How to Edit The We Are Very Excited To Be Able To Offer Another Great Program And We Hope We Have with ease Online
Start on editing, signing and sharing your We Are Very Excited To Be Able To Offer Another Great Program And We Hope We Have online refering to these easy steps:
- click the Get Form or Get Form Now button on the current page to make your way to the PDF editor.
- hold on a second before the We Are Very Excited To Be Able To Offer Another Great Program And We Hope We Have is loaded
- Use the tools in the top toolbar to edit the file, and the edited content will be saved automatically
- Download your modified file.
A top-rated Tool to Edit and Sign the We Are Very Excited To Be Able To Offer Another Great Program And We Hope We Have
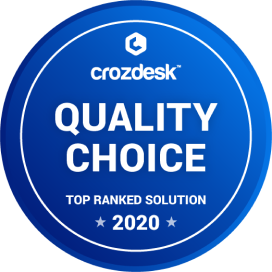
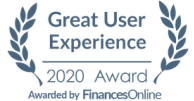
Start editing a We Are Very Excited To Be Able To Offer Another Great Program And We Hope We Have in a second
Get FormA clear tutorial on editing We Are Very Excited To Be Able To Offer Another Great Program And We Hope We Have Online
It has become quite simple recently to edit your PDF files online, and CocoDoc is the best PDF editor you have ever used to make changes to your file and save it. Follow our simple tutorial to start!
- Click the Get Form or Get Form Now button on the current page to start modifying your PDF
- Add, modify or erase your content using the editing tools on the tool pane above.
- Affter editing your content, put on the date and make a signature to complete it perfectly.
- Go over it agian your form before you click the download button
How to add a signature on your We Are Very Excited To Be Able To Offer Another Great Program And We Hope We Have
Though most people are in the habit of signing paper documents by writing, electronic signatures are becoming more popular, follow these steps to sign PDF online for free!
- Click the Get Form or Get Form Now button to begin editing on We Are Very Excited To Be Able To Offer Another Great Program And We Hope We Have in CocoDoc PDF editor.
- Click on the Sign icon in the tool menu on the top
- A box will pop up, click Add new signature button and you'll be given three options—Type, Draw, and Upload. Once you're done, click the Save button.
- Move and settle the signature inside your PDF file
How to add a textbox on your We Are Very Excited To Be Able To Offer Another Great Program And We Hope We Have
If you have the need to add a text box on your PDF and create your special content, do the following steps to carry it out.
- Open the PDF file in CocoDoc PDF editor.
- Click Text Box on the top toolbar and move your mouse to carry it wherever you want to put it.
- Fill in the content you need to insert. After you’ve typed the text, you can utilize the text editing tools to resize, color or bold the text.
- When you're done, click OK to save it. If you’re not settle for the text, click on the trash can icon to delete it and begin over.
An easy guide to Edit Your We Are Very Excited To Be Able To Offer Another Great Program And We Hope We Have on G Suite
If you are seeking a solution for PDF editing on G suite, CocoDoc PDF editor is a suggested tool that can be used directly from Google Drive to create or edit files.
- Find CocoDoc PDF editor and install the add-on for google drive.
- Right-click on a chosen file in your Google Drive and select Open With.
- Select CocoDoc PDF on the popup list to open your file with and allow CocoDoc to access your google account.
- Make changes to PDF files, adding text, images, editing existing text, annotate with highlight, polish the text up in CocoDoc PDF editor before pushing the Download button.
PDF Editor FAQ
How is Storm8/Team Lava still able to use bots?
Not sure if this is "entertaining", but hopefully this will be educational for everyone.I recently gave a talk at Casual Connect 2012 to give more information on Storm8 and one of the topics was how we leverage our strong network of gamers to grow our user base. When we release a new game, we tell our existing users to try it out and this is how we market our new titles.Here is some simple math:Storm8 DAU: 6,000,000+Installs: 50,000 (number that was quoted by Anon User)---------------------------------------Percentage: ~1%Each day we only need about 1% of our players to show interest in playing the new game and we can already achieve over 50,000 installs. I can tell you that our conversion number is a lot higher than 1% because our 6 M+ users are very targeted and dedicated social gamers.Yes, if you are "GREE, Zynga, TinyCo and other developers" that do not have this strong network of players, you will have to spend tons on advertising and marketing, but not when you have a network like Storm8's.Here's another example. We released our latest game called Bubble Mania and in the first 3 days, we hit over 1 Million installs and it is with $0 spent on ads (http://venturebeat.com/2012/08/01/bubble-mania-breaks-record/). It's a great game and our players were very excited to try it out!As for the reviews, RS:WG has been promoted for only 2 weeks so of course it only got 1500 reviews (very inline with other apps), where as Bubble Mania has been released for 2 months and it has over 36,000 reviews.As for the use of bots, Storm8 has never and has no need to use such tactic. The use of bots is against Apple's policy and Storm8 is very align with Apple to maintain the integrity of its ecosystem.To the Anon User above, we are growing rapidly at Storm8. We are always looking for talented game developers to join our team and you seem very passionate about this space. We are located at sunny Redwood Shores, California. We offer very competitive salaries, company bonus program and RSU's, free gourmet lunch and dinners, $ to pimp out your desk and the ability to work with one of the best gaming teams! If you’re interested to learn more about Storm8, please let me know, [email protected].
