How to Edit The Job Evaluation Scheme For Rank To Role with ease Online
Start on editing, signing and sharing your Job Evaluation Scheme For Rank To Role online under the guide of these easy steps:
- Click on the Get Form or Get Form Now button on the current page to access the PDF editor.
- Give it a little time before the Job Evaluation Scheme For Rank To Role is loaded
- Use the tools in the top toolbar to edit the file, and the edited content will be saved automatically
- Download your edited file.
The best-reviewed Tool to Edit and Sign the Job Evaluation Scheme For Rank To Role
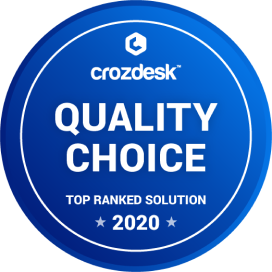
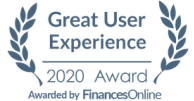
A simple tutorial on editing Job Evaluation Scheme For Rank To Role Online
It has become very easy lately to edit your PDF files online, and CocoDoc is the best online tool you would like to use to do some editing to your file and save it. Follow our simple tutorial to start!
- Click the Get Form or Get Form Now button on the current page to start modifying your PDF
- Create or modify your content using the editing tools on the top tool pane.
- Affter changing your content, put on the date and add a signature to complete it.
- Go over it agian your form before you save and download it
How to add a signature on your Job Evaluation Scheme For Rank To Role
Though most people are accustomed to signing paper documents by writing, electronic signatures are becoming more regular, follow these steps to sign documents online!
- Click the Get Form or Get Form Now button to begin editing on Job Evaluation Scheme For Rank To Role in CocoDoc PDF editor.
- Click on Sign in the tool menu on the top
- A popup will open, click Add new signature button and you'll have three choices—Type, Draw, and Upload. Once you're done, click the Save button.
- Drag, resize and position the signature inside your PDF file
How to add a textbox on your Job Evaluation Scheme For Rank To Role
If you have the need to add a text box on your PDF and create your special content, do some easy steps to accomplish it.
- Open the PDF file in CocoDoc PDF editor.
- Click Text Box on the top toolbar and move your mouse to drag it wherever you want to put it.
- Write down the text you need to insert. After you’ve inserted the text, you can take use of the text editing tools to resize, color or bold the text.
- When you're done, click OK to save it. If you’re not satisfied with the text, click on the trash can icon to delete it and begin over.
A simple guide to Edit Your Job Evaluation Scheme For Rank To Role on G Suite
If you are finding a solution for PDF editing on G suite, CocoDoc PDF editor is a suggested tool that can be used directly from Google Drive to create or edit files.
- Find CocoDoc PDF editor and install the add-on for google drive.
- Right-click on a PDF file in your Google Drive and select Open With.
- Select CocoDoc PDF on the popup list to open your file with and allow CocoDoc to access your google account.
- Edit PDF documents, adding text, images, editing existing text, mark with highlight, give it a good polish in CocoDoc PDF editor before hitting the Download button.
PDF Editor FAQ
Should I work under a QMAS visa in Hong Kong?
Hong Kong QMAS VisaHong Kong is offering permanent citizenship to foreign nationals who are skilled and willing to contribute to the local economy of the country. Hong Kong QMAS Visa is one such provision for candidates planning to immigrate to the Hong Kong Special Administrative Region (HKSAR) of the People’s Republic of China (PRC).IntroductionHong Kong QMAS Visa is a “quota based” entrance scheme for talented foreign nationals who opt to settle in Hong Kong. QMAS is an abbreviation for Quality Migrant Admission Scheme. In this migration process, it is not mandatory for the candidate to possess an employment offer from a local Hong Kong employer but there are certain prerequisites and the applicant must fulfill them all before applying for immigration to Hong Kong with the QMAS scheme.Each Candidate fills his/her application for either of the two tests under the Hong Kong QMAS Visa Application process – General Points Test and Achievement-Based Points Test. The candidate is then awarded points based on his/her personal details of education, work experience, and his/her caliber to be a catalyst to the economic growth of the nation. Based on these points, each candidate then competes with the other applicants for quota allocation.A candidate can only submit an application for one test at a time. Also, Hong Kong QMAS Visa scheme is not applicable to citizens of Cuba, Laos, Nepal, Vietnam, Korea, and Afghanistan.PrerequisitesThere are certain requirements for eligibility to QMAS Visa application, please read the following points for detailed information:1. Age – The candidate must be at least 18 years old at the time of filing the application under the Hong Kong QMAS Visa scheme2. Financial Stability – The applicant must be able to showcase the financial capabilities of supporting himself/herself and dependants, if any, without any public assistance from the Hong Kong government during their stay.3. Character Certificate – There must not be a criminal history of the candidate anywhere in the World. Also, during the candidate’s background check, there must not be any adverse immigration record within Hong Kong or anywhere else around the globe as well.4. Language Proficiency – As an important requirement of QMAS, the candidate must be expressive in both - written and spoken form of Chinese English (Putonghua or Cantonese)5. Educational Qualification – To be eligible to apply for QMAS, the applicant must possess a first class degree from any recognized university or a territorial educational institution. In a few rare cases, some exceptions are also entertained, like candidates with extraordinary technical qualifications or proven work capabilities, past job experience and/or achievements are considered to be eligible to apply the candidate can showcase proper documents to support the facts. In the lack of supporting documents, the candidate’s application may not be processed further and he/she may immediately be sent a refusal for immigration to Hong Kong.Rules for DependentsIf a candidate gets selected for immigration to Hong Kong, he/she can bring along their spouse or unmarried children below 18 years of age to reside with them in Hong Kong itself. This is allowed only if the candidate is able to demonstrate that he/she has enough financial resources to support his/her dependent’s stay in Hong Kong without asking or looking up to the Hong Kong government for any public assistance. All dependents will thus be sponsored by the applicant and their duration of stay shall be linked to the applicants only.As accepted by law, the spouse of the applying candidate can be the second party either in a same-sex civil union or opposite-sex civil union arrangement. Even candidate’s partners from same-sex civil partnership or opposite-sex civil partnership are considered legal spouses. Candidates can also bring along partners from “same-sex marriage”, or a marriage as performed by the candidate in accordance with the local law procedures of the place of marriage and/or the place of celebration and with the status that is legally