The Guide of finalizing A Model For Efficiency Analysis Of The Customer Satisfaction Process Online
If you are looking about Modify and create a A Model For Efficiency Analysis Of The Customer Satisfaction Process, here are the easy guide you need to follow:
- Hit the "Get Form" Button on this page.
- Wait in a petient way for the upload of your A Model For Efficiency Analysis Of The Customer Satisfaction Process.
- You can erase, text, sign or highlight through your choice.
- Click "Download" to download the materials.
A Revolutionary Tool to Edit and Create A Model For Efficiency Analysis Of The Customer Satisfaction Process
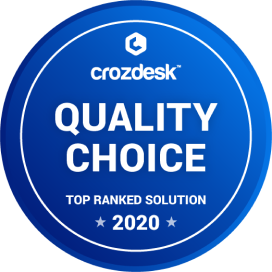
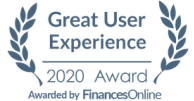
Edit or Convert Your A Model For Efficiency Analysis Of The Customer Satisfaction Process in Minutes
Get FormHow to Easily Edit A Model For Efficiency Analysis Of The Customer Satisfaction Process Online
CocoDoc has made it easier for people to Fill their important documents by the online platform. They can easily Fill through their choices. To know the process of editing PDF document or application across the online platform, you need to follow these steps:
- Open CocoDoc's website on their device's browser.
- Hit "Edit PDF Online" button and Upload the PDF file from the device without even logging in through an account.
- Edit your PDF for free by using this toolbar.
- Once done, they can save the document from the platform.
Once the document is edited using online browser, the user can export the form as what you want. CocoDoc ensures that you are provided with the best environment for implementing the PDF documents.
How to Edit and Download A Model For Efficiency Analysis Of The Customer Satisfaction Process on Windows
Windows users are very common throughout the world. They have met millions of applications that have offered them services in modifying PDF documents. However, they have always missed an important feature within these applications. CocoDoc intends to offer Windows users the ultimate experience of editing their documents across their online interface.
The steps of modifying a PDF document with CocoDoc is simple. You need to follow these steps.
- Pick and Install CocoDoc from your Windows Store.
- Open the software to Select the PDF file from your Windows device and continue editing the document.
- Fill the PDF file with the appropriate toolkit appeared at CocoDoc.
- Over completion, Hit "Download" to conserve the changes.
A Guide of Editing A Model For Efficiency Analysis Of The Customer Satisfaction Process on Mac
CocoDoc has brought an impressive solution for people who own a Mac. It has allowed them to have their documents edited quickly. Mac users can easily fill form with the help of the online platform provided by CocoDoc.
To understand the process of editing a form with CocoDoc, you should look across the steps presented as follows:
- Install CocoDoc on you Mac in the beginning.
- Once the tool is opened, the user can upload their PDF file from the Mac easily.
- Drag and Drop the file, or choose file by mouse-clicking "Choose File" button and start editing.
- save the file on your device.
Mac users can export their resulting files in various ways. Not only downloading and adding to cloud storage, but also sharing via email are also allowed by using CocoDoc.. They are provided with the opportunity of editting file through multiple methods without downloading any tool within their device.
A Guide of Editing A Model For Efficiency Analysis Of The Customer Satisfaction Process on G Suite
Google Workplace is a powerful platform that has connected officials of a single workplace in a unique manner. While allowing users to share file across the platform, they are interconnected in covering all major tasks that can be carried out within a physical workplace.
follow the steps to eidt A Model For Efficiency Analysis Of The Customer Satisfaction Process on G Suite
- move toward Google Workspace Marketplace and Install CocoDoc add-on.
- Attach the file and Push "Open with" in Google Drive.
- Moving forward to edit the document with the CocoDoc present in the PDF editing window.
- When the file is edited ultimately, share it through the platform.
PDF Editor FAQ
How do designers set prices for dresses and why do dresses often cost more on a designer's website than on purchase through an online retailer?
This is deceptively complex question as the answer requires an explanation for how consumer-fashion brands price products and subsequently the factors that drive price deviations. This answer is broken up into 2 parts:1. How are prices in consumer-fashion set?: This reviews the actual pricing analysis from two of previous footwear brands with illustrated examples to explain how pricing is done in the real world.2. Why are prices on a brand’s site different from a retailers? Illustrates the market, organizational, and strategic challenges facing the current landscape for consumer-fashion. These factors drive the price discrepancies & explains why they occur.As many of you know, I run a brand called Cloven Footwear - a men’s outdoor footwear brand that just launched SS12. For purposes of answering the question, I will illustrate in-depth analysis of how we priced one of our launch models and the problems that are inherently involved in the process. Since Cloven has not been in the market for consumers to purchase, the answer will pivot into actual data from the VÆL Project’s Deckard Boot to provide more insight into the observed pricing deviation.1. How are Prices set in Consumer-FashionConsumer-fashion is a bare knuckle, knock ‘em down, drag ‘em out brawl that is hands down on eof the most challenging, engaging, and addicting industries that I have ever worked in. It is an insanely ambiguous and obfuscated operating environment surrounding the horrendously complex brand development and diffusion processes.In order to effectively serve the marketplace, the consumer-fashion industry is split into two complimentary segments of brands and retailers. Brands focus on designing, producing, and delivering great products to the marketplace that engage their target demographic. Retailers focus on optimizing their brand assortment, inventory quantities, and demand generation that maximizes product / market fit for each retail location or property.GAP’s terrible financial performance throughout most of the aughts is probably the best example of the risks & challenges that can plague a vertically integrated retailer when one of these two divisions is lost (in GAP’s case it was that they lost their product-mojo and are only just starting to get it back). Hence the specialized focus of these two partners has governed the strategy for consumer-fashion & retail for the better part of the last 20 years - the core reasons that this has been successful the business model are:Retailers:Derive long-term value from major investments in building customer mindshare (i.e. ‘I think that I am going to stop by Bloomingdales on Saturday’)Have extensive customer insights that enables retailers to optimize their merchandising assortment of brands, styles, and inventory quantities dynamically for each location (i.e. GAP only sells GAP stuff and that’s risky if the GAP product sucks)Trying to own the brand side is hugely expensive & massively risky when the entire organization has been built around the retail sideBrandsGreat product design & authentic brand narratives emerge from data-informed & design-driven cultures (versus being reactionary in data-governed design - although Zara and H&M are dialing in a damn good solution that could prove this wrong)Brands begin life by focusing on one product vertical (i.e. Cloven for men’s outdoor footwear) & don’t have the resources nor the product depth required in the demand generation model (remember we are talking about the last 20-years - we’ll get to how the world is today)Need the freedom to work with hundreds of retailers to effectively distribute inventory risk and disseminate the brand narrative to ‘get the word out’Before the onset of the Great Recession & technical innovation began to sow the seeds of change, consumer-fashion brands’ B2B wholesale (i.e. retailers) generated around 80 - 90% of revenue from retailers for the first 2-years. This relationship creates two