I slept with a woman 30 years younger than me. She's even younger than my daughter. Is this immoral?
There *is* something wrong with this, even if the sex is consensual. I went anonymous here to protect myself, but I’m female—the first female to answer this question, in fact. I want to offer another perspective than the standard male “more power to you if she’s willing” that all the other answers exemplify here.I dated a 50 year old man starting when I was 30. It lasted 2 years. In that time, I fell deeply and passionately in love with him. He was older, wiser, incredibly intelligent, and very rich. His age and experience was a powerful aphrodisiac, and it intoxicated me. I felt that I *must* be smarter than average and worthy of respect “beyond my years” as proven by the fact that such an accomplished older man was interested in me. I craved a man who could expand my horizons and make me feel protected in a way that a younger man could not. Not to mention, the thought of being the beneficiary of so many more years experience in the bedroom is… exhilarating.But here’s the rub: think of the reasons *why* a 20 year old woman would be interested in you and not a man her own age. Really ask yourself this question.Here is my list of reasons for falling in love with a much older man. Keep in mind that I was not consciously aware of any of these motivations at the time, so if he would have asked me, I would not have been able to produce these at all. Keep in mind also that I had 10 more years of life experience than your girl does currently, and I have always been considered “mature for my age”:I craved validation that I was smart. On the cusp of taking on new roles in my career—ones that would define my path and demand a high level of thought leadership and responsibility for the first time—I was incredibly insecure about my intelligence. So I wanted the smartest man I could find—naturally, an older one. I assumed that he was primarily attracted to my mind, because we had known each other for a long time before we started dating and had many long, intelligent conversations where our attraction had a chance to grow and blossom through mutual respect (or so I thought).I felt lost and confused about where I was going with my career, and since he was a world-renowned professor in a field related to mine, I thought he could help me “fix” all my problems and provide expert mentoring.I was disillusioned by my sexual experiences with young men, who didn’t seem to be interested in doing anything other than mounting me and coming as quickly as possible. No passion, no taking their time— no making love to me. Just sex. I assumed an older man would be beyond this juvenile approach and actually be tender, take his time, and make me feel like a person and not just a body during sex.I had been sexually assaulted in the past, and never felt safe. I was convinced that I had nothing to fear in the arms of such an accomplished, strong, and wise man. He wasn’t like other young men I knew who would fly off the handle and get in fights for stupid reasons. He was steady, trained, and calculating. He was skilled in martial arts. He owned many guns, and was an expert in using all of them. His house felt like Fort Knox— like nothing could touch me there (but him). Being able to let go of my fear was such a departure from my everyday experience up until I met him that I didn’t want to let it go… ever. I wanted to be around him—to feel the safety he provided and that I craved—every second. It was a HUGE burden lifted from my mind to be in his presence.He had money. Lots of money. I come from a poor family, and I had never known what it felt like to literally be able to have anything I wanted. He had a reputation for taking his “girls” on extended vacations all over the world, and I had never traveled before. I’m not a gold digger and didn’t fall for him *because* of his money, but the prospect of a me—a small town girl who had never seen the world—having that opportunity at a young age was incredibly attractive.It was exciting to be in a “forbidden” relationship. One that even my closest family and friends couldn’t know about. Human nature dictates that we want things we perceive we can’t, or are not supposed to, have. Also, my parents were always extremely controlling and I took extreme pleasure in the thought that they would have absolutely no say in a decision that I *knew* they would disapprove of.Here’s how all of that played out over time…Turns out that, while I was incredibly attracted to his mind and thought I was getting intellectually validated, he was (big surprise) primarily excited about my body. How could the mind of a woman 20 years his junior actually excite him the way his did for me? Why did I ever think it could? I was, in a word, devastated. My deepest fear—that I wasn’t “smart enough”— eventually crept in and colored all of our interactions when I could tell that he was mostly just interested in the sex. I became deeply