The Guide of finalizing Impact Consent Form - Mayo Clinic Health System Online
If you are looking about Customize and create a Impact Consent Form - Mayo Clinic Health System, here are the step-by-step guide you need to follow:
- Hit the "Get Form" Button on this page.
- Wait in a petient way for the upload of your Impact Consent Form - Mayo Clinic Health System.
- You can erase, text, sign or highlight through your choice.
- Click "Download" to preserver the forms.
A Revolutionary Tool to Edit and Create Impact Consent Form - Mayo Clinic Health System
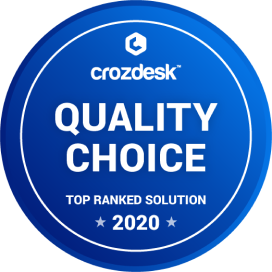
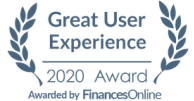
Edit or Convert Your Impact Consent Form - Mayo Clinic Health System in Minutes
Get FormHow to Easily Edit Impact Consent Form - Mayo Clinic Health System Online
CocoDoc has made it easier for people to Fill their important documents through the online platform. They can easily Modify through their choices. To know the process of editing PDF document or application across the online platform, you need to follow these simple ways:
- Open CocoDoc's website on their device's browser.
- Hit "Edit PDF Online" button and Select the PDF file from the device without even logging in through an account.
- Edit the PDF online by using this toolbar.
- Once done, they can save the document from the platform.
Once the document is edited using online browser, the user can export the form as you need. CocoDoc ensures to provide you with the best environment for consummating the PDF documents.
How to Edit and Download Impact Consent Form - Mayo Clinic Health System on Windows
Windows users are very common throughout the world. They have met lots of applications that have offered them services in modifying PDF documents. However, they have always missed an important feature within these applications. CocoDoc wants to provide Windows users the ultimate experience of editing their documents across their online interface.
The steps of modifying a PDF document with CocoDoc is simple. You need to follow these steps.
- Pick and Install CocoDoc from your Windows Store.
- Open the software to Select the PDF file from your Windows device and move toward editing the document.
- Fill the PDF file with the appropriate toolkit presented at CocoDoc.
- Over completion, Hit "Download" to conserve the changes.
A Guide of Editing Impact Consent Form - Mayo Clinic Health System on Mac
CocoDoc has brought an impressive solution for people who own a Mac. It has allowed them to have their documents edited quickly. Mac users can fill PDF form with the help of the online platform provided by CocoDoc.
To understand the process of editing a form with CocoDoc, you should look across the steps presented as follows:
- Install CocoDoc on you Mac in the beginning.
- Once the tool is opened, the user can upload their PDF file from the Mac in minutes.
- Drag and Drop the file, or choose file by mouse-clicking "Choose File" button and start editing.
- save the file on your device.
Mac users can export their resulting files in various ways. They can download it across devices, add it to cloud storage and even share it with others via email. They are provided with the opportunity of editting file through various ways without downloading any tool within their device.
A Guide of Editing Impact Consent Form - Mayo Clinic Health System on G Suite
Google Workplace is a powerful platform that has connected officials of a single workplace in a unique manner. When allowing users to share file across the platform, they are interconnected in covering all major tasks that can be carried out within a physical workplace.
follow the steps to eidt Impact Consent Form - Mayo Clinic Health System on G Suite
- move toward Google Workspace Marketplace and Install CocoDoc add-on.
- Attach the file and Click on "Open with" in Google Drive.
- Moving forward to edit the document with the CocoDoc present in the PDF editing window.
- When the file is edited ultimately, download it through the platform.
PDF Editor FAQ
How can deep neural networks be applied to healthcare?
