The Guide of drawing up Form W-4 (2012 Online
If you are curious about Alter and create a Form W-4 (2012, heare are the steps you need to follow:
- Hit the "Get Form" Button on this page.
- Wait in a petient way for the upload of your Form W-4 (2012.
- You can erase, text, sign or highlight of your choice.
- Click "Download" to conserve the changes.
A Revolutionary Tool to Edit and Create Form W-4 (2012
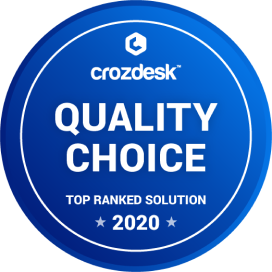
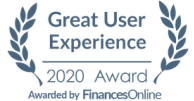
How to Easily Edit Form W-4 (2012 Online
CocoDoc has made it easier for people to Customize their important documents with the online platform. They can easily Edit through their choices. To know the process of editing PDF document or application across the online platform, you need to follow the specified guideline:
- Open the official website of CocoDoc on their device's browser.
- Hit "Edit PDF Online" button and Append the PDF file from the device without even logging in through an account.
- Edit your PDF document online by using this toolbar.
- Once done, they can save the document from the platform.
Once the document is edited using online website, the user can easily export the document as what you want. CocoDoc ensures that you are provided with the best environment for implementing the PDF documents.
How to Edit and Download Form W-4 (2012 on Windows
Windows users are very common throughout the world. They have met hundreds of applications that have offered them services in modifying PDF documents. However, they have always missed an important feature within these applications. CocoDoc are willing to offer Windows users the ultimate experience of editing their documents across their online interface.
The procedure of modifying a PDF document with CocoDoc is very simple. You need to follow these steps.
- Choose and Install CocoDoc from your Windows Store.
- Open the software to Select the PDF file from your Windows device and move on editing the document.
- Customize the PDF file with the appropriate toolkit offered at CocoDoc.
- Over completion, Hit "Download" to conserve the changes.
A Guide of Editing Form W-4 (2012 on Mac
CocoDoc has brought an impressive solution for people who own a Mac. It has allowed them to have their documents edited quickly. Mac users can create fillable PDF forms with the help of the online platform provided by CocoDoc.
In order to learn the process of editing form with CocoDoc, you should look across the steps presented as follows:
- Install CocoDoc on you Mac firstly.
- Once the tool is opened, the user can upload their PDF file from the Mac in seconds.
- Drag and Drop the file, or choose file by mouse-clicking "Choose File" button and start editing.
- save the file on your device.
Mac users can export their resulting files in various ways. With CocoDoc, not only can it be downloaded and added to cloud storage, but it can also be shared through email.. They are provided with the opportunity of editting file through multiple ways without downloading any tool within their device.
A Guide of Editing Form W-4 (2012 on G Suite
Google Workplace is a powerful platform that has connected officials of a single workplace in a unique manner. When allowing users to share file across the platform, they are interconnected in covering all major tasks that can be carried out within a physical workplace.
follow the steps to eidt Form W-4 (2012 on G Suite
- move toward Google Workspace Marketplace and Install CocoDoc add-on.
- Select the file and Hit "Open with" in Google Drive.
- Moving forward to edit the document with the CocoDoc present in the PDF editing window.
- When the file is edited completely, download or share it through the platform.
PDF Editor FAQ
There are only three types of bonds like single bond, double bond and triple bond in chemistry. Why isn't tetra bond possible between two atoms?
Quadruple bonds, quintuple bonds, and even sextuple chemical bonds exist.Quadruple bonds are rarer than single, double and triple bonds but occur amongst the transition metals especially for Cr, Mo, W, & Re. Eg [Mo2Cl8]4- and [Re2Cl8]2- . In the terminology of molecular orbital theory the bonding is described as sigma2pi4delta2.Chromium II acetate was the first chemical containing a quadruple bond to be synthesised. It was described in 1844 by E. Peligot, but it's distinctive bonding was not recognised for over 100 years.Many other compounds containing Quadruple bonds between transition metals have been described; often by Cotton and coworkers.Quadruple bonds between atoms of main group elements are unknown. However a very recent paper by S. Shaik et.al. ( Nature Chemistry 4 ( 2012 ) pp195 - 200 ) proposes that a quadruple bond exists in diatonic carbon, C2.A chemical compound containing the quintuple bond was first reported in 2005 for a dichromium compound.Several quintuple bonded compounds have been reported since that time ( 2005 ); the metal - metal bond usually stabilised within a complex.The quintuple bond may be described as a Sigma2pi4delta4 10 electron bond between two metal centres.Quintuple bonded complexes are usually achieved by the reduction of a bimetallic species with potassium graphite, KC8.A Sextuple chemical bond is a covalent chemical bond involving 12 bonding electrons. To date this type of bonding is only known to occur in gaseous Mo2, and W2.There is strong evidence in believing that no two elements in the periodic table below about atomic number 100 can form bonding orders above 6 ( See Roos,B.O, et.al, ( 2007),Angewandte Chemie International Edition 46 (9), 1469 - 72 ).