and officially recognized by the local authorities of the place of marriage and/or the place of celebration.To avoid any doubts and confusions, the terms “civil partnership” and “civil union” have been used in reference to clearly mention to a legal institution of a characteristic equivalent to the wedded or spousal relationship as in the case in a marriage.The applicant must submit all dependent’s visa applications along with their own entry permit applications. If the applicant is benefiting and gaining additional points on the basis of educational qualifications of the accompanying spouse and/or unmarried children under 18 years of age for the General Points Test, the applicant must submit their applications along with his/her own visa application itself.Selection ProcessOnce an applicant meets all prerequisites, he/she is eligible and may appear for either of the two tests – General Points Test or Achievement-based Points Test, based on their qualifications and skill set.The General Points Test has a six-point scoring factor whereas the Achievement-based Points Test has one-point scoring factor. There is a minimum passing mark requirement for both the tests. However, the candidates who choose to appear for the Achievement-based test are advised to re-evaluate the marking scheme to ensure they shall be able to achieve the bare minimum marks before appearing for the exam.The selection process for quota allotment to candidates for both the tests is done a regular basis. During the selection process, the candidates who fulfill the prerequisites and have scored the desired minimum marks are ranked on the basis of their scores. The highest scoring applications are shortlisted for further assessment. At this stage, the Director of Immigration may consult the Advisory Committee, formed by official and non-official members appointed by the Chief Executive of HKSAR and discuss the final selection of talented and skilled candidates for immigration to Hong Kong.The Advisory Committee considers varied factors like the social and economic requirements of Hong Kong, the amalgam of candidates based on education and sectors, etc and then recommends candidates to the Director of Immigration to have the best allocation of the quota to candidates in each selection process. Thus, merely meeting the prerequisites or scoring the minimum passing score does not guarantee a quota allotment to the candidate.The Director of Immigration is free to award points to candidates in both the tests as per his will. He has total discretion to approve or refuse an application without giving any prior reason. This scheme is subject to change without any prior notice as and when the Director feels the need to change.The results from each selection process clearly mentioning the candidate names that have been selected are published on the Immigration Department’s website. An application may seem to be under process until it either gets approved or receives refusal from the Immigration department.All applicants who get selected and are allotted a quota in the selection process receive an approval-in-principle letter. This letter does not still guarantee approval of the candidate for immigration to Hong Kong under the QMAS Scheme.The applicants who receive the letter are then asked to visit Hong Kong for an in-person interview and for verification of all documents that they had submitted during the application process. The candidates may visit Hong Kong on a visitor visa for this purpose. Once the documents are verified and the applicant fares well in the interview, he/she is then issued a Visa or entry permit under the Hong Kong QMAS Visa Scheme.General Points TestFollowing is the marking scheme of the General Points Test· Age - Maximum 30 pointso Between 18 – 39 years – 30 pointso Between 40 – 44 years – 20 pointso Between 45 – 50 years – 15 pointso Over 51 years – 0 points· Academic Qualifications – Maximum 70 pointso Doctoral Degree, or two or more Master’s Degree – 40 pointso Master’s Degree, or two or more Bachelor’s Degree – 20 pointso Bachelor’s Degree or any Professional Qualification awarded by an internationally or nationally recognized professional body which showcases that the holder has an exceptional level of technical talent, expertise or skill – 10 pointso Additional points if the Bachelor’s, Master’s or Doctoral Degree is awarded by an internationally renowned institution – 30 points· Job Experience – Maximum 55 pointso Minimum 10 years’ of graduate or specialist level job experience which also includes at least 5 years of work experience in a senior role – 40 pointso Minimum 5 years’ of graduate or specialist level job experience which also includes at least 2 years of work experience in a senior role – 30 pointso Minimum 5 years’ of graduate or specialist level job experience – 15 pointso Minimum 2 years’ of graduate or specialist level job experience – 5 pointso Additional points for a minimum of 2 years’ graduate or specialist level job experience with international work exposure – 15 points· Skill / Talent List – Maximum 30 pointso Additional points if the candidate meets the specifications of his/her respective profession in the talent list· Language Proficiency – Maximum 20 Pointso Proficient in both written and spoken English and Chinese (Putonghua or Cantonese) – 20 pointso Proficient in at least one foreign language (spoken and written) along with proficiency in written and spoken Chinese (Putonghua or Cantonese) or English – 15 pointso Proficient in written and spoken Chinese (Putonghua or Cantonese) or English – 10 points· Family Background – Maximum 20 pointso Anyone immediate family member is a Hong Kong permanent resident also currently residing in Hong Kong. An immediate family member can be a married spouse, parents, siblings, or children of the applicant – 5 pointso Dependent married spouse is qualified to the equivalent level of a degree or more – 5 pointso 5 points for each unmarried dependent child below the age of 18, maximum 10 points – 5 / 10 pointsThe minimum passing points for the General Points Test is 60. Each candidate must assess their personal details to see if their points tally is above the minimum passing score before submitting the application. Please note, the passing points are subject to change without any prior notice.Achievement-based Points TestA candidate with exceptional talent or skill set may opt to fill the application under the Achievement-bases Points Test and be assessed separately on the basis of his or her achievements. The requirements and skill expectations for candidates is very high under this test.A candidate who meets either of the criteria below is awarded 225 points:The candidate has been recognized with an award of exceptional achievement (For instance – Olympic medals, Nobel prize, national or international awards); orThe candidate is able to showcase that his/her contribution to work has been acknowledged by his/her co-workers or if he/she has made significant contributions to growth and development in his/her career field (for instance - a lifetime achievement award from industry).An application that fails to meet either of the criteria below is refused immediately.
What are your thoughts about Imran Khan as Pakistan's prime minister?