Throughout the course of comprehensive healthcare, many patients develop problems with their minds and bodies that can lead to severe discomfort, costly treatment, disabilities, and more. Predicting those escalations in advance offers healthcare providers the opportunity to apply preventative measure that might improve patient safety, and quality of care, while lowering medical costs. In simple terms, prediction using networks of big data used to evaluate specific people, and specific risk factors in certain illnesses could save lives, and avoid medical complications.Today, many prognostics methods turn to Artificial Neural Networks when attempting to find new insights into the future of patient healthcare. ANNs (Artificial Neural Networks) are just one of the many models being introduced into the field of healthcare by innovations like AI and big data. Their purpose is to transform huge amounts of raw data into useful decisions for treatment and care.What is a Neural Network?Understanding Neural Networks can be very difficult. After all, to many people, these examples of Artificial Intelligence in the medical industry are a futuristic concept.According to Wikipedia (the source of all truth) :“Neural Networks are a computational approach which is based on a large collection of neural units loosely modeling the way the brain solves problems with large clusters of biological neurons connected by axons. Each neural unit is connected with many others…These systems are self-learning and trained rather than explicitly programmed…”By Glosser.ca – Own work, Derivative of File:Artificial neural network.svg (https://commons.wikimedia.org/wiki/File:Artificial_neural_network.svg), CC BY-SA 3.0, LinkOne way to think of it is this: Imagine that a doctor wants to make a prediction regarding a patient’s health – for instance, whether she or he is at risk of suffering from a certain disease. How would a doctor be able to ascertain that information? In most cases, it would involve using blood tests, taking tests of the patient’s vitals, and more to identify features that have proven to be good predictors of patient health. However, what if doctors only know a handful of risk-factors for a specific disease – or worse, they don’t know the risk factors at all? It would be impossible to make predictions.ANNs help to provide the predictions in healthcare that doctors and surgeons simply couldn’t address alone. They work in moments wherein we can collect data, but we don’t understand which pieces of that data are vitally important yet. These abstractions can therefore capture complex relationships that might not be initially obvious – leading to better prediction for public health.What are the Possibilities for Neural Networks in Healthcare?Though they may seem like a futuristic concept, ANNs have been used in healthcare for several decades. In fact, the book “Neural Networks in Healthcare” covers the various uses of this system prior to 2006. Before 2006, the main successes of ANNs were found in areas like speech processing and image processing. Today, as new technologies emerge, capable of changing the way that we approach neural networks in the first place – it’s worth noting that there may be numerous new options for changing the industry. Today, the possibilities for Neural Networks in Healthcare include:Diagnostic systems – ANNs can be used to detect heart and cancer problems, as well as various other diseases informed by big data.Biochemical analysis – ANNs are used to analyze urine and blood samples, as well as tracking glucose levels in diabetics, determining ion levels in fluids, and detecting various pathological conditions.Image analysis – ANNs are frequently used to analyze medical images from various areas of healthcare, including tumor detection, x-ray classifications, and MRIs.Drug development – Finally, ANNs are used in the development of drugs for various conditions – working by using large amounts of data to come to conclusions about treatment options.Current Examples of Neural NetworksNeural networks can be seen in most places where AI has made steps within the healthcare industry. For instance, in the world of drug discovery, Data Collective and Khosla Ventures are currently backing the company “Atomwise“, which uses the power of machine learning and neural networks to help medical professionals discover safer and more effective medicines fast. The company recently published its first findings of Ebola treatment drugs last year, and the tools that Atomwise uses can tell the difference between toxic drug candidates and safer options.Similarly, options are being found that could insert neural networks into the realm of diagnostic. For instance, in 2014, Butterfly Networks, which are transforming the diagnostic realm with deep learning, devices, and the cloud, raised $100M for their cause. This organization currently works at the heart of the medicine and engineering sectors by bringing together world-class skills in everything from electrical engineering, to mechanical engineering, and medicine. At the same time, iCarbonX are developing artificial intelligence platforms to facilitate research relating to the treatment of various diseases and preventative care. The company believe that soon they will be able to help enable the future of truly personalized medicine.The Future of Healthcare…Perhaps the most significant problem with ANNs is that the learned features involved when it comes to assessing huge amounts of data can sometimes be difficult to interpret. This is potentially why ANNs are more commonly used during situations wherein we have a lot of data to ensure that the observed data doesn’t contain too many “flukes”. Think of it this way – if you toss a coin three times and receive “tails” every time, this doesn’t mean that a coin only has a “tails” side. It just means that you need further evaluation and more testing to get a proper reading of probability.ANNs are going to need some tweaking if they’re going to become the change that the healthcare industry needs. However, alongside new AI developments, it seems that neural networks could have a very important part to play in the future of healthcare.Healthcare organizations of all sizes, types, and specialties are becoming increasingly interested in how artificial intelligence can support better patient care while reducing costs and improving efficiencies.Over a relatively short period of time, the availability and sophistication of AI has exploded, leaving providers, payers, and other stakeholders with a dizzying array of tools, technologies, and strategies to choose from.Just learning the lingo has been a top challenge for many organizations.There are subtle but significant differences between key terms such as AI, machine learning, deep learning, and semantic computing.Understanding exactly how data is