transaction points where the product is 2x the cost basis for the preceding transaction - a theory in retailer called “Keystone Markup”.Keystone Markup is a pricing methodology that multiples the cost basis by a factor of two (sometimes can be up to 5x in the case of jewelry) to dictate the price for next rung in the value chain. Keystone markup arose as the simplest way to universally markup goods across the retailer to a profitable level. Generally speaking, it is logistically impossible to uniquely price each product (from 100s or even 1,000s in a given retail location) to reflect market conditions and retail demographics. The theory being that retailer profitability was more of a function of units sold versus maximizing each product’s price. Subsequently, this approach normalized prices across the marketplace (i.e. everyone is pricing a shirt at $100).Brand to Retailer: This is the B2B wholesale transaction where the brand sells products to the retailer at wholesale price. This B2B price is based on theory of audiences of scale (i.e. millions of customers going to Nordstrom brick and mortar retail stores and online properties) who should receive a discounted price (off retail price) for the retailer’s contribution in the consumer fashion dichotomy. The brand sells to hundreds of retailers to provide them with the volume of units required to run their business profitably.Retailer to Consumer: This is the B2C retail transaction where the retailers sells product to the customer at retail price. The retailer sources product from hundreds of brands to dial in their product mix for selling product to their customers.Now that we have established the basic building blocks for pricing, we need to establish the market rate to understand how individual products are priced in the marketplace. When you are in the beginning stages of launching a brand, you do a ‘market analysis’ to clearly define the competitive landscape. This competitive analysis determines the corresponding balance of style, price, and quality that enables the brand to most effectively capitalizes on the opportunity.The product’s price must reflect a value proposition relative to where the competition is positioned. The rationale is that the overall product landscape establishes the data points that a customer will evaluate the product relative to what the customer sees as comparable goods. In consumer-fashion there are general pricing bands that establish certain boundaries for potential pricing. For example, one of the launch styles for Cloven was a boat shoe where the pricing bands for a mid-tier boat shoe are about $70 - $90 retail price. This means that pricing the product outside of this range immediately draws a red flag in the eyes of the consumer as being out of place (i.e. not in the $70 - $90 expected price range) - so pricing is heavily dependent on consumer expectations.There are two important aspects of this foundation:Prices are confined to ranges of established norms outside of the brand’s control & constrained by the consumer’s expectations (i.e. ‘if you are going to make product for this market, you have to price within this range to even be viable’)The price range is a subjective analysis of the competitive landscape from the brand’s perspectiveTo illustrate how prices are set, let’s roll through the process for how Cloven conducted the market analysis and subsequently arrived at our retail price.1. Market Product Opportunity Analysis: The strategic analysis of how competitors have positioned their brands & products by price - where price is the defining metric to govern the product development of the startup2. Define Strategic Brand Position: Formally establishes where the brand will sit in the marketplace to serve as the unifying creative direction of the brand.The potential price is inherently defined by how the brand needs to position its product relative to where the competition is. The logic behind Cloven’s pricing is:The market is defined by the Sperry’s Authentic Original boat shoe - it has been in the market since 1935 and is one of the first shoe styles ever created - anything that Cloven would produce would be compared relative to this anchor product.Cloven uses a super interesting technology that will offer a dramatic improvement on fit & comfort over current Outsoles used in production - leading to a fit and comfortable advantage over the competitionCloven uses Outsole & Midsole tech to reduce leather quantities generating a lower cost basis that allows Cloven to compete on price as a small company without economies of scalePricing below Sperry will offer an incentive to the customer to try on the product - competing on product quality for brand-insensitive customers (i.e. ‘I don’t care what brand it is so long as it fits well’)[Note: For the rest of this answer, we are going to pivot to an actual example from my old brand VÆL Project’s Deckard Boot.]3. Wholesale Price: Wholesale price is the price charged by the brand to the retailer for product that they have ordered - it’s revenue to the brand and represents the cost basis of the retailer. The general rule of thumb is that wholesale price is a 2x keystone markup on the first cost (the total cost to create finished goods ready for sale in the marketplace) or in other words - wholesale price is a 50% discount (0.5x) retail price.However, the simple world of 2x keystone markup is ending as ERP and IT Systems enable new insights in all aspects of the retail business that were previously abstractions based on averages. Today, most brands are under considerable pricing pressure from retailers to markup wholesale price at less than 2x to provide the retailer with the margin required to profitably sell the product. Retailers are marking up wholesale price 2.1x to 2.4x to compensate for inventory markdown & return risks that delivers the overall margin required to achieve the profitability goals of the firm. This focus on margin is a function of increased adoption of IT Systems that allow deeper insight into product performance on the brand and product level versus the obfuscated aggregate view that plagued organizations just 5 - 7 years ago.Despite the major strides in retail analytics, it’s still a major challenge to dynamically price products based on demand signals and current stage of brand development - subsequently, retailers generally apply a universal markup on wholesale price to derive the retail price. Both brands and retailers live and die by the wholesale price - if it’s too low then the brand risks not generating enough Contribution Margin to run operations and conversely if its too high then the brand risk pricing the product outside the optimal competitive price range.The important aspects for a brand’s wholesale price are:Wholesale price is a dance that requires the brand to balance the scope of retailers markup strategies with the realities of order volume and profitability constraints on funding the brand’s operationsRecognizing that setting the wholesale price on the high end of the range allows the brand to always back off the price through discounts on product (i.e. 5% off wholesale price for 1,000 units) or marketing discounts (i.e. 5% discount for ad-spend or marketing placement).This is an example for how pricing works in the market:4. Product Development: After you have your target price and wholesale price, you begin to work backwards to define your costing & materials. The operating cycle in consumer-fashion is traditionally 12 - 15 months meaning the from the time you have your design to when it finishes its basic life cycle in the market.One of the main problems with the launching a new brand is that you have absolutely zero pricing power - meaning that you have the market setting the price within a narrow range & subsequently have a high cost basis to manufacture product. This begins the most challenging part of this stage - the tweaking of materials to levels that deliver sufficient quality right up to the monetizable equilibrium between the quality of the materials, construction of the product, and the customer’s willingness to pay for these costs. Keep in mind that $0.50 in manufacturing costs equals about $2 at retail (2x markup to wholesale and 2x markup to retail).Here is an example of the product development revisions made to the VÆL Deckard Boot to dial in the first cost subject to the constraints of wholesale price & retail price.We are in a very interesting period in the modern business environment as the fundamental business model that has ruled consumer-fashion for the last 20-years (what was referred to in the 90s as the ‘China Strategy’) is dying - the world of cheap goods is over. Factories in China are closing and the good factories are dropping small & unprofitable brands to stem the losses from 20% real wage growth & materials inflation like leather (cows eat lots of corn & most hides are shipped from the US to China for tanning).In footwear particularly, a brand has essentially zero pricing power or ability to generate economies of scale for a considerable period of time. The break points are generally around 15,000 pairs, 45,000 pairs, 80,000 pairs, 150,000 pairs, 400,000 pairs where prices drop by 10% - 15% successively at each interval.