What are the repercussions of hiring an undocumented person and paying them cash?
“What are the repercussions of hiring an undocumented person and paying them cash?”We presume that the cash payment is done without proper withholding and reporting of income. As others have noted in detail, there are several federal crimes associated with this practice, which can in heavy fines and potentially, in some cases, a prison sentence. In the US, when the IRS is involved, it typically takes a number of other steps before resorting to formal criminal prosecution for tax evasion. But those other measures can still ruin your day.There are immigration related statutes and federal revenue (tax) evasion issues involved:A good example is the indictment on tax charges of three men in Pennsylvania, Vanny Son, 33, Son Thach, 55, and Hung Danh, 54. They allegedly participated in a tax scheme costing the IRS over $1 million. U.S. Attorney Peter Smith alleges that Son and Thach operated five employee leasing businesses between 2006 and 2012, paying their employees over $7 million in cash without withholding income or payroll taxes.Employers must withhold income taxes from employee wages based on the number of allowances employees claim on the Form W-4. Employers also have to withhold FICA taxes from wages and promptly pay them. There are quarterly and annual employment tax forms too. Apart from the trust fund portion that represents money withheld from employee wages, the employer also pays a share.Source:Paying In Cash? Careful, It Can Mean Jail
What is the purpose for using slack variable in SVM?
The standard SVM classifier works only if you have a well separated categories. To be more specific, they need to be linearly separable. It means there exist a line (or hyperplane) such that all points belonging to a single category are either below or above it. In many cases that condition is not satisfied, but still the two classes are pretty much separated except some small training data where the two categories overlap. It wouldn’t be a huge error if we would draw a line (somewhere in between) and accept some level of error - having training data on the wrong side of the marginal hyperplanes. How do we measure the error? The answer is: slack variables. For each training data point we can define a variable that measures the distance of the point to its marginal hyperplane (dahsed line in the figure), lets call it [math]\xi^*_i[/math]. Whenever the point is on the wrong site of the marginal hyperplane we quantify the amount of error by the ratio between [math]\xi^*_i[/math] and half of the margin, i.e. distance between separating hyperplane and marginal hyperplane (M in the figure). Points on the correct site are not quantified as errors. This is a geometrical interpretation of slack variables [math]\xi_i[/math]. You can now go back to the initial SVM problem and maximize the margin in the presence of errors. The larger the error that you allow for, the wider the margin (numerical illustration at the end).Mathematical FormulationWe are dealing with a training set [math]\{y_i, \mathbf{x}_i\}_{i=1}^{N}[/math], where [math]y_i \in \{-1,+1\}[/math] and [math]\mathbf{x}_i \in R^{p}[/math] are p-dimensional vectors of features. The p-dimensional hyperplane [math]\mathcal{H}_{(\mathbf{w},w_0)}[/math] is defined as a collection of points [math]\mathbf{x} \in R^p[/math] satisfying the equation [math]\mathbf{w}^T \cdot \mathbf{x} + w_0 = 0[/math], i.e.[math]\mathcal{H}_{(\mathbf{w},w_0)} = \{\mathbf{x} \in R^{p}: \mathbf{w}^T \cdot \mathbf{x} + w_0 = 0 \}.[/math]Vector [math]\mathbf{w} \in R^p[/math] together with [math]w_0[/math] define the hyperplane.Hard marginThe model applies only to a linearly separable set for which there exists a hyperplane [math]\mathcal{H}_{(\mathbf{w},w_0)}[/math], that separates two categories, i.e.[math]\begin{cases} \mathbf{w}^T \cdot \mathbf{x}_i + w_0 \geqslant 1, & \text{if } y_i = +1,\\ \mathbf{w}^T \cdot \mathbf{x}_i + w_0 \leqslant -1, & \text{if } y_i = -1, \end{cases}[/math]or equivalently in a single equation:[math]y_i(\mathbf{w}^T \cdot \mathbf{x} + w_0) \geqslant 1.