Thank you for the A2A Koyel Bandyopadhyay and Surabhi Kanth(सुरभि कंठ) and apologies for the late reply.I’ll try to maintain a more upbeat and positive tone in this answer compared to my usual gloomy, pessimism .The fallacy of change.A lot of people call Imran Khan the “Donald Trump” of Pakistan because of similarities in his play boy, celebrity lifestyle with multiple marriages and it’s similarities to Trump. His aggressive politics, his upstart nature, his fans.Such comparisons are fraught with errors.Imran Khan is a product of the establishment, bought in to reign in the out of control PPP and PML-N that were increasingly becoming hostile to working with the Deep state elements. Trump on the other hand, bust his way into the center of American politics with his brash, populist politics and has for a large part upset the established order of American politics.Imran Khan has largely co-opted the strong ecological political traditions of his KPK home province that were rooted in North Western Marxist movements long before Imran Khan was even born. Trump on the other hand has shotgun blasted his way past any ecological agreement like Climate Change agreements and oil pipeline regulations.Imran Khan can broadly be categorized as Center-Right and belongs to an ethnic group that holds 2nd place in the corridors of Pakistani power politics. Trump comes from the privileged White majority class.And so on.Imran Khan has more in common with Trump’s predecessor: Obama. And the promise of a better tomorrow is laced with the dangers of a dark future.Pakistan’s Trump has not been elected yet and is waiting in the shadows of a potentially disenchanted electorate that will punish Imran Khan if he fails to deliver. And that Trump twin is none other than the Tehreek-e-Labaik Chief, Maulana Khadim Rizvi who has made a major breakthrough for his party in the urban landscape of Pakistan’s largest cities like Lahore and Karachi.The same way Obama’s deadlocked, centrist politics pleased none and empowered a far right backlash, Imran Khan’s tremolos grip on power with barely enough seats to form a majority on his own and unreliable coalition partners will have to do a lot to allay Pakistan’s current perils.The only thing they have going for them is the support of the Deep State establishment which paved the way for their electoral victory not through outright rigging but indirect support that boosted the party’s prospects.Even now, as the new government is formed, the unelected establishment views the tumbling rupee, the CPEC delays and other economic perils with growing anxiety. Imran is expected to deliver harsh technocratic remedies to address this economic decline so ‘the boys’ can keep getting their toys.Such is the way of Pakistani politics since time immemorial. The Superstructure of the Pakistani state that was left behind as the colonial apparatus of the British has never sought direct rule over the nation. When Yahya deposed Ayub Khan or Musharraf deposed Nawaz Sharif, the new coup-president’s first priorities were to always hold elections to garner a level of political legitimacy through allies in the elected assemblies.The reasons for this are simple: Pakistan is too ethnically diverse and politically fissured to rule through an iron fist. We are called the “Negotiated state” for a reason. We negotiated our way to independence and creation, with the British and Congress at first and between the provinces later as we developed into a state.While the Indian analysis always paints the Army as secretly ruling Pakistan, Pakistan has in truth, never been ruled by any single entity no matter what is its power.Imagine an India that comprised only Punjab, Kerala, Bengal, UP and Kashmir and you would get a hint of how diverse our nation is politically.The best case in point to illustrate this fact is the Kalabagh dam issue, where a military dictator at the height of his power could not gather enough consensus to build a single Dam. Did tanks roll down the Sindh Assembly or Paratroopers fly over KPK to enforce the issue? Nope. The military understands the limits of its powers.And thus, the nexus between deep state bureaucrats, judges, generals and allied politicians has always carefully balanced power among each other to ensure the status quo remains undisturbed against the indigenous bourgeoisie of the outer provinces that could threaten the power of the center.Imran Khan is no other than a new ally for the establishment to utilize to exercise control of the state with the fig leaf of legitimacy to cover up it’s naked involvement in political affairs.But then again, herein lies the cultural difference between Pakistan and other states: It is acceptable for the army to intervene in state affairs as far as the populace is concerned. Particularly the middle class, urban electorate which itself is what the army, judiciary and bureaucracy draws it’s ranks from.The structural issues of Pakistan and Imran’s hopelessness against them.Pakistan’s state structure has it’s roots in the last decades of the Mughal empire and the British colonial state that replaced it.The idea of a tributary system where networks of patronage extended the long arm of the Delhi sultanates over the distant hinterland are older than any living Pakistani today.Pakistan is quite libertarian in it’s attitude of what the role of the state is. Local clans and baradaries expect their chosen district leader to operate in the same way the Naibs and Nazims of the colonial and pre-colonial rule operated. Tribute and loyalties flowed to the center in exchange military protection and resource extraction rights.When the British replaced the Mughals, the colonial extraction state was perfected into the most efficient resource extraction mechanism modern civilization had produced so far. Local leaders pledged loyalty to the British in exchange for keeping their titles and lands and were protected by the British Indian army. Meanwhile, the Colonial bureaucratic apparatus extracted local resources in coordination with said local rulers and maintained the market for British products in the colony.The only threat against the entire colonial state was the development of a local political bourgeoisie who wanted more autonomy. Gandhi, Jinnah and Nehru fall under these. Parallel to these were the rise of revolutionary figureheads who wanted the complete expulsion of the British colonial extraction state. The NWFP rebellions under the Red banner and Chandra Bose were examples of these.The British created the powerful civil service and bureaucratic state (the so called “Iron frame of the Indian state”) to counter the indigenous political movements. A lot of India and Pakistan’s tussles between civil service bureaucrats and elected politicians have roots in the British legislation of the 1920s and 30s to empower British civil service officials to fight off the growing power of Indian political movements.And of course, the Brits formed the British Indian army for the revolutionaries.This is where Alvi’s Marxist doctrine describes the idea of an overdeveloped State apparatus presiding over territories not yet gelled together into a nation. An overdeveloped state presiding over an underdeveloped nation. The idea of a “Pakistan” and “India” did not exist back then. There was no sense of national identity. But a powerful state did exist over “Pakistan” and “India” which was rooted in colonial resource extraction concepts and composed of a highly developed bureaucracy and military to maintain state control against indigenous politicians and revolutionaries.In the West, the state developed first over centuries, organically, as long independence struggles formed the idea of national community with shared language, heritage and values gelling together into a nation with territorial definitions. These nations developed indigenous political movements which birthed their civil services and militaries.The reverse was true for us. Our over developed state presided over us long before we developed ideas for a shared nation and community. This rooted into us the ethnic conflict and political divisiveness we inherited as a nation upon independence. And its why our ex-colonial institutions like the judiciary, military and bureaucracy have long clashed with the indigenous political movements in the provinces.Post 1947, India manged to rid itself of most of these colonial extraction institutions under Nehru’s Jihads against the princes and their titles, his development of resource distribution institutions that redistributed extracted resources among the people rather than extracting them for a neo-colonial enterprise. His legacy has long been derided in India with slurs like the “license Raj” and “Hindu rate of growth” but his socialist practices were key to dismantling the colonial extraction institutions the British had left behind as well as granting local political autonomies among Indian regional elites so that the idea of an Indian nation could take root.Pakistan never underwent this process and although we have made major strides forward in this direction since the