frustrated, then angry and defensive. Since I had no idea that I was seeking external validation, it was a trap I couldn’t find my way out of.He tried to mentor me, but two things would happen: (a) he would start feeling like my father, which was weird. (b) he would suggest that I do things that were *incredibly* difficult, if not impossible, for me at the time. Because, naturally, those things were easy for him. When I was frustrated with how I was being treated by my boss, his solution was to win a promotion to oust him—because that’s what he would have done in that situation. When I struggled with learning how to program, he suggested I *start* with writing algorithms because “it’s easy!” He could never put himself back in the shoes of a person of my age and confidence to know how trapped I felt by his “suggestions,” no matter how hard he tried. I interpreted this, once again, as him being an insensitive jerk.Since my body was the most exciting thing about me, he didn’t “make love” to me as I had desperately hoped he would. On the contrary, he played out his own domination fantasies with me and dressed me up endlessly in little skimpy outfits… outfits that were not to my taste at all, and made me feel trampy. He just got endless pleasure out of my body. It was nice to be able to fulfill him at first, but then it just felt… disgusting. This was *not* how I wanted to fulfill him primarily. I wanted to be his intellectual equal, and every time we had sex I was reminded that I wasn’t. Not even close. The sexual games hurt, and bad.I was at his house constantly. I immediately felt a sense of dread when I stepped out his front door. The intense awareness of the safety I enjoyed in his house only magnified the intensity of my fear when I had to leave it. I slept over almost every night and was there every weekend, and started overstaying my welcome because of how addicting his home environment felt to me. He eventually pushed back and wanted his privacy. At the time, that felt like a HUGE betrayal. Like, how could he not understand how much I *needed* this place, and how much I *deserved* this place… ?We went on two exciting vacations. He paid for everything. He felt this justified him setting the terms on how we spent our time. When I wasn’t up for something he wanted, I was an ingrate and ruining his vacation. I came to understand that the money came with strings attached, so I pulled back. I went on a campaign of proving to him that I loved *him* and not his money (100% true), which I thought he would find endearing. But when I wasn’t interested in him buying me fancy clothes and purses (because I’m not into that sort of thing), he actually detested me for it. He had a huge resource that he desperately *wanted* to provide, and when I resisted him providing it to “prove my love”, he didn’t interpret that as love— he interpreted it as emasculating of him and a waste of his immense resource.We enjoyed several months of secret “trysts” that we had to lie to all our friends to pull off. He had much more to lose than I did, as a respected faculty member at a prestigious university. It was fun at first, but then my friendships started waning because I repeatedly declined offers to hang out with my friends so I could see Him. When I expressed an interest in revealing our relationship to my best friend, he repelled the thought and forbade me to tell anyone because he was afraid of the “professional consequences.” For over a year I obeyed, dealing with all of the emotional turmoil described above without the aid of my closest friends to help me process what was happening (any of them likely could have seen through everything and helped me get out sooner). Eventually I put my foot down and demanded to tell my best friend when the burden of lying got to be too great to bear. I thought things would get much easier for me once she knew, but they got much harder. Turns out that people get really pissed off when they find out you’ve been lying to them for over a year. I lost my best friend, and to this day we still don’t speak.I hope this has given you some perspective on your newfound, exciting Tinder match. Unfortunately, most women who are 20 (and apparently, even 30) are still working through the emotional baggage of their childhoods and figuring out who they are and who they want to be. You will become the epicenter of any and all fears and insecurities that exist in her life if you choose to enter into any sort of relationship with her—even a casual one. This will damage her emotionally and set back her personal and professional development, no matter how much you don’t want it to.Please think twice before abusing your power and choosing to date a much younger woman. You can’t possibly give her what she needs right now, though she will be convinced you can—and that will have her stay far past the point she can handle. It is a perfect storm, and it won’t have a happy ending.
How does LinkedIn's recommendation system work?