===============================================Why do dresses cost more on a designer's website?===============================================Consumer-fashion at its core is highly tactical, deeply psychological (a style/trend gaining momentum is highly reflexive of the mood that pervades social-cohorts), and heavily dependent on intellectual-capital (i.e. aesthetic, creative, and strategic direction requires immense cognitive investments).Direct business (brands/designers selling product directly to the customer via it’s website) has been an ancillary consideration to most brands (i.e. the direct business took the back seat to wholesale (brands traditional B2B revenue channel for major retailers like Nordstrom & Bloomingdales).2.1. Conflicting Priorities Destroy Feedback Loop:One of the fundamental problems driving price deviations in consumer-fashion is the dearth of communication & data sharing that adversely inhibits the development of a healthy & efficient feedback system between the two primary vertical partners, Brands & Retailers. This is a structural problem that arises from the business cycle at two distinctly unique phases:1. The Buying Cycle:The traditional (... and rapidly deteriorating) business model for consumer-fashion revolves around 9-month seasonal sales cycle, where 80% of a brand’s revenue is generate through B2B wholesale orders place by retailers (via ‘buyers’ - the person/team responsible for procuring inventory from brands that achieves the optimal merchandising assortment for the retailer) at major industry trade shows. The importance of trade shows in the archaic business model for consumer-fashion arose to provide a convenient, centralized location and time frame for retailers to touch, look, and feel all the products from hundreds of brands, dial in their merchandising assortment for complimentary products (i.e. boots from Brand X that would look great with the selvage denim from Brand Y) in direct, comparative context, and account for the production lead times for brands to manufacture the goods.At the trade shows, brands exhibit their seasonal collections of ‘samples’ - the array of potential products that are in the final stages of product development and represent the full product mix. Buyers subsequently place orders for the styles, variations, and quantities that they believe will work best for their customer based on what other products in the pipeline, the goals of the merchandising assortment, the retailer’s brand & market position, customer & sales development strategy, and customer demographics. Obviously, brands are only going to go into production on the styles & variations (variations are generally the different colors - they have the same parent style, but differ by color) that retail buyers have placed firm orders to produce.The trade shows are scheduled around the seasonal production cycle for brands:Jan - Feb: Trade ShowsMar - Jun: ProductionJul - Aug: Shipping & Inventory TurnSep - Nov: Selling SeasonThis process inherently does not make sense to me because an entire segment of the consumer-fashion industry is basing major, life-and-death decisions on product volumes for inventory based on a proxy demand signal - the retailer buyer serving as a representative agent for consumer demand. Now the most accurate forecasting methodologies still involve are a weighted algorithmic + Delphi Expert Model, but even with the incredible strides in retail analytics - the concept of elite group of people (buyers) controlling major segments of the ecosystem through their assessments of what they like or believe the customer likes. The prevailing theory that drove the evolution of this model was that buyers have all of the extensive customer intelligence gained by the retailer that is inherently put into practice through the B2B wholesale order placed with the brand at the trade show.The major problems with this stage of the pricing model are:- The order placed the buyer is a based on limited historical data constructed by vanity-analytics like gender, category, and price - these top-line numbers are generally too broad to deliver true insights to account for brand-specific factors- This order does not take into account endemic brand factors like the current stage of brand development, customer mindshare, marketing & promotional activities of the brand - all demand signals that will substantially impact retail performance- There is a disconnect between the end-customer even having the opportunity to see the scope of the product landscape (products that perform poorly with buyers at the trade shows are dropped and never make it to retail) - buyers are making decisions for the customer as their representative agents with only tertiary support of this relationship (by monetarily supporting the retailer based on the product assortment)Bottom line for how this influence priceThe marketplace is directed by buyers:placing orders for product constraining the product scopeforecasting quantity demanded by top-line data that doesn’t factor any endemic brand-specific factorsorder determined 9 - 12 months before the product hits retail shelves and the customer even has the opportunity to product ‘market fitting’ feedback[Note: I am not trying to take a piss on buyers - I have an immense amount of respect for what they do and consistently am awestruck by some of the most talented buyers in the industry.Being a great buyer requires that they procure products that customer’s will purchase for the current season, but they need to build a product pipeline of demand for styles that they are financially judged against. This means that they need to stay ¼ step ahead of their customer falling into 3 - 5 different customer-demand narratives to balance current demand while seeding trends for the next season. Great buyers introduce a style one season as an outlier styles that drive purchases next season to capitalize on demand as the trend diffuses over the current season. Furthermore, buyers need to prime for trend shifts that will be hitting the market in one year (and subsequently require introduction today to incept the idea and begin to trigger the want/demand at the correct time).Buyers are what we would know today as curators except they are under incredible levels of stress as their decisions are made way in advanced and responsible for financial performance of the retailer. If a buyer screws up one time - they can destroy profitability for entire product lines or divisions. After spending a lot pf time working with buyers over the last 10-years, I generally don’t like a lot of buyers because of the ‘too cool for school’ mentality that drive purchasing decisions based on ego rather than data-informed and intelligent. Hands down the best buyer in Men’s is Emma Lee, at Gilt Groupe - to say that girl is brilliant is an understatement as I consistently look at what her buys tell me about the industry.]2. The Sales Cycle:This is where the plot thickens and gets really interesting - let’s fast forward 9-months through the ordering, production, and shipping & fulfillment to a retail store - the product is now on the retailers website or store shelves. Customer’s now have the opportunity to purchase the brand’s product. In retail, there are certain sales level that innately occur simply by virtue of product being in the marketplace - the product is on the store’s