ingested, analyzed, and returned to the end user can have a big impact on expectations for accuracy and reliability, not to mention influencing any investments necessary to whip an organization’s data assets into shape.In order to efficiently and effectively choose between vendor products or hire the right data science staff to develop algorithms in-house, healthcare organizations should feel confident that they have a firm grasp on the different flavors of artificial intelligence and how they can apply to specific use cases.Deep learning is a good place to start. This branch of artificial intelligence has very quickly become transformative for healthcare, offering the ability to analyze data with a speed and precision never seen before.But what exactly is deep learning, how does it differ from other machine learning strategies, and how can healthcare organizations leverage deep learning techniques to solve some of the most pressing problems in patient care?DEEP LEARNING IN A NUTSHELLDeep learning, also known as hierarchical learning or deep structured learning, is a type of machine learning that uses a layered algorithmic architecture to analyze data.In deep learning models, data is filtered through a cascade of multiple layers, with each successive layer using the output from the previous one to inform its results. Deep learning models can become more and more accurate as they process more data, essentially learning from previous results to refine their ability to make correlations and connections.Deep learning is loosely based on the way biological neurons connect with one another to process information in the brains of animals. Similar to the way electrical signals travel across the cells of living creates, each subsequent layer of nodes is activated when it receives stimuli from its neighboring neurons.In artificial neural networks (ANNs), the basis for deep learning models, each layer may be assigned a specific portion of a transformation task, and data might traverse the layers multiple times to refine and optimize the ultimate output.These “hidden” layers serve to perform the mathematical translation tasks that turn raw input into meaningful output.An illustration of a deep learning neural networkSource: University of Cincinnati“Deep learning methods are representation-learning methods with multiple levels of representation, obtained by composing simple but non-linear modules that each transform the representation at one level (starting with the raw input) into a representation at a higher, slightly more abstract level,” explains a 2015 article published in Nature, authored by engineers from Facebook, Google, the University of Toronto, and Université de Montréal.“With the composition of enough such transformations, very complex functions can be learned. Higher layers of representation amplify aspects of the input that are important for discrimination and suppress irrelevant variations.”This multi-layered strategy allows deep learning models to complete classification tasks such as identifying subtle abnormalities in medical images, clustering patients with similar characteristics into risk-based cohorts, or highlight relationships between symptoms and outcomes within vast quantities of unstructured data.Unlike other types of machine learning, deep learning has the added benefit of being able to decisions with significantly less involvement from human trainers.While basic machine learning requires a programmer to identify whether a conclusion is correct or not, deep learning can gauge the accuracy of its answers on its own due to the nature of its multi-layered structure.“With the composition of enough such transformations, very complex functions can be learned.”Deep learning also requires less preprocessing of data. The network itself takes care of many of the filtering and normalization tasks that must be completed by human programmers when using other machine learning techniques.“Conventional machine-learning techniques are limited in their ability to process natural data in their raw form,” said the article from Nature.“For decades, constructing a pattern-recognition or machine-learning system required careful engineering and considerable domain expertise to design a feature extractor that transformed the raw data (such as the pixel values of an image) into a suitable internal representation or feature vector from which the learning subsystem, often a classifier, could detect or classify patterns in the input.”Deep learning networks, however, “automatically discover the representations needed for detection or classification,” reducing the need for supervision and speeding up the process of extracting actionable insights from datasets that have not been as extensively curated.Naturally, the mathematics involved in developing deep learning models are extraordinarily intricate, and there are many different variations of networks that leverage different sub-strategies within the field.The science of deep learning is evolving very quickly to power some of the most advanced computing capabilities in the world, spanning every industry and adding significant value to user experiences and competitive decision-making.WHAT ARE THE USE CASES FOR DEEP LEARNING IN HEALTHCARE?Many of the industry’s deep learning headlines are currently related to small-scale pilots or research projects in their pre-commercialized phases.However, deep learning is steadily finding its way into innovative tools that have high-value applications in the real-world clinical environment.Some of the most promising use cases include innovative patient-facing applications as well as a few surprisingly established strategies for improving the health IT user experience.Imaging analytics and diagnosticsOne type of deep learning, known as convolutional neural networks (CNNs), is particularly well-suited to analyzing images, such as MRI results or x-rays.CNNs are designed with the assumption that they will be processing images, according tocomputer science experts at Stanford University, allowing the networks to operate more efficiently and handle larger images.As a result, some CNNs are approaching – or even surpassing – the accuracy of human diagnosticians when identifying important features in diagnostic imaging studies.In June of 2018, a study in the Annals of Oncology showed that a convolutional neural network trained to analyze dermatology images identified melanoma with ten percent more specificity than human clinicians.Even when human clinicians were equipped with background information on patients, such as age, sex, and the body site of the suspect feature, the CNN outperformed the dermatologists by nearly 7 percent.