beginning of the 21st century, we still hold on to the same neo-colonial resource extraction model left behind by the British. It’s why we treated Bangladesh as a mere colony up until the 70s and are doing the same to Balochistan today. The state is only doing what it was originally designed to do: Colonial resource extraction through unelected bureaucratic and military complexes.What Imran Khan labels as corruption is merely the tributary system in place since the Mughals and the British: Votes go up, money goes down. Your clan or baradari vote for you to go to the center and expect state resources in the form of development projects and jobs to flow back to them. It’s the design of the Pakistani state since the British. A weak hold from a distant center which prefers to outsource the job of state power to local feudal lords and nazims rather than sending their own institutions in. Which makes sense because this system was put into place when the British and their military were numerically inferior to the vastness of India. And relied on the loyalty of local lords who in turn relied on the loyalty of their local people. Networks of patronage were developed where extracted resources were first redistributed by the British to local lords and by local lords to their enforcers. The system benefited all involved and was enforced by the British Indian army and civil service.Upon independence, the Pakistan Army and Pakistan civil service simply replaced the above mentioned roles. They formed a localized variant of the colonial enterprise who’s supra-state nature involved Pakistan itself being the local lord patronized by the global capitalist overlord, the US as the US took over global colonial enterprise networks from the British upon the onset of the cold war.If I as a bureaucrat or politician at the center want something done in a district in Chiniot, I contact the local feudal lord (dignified as an MPA or MNA) to do the job for me. His will is carried out since he is the conduit for the tributary system. Do I need someone arrested in his district or a school built? I tell him and pay him his fee for it. He splits the fee with his supporters and carries out the role of the state in it’s place. And i save money on police and schools (money which I don’t have anyway because the state keeps taxes low in order to placate it’s patronage networks).A weak, negotiated state with localized outsourcing. By design.Pray tell, what revolution will Imran Khan carry out when he has become a part of this state structure rather than dismantling it?Most of Pakistan’s corruption is white washed as legitimate in the form of development projects and defense budgets. People dont ask for 10% like Zardari anymore. They give out legitimate contracts to their buddy’s business and get consultant or referral fees. Or they spend on useless development projects with commissions and fees to local supporters.And this is culturally accepted in Pakistan because while we all demand the state do more for us, none of us are culturally inclined to pay more to the state, to let the state regulate our lives or live under the tight grip of it’s power. Pakistan has a long history of rebellion when such state power was imposed. Our political culture is too libertarian. And the clan/baradarie system too ingrained in our psyche. The rural areas see nothing wrong with being awarded development project money and government jobs in return for votes even though these are pretty blatant forms of corruption. We only look at the kickbacks received at the top when a politician takes a bribe but these ‘legitimate’ sources of corruption like Military generals being awarded jobs they aren’t qualified for after retirement or given agricultural land in valuable areas are never challenged. The housing schemes for judges. The tributary gifting of state money and resources to loyal voters in districts. And so on.The only real threats to such cultural and structural state issues were the Marxist movements of Pakistan which centered around land distribution and capturing state resources to distribute away from the center to local, regional centers.These movements also posed major threats to the Pakistani cultural matrix like the clan baradarie system and tribal politics with their revolutionary Marxist committees. And thus posed a major threat to the tributary and resource extraction systems built into the Pakistani state.Those movements have long died off with some remaining on the fringe (a socialist was elected in this election though).The only other movements challenging the status quo are the militant Islamists who funnily enough, have co-opted a ton of local Marxist agendas into their manifestos. When the Taliban first took Swat, their first step was to break the feudal hold by redistributing land and promising tax reforms and judicial relief. But nobody wants the Taliban in power, do we?Imran Khan’s promise of change is as restrained as Obama’s. Both are establishment candidates. They will not and cannot challenge the status quo since they are far from dangerous revolutionaries bent on overthrowing the system. And thus, they will remain confined to the superstructure of the state. And all the hereditary politics, tributary pay offs, legitimized corruption, resource extraction, exploitation and pandering to Islamists that goes with it. The PTI has already replaced the PML-N as the Patron to Punjabi Islamists like the SSP, who have murdered hundreds of Shia. Mr. Taseer has already tried running his son as his replacement in the assembly.Meet the new boss, same as the old boss. The real danger is on the horizon, possibly a decade from now. In the form of far right politicians like Khadim Rizvi and his TLP. They will be the Trump candidate of Pakistan.But there is a version of Pakistan that did radically alter and restructure the nature of their state: Modern day Bangladesh.Long treated as the colony of the Western wing, the rebels in the jungles of Bengal fulfilled all the necessary requirements for forging a nation state that Alvi predicted:A long drawn out independence struggle forged a post 1947 Bengali identity and sense of nation hood. A radical destruction of colonial extraction institutions and replacement with indigenous political movements to reacquire control of domestic resources took place. A military and civil service were forged under the umbrella of a new national identity and indigenous political bourgeois.When Bangladesh was forged, they were fraught with trouble trying to get rebels to lay down arms and join the national military, a decimated police force lay in tatters, the intelligentsia was slaughtered and poverty was so extreme there were accounts of people going to fish markets just to touch the fish oil and breath in it’s scent to fulfill their hunger.Yet the nation today is rushing past Pakistan in HDI and other economic indicators despite having less resources and advantages than Pakistan.The struggle that was required of them to forge this new nation is something most Pakistanis would not like to acknowledge because of the implications of how far we would have to go in terms of violence and revolution to achieve it.A note on India.As harsh as it may sound, the election has proven that the Pakistani electorate is still somewhat more to the left than India at the moment.The long war on terror has caused a significant halt to the rightwards drift of Pakistani politics for now, with terror attacks and violence dulling the aura of the Islamist appeal. It’s temporary but it is a halt. Small breakthroughs like the election of a Hindu MP from Sindh and a growing ruckus over social progress issues as well as the defeat of religious parties in the 2018 election all point to this, even as Imran Khan will probably reverse this anyway by making deals for coalition seats with religious conservatives (not confirmed as of yet).Indian observers were breathing a sigh of relief at the non-election of Hafiz Saeed but failing to note that several far right politicians have long been voted into Indian assemblies by the Indian electorate. So I’m not sure why there was such interest in making sure Pakistani far right candidates were defeated in elections when their far right equivalents in India are currently serving at the pleasure of the BJP.Also, I’m not sure if Hafiz Saeed’s defeat means much given how much inroads the TLP and other far right parties are making at the moment in Pakistani voting circles. Some would argue that the TLP are an even more dangerous strand of far right, extremist politics than anything we have seen before.Regarding peace with India, Imran Khan’s speech assigned it a fairly low priority with the bulk of his talk focusing on China, Afghanistan and the Islamic world at large (Iran and KSA in particular). This is because Imran knows that his benefactors in the GHQ would like him to keep his hands off the Indian foreign policy portfolio and he knows his place.The ‘boys’ didn’t splinter the PML-N’s right wing base with the Labaik protestss and send it’s top leadership to jail, paving the way for IK’s win, just to end