I gave this talk earlier this week at Hadoop World(http://www.hadoopworld.com/session/linkedin-products-you-may-like/), a conference that is evangelizing Hadoop by way of highlighting how people across the industry are solving big business challenges by leveraging Hadoop. I am posting here the slides with an approximate transcript of my talk.Ever since I studied Machine Learning and Data Mining at Stanford 3 years ago., I have been enamored by the idea that it is now possible to write programs that can sift through TBS of data to recommend useful things.So here I am with my colleague Adil Aijaz, for a talk on some of the lessons we learnt and challenges we faced in building large-scale recommender systemAt LinkedIn we believe in building platform, not verticals. Our talk is divided into 2 parts. In the first part of this talk, I will talk about our motivation for building the recommendation platform, followed by a discussion on how we do recommendations. No analytics platform is complete without Hadoop. So, in he next part of our talk, Adil will talk about leveraging Hadoop for scaling our products.‘Think Platform, Leverage Hadoop’ is our core message.Throughout our talk, we will provide examples that highlight how these two ideas have helped us ‘scale innovation’.With north of 135 million members, we’re making great strides toward our mission of connecting the world’s professionals to make them more productive and successful. For us this not only means helping people to find their dream jobs, but also enabling them to be great at the jobs they’re already in.With terabytes of data flowing through our systems, generated from member’s profile, their connections and their activity on LinkedIn, we have amassed rich and structured data of one of the most influential, affluent and highly-educated audience on the web.This huge semi-structured data is getting updated in real-time and growing at a tremendous pace, we are all very excited about the data opportunity at LinkedIn.For an average user, there is so much data, there is no way users can leverage all the data on their own.We need to put the right information, to the right user at the right time.With such rich data of members, jobs, groups, news, companies, schools, discussions and events. We do all kinds of recommendations in a relevant and engaging way.We have products like‘Job Recommendation’: here using profile data we suggest top top jobs that our member might be interested in. The idea is to let our users be aware of the possibilities out there for them.‘Talent Match’: When recruiters post jobs, we in real-time suggest top candidates for the job.‘News Recommendation’: Using articles shared per industry, we suggest top news that our users need to keep them updated with the latest happenings.‘Companies You May Want to Follow’: Using a combination of content matching and collaborative filtering, we recommend companies a user might be interested in keeping up-to date with.‘PYMK’: based on common attributes like connections, schools, companies and some activity based features, we suggest people that you may know outside of LinkedIn and may want to connect with at LinkedIn.‘Similar Profiles’: Finally our latest offering which we released a few months ago for recruiters, Similar Profiles. Given a candidate profile, we suggest top Similar candidates for hiring based on overall background, experience and skills.We have recommendation solutions for everyone, for individuals, recruiters and advertisersIn our view, recommendations are ubiquitous and they permeate the whole site.Before we discuss our motivation for building a recommendation platform or a discussion on how we do recommendation or how we leverage Hadoop. Let’s first answer a basic question: Are recommendations really important?To put things in perspective, 50% of total job applications and job views by members are a direct result of recommendations. Interestingly, in the past year and half it has risen from 6% to 50%.This kind of contribution is observed across all our recommendation products and is growing by the day.Let us start with an example of the kind of data we have.For a member, we have positions, education, Summary, Specialty, Experience and skills of the user from the profile itself. Then, from member’s activity we have data about members connections, the groups that the member has joined, the company that the member follows amongst others.Before we can start leveraging data for recommendation, we first need to clean and canonicalize it.Lets take an example for matching member to jobs,In order to accurately match members to jobs, we need to understand that all the ways of listing these titles refer to the same entity ‘Software Engineer’‘Technical Yahoo’ is a Software Engineer at Yahoo‘Member Technical Staff’ is a Software Engineer at Oracle‘Software Development Engineer’ is a Software Engineer at Microsoft‘SDE’ is a Software Engineer at AmazonSolving this problem is itself a research topic broadly referred to as ‘Entity Resolution’.As another example, How many variations do you think, we have for the company name ‘IBM’?When I joined LinkedIn, I was surprised to find that we had close to 8000+ user entered variations of the same company IBM.We apply machine learnt classifiers for the entity resolution using a host of features for company standardization.In summary, data canonicalization is the key to accurate matching and is one of the most challenging aspects of our recommendation platform.Now, we will discuss our motivation behind building a common platform by way of 3 key example trade-offs that we’ve encountered. In LinkedIn ecosystem, one trade-off that we encountered is that of real-time Vs time independent recommendations.Lets look at ‘News Recommendation’, which finds relevant news for our users. Relevant news today might be old news tomorrow. Hence, News recommendation has to have a strong real-time component.On the other hand, we have another product called ‘Similar Profiles’. The motivation here is that if a hiring manager, already know the kind of person that he wants to hire. He could be like person in his team already or like one of his connections on LinkedIn, then using that as the source profile, we suggest top Similar Profiles for hiring. Since, ‘people don’t reinvent themselves everyday’. ‘People similar today are most likely similar today’. So, we