“Our data clearly show that a CNN algorithm may be a suitable tool to aid physicians in melanoma detection irrespective of their individual level of experience and training,” said the team of researchers from a number of German academic institutions.In addition to being highly accurate, deep learning tools are fast.Researchers at the Mount Sinai Icahn School of Medicine have developed a deep neural network capable of diagnosing crucial neurological conditions, such as stroke and brain hemorrhage, 150 times faster than human radiologists.“Our data clearly show that a CNN algorithm may be a suitable tool to aid physicians in melanoma detection irrespective of their individual level of experience and training.”The tool took just 1.2 seconds to process the image, analyze its contents, and alert providers of a problematic clinical finding.“The expression ‘time is brain’ signifies that rapid response is critical in the treatment of acute neurological illnesses, so any tools that decrease time to diagnosis may lead to improved patient outcomes,” said Joshua Bederson, MD, Professor and System Chair for the Department of Neurosurgery at Mount Sinai Health System and Clinical Director of the Neurosurgery Simulation Core.Deep learning is so adept at image work that some AI scientists are using neural networks to create medical images, not just read them.A team from NVIDIA, the Mayo Clinic, and the MGH & BWH Center for Clinical Data Science has developed a method of using generative adversarial networks (GANs), another type of deep learning, which can create stunningly realistic medical images from scratch.The images use patterns learned from real scans to create synthetic versions of CT or MRI images. The data can be randomly generated and endlessly diverse, allowing researchers to access large volumes of necessary data without any concerns around patient privacy or consent.These simulated images are so accurate that they can help train future deep learning models to diagnose clinical findings.“Medical imaging data sets are often imbalanced as pathologic findings are generally rare, which introduces significant challenges when training deep learning models,” said the team. “We propose a method to generate synthetic abnormal MRI images with brain tumors by training a generative adversarial network.”“This offers an automatable, low-cost source of diverse data that can be used to supplement the training set. For example, we can alter a tumor’s size, change its location, or place a tumor in an otherwise healthy brain, to systematically have the image and the corresponding annotation.”Such a strategy could significantly reduce of AI’s biggest sticking points: a lack of reliable, sharable, high-volume datasets to use for training and validating machine learning models.Natural language processingDeep learning and neural networks already underpin many of the natural language processing tools that have become popular in the healthcare industry for dictating documentation and translating speech-to-text.Because neural networks are designed for classification, they can identify individual linguistic or grammatical elements by “grouping” similar words together and mapping them in relation to one another.This helps the network understand complex semantic meaning. But the task is complicated by the nuances of common speech and communication. For example, words that always appear next to each other in an idiomatic phrase, may end up meaning something very different than if those same words appeared in another context (think “kick the bucket” or “barking up the wrong tree”).While acceptably accurate speech-to-text has become a relatively common competency for dictation tools, generating reliable and actionable insights from free-text medical data is significantly more challenging.Unlike images, which consist of defined rows and columns of pixels, the free text clinical notes in electronic health records (EHRs) are notoriously messy, incomplete, inconsistent, full of cryptic abbreviations, and loaded with jargon.Currently, most deep learning tools still struggle with the task of identifying important clinical elements, establishing meaningful relationships between them, and translating those relationships into some sort of actionable information for an end user.A recent literature review from JAMIA found that while deep learning surpasses other machine learning methods for processing unstructured text, several significant challenges, including the quality of EHR data, are holding these tools back from true success.“Researchers have confirmed that finding patterns among multimodal data can increase the accuracy of diagnosis, prediction, and overall performance of the learning system. However, multimodal learning is challenging due to the heterogeneity of the data,” the authors observed.Accessing enough high-quality data to train models accurately is also problematic, the article continued. Data that is biased or skewed towards particular age groups, ethnicities, or other characteristics could create models that are not equipped to accurately assess a broad variety of real-life patients.Still, deep learning represents the most promising pathway forward into trustworthy free-text analytics, and a handful of pioneering developers are finding ways to break through the existing barriers.A team from Google, UC San Francisco, Stanford Medicine, and the University of Chicago Medicine, for example, developed a deep learning and natural language processing algorithm that analyzed more than 46 billion data points from more than 216,000 EHRs across two hospitals.The tool was able to improve on the accuracy of traditional approaches for identifying unexpected hospital readmissions, predicting length of stay, and forecasting inpatient mortality.“This predictive performance was achieved without hand-selection of variables deemed important by an expert, similar to other applications of deep learning to EHR data,” the researchers said.“Instead, our model had access to tens of thousands of predictors for each patient, including free-text notes, and identified which data were important for a particular prediction.”While the project is only a proof-of-concept study, Google researchers said, the findings could have dramatic implications for hospitals and health systems looking to reduce negative outcomes and become more proactive about delivering critical care.Drug discovery and precision medicinePrecision medicine and drug discovery are also on the agenda for deep learning developers. Both tasks require processing truly enormous volumes of genomic, clinical, and population-level data with the goal of identifying hitherto unknown associations between genes, pharmaceuticals, and physical environments.Deep learning is an ideal strategy for researchers and pharmaceutical stakeholders looking to highlight new patterns in these relatively unexplored data sets – especially because many precision medicine researchers don’t yet know exactly what they should be looking for.