shelf, the customers it, likes it, tries it on, and buys it - easy peasy lemon squeezy. However the vast majority of sales are generated following about 11-touch points with the trend/style/brand/products - meaning that converting consumers into customers requires on average about 11 touch points with the product on blogs, in marketing, at retail, or the consumers wearing the product to generate sufficient customer mindshare that drives purchase actions.The most exciting data is the on the ground sales staff qualitative data collection and archetypal narrative development. Talk to a really good sales reps at Nordstrom - they have massive mental databases about the most valuable customer behavior and how customers break down aesthetically to certain groups (this is highly subjective data and definitely subject to huge bias - but the fact that it’s based on a significant amount of time & transaction by sales reps building the insight on the actual transaction level makes it far more interesting than #s on a page as each instance is embodied by a visual reference).Remember how in the ‘Buying Cycle’ section, I commented on the prevailing logic was that retailers have all the customer insight? Well, the systems is fundamentally screwed up in that retailers do not share any of this data with their vertical partners - it is viewed as a competitive advantage and subsequently the property of the retailer. This data-silo’ing behavior adversely constrains brand partners from foundation level data to inform the basic comparative framework in the ‘Market Analysis’.Retailers protect this data because they are desperately clinging to this as a competitive advantage (like Networks in releasing content to the web that fuels piracy) and do not share it because they are scared that brands will usurp the retailer and go straight to the customer. Ironically, it’s this protectionism that is forcing brands to build their own consumer-direct relationships to gain this information.This protectionism mentality creates the following main challenges in the ‘Buying Cycle’:Retailers do not have the resources nor the incentive to effectively make use of this purchase data for the 100s of brands that retailers stockDemand generation is the responsibility of the brand - how can brands make meaning use of data to refine the product mix & pricing mix if the market feedback is hidden from them? For example, let’s say I ran a marketing campaign with a discount of 15% off a pair of shoes at Bloomingdales San Francisco. that discount code must be able to be scanned and accepted by the Bloomingdales ERP - meaning that the brand loses any insight about the customer and has given up generating any long term value from issuing this discount.By not sharing feedback like this and relying on the brand to drive demand generation (i.e. the brand driving traffic to the retail partner), the brand is incentivized to full that support from the retailer and focus on owning the customer relationship in a way that creates the most strategic, long term value for the brandIt would make sense to drive traffic to the brand’s core strategic objectives like the brand’s Facebook Fan Page or to the brand’s E-Commerce site to work on building the customer relationship.- How do brands discover marketing channels when customer insights about referral traffic is deliberately hidden from the brand? If the brand is responsible for generating online traffic and sending traffic to Bloomingdales but cannot benefit from the customer insights then how can brands tailor marketing campaigns?- [Most Important] Brands cannot effectively price products without substantive insights into the data feed meaning that there are systemic problems with this modelBottom line for how this impacts pricing: Conflicting priorities between vertical partners drive information asymmetries that inherently inhibit accurate insights into the ‘market fitting’ pricing feedback and leads to widely inaccurate information fueling pricing discrepancies.2.2. High Subjectivity and Large Biases Adversely Impacting the Comparative Pricing Analysis:In Section 1, we discussed that the first stage of pricing involves the brand conducting a market analysis of the competitive landscape. This market analysis establishes the brand’s evaluation of the strengths and weaknesses of competitors products and determines an implicit estimation for how the brand will balance style, price, and quality that will most effectively capitalize on opportunities in the marketplace.This market analysis is an extremely challenging process because of the dearth of information available at the product level (retailers protect this data) and the high degree of managerial bias in evaluating competing brands & products for this framework - there is simply no way to extract transaction data from the marketplace. There are proxy signals like time to discount and channel checking via local retailers, takes time that’s not really practical as pricing decisions are made 9 - 12 months before products hit retail shelves - but no hard data available to brands to truly substantiate traction based and product / market fit effectiveness for products in the analysis.Additionally, the pricing strategy is driven by highly-emotionally biased managers that deeply invested & fluent in brand-specific details. This leads to a managerial bias that outweighs product differences in favor of the brand - most of which is are not reciprocated by the uninitiated new market participants (i.e. customers).This problem is seen all too often in tech when founders focus too deeply on a niche and product features as a source of competitive advantage. For example, let’s take a fictitious daily deal startup that also schedules the local services in conjunction with the daily deal (i.e. eat at this restaurant at 2 PM or the massage at 3 PM). I have heard probably 20 - 30 pitches over the last year where this occurs that the founder will say that the scheduling is what differentiates them from Groupon or LivingSocial and has lost site that features are not a source of competitive advantage. In the same vein, people who work in consumer-fashion (and I, admittedly, have found myself to have done this numerous times in hindsight) become so ingrained with their products that profound product differences to internal team does not translate into how the customer sees it.Furthermore, the current phase of the brand’s development process dramatically skews the comparative analysis. New brands that are just entering the market have little traction and social proof to validate their brand & product strategy leading to an inherent undervaluation of their product and overvaluation of their competitors (the though being “well, this more established player is priced at $80, so we need to be below them because no one knows who we are.”). Subsequently, a brand that has caught fire and are generating substantial traction begin to overvalue their historical success as the basis for vertical product extensions and new found ego-based biases that overvalue their pricing power to command above market rates.Bottom line for how this influences prices: The subjectivity and biases associated with the initial pricing framework is one of the most important factors in understanding why prices deviate between retailers and consequently from retailers to brand direct. The price is established by emotionally-invested managers that are heavily entrenched in relative valuations of their products and do not have the information resources to effectively adjust the pricing strategy before going to market.2.3 Supporting Legacy Revenue Channels with Conflicting AgendasAs we established in section 1, Retail Price is a function of Wholesale Price that the brand sets based on the market analysis and Brand Strategy. In the traditional consumer-fashion retail environment where B2b Wholesale represents upwards of 80% of the brand’s revenue, brands set Suggested Retail Price on their websites at the top end of the marketplace, and often times above the ‘actual’ retail price quoted to retail partners, to support the B2B revenue channel and make retailers appear like a good value / discount.Prior to 2010, it was an inordinately challenging process for brands to generate sufficient online traffic in consumer direct (i.e. BrandXYZ.com E-Commerce Business) to be a strategic priority for most resource starved brands. The prevailing theory was that retailers have made the significant investments to build customer mindshare and owned the customer relationship so it was in the best interest of the brand to drive traffic to their retail partners.For most brands without significant online consumer-direct presences, which was most brands before 2010 when you began to see brands 10Qs pepper in statements targeting 15 - 25% of revenues coming from consumer direct channels, the brand’s site served as an authoritative reference for product information to consumers on price. This meant that on artifically high prices set on the brand’s site and comparatively ‘better’ price on the retailers would generate opportunity costs to drive purchase via the retailer.In the majority of cases, a brand’s site served as an authoritative reference of product information source about brand’s products where an artificially high price would make a comparatively lower price at the retailer assuage concerns with any consumer. With B2B Wholesale responsible for such a large portion of revenues, it was thought that driving a small number of traffic (the aforementioned inordinately challenging process) would assuage price-checking customers concerns to deliver these customers to the most strategic purchasing channel.One of the core value propositions that retailers create is the cultivation of mindshare (‘I am going to go to Bloomingdales on Saturday’) and generation of significant amount of goodwill as the customer - whereby the customer had dealt with the retailer & the brand served as the product of the retailer. If the customer loved the brand, the retailer was the beneficiary of the customer’s goodwill/happiness as the provider of the garment (versus the brand as the producer of the product - it's a level of abstraction away from a direct relationship between product & consumer). The retailer controlled the relationship and therefore is the recipient of the customer's projected satisfaction or enjoyment of the product (as opposed to the brand that created the product).Establishing mindshare & building goodwill deeply connects consumers with retailers - a trend that is even far more pervasive online as offline. Online shopping for consumer-fashion is a world of information asymmetries that, when done properly, engenders significant brand engagement & loyalty (i.e. fanatic Zappos customers thrilled by overnight shipping, free returns, and killer customer service). One of the most interesting things about a high Suggested Retail Price on a brand’s site enabled a certain flexibility for retailers to set prices at optimal levels for their target demographics.To support their retail partners, brands would set the Suggested Retail Price at the top line of the marketplace with the understanding that retailers would adjust their prices to what works for their customers.Historically (i.e. before 10 years ago), if a fashion-savvy teenager wanted to purchase an item from Abercrombie & Fitch & there wasn't one located in the local mall - there were two options: 1. purchase via mail order catalog or 2. wait until they took a vacation to a location that had an Abercrombie retail store. Great consumer-fashion E-Commerce sites understood that their value proposition was in their ability to generate significant loyalty by serving the void in the marketplace that the fashion-savvy teenager wants across the country.This process of E-Commerce sites being one of a very select # of Online Retailers to retail the cool brands has the same impact that boutiques traditionally held & create pretty loyal customers that are branded to one property over another - say Tobi.com to RevolveClothing.com. This is not like daily deal sites like the zero sum game that LivingSocial & Groupon engaged in an epic trench-style war of attrition - customers are predominately StyleABC site or StyleXYZ site with little overlap.By pricing on the high-end, the brand enables the retailers to dial in their pricing model to what works for their customer & explains why this prices can vary significantly from retailer to retailer as well as designer/brand to retailer.2.3.1 Tertiary Drivers in the Legacy Channel1. Cash Requirements:Product Information Management is a major challenge for any E-Commerce company & consumer-fashion brand - especially when technical development is not an in-house core competency of the firm. Therefore, more E-Commerce sites historically implement blanket discounting models - if the product has X% remaining stock after 4-week then the product is automatically discounted by 10%.Once an order to a retailer leaves the docking bay, the ownership of the product changes to the retailer - an accounting & legal concept call FOB or ‘Free on Board’. Thus, the brand has little functional control as the retailer owns the product & has the financial imperative to ‘turn’ the inventory. The brand can do things like threaten to pull the account from a retailer - but this does not happen most of the time because the brand needs the retailer more than the retailer needs the brand.2. Organization Oriented Around B2B Wholesale Business:Brands are still predominately organized to serve the business model of the last 20 years - where sales reps ( glorified order takers ) form the backbone of the revenue generation priorities of the firm. A small consumer-fashion brand ( <$10m) has approximately 3 - 7 sales reps covering geographic locales - remember this model is geared around sales reps who would put samples in the car and drive around their territory to sell shops.In the wake of the financial devastation brought on by the Great Recession, management teams for consumer-fashion companies retreated back to what they know best. Generally speaking these are guys and girls in the 30s & 40s (Gen-y’ers) whose first response is to get back to basics (i.e. the dying 20-year business model) to keep the company alive - thereby reinforcing the sales rep’s sole in the company & managerial support for this model3. Drive Retail SupportMajor retailers were the primary revenue channel for brands & their scale enabled brands to achieve the volumes that made the business attractive. The goal of the brand was to support the retailer and stay “in their good graces” by driving potential traffic to the retailer. Here was the basic strategy:A major retailer like Nordstrom.com will place a pre-season order for 1,300 units with a wholesale price of $105.00The Brand sets the Suggested Retail Price at $245.00, while the retailer prices it at $230 representing a 6.1% discountAssume that seasonal mins are 2,500 units - a minimum is the # of units set by the factory that must be purchased by the brand prior to the order being accepted.Here are how the #s breakdown in this example:By virtue of Nordstrom’s scale, their one test order of 1,300 units ($136k) essentially pays for production for the entire season ($135k). However, the real value is in working with Nordstrom over numerous season, when the numbers can look like:In order to illustrate the huge challenges in building an E-Commerce presence for a consumer-fashion brand, take a look at some statistics from VAEL Project.com in Season 4 of the brand.Keep in mind these traffic #s are based on a brand that first hit the marketplace in Jan ‘08 - so these visitors are based on a product in the marketplace with 1000s of promotional blog articles. In my example about Nordstrom’s, we are showing that Nordstrom *could* be generation something like $5.25m in top line revenue. Additionally, if we extrapolate this VAELProject.com E-Commerce out (assume $40k/mo), we are looking at brand direct at about 8% of total revenue.2.4. Emotional Engagement Drives Pricing Power:I wholeheartedly believe that modern brands are evolving into more substantive, direct, and engaging relationships with their consumers to the level of aesthetic & intellectual peers. This transformation has is roots in the Great Recession when the macroeconomic devastation sparked a reevaluation of consumption psychology to and technology opened the door to a new world. Consumers writhing in pain from the market’s ferociously bite after they played by the ‘rules’ of the aughts (purchased a single family home, worked in