can potentially do this computation completely offline with a more sophisticated model.These are the 2 extreme cases in terms of ‘freshness’: Most examples fall into an intermediate category. For E.g. new jobs gets posted by the hour and they expire when they get filled up. All jobs posted today don’t expire the same day. Hence, we cache job recommendation for members for some time as it is OK to not recommend the absolute latest jobs instantly to all members.In solving the completely real-time Vs completely offline problem, we could have gone down the route of creating separate solutions optimized for the use-case. In the short run, that would have been a quicker solution.But we went down the platform route because we realized that we would churn out more and more such verticals as LinkedIn grows. Now, as a result of which we have the same code that computes recommendations online as well as offline. Moreover, in the production system caching and an expiry policy allows us to keep recommendations fresh irrespective of how we compute the recommendations. Now, as a result for newer verticals. We can easily get ‘freshness’ of recommendations irrespective of whether we compute recommendations online or offline.Another interesting trade-off, is choosing between content analysis and collaborative filtering.Historically speaking: ‘job posting has been a post-and-prey’ model. Where job posters post a job and pray that hopefully someone will apply for the job. But we at LinkedIn, believe in ‘post-and-produce’ so we go ahead and produce matches to the job poster in real-time right after the job gets posted. When someone posts a job, the job poster naturally expects the candidates to have a strong match between job and the profile. Hence, this type of recommendation is heavy on content analysis.On the other hand, we have a product called ‘Viewers of the profile also viewed ..’. When a member views more than 1 profile within a single session. We record it as a co-view. Then on aggregating these co-views for every member. We get the data for all profiles that get co-viewed, when someone visits any given profile. This is a classical collaborative filtering based recommendation much like Amaozon’s ‘people who viewed this item also viewed’Most other recommendations are hybrid. For e.g. for ‘SimilarJobs’ jobs that have high content overlap with each other are similar. Interestingly, jobs that get applied to or viewed by the same members are also Similar. So, Similar Jobs is a nice mix of content and collaborative filtering.Again, Because of a platform approach, we can re-use the content matching and the collaborative filtering components to come up with newer verticals without re-inventing the wheel.Finally, the last key trade-off is of precision vs recall.On our homepage, we suggest jobs that are good fit for our users with the motivation for them to be more aware of the possibilities out there. In some sense, we are pushing recommendations to you, as opposed to you actively looking for them.Even if a single job recommendation looks bad to the user either because of a lower seniority of the job or because the recommendation is for the company that the user is not fond of, our users might feel less than pleased.Here, getting the absolute best 3 jobs even at the cost of aggressively filtering out a lot of jobs is acceptable.On the other hand, We have another recommendation product called ‘Similar Profiles’ for hiring managers who are actively looking for candidates. Here, if one finds a candidate we suggest other candidates like the original one in terms of overall experience, specialty, education background and a host of other features.Since, the hiring manager is actively looking so in this case they are more open to getting a few bad ones as long as they get a lot of good ones too. So in essence, recall is more important here.Again, because of a platform approach and because we can re-use features, filters and code-base across verticals. So, tuning the knob of more precision vs more recall is mostly a matter of figuring out 1. how complicated the matching model should be and 2. how aggressively we want to apply filters for all recommendation verticals. Hence, our core message ‘Think Platform’. Now, we will discuss in some detail how our recommendations work.Lets see how we do recommendations by taking an example of ‘Similar Profiles’ that we just discussed. Given a member profile, the goal is to find other similar people for hiring. Lets try to find profiles similar to me.Here, we look at host of different features, such as1. User provided features like ‘Title, Specialty, Education, experience amongst others’2. Complex derived features like ‘Seniority’ and ‘Skills’, computed using machine learnt classifiers.3. Both these kinds of features help in precision, we also have features like ‘Related Titles’ and ‘Related Companies’ that help increase the recall.Intuitively, one might imagine that we use the following pair of features to compute Similar Profiles. In the next slide, we will discuss a more principled approach to figuring out pair of features to match against.Here in order to compute overall of similarity between me and Adil, we are first computing similarity between our specialties, our skills, our titles and other attribute.With this we get a ‘Similarity score vector’ for how Similar Adil is to me, Similarly we can get such a vector for other profiles.Now we somehow need to combine the similarity score in the vector to a single number s.t. profiles that have higher similarity score across more dimensions get ranked higher, as similar profiles for me.Moreover, the fact that our skills match might matter more for hiring than whether our education matches. Hence, there should be relative importance of one feature over the others.Once we get the topK recommendations, we also apply application specific filtering with the goal of leveraging domain knowledgeFor example, it could be the case that for a ‘Data Engineer role’ you as a hiring manager are looking for a candidate like one of your team member but who is local. Whereas, for all you know the ideal ‘Data Engineer most similar to the one you are looking for in terms of skills might be working somewhere in INDIA’ .To ensure our recommendation quality keeps improving as more and more people use our products, we use explicit and