up losing power to IK.This isn’t necessarily a bad thing because the military and the deep state have long indicated that they are willing to boost ties with India even if some mediocre arrangement on Kashmir is made. Several Pak Army leadership have expressed the desire to integrate India into CPEC (possibly at the urging of China) as well as become a transit hub for Indian trade to West Asia.The stumbling block has been the low intensity conflict in Kashmir which the hard liners are loath to block before any settlement on Kashmir.And on the Indian side, the BJP must make a strong show of force against the Paks especially as the elections draw near and they have their own PR image to maintain {56 inch chests and all).Indian political leaders are also loathe to negotiate with Pak Army generals. And history has shown that Indian leaders are far more generous even to weak Pakistani politicians than they are to Pakistani un-elected establishment. Indra Gandhi didn’t give an inch to Yahya but gave everything to Bhutto. Modi and Vajpayee were loath to touch shoulders with the boys in the GHQ but gave bear hugs to their elected counterparts in Pakistan.So it remains to be seen if the Imran Khan-Pak establishment nexus can provide a unified agenda which the Indians can agree on. But the Indian political leadership is well aware that they would be negotiating with the Pakistan army by proxy. Something they have loathed to do in the past.The spheres of ruler ship in Pakistan.The Pakistan Army does not want Imran Khan to rule Pakistan. They want him to govern it. And understanding the difference is key.The future direction of Pakistan on core issues like India and national security (whose definition remains murky by deliberate policy) will be above Imran Khan’s pay grade for the most part. But Imran is free to rule in spheres where the establishment holds little interest. Planting trees and education schemes I suppose. He will have to keep his hands off the defense budget. He cant negotiate with the Indians on his own. He cant stand up to the good generals.He can’t dismantle or alter the super structure of the Pakistani state.But he can affect change in other spheres. Right now he will be closely watched to see how he addresses issues of concern to the military. Afghanistan, India, the economy etc.But he cannot stop the patronage and pandering of extremists who are deemed useful to the state. He cannot stop the growing share of the military in the national economic pie. He cannot stop the legitimized corruption in the development budget, the public sector entities and the defense budget.He is expected to be an extension of the establishment in the legislature. And from that platform, he can do only what he can. What he is allowed to.No Promises.Back in the late 80s, Pakistan’s PM Junejo upon taking over power after Zia’s death was hailed as the accountability PM, the clean chit, fair ruler of Pakistan. His elevation to power was marked by his declaration that he would forgo the use of BMWs and Mercedez and would launch anti-corruption drives.How little Pakistan has changed since then, with Junejo’s accountability drive evaporating in the lost decade of the 90s with constant government changes and instability.Let’s take the promise of the PM house being turned into a university. The PM house university scheme has long been promised by every Prime Minister elect including Imran Khan’s predecessor Nawaz Sharif. However, the placement of the PM House in the security green zone and the impossibility of replacing the military liaison and coordination offices in the PM house made this impossible. Same for the KP CM’s promise of making the KPK CM house a university which was again located inside a security cordon and the CM house staff would get stuck in traffic if they CM was not located in the CM house.A pretty good example of the structural constraints of the state limiting the delivery of promises.But for Pakistan’s sake, I hope the nexus that rules Pakistan understands the urgency of all that is at stake. And delivers atleast some limited relief to the nation.In the bowl of this worldLook at the rose of our passion, my friendEven if we don't eat togetherEven if we don't sit togetherWe can at least dream together, my friendEven if we don't drink togetherEven if we are strangersLet us consider the colour of our wine, my friendThe sun is setting on the lanesThe river is almost at my doorLet us examine our restless hearts, my friendIn the Bowl of this World, Riffat AbbasPakistan Election 2018 results: Imran Khan declares victory, opponents allege rigging
How does LinkedIn's recommendation system work?
I gave this talk earlier this week at Hadoop World(http://www.hadoopworld.com/session/linkedin-products-you-may-like/), a conference that is evangelizing Hadoop by way of highlighting how people across the industry are solving big business challenges by leveraging Hadoop. I am posting here the slides with an approximate transcript of my talk.Ever since I studied Machine Learning and Data Mining at Stanford 3 years ago., I have been enamored by the idea that it is now possible to write programs that can sift through TBS of data to recommend useful things.So here I am with my colleague Adil Aijaz, for a talk on some of the lessons we learnt and challenges we faced in building large-scale recommender systemAt LinkedIn we believe in building platform, not verticals. Our talk is divided into 2 parts. In the first part of this talk, I will talk about our motivation for building the recommendation platform, followed by a discussion on how we do recommendations. No analytics platform is complete without Hadoop. So, in he next part of our talk, Adil will talk about leveraging Hadoop for scaling our products.‘Think Platform, Leverage Hadoop’ is our core message.Throughout our talk, we will provide examples that highlight how these two ideas have helped us ‘scale innovation’.With north of 135 million members, we’re making great strides toward our mission of connecting the world’s professionals to make them more productive and successful. For us this not only means helping people to find their dream jobs, but also enabling them to be great at the jobs they’re already in.With terabytes of data flowing through our systems, generated from member’s profile, their connections and their activity on LinkedIn, we have amassed rich and structured data of one of the most influential, affluent and highly-educated audience on the web.This huge semi-structured data is getting updated in real-time and growing at a tremendous pace, we are all very excited about the data opportunity at LinkedIn.For an average user, there is so much data, there is no way users can leverage all the data on their own.We need to put the right information, to the right user at the right time.With such rich data of members, jobs, groups, news, companies, schools, discussions and events. We do all kinds of recommendations in a relevant and engaging way.We have products like‘Job Recommendation’: here using profile data we suggest top top jobs that our member might be interested in. The idea is to let our users be aware of the possibilities out there for them.‘Talent Match’: When recruiters post jobs, we in real-time suggest top candidates for the job.‘News Recommendation’: Using articles shared per industry, we suggest top news that our users need to keep them updated with the latest happenings.‘Companies You May Want to Follow’: Using a combination of content matching and collaborative filtering, we recommend companies a user might be interested in keeping up-to date with.‘PYMK’: based on common attributes like connections, schools, companies and some activity based features, we suggest people that you may know outside of LinkedIn and may want to connect with at LinkedIn.