secure corporate America, and leverage home equity to buy TVs). After the house was foreclosed on, Corporate job lost, and all the debt-fueled toys were taken away - the pervasive American consumption psyche has started a fundamental change as consumers (consciously & subconscious) are shifting their consumption dollars to products & brands that deliver greater value beyond product & with whom consumers identify with as individuals and feel like their business matters to the companies they are supporting.This shift in consumer psychology was enabled by the explosion of the “social web” that collapsed the barriers for consumers to discover about brands & products and learn about who they are as people. The brilliance of the social web is that it enables engagement to be driven by rich, personal, and substantive relationships that support companies & people that you actually like and identify with. From Quora to Gilt Groupe, every transformative startup over the last 5 years has demonstrated that great products require the creation of a meaningful emotional bond between the user/customer & the product/brand that fosters a sense of personality, ethical, & philosophical congruency. This is what we now call ‘emotional engagement’ - where the combination of product, brand, and social experience cultivate a friendship between the people at the brand and the customer.The phenomenal successes of Etsy, Kickstarter, and invested.in demonstrates that consumers will support independents that are less price-sensitive when they know it’s helping the little guy. The most interesting thing is that customers are implicitly beginning to monetize the brand experience & deep emotional engagement of these types of products.
How does BPM / business process management add a competitive advantage to an organization?
BPM or Business Process Management is the study and analysis of a business or organizations processes (how they do things) such as sales, customer services, HR and manufacturing. And quite often there are small processes inside larger processes all of which can be analyzed and studied to untap their potential for increased efficiency, customer satisfaction or service (and product) quality.Take a look at the definition of BPM here.The improvement of such functions leads to more efficient processes, that can be constantly analyzed to eake out as much potential as possible, leading to lower costs, an elimination of bottlenecks and other wastages, unlocking this potential inevitably results in increased profits for the company and of course, happy shareholders!With the global economic environment being as cut-throat and competitive as it is today, the smallest differences can become the biggest difference between rival companies, that compete based on product/service, cost and volume, while possibly ignoring customer satisfaction, quality and inefficiencies. With this in mind, BPM and the study of processes can be the competitive advantage your business needs to out perform the competition!For an interesting read on the benefits of BPM check this out!Check out how Heflo can help you achieve this with their free BPM modeling tool, register and start modeling processes now and get a head start on the competition!
What is the difference between Data Analytics, Data Analysis, Data Mining, Data Science, Machine Learning, and Big Data?
I had been wanting to take a stab at this one since a few days, but it always looked like an enormous task, because this question has used too many words. In addition, this is a question on which a lot of people have their eyes, and a lot of others have already written elaborate answers.Let me first re-order all the important words:Big dataData miningData analysisAnalyticsMachine learningData scienceImagine that you want to become a data scientist, and work in a big organization like Amazon, Intel, Google, FB, Apple and so on.How would that look like?You would have to deal with big data, you would have to write computer programs in SQL, Python, R, C++, Java, Scala, Ruby…and so on, to only maintain big-data databases. You would be called a database manager.As an engineer working on process control, or someone wanting to streamline operations of the company, you would perform Data Mining, and Data Analysis; You may use simple software to do this where you would only run a lot of codes written by others, or you may be writing your elaborate codes in SQL, Python, R and you would be doing data mining, data cleaning, data analysis, modeling, predictive modeling and so on.All this will be called Analytics. Several software exist to do this. One popular one is Tableau. Some others are JMP and SAS. Lot of people do everything online where a SAP based business intelligence setup can be used. Here, simple reporting can be done easily.Further, you would then be able to use machine learning to derive conclusions, and come up with predictions, wherever analytical answers are not possible. Think of analytical answers as [If/then] type of computer programs, where all the input conditions are already known, and only a few parameters change.Machine learning uses statistical analysis to partition data. An example would be this: Read the comments written by various people on Yelp, and predict from the comments whether the person would have marked a restaurant 4 star or 5 star.If that is not enough, you would be able to use deep learning as well. Deep learning is used to process data such as musical files, images, even text data such as natural languages, where data are enormous, but their type is very diverse.You would use everything to your advantage ~ analytical solutions, partitioning data, hacking mindset, automation by programming, reporting, deriving conclusions, making decisions, taking actions, and telling stories about your data.Last but not the least, a part of this will be happening on cruise control, where you may not be there physically, but the programs you may have created would do most of the things themselves. Probably if you take it to the level of AI, one day it may get smarter than you, needless to say it would already be faster than you. One day it can go to the level that it can surprise you with the solutions that you may not even have imagined.Now you are a data scientist, and what you would do is called Data-science.Whatever you would do may or may not be seen by people outside your company such as people asking Alexa various questions if you work for Amazon, or people asking questions to ok Google if you work for Google. Or they may not be getting to see anything you do. Your functions would be helping the companies engineer things better.To do all this, you may need lots of expertise in handling data and knowledge of a few programming languages.One popular data science Venn Diagram I have seen on internet is here: Note that a data scientist is at the intersection of a lot of things. Communication, statistics, programming, and business.Read also:Rohit Malshe's answer to How do I learn machine learning?Rohit Malshe's answer to How should I start learning Python?Rohit Malshe's answer to What is deep learning? Why is this a growing trend in machine learning? Why not use SVMs?Rohit Malshe's answer to Are ‘curated paths to a Data Science career’ on Coursera worth the money and time?In all the seriousness, if you want a elaborate documentation on all this, I would suggest, go ahead and read this McKinsey report to get a full understanding. I only extracted a few sections out of it conveniently because I only wanted to add on the top of someone else’s knowledge, and put together these concepts like a story so as to inspire the people to think about this subject and begin their own journeys.Big data: The next frontier for innovation, competition, and productivityI will answer a few questions step by step, and wherever possible, I will give a few pictures, or plots to show you how things look like.McKinsey consultants! You are amazing, so if you read things written in this answer that were typed by you at some point in time, I give full credit to you.What do we mean by "big data"?