How to Edit Your Improved Credit Scoring With Multilevel Statistical Modelling Online Lightning Fast
Follow the step-by-step guide to get your Improved Credit Scoring With Multilevel Statistical Modelling edited with efficiency and effectiveness:
- Hit the Get Form button on this page.
- You will go to our PDF editor.
- Make some changes to your document, like highlighting, blackout, and other tools in the top toolbar.
- Hit the Download button and download your all-set document into you local computer.
We Are Proud of Letting You Edit Improved Credit Scoring With Multilevel Statistical Modelling Like Using Magics
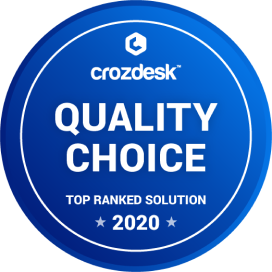
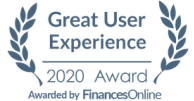
Discover More About Our Best PDF Editor for Improved Credit Scoring With Multilevel Statistical Modelling
Get FormHow to Edit Your Improved Credit Scoring With Multilevel Statistical Modelling Online
If you need to sign a document, you may need to add text, Add the date, and do other editing. CocoDoc makes it very easy to edit your form with the handy design. Let's see how do you make it.
- Hit the Get Form button on this page.
- You will go to this PDF file editor webpage.
- When the editor appears, click the tool icon in the top toolbar to edit your form, like adding text box and crossing.
- To add date, click the Date icon, hold and drag the generated date to the target place.
- Change the default date by changing the default to another date in the box.
- Click OK to save your edits and click the Download button for sending a copy.
How to Edit Text for Your Improved Credit Scoring With Multilevel Statistical Modelling with Adobe DC on Windows
Adobe DC on Windows is a useful tool to edit your file on a PC. This is especially useful when you finish the job about file edit without using a browser. So, let'get started.
- Click the Adobe DC app on Windows.
- Find and click the Edit PDF tool.
- Click the Select a File button and select a file from you computer.
- Click a text box to edit the text font, size, and other formats.
- Select File > Save or File > Save As to confirm the edit to your Improved Credit Scoring With Multilevel Statistical Modelling.
How to Edit Your Improved Credit Scoring With Multilevel Statistical Modelling With Adobe Dc on Mac
- Select a file on you computer and Open it with the Adobe DC for Mac.
- Navigate to and click Edit PDF from the right position.
- Edit your form as needed by selecting the tool from the top toolbar.
- Click the Fill & Sign tool and select the Sign icon in the top toolbar to customize your signature in different ways.
- Select File > Save to save the changed file.
How to Edit your Improved Credit Scoring With Multilevel Statistical Modelling from G Suite with CocoDoc
Like using G Suite for your work to complete a form? You can make changes to you form in Google Drive with CocoDoc, so you can fill out your PDF without worrying about the increased workload.
- Go to Google Workspace Marketplace, search and install CocoDoc for Google Drive add-on.
- Go to the Drive, find and right click the form and select Open With.
- Select the CocoDoc PDF option, and allow your Google account to integrate into CocoDoc in the popup windows.
- Choose the PDF Editor option to open the CocoDoc PDF editor.
- Click the tool in the top toolbar to edit your Improved Credit Scoring With Multilevel Statistical Modelling on the needed position, like signing and adding text.
- Click the Download button to save your form.
PDF Editor FAQ
What are the stastical topics in data science?