implicit user feedback combined with crowd-sourcing, to construct high quality training and test sets for constructing the ‘Importance weight vector’. Moreover, classifier with L1 regularization helps prune out the weakly correlated features. We use this for figuring out which features to match profiles against.We just discussed an example. However, the same concepts apply to all the recommendation verticals.And now the technologies that drives it all.The core our matching algorithm uses Lucene with our custom query implementation.We use Hadoop to scale our platform. It serves a variety of needs from computing Collaborative filtering features, building Lucene indices offline, doing quality analysis of recommendation and host of other exciting things that Adil will talk about in a bit.Lucene does not provide fast real-time indexing. To keep our indices up-to date, we use a real-time indexing library on top of Lucene called Zoie.We provide facets to our members for drilling down and exploring recommendation results. This is made possible by a Faceting Search library called Bobo.For storing features and for caching recommendation results, we use a key-value store Voldemort.For analyzing tracking and reporting data, we use a distributed messaging system called Kafka.Out of these Bobo, Zoie, Voldemort and Kafka are developed at LinkedIn and are open sourced. In fact, Kafka is an apache incubator project.Historically, we have used R for model training. We have recently started experimenting with Mahout for model training and are excited about it.All the above technologies, combined with great engineers powers LinkedIn’s Recommendation platform.Now Adil will talk about how we leverage Hadoop.In the second half of our talk we will present case studies on how Hadoop has helped us scale innovation for our Recommendation Platform. We will use the ‘Similar Profiles’ vertical which was discussed earlier as the example for each case study. As a quick reminder, similar profiles recommends profiles that are similar to the one a user is interested in. Some of its biggest customers are hiring managers and recruiters. For each of the case studies, we will lay out the solutions we tried before turning to Hadoop, analyze the pros and cons of the approaches before and after Hadoop, and finally derive some lessons that are applicable to folks working on large scale recommendation systems.When it comes to recommendations, relevance is the most important consideration. However, with over 120M members, and billions of recommendation computations, the latency of our recommendations becomes equally important. No mater how great our recommendations are, they wont be of utility to our members if we take too long to return recommendations. Among our many products, ‘Similar Profiles’ is a particularly challenging product to speed up. Our plain vanilla solution involved using a large number of features to mine the entire member index for the best matches. The latency of this solution was in the order of seconds. Clearly, no matter how relevant our recommendations were, with that type of latency, our members would not even wait for the results. So, something had to be done. We needed a solution that could pre-filter most of the irrelevant results while maintaining a high precision on the documents that survived the filter. One technique that meets these conditions is minhashing. On a very high level, minhashing involves running each document, in our case member profiles, through k hash functions to to construct a bit vector. One can play with ANDING/Oring of subsets of the bit vector, to get the right balance between recall and precision. As our second pass solution, we minhashed each document and stored the resulting bit array in the member index. At query time, we minhashed the query into a bit array, filtered out documents that did not have the exact same subsets of the bit array, and finally did advanced matching on documents that survived the filtration. This solution brought down the latency well below a second, however, minhashing did not give us the recall we had hoped for. This was a really disappointing result since we had spent significant engineering resources in productionalizing minhashing, yet it was all for nought.So, we went back to the drawing board and started thinking about how we can use Hadoop to solve this problem. The key breakthrough was when we realized that people do not reinvent themselves everyday. The folks I was similar to yesterday, are likely to be the same folks I am similar to today. This meant that we could serve ‘similar profiles’ recommendations from cache. When the cache would expire, we could compute fresh recommendations online and repopulate the cache. This meant that the user would almost always be served from cache. Great: but we still have to populate the cache somehow. This is where Hadoop comes into the picture. By opening an index shard per mapper, we can generate a portion of the recommendations in each mapper, and combine them into a final recommendation set in the reducers. With the distributed computation of Hadoop, we easily generate similar profiles for each member, and then copy over the results to online caches. So the three elements of:a) Offline batch computations on Hadoop copied to online caches.b) Aggressive online caching policyc) Online computation when the cache expiresHave scaled our similar profiles recommendations while maintaining a high precision of recommendations.So, the key takeaway from this case study of scaling is that if one is facing the problem of:a) High latency computationb )High qpsc) And not so stringent freshness requirementsThen one should leverage Hadoop and caching to scale the recommendation computation.With our scaling problems solved, we rolled out Similar Profiles to our members. The reception was amazing. However, we felt that we could do even better by going beyond content based features alone. One such feature that we wanted to experiment with was collaborative filtering. More specifically, if a member browses multiple member profiles within a single session, aka coviews, it is quite likely that those member profiles are very similar to each other. How we blend collaborative filtering with existing content based recommendations is the subject of our second case study: “blending multiple recommendation algorithms”.Our basic