“Our model had access to tens of thousands of predictors for each patient, including free-text notes, and identified which data were important for a particular prediction.”The world of genetic medicine is so new that unexpected discoveries are commonplace, creating an exciting proving ground for innovative approaches to targeted care.The National Cancer Institute and the Department of Energy are embracing this spirit of exploration through a number of joint projects focused on leveraging machine learning for cancer discoveries.The combination of predictive analytics and molecular modeling will hopefully uncover new insights into how and why certain cancers form in certain patients.Deep learning technologies will accelerate the process of analyzing data, the two agencies said, shrinking the processing time for key components from weeks or months to just a few hours.The private sector is similarly committed to illustrating how powerful deep learning can be for precision medicine.A partnership by GE Healthcare and Roche Diagnostics, announced in January of 2018, will focus on using deep learning and other machine learning strategies to synthesize disparate data sets critical to developing precision medicine insights.The two companies will work to combine in-vivo and in-vitro data, EHR data, clinical guidelines, and real-time monitoring data to support clinical decision-making and the creation of more effective, less invasive therapeutic pathways.“By leveraging this combined data set using machine learning and deep learning, it may be possible in the future to reduce the number of unnecessary biopsies that are performed due to suspicious findings in the mammograms and possibly also reduce mastectomies that are performed to combat ductal carcinoma in situ, a condition that may evolve into invasive breast cancer in some cases,” said Nadeem Ishaque, Chief Innovation Officer, GE Healthcare Imaging.A separate study, conducted by researchers from the University of Massachusetts and published in JMIR Medical Informatics, found that deep learning could also identify adverse drug events (ADEs) with much greater accuracy than traditional models.“By leveraging this combined data set using machine learning and deep learning, it may be possible in the future to reduce the number of unnecessary biopsies.”The tool combines deep learning with natural language processing to comb through unstructured EHR data, highlighting worrisome associations between the type, frequency, and dosage of medications. The results could be used for monitoring the safety of novel therapies or understanding how new pharmaceuticals are being prescribed in the real-world clinical environment.Clinical decision support and predictive analyticsIn a similar vein, the industry has high hopes for the role of deep learning in clinical decision support and predictive analytics for a wide variety of conditions.Deep learning may soon be a handy diagnostic companion in the inpatient setting, where it can alert providers to changes in high-risk conditions such as sepsis and respiratory failure.Researchers from the MIT Computer Science and Artificial Intelligence Laboratory (CSAIL) have created a project called ICU Intervene, which leverages deep learning to alert clinicians to patient downturns in the critical care unit.“Much of the previous work in clinical decision-making has focused on outcomes such as mortality (likelihood of death), while this work predicts actionable treatments,” said PhD student and lead author Harini Suresh. “In addition, the system is able to use a single model to predict many outcomes.”The tool offers human clinicians a detailed rationale for its recommendations, helping to foster trust and allowing providers to have confidence in their own decision-making when potentially overruling the algorithm.Google is also on the leading edge of clinical decision support, this time for eye diseases. The company’s UK-based subsidiary, DeepMind, is working to develop a commercialized deep learning CDS tool that can identify more than 50 different eye diseases – and provide treatment recommendations for each one.In a supporting study published in Nature, DeepMind and Moorfields Eye Hospital found that the tool is just as accurate as a human clinician, and has the potential to significantly expand access to care by reducing the time it takes for an exam and diagnosis.“Currently, eye care professionals use optical coherence tomography (OCT) scans to help diagnose eye conditions. These 3D images provide a detailed map of the back of the eye, but they are hard to read and need expert analysis to interpret,” explained DeepMind.“The time it takes to analyze these scans, combined with the sheer number of scans that healthcare professionals have to go through (over 1,000 a day at Moorfields alone), can lead to lengthy delays between scan and treatment – even when someone needs urgent care. If they develop a sudden problem, such as a bleed at the back of the eye, these delays could even cost patients their sight.”With deep learning, the triage process is nearly instantaneous, the company asserted, and patients do not have to sacrifice quality of care.“This is a hugely exciting milestone, and another indication of what is possible when clinicians and technologists work together,” DeepMind said.WHAT IS THE FUTURE OF DEEP LEARNING IN HEALTHCARE?As intriguing as these pilots and projects can be, they represent only the very beginning of deep learning’s role in healthcare analytics.Excitement and interest about deep learning are everywhere, capturing the imaginations of regulators and rule makers, private companies, care providers, and even patients.The Office of the National Coordinator (ONC) is one organization with particularly high hopes for deep learning, and it is already applauding some developers for achieving remarkable results.In a recent report on the state of AI in the healthcare setting, the agency noted that some deep learning algorithms have already produced “transformational” outcomes.“There have been significant demonstrations of the potential utility of artificial Intelligence approaches based on deep learning for use in medical diagnostics,” the report said.“Where good training sets represent the highest levels of medical expertise, applications of deep learning algorithms in clinical settings provide the potential of consistently delivering high quality results.”The report highlighted early successes in diabetic retinal screenings and the classification of skin cancer as two areas where deep learning may already be changing the status quo.On the clinical side, imaging analytics is likely to be the focal point for the near future, due to the fact that deep learning already has a head start on many high-value applications.