It is important to understand the ideas behind the various techniques, in order to know how and when to use them. One has to understand the simpler methods first, in order to grasp the more sophisticated ones. It is important to accurately assess the performance of a method, to know how well or how badly it is working.1 . Subset Selection:This approach identifies a subset of the p predictors that we believe to be related to the response. We then fit a model using the least squares of the subset features.Best-Subset Selection: Here we fit a separate OLS regression for each possible combination of the p predictors and then look at the resulting model fits. The algorithm is broken up into 2 stages: (1) Fit all models that contain k predictors, where k is the max length of the models, (2) Select a single model using cross-validated prediction error. It is important to use testing or validation error, and not training error to assess model fit because RSS and R² monotonically increase with more variables. The best approach is to cross-validate and choose the model with the highest R² and lowest RSS on testing error estimates.Forward Stepwise Selection considers a much smaller subset of ppredictors. It begins with a model containing no predictors, then adds predictors to the model, one at a time until all of the predictors are in the model. The order of the variables being added is the variable, which gives the greatest addition improvement to the fit, until no more variables improve model fit using cross-validated prediction error.Backward Stepwise Selection begins will all p predictors in the model, then iteratively removes the least useful predictor one at a time.Hybrid Methods follows the forward stepwise approach, however, after adding each new variable, the method may also remove variables that do not contribute to the model fit.2 . Resampling Methods:Resampling is the method that consists of drawing repeated samples from the original data samples. It is a non-parametric method of statistical inference. In other words, the method of resampling does not involve the utilization of the generic distribution tables in order to compute approximate p probability values.Resampling generates a unique sampling distribution on the basis of the actual data. It uses experimental methods, rather than analytical methods, to generate the unique sampling distribution. It yields unbiased estimates as it is based on the unbiased samples of all the possible results of the data studied by the researcher. In order to understand the concept of resampling, you should understand the terms Bootstrapping and Cross-Validation:Bootstrapping is a technique that helps in many situations like validation of a predictive model performance, ensemble methods, estimation of bias and variance of the model. It works by sampling with replacement from the original data, and take the “not chosen” data points as test cases. We can make this several times and calculate the average score as estimation of our model performance.On the other hand, cross validation is a technique for validating the model performance, and it’s done by split the training data into k parts. We take the k — 1 parts as our training set and use the “held out” part as our test set. We repeat that k times differently. Finally, we take the average of the k scores as our performance estimation.Usually for linear models, ordinary least squares is the major criteria to be considered to fit them into the data. The next 3 methods are the alternative approaches that can provide better prediction accuracy and model interpretability for fitting linear models.3 . Tree-Based Methods:Tree-based methods can be used for both regression and classification problems. These involve stratifying or segmenting the predictor space into a number of simple regions. Since the set of splitting rules used to segment the predictor space can be summarized in a tree, these types of approaches are known as decision-tree methods. The methods below grow multiple trees which are then combined to yield a single consensus prediction.Bagging is the way decrease the variance of your prediction by generating additional data for training from your original dataset using combinations with repetitions to produce multistep of the same carnality/size as your original data. By increasing the size of your training set you can’t improve the model predictive force, but just decrease the variance, narrowly tuning the prediction to expected outcome.Boosting is an approach to calculate the output using several different models and then average the result using a weighted average approach. By combining the advantages and pitfalls of these approaches by varying your weighting formula you can come up with a good predictive force for a wider range of input data, using different narrowly tuned models.The random forest algorithm is actually very much like bagging. Also here, you draw random bootstrap samples of your training set. However, in addition to the bootstrap samples, you also draw a random subset of features for training the individual trees; in bagging, you give each tree the full set of features. Due to the random feature selection, you make the trees more independent of each other compared to regular bagging, which often results in better predictive performance (due to better variance-bias trade-offs) and it’s also faster, because each tree learns only from a subset of features.4 . Unsupervised Learning:So far, we only have discussed supervised learning techniques, in which the groups are known and the experience provided to the algorithm is the relationship between actual entities and the group they belong to. Another set of techniques can be used when the groups (categories) of data are not known. They are called unsupervised as it is left on the learning algorithm to figure out patterns in the data provided. Clustering is an example of unsupervised learning in which different data sets are clustered into groups of closely related items. Below is the list of most widely used unsupervised learning algorithms:Principal Component Analysis helps in producing low dimensional representation of the dataset by identifying a set of linear combination of features which have maximum variance and are mutually un-correlated. This linear dimensionality technique could be helpful in understanding latent interaction between the variable in an unsupervised setting.k-Means clustering: partitions data into k distinct clusters based on distance to the centroid of a cluster.Hierarchical clustering: builds a multilevel hierarchy of clusters by creating a cluster tree.This was a basic run-down of some basic statistical techniques that can help a data science program manager and or executive have a better understanding of what is running underneath the hood of their data science teams. Truthfully, some data science teams purely run algorithms through python and R libraries. Most of them don’t even have to think about the math that is underlying. However, being able to understand the basics of statistical analysis gives your teams a better approach. Have insight into the smallest parts allows for easier manipulation and abstraction. I hope this basic data science statistical guide gives you a decent understanding!Credit : Intro to Statistical Learning book .
- Home >
- Catalog >
- Life >
- Invitation Template >
- free invitations online >
- Improved Credit Scoring With Multilevel Statistical Modelling