[/math]Every training point is either above the hyperplane [math]\mathcal{H}_(\mathbf{w},w_0-1)[/math] or below the hyperplane [math]\mathcal{H}_(\mathbf{w},w_0+1)[/math] . We call them marginal hyperplanes. Of course there are infinitely many hyperplanes for which the condition is satisfied, but the hyperplane with the largest margin is the one that appears in the Support Vector Machine model. Margin is the distance between marginal hyperplanes. It is inversely proportional to the length of the vector [math]\mathbf{w}[/math] (2M in the figure below). The optimization problem is equivalently stated as the minimization of the inverse squared (of the margin):[math]\min\limits_{\mathbf{w},w_0}\left\{ \frac{1}{2}||\mathbf{w}||^2\right\},[/math]subject to the condition of linear separability.Slack variablesImagine that you have a training set that violates the linear separability criterion. At the same time you observe that the two categories are pretty much separated (except some group of points). It would still be nice to find a hyperplane that marks the boundary between the two classes.In the above figure we see that blue and red points are spatially separated, but there is a small overlapping region. Drawing a line somewhere between the two blobs wouldn’t be very objective and each time you try it would be different. However, we can define a separating hyperplane in a systematic way by introducing slack variables [math]\xi_i[/math] and minimizing the total error:[math]\min\limits_{\mathbf{w},w_0,\xi}\left\{ \sum\limits_{i=1}^{N}\xi_i\right\}.[/math]Slack variables are positive (or zero), local quantities that relax the stiff condition of linear separability, where each training point is seeing the same marginal hyperplane. Now, each individual training point can see a different, but parallel hyperplane:[math]\begin{cases} \mathbf{w}^T \cdot \mathbf{x}_i + w_0 \geqslant 1 - \xi_i, & \text{if } y_i = +1\\ \mathbf{w}^T \cdot \mathbf{x}_i + w_0 \leqslant -1 + \xi_i, & \text{if } y_i = -1 \end{cases} [/math]which can be cast into a single equation:[math]y_i(\mathbf{w}^T \cdot \mathbf{x} + w_0) \geqslant 1 - \xi_i.[/math]In this equation, slack variables [math]\xi_i \geqslant 0[/math] can be any positive numbers. Literally any! There is no upper bound. However, I wrote earlier that we should minimize the error and that brings us to the first estimate of their value:[math]\xi_i = \max(0, 1-y_i(\mathbf{w}^T \cdot \mathbf{x}_i + w_0)),[/math]where the right hand side is also called the Hinge loss function. The next step is to find a hyperplane that minimizes the collective Hinge loss:[math]\min\limits_{\mathbf{w},w_0}\left\{ \sum\limits_{i=1}^{N}\max(0, 1-y_i(\mathbf{w}^T \cdot \mathbf{x}_i + w_0))\right\}.[/math]As you can see, it is optimal to have as many points as possible on the correct side of the marginal hyperplanes, because then [math]\xi_i=0[/math]. Further optimization tries to minimize the relative distance between the training point and the corresponding marginal hyperplane (see figure below). I wrote relative, because slack variables can be geometrically defined as the ratio between the distance [math]\xi_i^*[/math], from a training point to a marginal hyperplane, and half of the margin [math]M=1/||\mathbf{w}||[/math], i.e. [math]\xi_i = \xi_i^*/M[/math]. In order to minimize the error the margin size will tend to be small.Soft margin HyperplaneWe can now consider a combined effect. We would like to maximize the margin and allow for total error of some magnitude. Optimization problem takes the form[math]\min\limits_{\mathbf{w},w_0,\xi}\left\{ \frac{1}{2}||\mathbf{w}||^2 + C\sum\limits_{i=1}^{N}\xi_i\right\},[/math]subject to constraints,[math]y_i(\mathbf{w}^T \cdot \mathbf{x} + w_0) \geqslant 1 - \xi_i, \\ \xi_i \geqslant 0.[/math]The variable [math]C[/math] is the penalty strength, which specifies how much do we care about errors (training points that are on the wrong side). The [math]C=\infty[/math] corresponds to a hard-margin (if possible). Still, if you want to minimize the above equation, slack variables are given by the Hinge loss function.The effect of the penalty strength is shown below.Lastly, one important thing. In the figure you see that some of the points have a black ring around. They are called support vectors. Linear combination of those training points define [math]\mathbf{w}[/math]. If the penalty is small the number of training points that define the separating hyperplane is large.ReferencesC. Cortes, V. Vapnik, Support-vector networks, Machine Learning, 20, 273-297 (1995).Trevor Hastie, Robert Tibshirani & Jerome Friedman, The elements of statistical learning, Springer (2009).Kevin P. Murphy, Machine learning: a probabilistic perspective, The MIT Press (2012).Meshryar Mohri, Afshin Rostamizadeh & Ameet Talwalkar, Foundations of machine learning, The MIT Press (2012).
- Home >
- Catalog >
- Life >
- Citizenship And Immigration Form >
- Form I-9 >
- Form W-4 (2012