“Applications of deep learning algorithms in clinical settings provide the potential of consistently delivering high quality results.”But purely clinical applications are only one small part of how deep learning is preparing to change the way the healthcare system functions.The strategy is integral to many consumer-facing technologies, such as chatbots, mHealth apps, and virtual personalities like Alexa, Siri, and Google Assistant.These tools have the potential to radically alter the way patients interact with the healthcare system, offering home-based chronic disease management programming, 24/7 access to basic triage, and new ways to complete administrative tasks.By 2019, up to 40 percent of businesses are planning to integrate one or more of these popular consumer technologies into their internal or external workflows.Customer support and communication are two early implementations. But with market-movers like Amazon rumored to start rolling out more consumer-facing health options to patients, it may only be a matter of time before chatting with Alexa becomes as common as shooting the breeze with a medical assistant.Voice recognition and other analytics based on deep learning also have the near-term potential to provide some relief to physicians and nurses struggling with their EHRs.Google appears particularly interested in capturing medical conversations in the clinic and using deep learning to reduce administrative burdens on providers.One recent research paper illustrated the potential to use deep learning and NLP to understand casual conversation in a noisy environment, giving rise to the possibility of using an ambient, intelligent scribe to shoulder the onus of documentation.“We wondered: could the voice recognition technologies already available in Google Assistant, Google Home, and Google Translate be used to document patient-doctor conversations and help doctors and scribes summarize notes more quickly?” a Google team posited.“While most of the current automatic speech recognition (ASR) solutions in medical domain focus on transcribing doctor dictations (i.e., single speaker speech consisting of predictable medical terminology), our research shows that it is possible to build an ASR model which can handle multiple speaker conversations covering everything from weather to complex medical diagnosis,” the blog post says.Google will work with physicians and data scientists at Stanford to refine the technology and understand how it can be best applied to the clinical setting.“We hope these technologies will not only help return joy to practice by facilitating doctors and scribes with their everyday workload, but also help the patients get more dedicated and thorough medical attention, ideally, leading to better care,” the team said.EHR vendors are also taking a hard look at how machine learning can streamline the user experience by eliminating wasteful interactions and presenting relevant data more intuitively within the workflow.“Taking out the trash” by using artificial intelligence to learn a user’s habits, anticipate their needs, and display the right data at the right time is a top priority for nearly all of the major health IT vendors – vendors who are finding themselves in the hot seat as unhappy customers plead for better solutions for their daily tasks.Both patients and providers are demanding much more consumer-centered tools and interactions from the healthcare industry, and artificial intelligence may now be mature enough to start delivering.“We finally have enough affordable computing power to get the answers we’re looking for,” said James Golden, PhD, Senior Managing Director for PwC’s Healthcare Advisory Group, to Healthcare IT Analytics News on Healthcare BI, Population Health and Data Management in February of 2018.“When I did my PhD in the 90s on back propagation neural networks, we were working with an input layer, an output layer, and two middle layers,” he recalled.“That’s not extremely complex. But it ran for four days on an Apple Lisa before producing results. I can do the same computation today in a picosecond on an iPhone. That is an enormous, staggering leap in our capabilities.”The intersection of more advanced methods, improved processing power, and growing interest in innovative methods of predicting, preventing, and cheapening healthcare will likely bode well for deep learning.With an extremely high number of promising use cases, strong investment from major players in the industry, and a growing amount of data to support cutting-edge analytics, deep learning will no doubt play a central role in the quest to deliver the highest possible quality care to consumers for decades to come.References :What Is Deep Learning and How Will It Change Healthcare?https://royaljay.com/healthcare/neural-networks-in-healthcare/
What organizations are coordinating support for SB277, the California bill to abolish personal belief exemptions to vaccine mandates?
Yes. There is a grass-roots organization providing letter templates, telling people which senators to call, and so on.:Vaccinate California SB 277 has a powerful roster of supporters, as of April 8 (I haven't updated the list since then):Vaccinate California (sponsor)American Academy of PediatricsAmerican Lung AssociationBiocomCalifornia Association of Nurse PractitionersCalifornia Chapter of the American College of Emergency PhysiciansCalifornia Children’s Hospital AssociationCalifornia Coverage and Health InitiativesCalifornia Immunization CoalitionCalifornia Medical AssociationCalifornia Optometric AssociationCalifornia School Nurses OrganizationCalifornia State Parent-Teacher AssociationChildren NowChildren’s Defense Fund CaliforniaChildren’s Specialty Care CoalitionCounty of Los AngelesCounty of Santa CruzHealth Officers Association of CaliforniaInsurance Commissioner Dave JonesKaiser PermanenteMarch of Dimes California ChapterProvidence Health and Services Southern CaliforniaSan Francisco Unified School DistrictSecular Coalition for CaliforniaSilicon Valley Leadership GroupSolano Beach School DistrictThe Children’s PartnershipHundreds of individualsBy contrast, here are the organizations (with my commentary) opposing SB277.[Health Committee Analysis] Association of American Physicians and Surgeons (AAPS)"The Association of American Physicians and Surgeons Inc. state that the need for informed consent is a firmly established principal of medical ethics and human rights and that the state has no right to force medical interventions on people without their consent"About AAPShttp://www.aapsonline.org/Rational Wiki:Association of American Physicians and SurgeonsThe Association of American Physicians and Surgeons is a small group of physicians who advocate for far-right conservative values in the practice of medicine. While purporting to have high regard for the Hippocratic Oath, "the sanctity of the patient-physician relationship, and the "practice of private medicine"[1], it appears to treat these concepts as terms of art. Despite also calling itself "non-partisan", its main focus appears to be opposing abortion, vaccination, universal health care coverage and Obamacare in particular, and birth control.