‘Similar Profiles’: Finally our latest offering which we released a few months ago for recruiters, Similar Profiles. Given a candidate profile, we suggest top Similar candidates for hiring based on overall background, experience and skills.We have recommendation solutions for everyone, for individuals, recruiters and advertisersIn our view, recommendations are ubiquitous and they permeate the whole site.Before we discuss our motivation for building a recommendation platform or a discussion on how we do recommendation or how we leverage Hadoop. Let’s first answer a basic question: Are recommendations really important?To put things in perspective, 50% of total job applications and job views by members are a direct result of recommendations. Interestingly, in the past year and half it has risen from 6% to 50%.This kind of contribution is observed across all our recommendation products and is growing by the day.Let us start with an example of the kind of data we have.For a member, we have positions, education, Summary, Specialty, Experience and skills of the user from the profile itself. Then, from member’s activity we have data about members connections, the groups that the member has joined, the company that the member follows amongst others.Before we can start leveraging data for recommendation, we first need to clean and canonicalize it.Lets take an example for matching member to jobs,In order to accurately match members to jobs, we need to understand that all the ways of listing these titles refer to the same entity ‘Software Engineer’‘Technical Yahoo’ is a Software Engineer at Yahoo‘Member Technical Staff’ is a Software Engineer at Oracle‘Software Development Engineer’ is a Software Engineer at Microsoft‘SDE’ is a Software Engineer at AmazonSolving this problem is itself a research topic broadly referred to as ‘Entity Resolution’.As another example, How many variations do you think, we have for the company name ‘IBM’?When I joined LinkedIn, I was surprised to find that we had close to 8000+ user entered variations of the same company IBM.We apply machine learnt classifiers for the entity resolution using a host of features for company standardization.In summary, data canonicalization is the key to accurate matching and is one of the most challenging aspects of our recommendation platform.Now, we will discuss our motivation behind building a common platform by way of 3 key example trade-offs that we’ve encountered. In LinkedIn ecosystem, one trade-off that we encountered is that of real-time Vs time independent recommendations.Lets look at ‘News Recommendation’, which finds relevant news for our users. Relevant news today might be old news tomorrow. Hence, News recommendation has to have a strong real-time component.On the other hand, we have another product called ‘Similar Profiles’. The motivation here is that if a hiring manager, already know the kind of person that he wants to hire. He could be like person in his team already or like one of his connections on LinkedIn, then using that as the source profile, we suggest top Similar Profiles for hiring. Since, ‘people don’t reinvent themselves everyday’. ‘People similar today are most likely similar today’. So, we can potentially do this computation completely offline with a more sophisticated model.These are the 2 extreme cases in terms of ‘freshness’: Most examples fall into an intermediate category. For E.g. new jobs gets posted by the hour and they expire when they get filled up. All jobs posted today don’t expire the same day. Hence, we cache job recommendation for members for some time as it is OK to not recommend the absolute latest jobs instantly to all members.In solving the completely real-time Vs completely offline problem, we could have gone down the route of creating separate solutions optimized for the use-case. In the short run, that would have been a quicker solution.But we went down the platform route because we realized that we would churn out more and more such verticals as LinkedIn grows. Now, as a result of which we have the same code that computes recommendations online as well as offline. Moreover, in the production system caching and an expiry policy allows us to keep recommendations fresh irrespective of how we compute the recommendations. Now, as a result for newer verticals. We can easily get ‘freshness’ of recommendations irrespective of whether we compute recommendations online or offline.Another interesting trade-off, is choosing between content analysis and collaborative filtering.Historically speaking: ‘job posting has been a post-and-prey’ model. Where job posters post a job and pray that hopefully someone will apply for the job. But we at LinkedIn, believe in ‘post-and-produce’ so we go ahead and produce matches to the job poster in real-time right after the job gets posted. When someone posts a job, the job poster naturally expects the candidates to have a strong match between job and the profile. Hence, this type of recommendation is heavy on content analysis.On the other hand, we have a product called ‘Viewers of the profile also viewed ..’. When a member views more than 1 profile within a single session. We record it as a co-view. Then on aggregating these co-views for every member. We get the data for all profiles that get co-viewed, when someone visits any given profile. This is a classical collaborative filtering based recommendation much like Amaozon’s ‘people who viewed this item also viewed’Most other recommendations are hybrid. For e.g. for ‘SimilarJobs’ jobs that have high content overlap with each other are similar. Interestingly, jobs that get applied to or viewed by the same members are also Similar. So, Similar Jobs is a nice mix of content and collaborative filtering.Again, Because of a platform approach, we can re-use the content matching and the collaborative filtering components to come up with newer verticals without re-inventing the wheel.Finally, the last key trade-off is of precision vs recall.On our homepage, we suggest jobs that are good fit for our users with the motivation for them to be more aware of the possibilities out there. In some sense, we are pushing recommendations to you, as opposed to you actively looking for them.Even if a single job recommendation looks bad to the user either because of a lower seniority of the job or because the recommendation is for the company that the user is not fond of, our users might feel less than pleased.Here, getting the absolute best 3 jobs even at the cost of aggressively filtering out a lot of jobs is acceptable.On the other hand, We have another recommendation product called ‘Similar Profiles’ for hiring managers who are actively looking for candidates. Here, if one finds a candidate we suggest other candidates like the original one in terms of overall experience, specialty, education background and a host of other features.Since, the hiring manager is actively looking so in this case they are more open to getting a few bad ones as long as they get a lot of good ones too. So in essence, recall is more important here.Again, because of a platform approach and because we can re-use features, filters and code-base across verticals. So, tuning the knob of more precision vs more recall is mostly a matter of figuring out 1. how complicated the matching model should be and 2. how aggressively we want to apply filters for all recommendation verticals. Hence, our core message ‘Think Platform’. Now, we will discuss in some detail how our recommendations work.Lets see how we do recommendations by taking an example of ‘Similar Profiles’ that we just discussed. Given a member profile, the goal is to find other similar people for hiring. Lets try to find profiles similar to me.Here, we look at host of different features, such as1. User provided features like ‘Title, Specialty, Education, experience amongst others’2. Complex derived features like ‘Seniority’ and ‘Skills’, computed using machine learnt classifiers.3. Both these kinds of features help in precision, we also have features like ‘Related Titles’ and ‘Related Companies’ that help increase the recall.Intuitively, one might imagine that we use the following pair of features to compute Similar Profiles. In the next slide, we will discuss a more principled approach to figuring out pair of features to match against.Here in order to compute overall of similarity between me and Adil, we are first computing similarity between our specialties, our skills, our titles and other attribute.With this we get a ‘Similarity score vector’ for how Similar Adil is to me, Similarly we can get such a vector for other profiles.Now we somehow need to combine the similarity score in the vector to a single number s.t. profiles that have higher similarity score across more dimensions get ranked higher, as similar profiles for me.Moreover, the fact that our skills match might matter more for hiring than whether our education matches. Hence, there should be relative importance of one feature over the others.Once we get the topK recommendations, we also apply application specific filtering with the goal of leveraging domain knowledgeFor example, it could be the case that for a ‘Data Engineer role’ you as a hiring manager are looking for a candidate like one of your team member but who is local. Whereas, for all you know the ideal ‘Data Engineer most similar to the one you are looking for in terms of skills might be working somewhere in INDIA’ .To ensure our recommendation quality keeps improving as more and more people use our products, we use explicit and implicit user feedback combined with crowd-sourcing, to construct high quality training and test sets for constructing the ‘Importance weight vector’. Moreover, classifier with L1 regularization helps prune out the weakly correlated features. We use this for figuring out which features to match profiles against.We just discussed an example. However, the same concepts apply to all the recommendation verticals.And now the technologies that drives it all.The core our matching algorithm uses Lucene with our custom query implementation.We use Hadoop to scale our platform. It serves a variety of needs from computing Collaborative filtering features, building Lucene indices offline, doing quality analysis of recommendation and host of other exciting things that Adil will talk about in a bit.Lucene does not provide fast real-time indexing. To