“Big data” refers to datasets whose size is beyond the ability of typical database software tools to capture, store, manage, and analyze. This definition is intentionally subjective and incorporates a moving definition of how big a dataset needs to be in order to be considered big data—i.e., we need not define big data in terms of being larger than a certain number of terabytes (thousands of gigabytes). We assume that, as technology advances over time, the size of datasets that qualify as big data will also increase. Also note that the definition can vary by sector, depending on what kinds of software tools are commonly available and what sizes of datasets are common in a particular industry. With those caveats, big data in many sectors today will range from a few dozen terabytes to multiple petabytes (thousands of terabytes).What is a typical size of data I may have to deal with? Sometimes GBs, sometimes just a few MBs, sometimes up to as high as 1TB. Sometimes the complexity is nothing. The data may be representing the same thing. Sometimes the complexity can be very high. I might have a giant file full of a lot of data and logs which can be structured or unstructured.Think for example about Macy’s. There are thousands of stores, selling thousands of items per day to millions of customers. If Macy’s wants to derive a conclusion ~ should they rather diversify in shoes, or should they rather diversify in women’s purses? How would they make this decision?Well then, a natural question is: How do we measure the value of big data?Measuring data Measuring volumes of data provokes a number of methodological questions. First, how can we distinguish data from information and from insight? Common definitions describe data as being raw indicators, information as the meaningful interpretation of those signals, and insight as an actionable piece of knowledge.For example - In this chart, someone has plotted cost per student for various regions. It makes a few of them stand out.Let us now talk about analysis: This is big part of being a data scientist.TECHNIQUES FOR ANALYZING BIG DATAThere are many techniques that draw on disciplines such as statistics and computer science (particularly machine learning) that can be used to analyze datasets. This list is by no means exhaustive. Indeed, researchers continue to develop new techniques and improve on existing ones, particularly in response to the need to analyze new combinations of data.Also, note that not all of these techniques strictly require the use of big data—some of them can be applied effectively to smaller datasets (e.g., A/B testing, regression analysis). However, all of the techniques listed here can be applied to big data and, in general, larger and more diverse datasets can be used to generate more numerous and insightful results than smaller, less diverse ones.A/B testing. A technique in which a control group is compared with a variety of test groups in order to determine what treatments (i.e., changes) will improve a given objective variable, e.g., marketing response rate. This technique is also known as split testing or bucket testing. An example application is determining what copy text, layouts, images, or colors will improve conversion rates on an e-commerce Web site. Big data enables huge numbers of tests to be executed and analyzed, ensuring that groups are of sufficient size to detect meaningful (i.e., statistically significant) differences between the control 28 and treatment groups (see statistics). When more than one variable is simultaneously manipulated in the treatment, the multivariate generalization of this technique, which applies statistical modeling, is often called “A/B/N” testing. What would an example look like?Imagine that Coke signs up with Facebook to work on marketing and sales. Facebook would put advertisements according to the customers. It can create versions of advertisements. Not all versions will suit to every geography. Some will suit to USA, some will suit to India. Some can suit to Indians living in USA. What Facebook can do is to choose a subset of people from a massive pool, and pass advertisements to them in their feed according to whether those people love food or not. For each advertisement, Facebook will collect the responses and accordingly determine which advertisement does better, and on a larger pool of people it will use a better one. Does data science let someone determine better what the answer should be? Absolutely!Association rule learning. A set of techniques for discovering interesting relationships, i.e., “association rules,” among variables in large databases. These techniques consist of a variety of algorithms to generate and test possible rules. One application is market basket analysis, in which a retailer can determine which products are frequently bought together and use this information for marketing (a commonly cited example is the discovery that many supermarket shoppers who buy diapers also tend to buy beer).Classification. A set of techniques to identify the categories in which new data points belong, based on a training set containing data points that have already been categorized. One application is the prediction of segment-specific customer behavior (e.g., buying decisions, churn rate, consumption rate) where there is a clear hypothesis or objective outcome. These techniques are often described as supervised learning because of the existence of a training set; they stand in contrast to cluster analysis, a type of unsupervised learning.Cluster analysis. A statistical method for classifying objects that splits a diverse group into smaller groups of similar objects, whose characteristics of similarity are not known in advance. An example of cluster analysis is segmenting consumers into self-similar groups for targeted marketing. This is a type of unsupervised learning because training data are not used. This technique is in contrast to classification, a type of supervised learning.Crowdsourcing. A technique for collecting data submitted by a large group of people or community (i.e., the “crowd”) through an open call, usually through networked media such as the Web.28 This is a type of mass collaboration and an instance of using Web 2.0.29 Data fusion and data integration.A set of techniques that integrate and analyze data from multiple sources in order to develop insights in ways that are more efficient and potentially more accurate than if they were developed by analyzing a single source of data.Data mining. A set of techniques to extract patterns from large datasets by combining methods from statistics and machine learning with database management. These techniques include association rule learning, cluster analysis, classification, and regression. Applications include mining customer data to determine segments most likely to respond to an offer, mining human resources data to identify characteristics of most successful employees, or market basket analysis to model the purchase behavior of customers.Ensemble learning. Using multiple predictive models (each developed using statistics and/or machine learning) to obtain better predictive performance than could be obtained from any of the constituent models. This is a type of supervised learning.Genetic algorithms. A technique used for optimization that is inspired by the process of natural evolution or “survival of the fittest.” In this technique, potential solutions are encoded as “chromosomes” that can combine and mutate. These individual chromosomes are selected for survival within a modeled “environment” that determines the fitness or performance of each individual in the population. Often described as a type of “evolutionary algorithm,” these algorithms are well-suited for solving nonlinear problems. Examples of applications include improving job scheduling in manufacturing and optimizing the performance of an investment portfolio.Machine learning. A subspecialty of computer science (within a field historically called “artificial intelligence”) concerned with the design and development of algorithms that allow computers to evolve behaviors based on empirical data. A major focus of machine learning research is to automatically learn to recognize complex patterns and make intelligent decisions based on data. Natural language processing is an example of machine learning.Natural language processing (NLP). A set of techniques from a sub-specialty of computer science (within a field historically called “artificial intelligence”) and linguistics that uses