---------------------[Health Committee Analysis] California Chiropractic Association"The California Chiropractic Association mentions that the Mayo Clinic warns against undermining the principle of informed consent in favor of universal vaccination and further states we ought not let a handful of measles cases at Disneyland turn into a full-scale assault on civil and human rights in America. "About CCAhttps://www.calchiro.org/Oppose SB 277 - Elimination of Vaccination Personal Belief Exemption"Stay with the framing of the issue provided. It’s about choice/informed consent. We are NOT anti-vaccine we are pro-informed consent and choice. Make the case that the data (provided in our talking points) demonstrates there are risks to taking the vaccine so there should be choice. "-------------------------[Health Committee Analysis] California Naturopathic Doctors Association (unless amended)"California Naturopathic Doctors Association (CNDA) states that it supports immunization for the prevention of disease and the public health objective of achieving high rates of immunity to infectious disease. CNDA states that as licensed primary care doctors who can diagnose medical conditions such as anaphylaxis and immunodeficiency, reasons outlined in the CDC’s list of contraindications to common pediatric vaccinations, naturopathic doctors must also be able to sign medical waivers for vaccination, when such medical conditions exist. CNDA opposes this bill unless it is amended to include NDs as providers who can sign medical waivers for vaccination."About CNDAhttp://www.calnd.org/[nothing on the website about SB277. Marty Block toured Bastyr University California in October 2014., and is the author of an expansion of practice bill for naturopaths.During the tours, students spoke about their education and their chosen profession. Says Adam Silberman, a third-year naturopathic medical student, “Being able to walk Senator Block and his staffer Roberto Alcantar through our clinic – explaining to them our philosophy of care and strong clinical training – brought me a strong sense of pride and excitement. How we are learning to work with patients will transform health care in California, and I think Senator Block and Congressman Hunter both saw that first-hand during their visits.”Lawmakers Tour Bastyr University CaliforniaMarty Block is the only sponsor for SB538, a bill that would greatly increase naturopaths' scope of practice.Bill StatusCuriously, there's nothing about the bill on his website.Senator Marty Block Legislative Update--------[Health Committee Analysis] California Nurses for Ethical StandardsAbout CNESBig issue is opposition to abortion and sex ed in schools.http://ethicalnurses.org/http://ethicalnurses.org/?p=2703SB277 seeks to remove the Personal Belief Exemption and mandate full vaccination of any pupil, from daycare through secondary school, public and private, in order to attend. Additionally, SB277 does not limit the ever-expanding vaccine schedule or the requirement of additional vaccines to attend school; in 2012 there were nearly 300 new vaccines in the pipeline. CNES strongly asserts principals of informed consent, personal belief, medical, religious, and philosophic exemptions, in accordance with the AMA and in the interest of maintaining ethical integrity in our public health laws. California Nurses For Ethical Standards oppose SB277.“If there is a risk then there should be a choice”[from hearing ] 1:30:03 California Nurses for Ethical Standards--------[Health Committee Analysis] California ProLife CouncilAbout California ProLife CouncilAction Alert!SB 277 – Mandated Childhood Vaccines Intrude on Parental Rights (Pan) – OPPOSEThis bill strives to eliminate the personal belief exemption option from the school immunization law forcing parents to vaccinate their children from all diseases deemed necessary by the Department of Public Health (DPH). Some immunizations use tissue from aborted babies.------------[Health Committee Analysis] California Right to Life Committee, Inc.http://www.calright2life.org/[nothing on webpage, most likely similar to California ProLife Council.]------------[Health Committee Analysis] Canary Party"The Canary Party maintains that the United States Justice System deems each and every vaccine on the market as “unavoidably unsafe”, which means that even when used as directed, someone will be harmed, or may even die from the vaccine. "About the Canary Party"The Canary Party is a tiny organization focused on promoting the idea that vaccines cause autism. They have branched out some with GMOs and “health freedom”, but their core seems to be the failed idea that vaccines cause autism. "More Canary Party financial documentsJennifer Larson, who is with the tiny “Canary Party”. Tax documents indicate that Ms. Larson is a large financial backer of the Canary Party. Searching for what donations Ms. Larson has made (using OpenSecrets.org, the Center for Responsive Politics) I found a total of $40,000 donations to Congressman Issa, his PAC and the Republican National Committee though Ms. Larson and her company Vibrant TechnologiesOne year and $40,000 later and another hearinghttp://www.canaryparty.org/[almost defunct organization; has been replaced by Barry Segal's Focus for Health, it appears. ]You Simply Have To Be Pro-Health - Focus for Health[from hearing ] 1:21:03 Canary Party------------[Health Committee Analysis] Capitol Resource Institute"The Capitol Resource Institute notes that following the passage of AB 2109 the personal belief exemption rate fell from 3.1 percent in 2013 to 2.5 percent in 2014 after only a partial implementation of the law, and that this bill is far reaching and unnecessary. "About the Capitol Resource Institutehttp://capitolresource.org/about-us/"CRI’s mission is to educate and strengthen families and we do that by working to influence public policy. It’s imperative that citizens join with us in staying up to speed on current legislation affecting family values! As your watchdog for family values here in Sacramento, CRI is committed to keeping you informed about important legislation. So, stay tuned!"Against LGBT issues being taught in school, against same-sex marriage, extremely Christian oriented.