keep our indices up-to date, we use a real-time indexing library on top of Lucene called Zoie.We provide facets to our members for drilling down and exploring recommendation results. This is made possible by a Faceting Search library called Bobo.For storing features and for caching recommendation results, we use a key-value store Voldemort.For analyzing tracking and reporting data, we use a distributed messaging system called Kafka.Out of these Bobo, Zoie, Voldemort and Kafka are developed at LinkedIn and are open sourced. In fact, Kafka is an apache incubator project.Historically, we have used R for model training. We have recently started experimenting with Mahout for model training and are excited about it.All the above technologies, combined with great engineers powers LinkedIn’s Recommendation platform.Now Adil will talk about how we leverage Hadoop.In the second half of our talk we will present case studies on how Hadoop has helped us scale innovation for our Recommendation Platform. We will use the ‘Similar Profiles’ vertical which was discussed earlier as the example for each case study. As a quick reminder, similar profiles recommends profiles that are similar to the one a user is interested in. Some of its biggest customers are hiring managers and recruiters. For each of the case studies, we will lay out the solutions we tried before turning to Hadoop, analyze the pros and cons of the approaches before and after Hadoop, and finally derive some lessons that are applicable to folks working on large scale recommendation systems.When it comes to recommendations, relevance is the most important consideration. However, with over 120M members, and billions of recommendation computations, the latency of our recommendations becomes equally important. No mater how great our recommendations are, they wont be of utility to our members if we take too long to return recommendations. Among our many products, ‘Similar Profiles’ is a particularly challenging product to speed up. Our plain vanilla solution involved using a large number of features to mine the entire member index for the best matches. The latency of this solution was in the order of seconds. Clearly, no matter how relevant our recommendations were, with that type of latency, our members would not even wait for the results. So, something had to be done. We needed a solution that could pre-filter most of the irrelevant results while maintaining a high precision on the documents that survived the filter. One technique that meets these conditions is minhashing. On a very high level, minhashing involves running each document, in our case member profiles, through k hash functions to to construct a bit vector. One can play with ANDING/Oring of subsets of the bit vector, to get the right balance between recall and precision. As our second pass solution, we minhashed each document and stored the resulting bit array in the member index. At query time, we minhashed the query into a bit array, filtered out documents that did not have the exact same subsets of the bit array, and finally did advanced matching on documents that survived the filtration. This solution brought down the latency well below a second, however, minhashing did not give us the recall we had hoped for. This was a really disappointing result since we had spent significant engineering resources in productionalizing minhashing, yet it was all for nought.So, we went back to the drawing board and started thinking about how we can use Hadoop to solve this problem. The key breakthrough was when we realized that people do not reinvent themselves everyday. The folks I was similar to yesterday, are likely to be the same folks I am similar to today. This meant that we could serve ‘similar profiles’ recommendations from cache. When the cache would expire, we could compute fresh recommendations online and repopulate the cache. This meant that the user would almost always be served from cache. Great: but we still have to populate the cache somehow. This is where Hadoop comes into the picture. By opening an index shard per mapper, we can generate a portion of the recommendations in each mapper, and combine them into a final recommendation set in the reducers. With the distributed computation of Hadoop, we easily generate similar profiles for each member, and then copy over the results to online caches. So the three elements of:a) Offline batch computations on Hadoop copied to online caches.b) Aggressive online caching policyc) Online computation when the cache expiresHave scaled our similar profiles recommendations while maintaining a high precision of recommendations.So, the key takeaway from this case study of scaling is that if one is facing the problem of:a) High latency computationb )High qpsc) And not so stringent freshness requirementsThen one should leverage Hadoop and caching to scale the recommendation computation.With our scaling problems solved, we rolled out Similar Profiles to our members. The reception was amazing. However, we felt that we could do even better by going beyond content based features alone. One such feature that we wanted to experiment with was collaborative filtering. More specifically, if a member browses multiple member profiles within a single session, aka coviews, it is quite likely that those member profiles are very similar to each other. How we blend collaborative filtering with existing content based recommendations is the subject of our second case study: “blending multiple recommendation algorithms”.Our basic blending solution is this: While constructing the query for content based similar profiles, we fetch collaborative filtering recommendations and their scores, and attach them to the query. In the scoring of content based recommendations, we can use the collaborative filtering score as a boost. An alternative approach is a bag of models approach with content and collaborative filtering serving as two of the models in the bag.In either solution, we need a way to keep collaborative filtering results fresh. If two member profiles were coviewed yesterday, we should be able to use that knowledge today.We first sketched out a completely online solution. This online solution involved keeping track of the state of each member session, accumulating all the profile views within that session. At the end of each session, we updated the counts of the various coview pairs. As you can appreciate, such a stateful solution can get very complicated very quickly. As an example, we have to worry about machine failures, multi data center coordination just to name two challenges. In essence such a solution could introduce more problems than it solves. So, we scratched this solution even before implementing it.We thought more about this problem and realized two important aspects: 1) Coview counts can be updated in batch mode. 2) We can tolerate delay in updating our collaborative filtering results.These two properties, batch computation and tolerance for delay in impacting the online world, led us to leverage Hadoop to solve this problem. Our production servers can produce tracking events everytime a member profile is viewed. These tracking events are copied over to hdfs where everyday, we use these tracking events to batch compute a fresh set of collaborative filtering recommendations. These recommendations are then copied to online key value stores where we use the blending approaches outlined earlier to blend collaborative filtering and content based recommendations.Compared to the purely online solution, the Hadoop solution is simpler in complexity, less error prone, but introduces a lag between the time two profiles are coviewed and the time that coview has an impact on similar profiles. For us, this solution works great. The other great thing about this solution is that it can be easily extended to blend social or globally popular recommendations in addition to collaborative filtering.The lesson we derive from this case study is that by leveraging Hadoop, we were able to experiment with collaborative filtering in similar profiles without significant investment in an online system to keep collaborative filtering results fresh. Once our proof of concept was successful, we could always go back and see if reducing the lag between a profile coview and its impact on similar profile by building an online system would be useful. If it is, we could invest in a non-Hadoop system. However, by leveraging Hadoop, we were able to defer that decision till the point when we had data to backup our assumptions.A consistent feedback from hiring managers using Similar Profiles was that while the recommendations were highly relevant, often times the recommended members were not ready to take the next steps in their professional career. Such recommended members would thus respond negatively to a contact from the hiring manager, leading to a bad experience for the hiring manager. This feedback indicated a strong preference from our users that they would like a tradeoff between relevance of recommendations and the responsiveness of those recommendations. One can imagine a