computer Animation Production System by Pixar, which allowed computer generated images to seamlessly integrate with hand-drawn animations, a new period in Disney animation history started; The Disney Renaissance. The first animated film to use this technology was The Little Mermaid, which became a major blockbuster. It was followed shortly by The Rescuers Down Under, Disney’s first sequel. Even though the latter received a lukewarm reception, all the other films in the Renaissance period were massive successes.It was films like The Little Mermaid and The Beauty and The Beast that would lead to the well-known Broadway musical-comedy formula of Disney films to come.Corporate tension rose again with the dawn of the 90’s and the decline of the lucrative Disney Renaissance. Jeffrey Katzenberg, chairman of the film division, started taking much of the credit for Walt Disney Feature Animation’s successes. It got Katzenberg fired from his position. He would later become one of the founders of Dreamworks Animation SKG, one of Disney’s biggest competitors. The 90’s would also see fluctuating revenues with hits like Lion King, and flops like Pocahontas. This led to the studio hiring executives to closely supervise future productions to increase productivity and market value of upcoming films, to the dismay of overworked animators.The turn of the century would see Disney’s very first attempt at CG animated films, with the release of Dinosaur in 2000. It was the first project of the newly established CG dedicated studio The Secret Lab. The film managed to earn in 349 Million Dollars against its 128 Million Dollars budget, but the board still considered it a flop. The Secret Labs closed its doors. Walt Disney Studio wouldn’t attempt another CG animated film until 2005 with Chicken Little, despite Pixar and Dreamworks seeing massive success with their own CG animated films.Walt Disney Studio would continue to see low revenues. None of the hand-drawn animated films saw much success either, compared to their rivals. This led to the studio focusing more on direct-to-video sequels and TV shows. With the failure of Brother Bear and Home On The Range, the studio decided to convert the animation department into a dedicated CGI animation studio. Unfortunately things didn’t go smoothly as it could, forcing Michael Eisner out of his CEO position. After the lukewarm reception of Chicken Little in 2005, Eisner resigned and Bob Iger took over .With Bob Iger at the helm, Disney and Pixar made a new deal. Walt Disney Studio would buy Pixar for 7.4 Billion Dollars, with one of the terms being that Edwin Catmull and John Lasseter would become president and chief creative officer of both Pixar and Walt Disney Animation Studio.While in office, Catmull and Lasseter prevented the board from closing down the feature animation department. They convinced them to allow them to turn the tables. They also removed mid-level executives, or “gatekeepers”, and personally met with each filmmaker every week to help every project reach its full potential.In 2009 Walt Disney Animation Studio released its second to last hand-drawn animated feature, Princess and the Frog. Even though the film met wide-spread critical acclaim, it still didn’t hold up at the box-office, mainly due to going up against James Cameron’s Avatar. The very last hand-drawn film would be Winnie The Pooh. It also saw great reviews, but low revenue. Tangled was released in 2010, and was the most expensive animated film in Disney history with a budget of 250 Million Dollars. The film was a massive success both critically and financially, and would mark the period in which Walt Disney Animation Studios fully embraced CGI as a viable and lucrative animation style.The studio has been around for almost a century, and it has long been considered the pinnacle of animation worldwide. The animation techniques and rules they developed became the industry standard, even at the advent of more modern CG animation.At current date the studio has produced 55 animated feature films.Highest awarded film:Frozen (72 Awards)Highest grossing film:Frozen (1.287 Billion Dollars)Dreamworks Animation SKGLocation: Glendale, California, USAAnimation style: CG AnimationFounded: October 12th, 1994Founder(s): Steven Spielberg, Jeffrey Katzenberg and David GeffenEmployees: 2700Notable employees: John Stevenson, Mark OsborneStudio History:In 1994, film director Steven Spielberg, former Disney chairman Jeffrey Katzenberg, and music executive David Geffen got together to create Dreamworks SKG. A division of the film studio Dreamworks, owned by Steven Spielberg. To build up the workforce, Spielberg brought in animators from his own animation studio, Amblimation. Additionally, Katzenberg siphoned top animators from Walt Disney Feature Animation.Dreamworks SKG first broke into the industry with the CG film, Antz, and a hand-drawn film, Prince of Egypt. They released both in 1998. In 1997 Dreamworks partnered with the British animation studio Aardman Animations to co-produce and release their next stop-motion film; Chicken Run. They extended the deal to four more films in 1999. Dreamworks would help with the production of stop-motion films in the UK and Aardman would help with CG films in the US.In 2001, Dreamworks Animation Studios released Shrek to great critical acclaim and audience response. The film won an academy award for Best Animated Feature Film. Due to the success of Shrek, Dreamworks