------------[Health Committee Analysis] Educate. Advocate."Educate. Advocate. writes that every medical intervention has both benefits and risks and that parents, not the State of California, have the right to decide which medical interventions their children receive"About Educate.Advocate."To assist and provide support to anyone with or connected to someone with special needs and/or disabilities at any age in the area surrounding mainly San Bernardino and Riverside county in California."Board members:Kristie Reneé Sepulveda-Burchit, Amy Carrillo, Marilyn Interian, Jerri Carpinteyro[many members believe their children are vaccine injured][from hearing ] 2:12:24 Educate Advocate------------[Health Committee Analysis] Families for Early Autism Treatment [FEAT]" Families for Early Autism Treatment contend that this bill is contrary to the rights protected by the State and Federal Constitutions as it denies rights to privacy, education, free assembly, religious expression, consensual use of one’s physical body and liberty. "About FEAT"FEAT is a non-profit, volunteer-driven organization of parents, family members, and treatment professionals dedicated to providing best outcome Education, Advocacy and Support for the Northern California Autism Community."[many members believe that vaccines caused their children's autism]------------[Health Committee Analysis] Homeschool Association of California"The Homeschool Association of California states this bill will negatively impact the freedom to homeschool and would make it impossible for many families to choose to homeschool legally. They argue that almost all homeschooling families use a legal option that involves attendance at some form of public or private school, either operated by a third party or operated by parents who file a private school affidavit, yet current law requires children admitted to private schools be fully vaccinated in accordance with existing law."AboutHSC[nothing on the website.][from hearing ] 1:25:40 Home School Association of California------------[Health Committee Analysis] National Vaccine Information Center"The National Vaccine Information Center argues that it’s particularly disturbing that physicians in the American Medical Association Code of Ethics affirm philosophical and religious exemptions for themselves yet want to remove this right for California parents."About NVICDespite its name, it is not a government body. It is a nonprofit whose mission is to create fear and doubt about the safety and efficacy of vaccines. The best introduction:NVIC? Know the Omissions------------[Health Committee Analysis] Pacific Justice Institute Center for Public Policy"The Pacific Justice Institute argues that statewide vaccination rates exceed the threshold for herd immunity and, to the extent that a few communities have fallen below vaccination levels needed for herd immunity, the Legislature could consider approaches that allow greater local decision-making and accountability rather than imposing across-the-board statewide mandates."About -- from Wikipedia.PJI provides pro bono representation in matters involving the exercise of religion and other civil liberties. It has supported the recitation of "under God" as part of the Pledge of Allegiance in public schools, homeschooling, and the enforcement of the Religious Land Use and Institutionalized Persons Act.In 2014, the Southern Poverty Law Center designated the Pacific Justice Institute as an anti-LGBT hate group.------------[Health Committee Analysis] ParentalRights.orgAbout"ParentalRights.Org's mission is to protect children by empowering parents through adoption of the Parental Rights Amendment to the U.S. Constitution and by preventing U.S. ratification of UN Conventions that threaten parental right:" It is primarily organized in opposition to the UN Convention On The Rights Of The Child, which has long been a dog-whistle element in the far right.------------[Health Committee Analysis] SafeMindsAboutSafeMinds is an acronym for Sensible Action For Ending Mercury Induced Neurological Disorders. It is a parent-advocacy group that originally advanced the belief that thimerosal in vaccines cause autism; it has since morphed into a general-purpose anti-vaccine, autism-hating organization. It used to have rather a lot of clout; the failure of the mercury-causing-autism hypothesis in the Omnibus Autism Hearing (OAR) seems to have made the organization much less relevant.SAFEMINDS board:Sallie Bernard (New Jersey)Lyn Redwood, RN, MSN (Georgia)Heidi Roger (New Jersey/New York)Laura Bono (North Carolina)Maria Dwyer (New York)Albert EnayatiStephen D. KetteScott LasterJackie Lombardo (Virginia)Cynthia Nevison (Colorado)Katie Weisman (New York)Katie Wright (Connecticut)------------Hundreds of individuals------[from hearing ] 1:16:25 Ruth Westerich (sp) claiming to speaking for a San Diego "group of over 500,000 healthcare practitioners"-------[from hearing ] 1:17:59 Wendy Silver; Million Mamas MovementPrimarily a peace movement but also no vaccines, evidently.Become a Million Mama - Million Mamas Movement-------[from hearing ] 1:18:35 Slow and No Vax Moms"extensive yahoo group in your area for parents. It's called slow and no vax moms. Look it up, and they should be able to help you."Yahoo! Groups"This group does not advocate any vaccine over another as they all have adverse effects. All discussion is from a "con" stance for each and every vaccine. This is not a place for debate of the vaccine issues. Discussing the "pro" viewpoint of any vaccine is not a part of this forum in any way."---------[from hearing ] 1:18:55 Campaign for Liberty, asks Senator Allen for a dateRon Paul's groupCampaign for Liberty - Reclaim the Republic. Restore the Constitution.---------[from hearing ]1:21:56 Laura Hayes; Mind Institute, FEAT, California Coalition for Health Choice, Canary PartyHayes of the founding families of MIND Institute; still fixated on vaccines cause autism. She's been published at the anti-vaccine, autism-hating blog Age of Autism.David Gorski, writing as Orac, has addressed her issues:No, no, no! Fifteen times, no!Tactics and tropes of the antivaccine movement (2014 edition)The annals of “I’m not antivaccine,” part 14: Vaccine “Trafficking” and beyond-----------[from hearing ]1:27:51 Our Kids, Our Choice(seems to be a subgroup of the other antis, focusing on producing Youtube Videos)-------[from hearing ] 1:30:30 Congressional Prayer Conference of Washington DC, spoke about the letter they sent to Senator Pan the day before and is very aggressive in tone. Laughter from oppo when he finishes------[from hearing ] 1:40:35 Dr. Bob Sears[anti-vaccine pediatrician]-------[from hearing ] 1:48:20 Environmental Voiceshttp://environmentalvoices.org/index.htmlRocklin-based anti-GMO, anti-toxins organization.--------[from hearing ] 2:00:03 National Autism Association of CAHelp and Hope for Families Affected by Autism[believes that vaccines cause some or most cases of autism. National organization with state chapters -- several in California]-----[from hearing] Dana Gorman, Thriiive.com and #b1less
- Home >
- Catalog >
- Life >
- Score Sheet >
- Interview Score Sheet >
- candidate evaluation form sample interviewer >
- Impact Consent Form - Mayo Clinic Health System