computer algorithms to analyze human (natural) language. Many NLP techniques are types of machine learning. One application of NLP is using sentiment analysis on social media to determine how prospective customers are reacting to a branding campaign. Data from social media, analyzed by natural language processing, can be combined with real-time sales data, in order to determine what effect a marketing campaign is having on customer sentiment and purchasing behavior.Neural networks. Computational models, inspired by the structure and workings of biological neural networks (i.e., the cells and connections within a brain), that find patterns in data. Neural networks are well-suited for finding nonlinear patterns. They can be used for pattern recognition and optimization. Some neural network applications involve supervised learning and others involve unsupervised learning. Examples of applications include identifying high-value customers that are at risk of leaving a particular company and identifying fraudulent insurance claims.Network analysis. A set of techniques used to characterize relationships among discrete nodes in a graph or a network. In social network analysis, connections between individuals in a community or organization are analyzed, e.g., how information travels, or who has the most influence over whom. Examples of applications include identifying key opinion leaders to target for marketing, and identifying bottlenecks in enterprise information flows.Optimization. A portfolio of numerical techniques used to redesign complex systems and processes to improve their performance according to one or more objective measures (e.g., cost, speed, or reliability). Examples of applications include improving operational processes such as scheduling, routing, and floor layout, and making strategic decisions such as product range strategy, linked investment analysis, and R&D portfolio strategy. Genetic algorithms are an example of an optimization technique. Same way, mixed integer programming is another way.Pattern recognition. A set of machine learning techniques that assign some sort of output value (or label) to a given input value (or instance) according to a specific algorithm. Classification techniques are an example.Predictive modeling. A set of techniques in which a mathematical model is created or chosen to best predict the probability of an outcome. An example of an application in customer relationship management is the use of predictive models to estimate the likelihood that a customer will “churn” (i.e., change providers) or the likelihood that a customer can be cross-sold another product. Regression is one example of the many predictive modeling techniques.Regression. A set of statistical techniques to determine how the value of the dependent variable changes when one or more independent variables is modified. Often used for forecasting or prediction. Examples of applications include forecasting sales volumes based on various market and economic variables or determining what measurable manufacturing parameters most influence customer satisfaction. Used for data mining.Sentiment analysis. Application of natural language processing and other analytic techniques to identify and extract subjective information from source text material. Key aspects of these analyses include identifying the feature, aspect, or product about which a sentiment is being expressed, and determining the type, “polarity” (i.e., positive, negative, or neutral) and the degree and strength of the sentiment. Examples of applications include companies applying sentiment analysis to analyze social media (e.g., blogs, microblogs, and social networks) to determine how different customer segments and stakeholders are reacting to their products and actions.Signal processing. A set of techniques from electrical engineering and applied mathematics originally developed to analyze discrete and continuous signals, i.e., representations of analog physical quantities (even if represented digitally) such as radio signals, sounds, and images. This category includes techniques from signal detection theory, which quantifies the ability to discern between signal and noise. Sample applications include modeling for time series analysis or implementing data fusion to determine a more precise reading by combining data from a set of less precise data sources (i.e., extracting the signal from the noise). Signal processing techniques can be used to implement some types of data fusion. One example of an application is sensor data from the Internet of Things being combined to develop an integrated perspective on the performance of a complex distributed system such as an oil refinery.Spatial analysis. A set of techniques, some applied from statistics, which analyze the topological, geometric, or geographic properties encoded in a data set. Often the data for spatial analysis come from geographic information systems (GIS) that capture data including location information, e.g., addresses or latitude/longitude coordinates. Examples of applications include the incorporation of spatial data into spatial regressions (e.g., how is consumer willingness to purchase a product correlated with location?) or simulations (e.g., how would a manufacturing supply chain network perform with sites in different locations?).Statistics. The science of the collection, organization, and interpretation of data, including the design of surveys and experiments. Statistical techniques are often used to make judgments about what relationships between variables could have occurred by chance (the “null hypothesis”), and what relationships between variables likely result from some kind of underlying causal relationship (i.e., that are “statistically significant”). Statistical techniques are also used to reduce the likelihood of Type I errors (“false positives”) and Type II errors (“false negatives”). An example of an application is A/B testing to determine what types of marketing material will most increase revenue.Supervised learning. The set of machine learning techniques that infer a function or relationship from a set of training data. Examples include classification and support vector machines.30 This is different from unsupervised learning.Simulation. Modeling the behavior of complex systems, often used for forecasting, predicting and scenario planning. Monte Carlo simulations, for example, are a class of algorithms that rely on repeated random sampling, i.e., running thousands of simulations, each based on different assumptions. The result is a histogram that gives a probability distribution of outcomes. One application is assessing the likelihood of meeting financial targets given uncertainties about the success of various initiatives.Time series analysis. Set of techniques from both statistics and signal processing for analyzing sequences of data points, representing values at successive times, to extract meaningful characteristics from the data. Examples of time series analysis include the hourly value of a stock market index or the number of patients diagnosed with a given condition every day.Time series forecasting. Time series forecasting is the use of a model to predict future values of a time series based on known past values of the same or other series. Some of these techniques, e.g., structural modeling, decompose a series into trend, seasonal, and residual components, which can be useful for identifying cyclical patterns in the data. Examples of applications include forecasting sales figures, or predicting the number of people who will be diagnosed with an infectious disease.Unsupervised learning. A set of machine learning techniques that finds hidden structure in unlabeled data. Cluster analysis is an example of unsupervised learning (in contrast to supervised learning).Visualization. Techniques used for creating images, diagrams, or animations to communicate, understand, and improve the results of big data analyses. This expands into creating dashboards, on web or desktop platforms.Hope this somewhat elaborate write up gives you some inspiration to hold on to. Stay blessed and stay inspired!
- Home >
- Catalog >
- Life >
- Funeral Template >
- Obituary Examples >
- short obituary examples >
- A Model For Efficiency Analysis Of The Customer Satisfaction Process