blending solution is this: While constructing the query for content based similar profiles, we fetch collaborative filtering recommendations and their scores, and attach them to the query. In the scoring of content based recommendations, we can use the collaborative filtering score as a boost. An alternative approach is a bag of models approach with content and collaborative filtering serving as two of the models in the bag.In either solution, we need a way to keep collaborative filtering results fresh. If two member profiles were coviewed yesterday, we should be able to use that knowledge today.We first sketched out a completely online solution. This online solution involved keeping track of the state of each member session, accumulating all the profile views within that session. At the end of each session, we updated the counts of the various coview pairs. As you can appreciate, such a stateful solution can get very complicated very quickly. As an example, we have to worry about machine failures, multi data center coordination just to name two challenges. In essence such a solution could introduce more problems than it solves. So, we scratched this solution even before implementing it.We thought more about this problem and realized two important aspects: 1) Coview counts can be updated in batch mode. 2) We can tolerate delay in updating our collaborative filtering results.These two properties, batch computation and tolerance for delay in impacting the online world, led us to leverage Hadoop to solve this problem. Our production servers can produce tracking events everytime a member profile is viewed. These tracking events are copied over to hdfs where everyday, we use these tracking events to batch compute a fresh set of collaborative filtering recommendations. These recommendations are then copied to online key value stores where we use the blending approaches outlined earlier to blend collaborative filtering and content based recommendations.Compared to the purely online solution, the Hadoop solution is simpler in complexity, less error prone, but introduces a lag between the time two profiles are coviewed and the time that coview has an impact on similar profiles. For us, this solution works great. The other great thing about this solution is that it can be easily extended to blend social or globally popular recommendations in addition to collaborative filtering.The lesson we derive from this case study is that by leveraging Hadoop, we were able to experiment with collaborative filtering in similar profiles without significant investment in an online system to keep collaborative filtering results fresh. Once our proof of concept was successful, we could always go back and see if reducing the lag between a profile coview and its impact on similar profile by building an online system would be useful. If it is, we could invest in a non-Hadoop system. However, by leveraging Hadoop, we were able to defer that decision till the point when we had data to backup our assumptions.A consistent feedback from hiring managers using Similar Profiles was that while the recommendations were highly relevant, often times the recommended members were not ready to take the next steps in their professional career. Such recommended members would thus respond negatively to a contact from the hiring manager, leading to a bad experience for the hiring manager. This feedback indicated a strong preference from our users that they would like a tradeoff between relevance of recommendations and the responsiveness of those recommendations. One can imagine a similar scenario playing out for a sales person looking for recommendations for potential clients.As our next case study, let’s take a look at how we approached solving this problem for our users. Let’s say we come up with an algorithm that assigns each LinkedIn member a ‘JobSeeker’ score which indicates how open is she to taking the next step in her career. As we said already, this feature would be very useful for ‘Similar Profiles’. However, the utility of this feature would be directly related to how many members have this score: aka coverage. The key challenge we faced was that since ‘Similar Profiles’ was already in production, we had to add this new feature while continuing to serve recommendations. We call this problem “grandfathering”.A naïve solution, could be to assign a ‘jobseeker’ score to a member next time she updates her profile. This approach will have minimal impact on the system serving traffic, however, we will not have all members tagged with such a score for a very long time, which impacts the utility of this feature for ‘Similar Profiles’.So, we scratch the naïve solution and look for a solution that will batch update all members with this score in all data centers while serving traffic.A second pass solution is to run a ‘batch’ feature extraction pipeline in parallel to the production feature extraction pipeline. This batch pipeline will query the db for all members and add a ‘job seeker score’ to every member. This solution ensures that we have an upper bound on the time it takes to grandfather all members with job seeker score. It will work great for small startups whose member base is in a few million range.However, the downside of this solution at LinkedIn scale is:1) It adds load on the production databases serving live traffic.2) To avoid the load, we end up throttling the batch solution which in turn makes the batch pipeline run for days or weeks. This slows down rate of batch update.3) Lastly, the two factors above combine to make grandfathering a ‘dreaded word’. You only end up grandfathering ‘once a quarter’ which is clearly not helpful in innovating faster.So, we clearly cannot use that solution either. However, one good aspect of this solution is the batch update which leads us into a Hadoop based solution.Using Hadoop, we take a snapshot of member profiles in production, move it to hdfs, grandfather members with a ‘job seeker score’ in a matter of hours, and copy this data back online. This way we can grandfather all members with a job seeker score in hours.The biggest advantage of using Hadoop here is that grandfathering is no longer a ‘dreaded word’. We can grandfather when ready instead of grandfather every quarter which speeds up innovation.So, in a nutshell, if one finds oneself slowed down due to constraints