similar scenario playing out for a sales person looking for recommendations for potential clients.As our next case study, let’s take a look at how we approached solving this problem for our users. Let’s say we come up with an algorithm that assigns each LinkedIn member a ‘JobSeeker’ score which indicates how open is she to taking the next step in her career. As we said already, this feature would be very useful for ‘Similar Profiles’. However, the utility of this feature would be directly related to how many members have this score: aka coverage. The key challenge we faced was that since ‘Similar Profiles’ was already in production, we had to add this new feature while continuing to serve recommendations. We call this problem “grandfathering”.A naïve solution, could be to assign a ‘jobseeker’ score to a member next time she updates her profile. This approach will have minimal impact on the system serving traffic, however, we will not have all members tagged with such a score for a very long time, which impacts the utility of this feature for ‘Similar Profiles’.So, we scratch the naïve solution and look for a solution that will batch update all members with this score in all data centers while serving traffic.A second pass solution is to run a ‘batch’ feature extraction pipeline in parallel to the production feature extraction pipeline. This batch pipeline will query the db for all members and add a ‘job seeker score’ to every member. This solution ensures that we have an upper bound on the time it takes to grandfather all members with job seeker score. It will work great for small startups whose member base is in a few million range.However, the downside of this solution at LinkedIn scale is:1) It adds load on the production databases serving live traffic.2) To avoid the load, we end up throttling the batch solution which in turn makes the batch pipeline run for days or weeks. This slows down rate of batch update.3) Lastly, the two factors above combine to make grandfathering a ‘dreaded word’. You only end up grandfathering ‘once a quarter’ which is clearly not helpful in innovating faster.So, we clearly cannot use that solution either. However, one good aspect of this solution is the batch update which leads us into a Hadoop based solution.Using Hadoop, we take a snapshot of member profiles in production, move it to hdfs, grandfather members with a ‘job seeker score’ in a matter of hours, and copy this data back online. This way we can grandfather all members with a job seeker score in hours.The biggest advantage of using Hadoop here is that grandfathering is no longer a ‘dreaded word’. We can grandfather when ready instead of grandfather every quarter which speeds up innovation.So, in a nutshell, if one finds oneself slowed down due to constraints of updating features in the online world, consider batch updating the features offline using Hadoop and swapping them out online.With the first few versions of similar profiles out the door, we began to simultaneously investigate a number of avenues for improvement. Some of us investigated different model families, say logistic regression vs SVM, others investigated new features with the existing model. In this case study, we will talk about how we decided which one of these experiments would actually improve the online relevance of similar profiles so we could double down on getting them out to production. We are not concerned with ‘how’ we come up with these new models. For all that matters, we hand tuned a common sense model. The question is how to decide whether or not to move that new model to production.Now, as a base solution, we can always move every model to production. We can A/B test the model with real traffic and see which models sink and which ones float. The simplicity of this approach is very attractive, however, there are some major flaws with it:1) For each models we have to push code to production. This takes up valuable development resources.2) There is an upper limit on the number of A/B tests one can run at the same time. This can be due to user experience concerns and/or revenue concerns.3) Since online tests need to run for days before enough data is accumulated to make a call, this approach slows down rate of progress.Ideally, we would like to try all our ideas offline, evaluate them, and only push to production the best ones. Hadoop proves critical in the evaluation step. Overtime, using implicit and explicit feedback combined with crowdsourcing, we have accumulated a huge gold test set for ‘similar profiles’. We rank the gold set with each model on Hadoop, and use standard ranking metrics to evaluate which one performs best.As you can guess, Hadoop provides a very good sandbox for our ideas. We are able to filter many of the craziest ideas, and double down on only those few that show promise. Plus, it allows us to have relatively large gold sets which gives us strong confidence in our evaluation results.Now that we have learned a new model for ‘Similar Profiles’ that performs well in our offline evaluation framework, we need to test it online. An industry standard approach to this problem is known as A/B testing or bucket testing. Formally, AB testing involves partitioning real traffic between alternatives and then evaluating which alternative maximizes the desired objective. Typical desired objectives are CTR, revenue, or number of views.The key requirements of AB testing is that: time to evaluate which bucket to send traffic to should ideally be < 1ms, at-worst a few ms.Let’s discuss how we would do A/B testing for our new model. For simple partitioning requirements, one can use a mod-based scheme. This is very fast and very simple and can satisfy most use cases. However, if one wishes to partition traffic based on profile and member activity criteria for e.g. “Send 10% of members who have greater than 100 connections AND who have logged in the last one week AND who are based in Europe” then doing this online is too expensive. Keep in mind that deciding which bucket to send traffic to should be very fast, ideally less than a millisecond. In worst case scenario a few milliseconds. I am not going to even attempt an online solution for this problem.So, we go straight to Hadoop. For complex criteria like this, we run over our entire member base on Hadoop every couple of hours, assigning them to the appropriate bucket for each test. The results of this computation are pushed online, where the problem of A/B testing reduces to given a member and a test, fetching which bucket to send the traffic to from cache.The take home message here is: If you need complex targeting and A/B testing, leverage Hadoop.Our last case study involves the last step of a model deployment process: tracking and reporting. These two steps allow us to have an unbiased, data-driven way of saying whether or not a new model is successful in lifting our desired metrics: ctr or revenue or engagement or whatever else one is interested in. Our production servers generate tracking events every time a recommendation is impressed, clicked or rejected by the member.Before Hadoop, we used to have an online reporting tool that would listen to tracking events over a moving window of time, doing in-memory joins of different streams of events and reporting up-to-the minute performance of our models. The clear advantages of this approach were that we could see exactly how the model was performing online at this moment. However, there are a few downsides such asa) One cannot look at greater than certain amount of time window in the past..b) As the number of tracking streams increases, it becomes harder and harder to join them online.To increase the time window, we will have to spend significant engineering resources in architecting a scalable reporting system which would be an overkill. Instead we placed our bet on Hadoop. All tracking events at LinkedIn are stored on HDFS. Add to this data Pig or plain Map-Reduce, we can do arbitrary k-way joins across billions of rows to come up with reports that can look as far in the past as we want to.The advantages of this approach are quite clear. Complex joins are easy to compute and reporting is flexible on time windows. However, we cannot have up-to-the minute reports since we copy over tracking events in batch to hdfs. If we ever need that level of reporting, we can always use our online solution.We can say without any hesitation that Hadoop has now become an integral part of the whole life-cycle of our workflow starting from prototyping a new idea to eventually tracking the impact of that idea.By thinking about platform and not verticals, we are able to to come with newer verticals at a fast pace.By leveraging Hadoop, we were able to continuously improve the quality and scale the computations.Hence, these 2 ideas helped us ‘scale innovation’ at Linkedin.To conclude, we want to say that the data opportunity at LinkedIn is HUGE and so come work with us at LinkedIn!
- Home >
- Catalog >
- Miscellaneous >
- Evaluation Form >
- Job Evaluation >
- importance of job evaluation >
- Job Evaluation Scheme For Rank To Role