decided to scrap all hand-drawn animated projects in favor of focusing on CG animation.In 2004, Dreamworks SKG changed its name to Dreamworks Animation SKG. Additionally, Dreamworks bought up the majority of stocks at Pacific Data Images to integrate it into the production. Apart from the films produced by Aardman, all films after Shrek 2 and Shark Talewould be entirely CG. This was the first time Dreamworks Animation SKG released two animated films in one year.When Dreamworks SKG became Dreamworks Animation SKG, Jeffrey Katzenberg took over as head of the division. Steven Spielberg and David Geffen acted as investors and consultants.In 2006 they entered into a distribution deal with Paramount Pictures. This gave paramount worldwide distribution rights to all Dreamworks Animation films, until either a twelfth release or December 31st, 2012. The same year, the partnership between Dreamworks and Aardman ended due to creative differences after the release of Flushed Away.As the studio entered a new decade, Dreamworks announced that they would release five films every two years. However, Katzenberg decided to not fully commit into such a task quite yet. However, in 2010 the studio became the very first to release three animated feature films in one year.In 2012 Dreamworks acquired Classic Media, and launched Oriental Dreamworks in China. The same year a renewal for the Dreamworks/Paramount distribution deal was up for negotiations. However, Paramount tried to swing the deal more to their own favor. Dreamworks started looking elsewhere, specifically Sony and 20th Century Fox. They ended up going for 20th Century Fox for a five-year distribution deal for worldwide release, but Paramount retained the right to distribute previously released films.The last couple of years would see a scramble from the studio to keep itself afloat. The release of too many films with massive budgets in a short timespan took its toll. The studio was constantly struggling, no matter how much they earned. They started closing down parent companies to save money, as well as selling off one of their campuses in California and cutting down releases to one film every two years.Highest awarded film:Shrek (38 Awards)Highest grossing film:Shrek 2 (441 Million Dollars)Sony Pictures AnimationLocation: Culver City, California, USAAnimation style: CG AnimationFounded: May, 2002Employees: 50Notable employees: Genndy TartakovskyStudio History:Sony Pictures Animation came to be after Sony considered selling the VFX division Sony Pictures Imageworks, but there were no buyers. Instead, they decided to split it into a CG animation studio after doing impressive work on Stuart Little 2, and seeing how much revenue movies like Shrek brought in. Sony Pictures Imageworks would continue to work on visual effects, while Sony Pictures Animation focused on animated films.The studio’s first film was the adaptation of the Japanese animated series Astro Boy. It was in development since 1997. The film did not do well in cinemas, but Sony still had faith in the studio and charged the studio with making three more animated films; Open Season, Cloudy With A Chance of Meatballs, and Surfs Up. All three saw great successes, and Surfs Up even won the studio its first Oscar award.In 2007 Sony Pictures Animation partnered up with Aardman Animations after a falling out between Aardman and Dreamworks. Together they worked on the animated Christmas film Arthur Christmas and the pirate film Pirates: Band of Misfits.Currently Sony Pictures Animation is working on nine separate animated films set for release between 2017 and 2018.Highest awarded film:Hotel Transylvania (3 awards)Highest grossing film:Hotel Transylvania 2 (169.7 Million Dollars)Blue Sky StudiosLocation: Greenwich, Connecticut, USAAnimation style: CG animationFounded: February, 1987Founder(s): Alison Brown, David Brown, Michael Ferraro, Carl Ludwig, Dr. Eugene Troubetzkoy and Chris WedgeEmployees: 600Notable employees: Steve Martino, Chris Wedge, Carlos SaldanhaStudio History:Blue Sky Studios was founded in 1987 after the VFX studio MAGI, previously working on Tron, closed its doors. This led to some of its former employees to found their own studio.Using its own in-house rendering software, Blue Sky Studios focused primarily on visual effects for commercials and films. Eventually they decided to dedicate themselves toward CG animation.With a team consisting of everything from artists to theoretical physicists, and even a NASA engineer, the studio work towards their ambitions of becoming a one of the leading CG animation studios.In 1997, 20th Century Fox acquired Blue Sky Studios via their VFX studio, VIFX, to work on visual effects on X-Files, Blade, Armageddon, Titanic, and Alien: Resurrection.In 1999, Fox had sold off VIFX, and was considering selling of Blue Sky Studios as well. Instead, they decided to give them the chance to make their own CG animated film; Ice Age.The film was a massive success, and led to following Pixar and Dreamworks’ road of successful animation production.Highest awarded film:Ice Age (5 awards)Highest grossing film:Ice Age (484.6 Million Dollars)Illumination EntertainmentLocation: Santa Monica, California, USAAnimation style: CG AnimationFounded: 2007Founder(s): Chris MeledandriEmployees: 300Notable employees: Pierre CoffinStudio History:Chris Meledandri was the president of 20th Century Fox Animation, where he supervised the production of Ice Age, Alvin