of updating features in the online world, consider batch updating the features offline using Hadoop and swapping them out online.With the first few versions of similar profiles out the door, we began to simultaneously investigate a number of avenues for improvement. Some of us investigated different model families, say logistic regression vs SVM, others investigated new features with the existing model. In this case study, we will talk about how we decided which one of these experiments would actually improve the online relevance of similar profiles so we could double down on getting them out to production. We are not concerned with ‘how’ we come up with these new models. For all that matters, we hand tuned a common sense model. The question is how to decide whether or not to move that new model to production.Now, as a base solution, we can always move every model to production. We can A/B test the model with real traffic and see which models sink and which ones float. The simplicity of this approach is very attractive, however, there are some major flaws with it:1) For each models we have to push code to production. This takes up valuable development resources.2) There is an upper limit on the number of A/B tests one can run at the same time. This can be due to user experience concerns and/or revenue concerns.3) Since online tests need to run for days before enough data is accumulated to make a call, this approach slows down rate of progress.Ideally, we would like to try all our ideas offline, evaluate them, and only push to production the best ones. Hadoop proves critical in the evaluation step. Overtime, using implicit and explicit feedback combined with crowdsourcing, we have accumulated a huge gold test set for ‘similar profiles’. We rank the gold set with each model on Hadoop, and use standard ranking metrics to evaluate which one performs best.As you can guess, Hadoop provides a very good sandbox for our ideas. We are able to filter many of the craziest ideas, and double down on only those few that show promise. Plus, it allows us to have relatively large gold sets which gives us strong confidence in our evaluation results.Now that we have learned a new model for ‘Similar Profiles’ that performs well in our offline evaluation framework, we need to test it online. An industry standard approach to this problem is known as A/B testing or bucket testing. Formally, AB testing involves partitioning real traffic between alternatives and then evaluating which alternative maximizes the desired objective. Typical desired objectives are CTR, revenue, or number of views.The key requirements of AB testing is that: time to evaluate which bucket to send traffic to should ideally be < 1ms, at-worst a few ms.Let’s discuss how we would do A/B testing for our new model. For simple partitioning requirements, one can use a mod-based scheme. This is very fast and very simple and can satisfy most use cases. However, if one wishes to partition traffic based on profile and member activity criteria for e.g. “Send 10% of members who have greater than 100 connections AND who have logged in the last one week AND who are based in Europe” then doing this online is too expensive. Keep in mind that deciding which bucket to send traffic to should be very fast, ideally less than a millisecond. In worst case scenario a few milliseconds. I am not going to even attempt an online solution for this problem.So, we go straight to Hadoop. For complex criteria like this, we run over our entire member base on Hadoop every couple of hours, assigning them to the appropriate bucket for each test. The results of this computation are pushed online, where the problem of A/B testing reduces to given a member and a test, fetching which bucket to send the traffic to from cache.The take home message here is: If you need complex targeting and A/B testing, leverage Hadoop.Our last case study involves the last step of a model deployment process: tracking and reporting. These two steps allow us to have an unbiased, data-driven way of saying whether or not a new model is successful in lifting our desired metrics: ctr or revenue or engagement or whatever else one is interested in. Our production servers generate tracking events every time a recommendation is impressed, clicked or rejected by the member.Before Hadoop, we used to have an online reporting tool that would listen to tracking events over a moving window of time, doing in-memory joins of different streams of events and reporting up-to-the minute performance of our models. The clear advantages of this approach were that we could see exactly how the model was performing online at this moment. However, there are a few downsides such asa) One cannot look at greater than certain amount of time window in the past..b) As the number of tracking streams increases, it becomes harder and harder to join them online.To increase the time window, we will have to spend significant engineering resources in architecting a scalable reporting system which would be an overkill. Instead we placed our bet on Hadoop. All tracking events at LinkedIn are stored on HDFS. Add to this data Pig or plain Map-Reduce, we can do arbitrary k-way joins across billions of rows to come up with reports that can look as far in the past as we want to.The advantages of this approach are quite clear. Complex joins are easy to compute and reporting is flexible on time windows. However, we cannot have up-to-the minute reports since we copy over tracking events in batch to hdfs. If we ever need that level of reporting, we can always use our online solution.We can say without any hesitation that Hadoop has now become an integral part of the whole life-cycle of our workflow starting from prototyping a new idea to eventually tracking the impact of that idea.By thinking about platform and not verticals, we are able to to come with newer verticals at a fast pace.By leveraging Hadoop, we were able to continuously improve the quality and scale the computations.Hence, these 2 ideas helped us ‘scale innovation’ at Linkedin.To conclude, we want to say that the data opportunity at LinkedIn is HUGE and so come work with us at LinkedIn!
- Home >
- Catalog >
- Life >
- Log Template >
- Activity Log Template >
- daily activity log template pdf >
- We Are Very Excited To Be Able To Offer Another Great Program And We Hope We Have