and the Chipmunks, Robots, and Horton Hears A Who, but in 2007 he left the company to start his own production studio.The next year, Meledandri made a deal with Universal to produce at least one film a year, starting from 2010. Illumination Entertainment retained their independent right to work on whatever they wanted within the deal. In 2011, they acquired the French animation studio Mac Gruff. They put them to work on what would become the studio’s most popular franchise; Despicable Me.Chris Meledandri had stated that the reason behind the success of his studio is keeping all projects under 100 Million Dollars. They use cost-effective animation and rendering technologies to keep the budget down without affecting the quality.Highest awarded film:Despicable Me 2 (12 awards)Highest grossing film:Minions (1.159 Billion Dollars)Aardman AnimationsLocation: Bristol, England, UKAnimation style: Stop-Motion animationFounded: 1972Founder(s): Peter Lord and David SproxtonNotable employees: Peter Lord, Nick Park, Steve BoxStudio History:Aardman Animations was founded by Peter Lord and David Sproxton with the ambition of animating a feature-length film. Their first step into that goal was working for BBC on a series called Vision On. A kids TV show aimed at deaf children.They stepped into the field of adult animations when they produced Down and Out and Confessions of a Foyer Girl for BBC’s Animated Conversations. They based it on real-life sound recordings. This lead to Aardman Animations working for Channel Four on the Conversation Pieces series. It created a new form of animated comedy by matching animations to sound recordings. In 1985, Nick Park (who Lord and Sproxton already knew from their time at the National Film & Television School) joined the team.What would eventually put the studio on the map was their internationally famous music video for Peter Gabriel’s Sledgehammer in 1986. It led to them producing the music video for Nina Simone’s My Baby Just Cares For Me in 1987.During Aardman’s period of working for Channel Four, Lord and Sproxton started recruiting new directors and animators to their team. They used their new series Lip Synch as a sort of test for up and coming talents. Along with Lip Synch, Aardman created several other animated shorts and series.One of those creations was Creature Comfort, an animated series in which animals talked about what’s it like living in a zoo. This series won an Academy Award for Best Animated Short film in 1990.In 1993, Aardman Animation completed a short film that would contain characters that would become the head figures of the Aardman Animations brand of animation and characters.Wrong Trouser told the story of the inventor Wallace, who lives with his dog Gromit, creating a pair of mechanical trousers that does all the walking for the wearer. The short film went on to win thirty awards, including an Academy Award. Just two years later Aardman Animations won their third Academy Award for their short film A Close Shave, which cemented Wallace and Gromit as a household name and the studio’s mascots.In 1997, Aardman Animations entered into a partnership with Dreamworks SKG. It says they would co-finance and co-produce Aardman’s first feature-length film; Chicken Run. In 1998, Aardman signed a new contract with Dreamworks, in which they would produce four animated films in a period of twelve years. Their first film for Dreamworks was suppose to be The Tortoise and The Hare, but it kept getting delayed due to script issues, so Chicken Runbecame the first film, released in 2000 after four years of production.In 2005 Aardman released their second feature-film; Wallace and Gromit: Curse of the Were-Rabbit. This would be the first time the characters of Wallace and Gromit would appear in cinema. The movie earned the studio its fourth Academy Award. Unfortunately, later that year, a fire broke out at a storage facility in Bristol, destroying most of the models, equipment and art used for Aardman’s productions, including thirty awards collected by the studio for its achievements. Luckily they stored all their films somewhere else.In 2006 Aardman completed the work on Flushed Away, the studio’s first CG animated feature-film. As it was with Chicken Run, Flushed Away was a close co-operation between Aardman and Dreamworks. However, with the release of their latest film, Aardman announced that they would no longer work with Dreamworks due to creative and financial differences. The contract has officially ended the next year. Before the contract ended, Aardman was working on Crood Awakening, which was co-written by John Cleese. However, when thy cancelled the contract, the film rights reverted to Dreamworks, who renamed the film The Croods and rewrote the script.In 2007, Aardman signed a three-year contract with Sony Pictures Entertainment to finance, co-produce and distribute their films. Aardman returned to making animated shorts after Flushed Away. They release of more Wallace and Gromit shorts, and created a new IP; Shaun the Sheep. The studio also created a new series of Creature Comfort shorts, aimed at the US market.In 2008 Aardman also partnered up with Tate Museums and Legacy Trust UK. They asked children all over the UK to make suggestions for a film to promote creativity. The film was The Tate Movie Project, and set the Guinness World Record for most contributors in a single film project. Then they joined up with Channel Four to create 4mation, a portal for user-generated animations. Then releasing a series of flash games on Newgrounds, and joining up with Nintendo on a challenge to create animations using only the Flipnote Studio on a Nintendo DSi.The first film to arrive from the Aardman and Sony partnership was Arthur Christmas in 2011. It was Aardman’s second CG film, but it was their first 3D film. In 2012 they released their next feature-length stop-motion film; The Pirates! Band of Misfits. This film would become the very first stop-motion animated film screened in 3D. Then in 2015, Aardman Animations bought up the majority shares of the New York based animation studio Nathan Love.Highest awarded film:Wrong Trousers (30 Awards)Highest grossing film:Chicken Run (224.8 Million Dollars)Studio GhibliLocation: Koganei, Tokyo, JapanAnimation style: 2D AnimationFounded: June 15th, 1985Founder(s): Hayao Miyazaki, Isao Takahata, Toshio Suzuki and Yasuyoshi TokumaEmployees: 300Notable employees: Hayao Miyazaki, Gorô Miyazaki, Isao TakahataStudio History:When they founded in 1985, Hayao Miyazaki and Isao Takahata were already seasoned veterans in the Japanese animation industry. They built the studio up on the success of their first film Nausicaä of the Valley of the Wind in 1984. This film would also lead to the studio’s strict “No-Edit” policy, due to the heavy editing it received prior to the North American release.It is technically not a Studio Ghibli film, since Topcraft produced it and Toei Company distributed it, but it is still the studio’s first feature-film.Toshio Suzuki, who had a long career as a producer and editor, joined the team. Along with Yasuyoshi Tokuma – Studio Ghibli was born. Hayao Miyazaki directed most of the studio’s films, but others would take the director seat as well. His partner Isao Takahata and his son Gorô Miyazaki.In 1996, Studio Ghibli partnered with Disney, letting them distribute all their films internationally. Toho worked on domestic releases. Studio Ghibli released Princess Mononoke in 1997. It became the studio’s first international hit and earned the studio its first Academy Award at the Best International Film category. It was the first animated film ever to win that award.The film would also further cement the “No-Edit” policy. The co-chairman of Miramax, Harvey Weinstein, wanted to re-edit the film to suit the US market. One of Studio Ghibli’s producers then sent him a Japanese Katana, with a simple message saying “No cuts!”.In 1999 Tokuma Shoten bought Studio Ghibli. By 2001 the Studio Ghibli museum opened to the public, containing exhibits from their films. It even showed animated shorts that were never released. In the same year, the studio released Spirited Away. It became a massive hit internationally, and the studio’s highest earning film, winning them their second Academy Award. Then, in 2005, Studio Ghibli bought themselves up and became an independent studio.In 2013, Hayao Miyazaki announced that he would retire as a director. In 2014 Suzuki announced that Ghibli would step back and reconsider their options because of Miyazaki’s retirement. Since then Studio Ghibli hasn’t released any films, other than the re-release of Only Yesterday from 1991.Highest awarded film:Spirited Away (53 Awards)Highest grossing film:Spirited Away (289.1 Million Dollars)Laika EntertainmentLocation: Hillsboro, Oregon, USAAnimation style: Stop-motion AnimationFounded: 2005Founder(s): Phil KnightEmployees: 395Notable employees: Henry Selick, Anthony F.Stacchi, Graham AnnableStudio History:Laika Entertainment had its start as a completely different studio, called Will Vinton Studio, which also worked primarily in stop-motion. In the 1990’s, the studio was struggling financially and was looking for investments where ever they could. Enter Phil Knight, who invested in the studio in 1998.In 2002, Phil Knight bought up the studio and re-purposed it into a feature film production studio.The next year the studio Henry Selick joined the studio. He previously worked as a director on Tim Burton’s Nightmare Before Christmas. In 2005, Will Vinton Studio changed its name to Laika Entertainment, and split into a film and commercials divisions.The first film from the studio was Coraline. It was a modest success, but was praised critically and nominated for an Academy Award. This was supposed to be followed by Jack & Ben’s Animated Adventure, but the film was cancelled, which led to the studio laying off a sizeable portion of its staff.Henry Selick left the studio in 2009, due to a failed contract negotiation, despite his success with Coraline and Moongirl. Afterwards the studio laid off more of its staff, particularly in the CG department. They released Paranorman in 2012 to great accolade, but earned in less money then Coraline did.In 2014, the commercial production department of Laika Entertainment detached from the main studio, and became House Special. The same year, the studio released Boxtrolls. The film didn’t do better than ParaNorman at the box-office, but it did earn the studio its very first Oscar. Currently the studio is expanding its operation, thus allowing them to release at least one film per year.Highest awarded film:ParaNorman (14 Awards)Highest grossing film:Coraline (124 Million Dollars)
- Home >
- Catalog >
- Life >
- Handicraft Template >
- Numbers Templates >
- Number Cards Templates >
- free printable numbers >
- Mapping Nominal Values To Numbers For Effective Visualization