How to Edit The Combining Probability And Non Probability Sampling Methods freely Online
Start on editing, signing and sharing your Combining Probability And Non Probability Sampling Methods online under the guide of these easy steps:
- Click on the Get Form or Get Form Now button on the current page to direct to the PDF editor.
- Give it a little time before the Combining Probability And Non Probability Sampling Methods is loaded
- Use the tools in the top toolbar to edit the file, and the change will be saved automatically
- Download your edited file.
The best-reviewed Tool to Edit and Sign the Combining Probability And Non Probability Sampling Methods
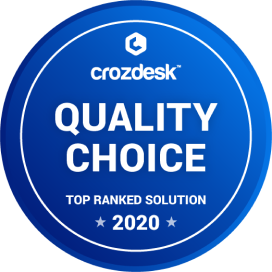
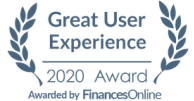
Start editing a Combining Probability And Non Probability Sampling Methods in a minute
Get FormA simple guide on editing Combining Probability And Non Probability Sampling Methods Online
It has become very simple just recently to edit your PDF files online, and CocoDoc is the best PDF online editor you would like to use to make some editing to your file and save it. Follow our simple tutorial to start trying!
- Click the Get Form or Get Form Now button on the current page to start modifying your PDF
- Create or modify your content using the editing tools on the tool pane on the top.
- Affter changing your content, put the date on and add a signature to bring it to a perfect comletion.
- Go over it agian your form before you click and download it
How to add a signature on your Combining Probability And Non Probability Sampling Methods
Though most people are accustomed to signing paper documents with a pen, electronic signatures are becoming more accepted, follow these steps to eSign PDF!
- Click the Get Form or Get Form Now button to begin editing on Combining Probability And Non Probability Sampling Methods in CocoDoc PDF editor.
- Click on Sign in the tools pane on the top
- A popup will open, click Add new signature button and you'll be given three choices—Type, Draw, and Upload. Once you're done, click the Save button.
- Drag, resize and position the signature inside your PDF file
How to add a textbox on your Combining Probability And Non Probability Sampling Methods
If you have the need to add a text box on your PDF in order to customize your special content, do some easy steps to get it done.
- Open the PDF file in CocoDoc PDF editor.
- Click Text Box on the top toolbar and move your mouse to drag it wherever you want to put it.
- Write down the text you need to insert. After you’ve input the text, you can take full use of the text editing tools to resize, color or bold the text.
- When you're done, click OK to save it. If you’re not satisfied with the text, click on the trash can icon to delete it and start again.
A simple guide to Edit Your Combining Probability And Non Probability Sampling Methods on G Suite
If you are finding a solution for PDF editing on G suite, CocoDoc PDF editor is a commendable tool that can be used directly from Google Drive to create or edit files.
- Find CocoDoc PDF editor and set up the add-on for google drive.
- Right-click on a PDF file in your Google Drive and choose Open With.
- Select CocoDoc PDF on the popup list to open your file with and give CocoDoc access to your google account.
- Edit PDF documents, adding text, images, editing existing text, highlight important part, trim up the text in CocoDoc PDF editor before saving and downloading it.
PDF Editor FAQ
What are the sampling methods?
There are basically two types of sampling:• Probability Sampling: The sample has a known probability of being selected.• Non-probability Sampling: The sample does not have a known probability of being selected, as in convenience or voluntary response surveys or surveys based on the subjective judgment of the researcher.The latter can often be viewed as an inferior alternative to probability sampling techniques and are not used to make generalizations (i.e., statistical inferences).That being said, we focus on the first one, which eliminates possible conscious or inherent bias in those conducting the study. These methods are considered representatives of the population as a whole and are used to make generalizations (i.e., statistical inferences).These are the basic probability sampling types:Simple random samplingAll possible samples of n objects from the population are equally likely to be selected to be in the sample. The most common real life equivalent would be a lottery or sweepstake.Researchers can create a simple random sample with a lottery, where each member of the population is assigned a number, after which these are selected at random. For larger size populations, this method can be quite time consuming. Selecting a random sample from a large population usually requires a computer generating pseudo-random numbers and a process similar to the lottery is used; only the number assignments and subsequent selections are performed by computers instead of humans.Stratified samplingThe population is divided into groups, called strata, based on some pre-defined characteristic. Then, within each group, a simple random sample is selected. As an example, suppose we conduct a survey and we divide the population into groups based on geography - north, east, south, and west. Then, within each group, we randomly select respondentsCluster samplingIn this method, every member of the population is assigned to one, and only one, group, called a cluster. A sample of clusters is chosen, using a simple random sample. Only individuals within sampled clusters are surveyed.To proceed, the population is divided first into N groups or clusters. Then n clusters are randomly selected to include in the sample. Each element of the population can be assigned to only one cluster. This method is better used when the population is concentrated in "natural" clusters (city blocks, schools, hospitals, etc.).For example, to conduct personal interviews of emergency room doctors, it might make sense to randomly select a sample of hospitals and then interview all of the emergency room doctors at that hospital. Using cluster sampling, the interviewer could conduct many interviews in a single day at a single hospital. Simple random sampling, in contrast, might require the interviewer to spend all day traveling to conduct a single interview at a single hospital and so on to cover as many hospitals as needed for the sampling.The difference between cluster sampling and stratified sampling is that with stratified sampling, the sample includes elements from each stratum and with cluster sampling, the sample includes elements only from sampled clusters.Systematic random samplingWith this method, we create a list of every member of the population. From this list, we randomly select the first sample element from the first k elements . This interval (k), called the sampling interval, is calculated by dividing the population size by the desired sample size. Thereafter, we select every kth element on the list.Despite the sample population being selected in advance, systematic sampling is still thought of as being random if the periodic interval k is determined beforehand and the starting point is random. However, this method is different from simple random sampling since every possible sample of n elements is not equally likely.For example, a researcher has a population total of 100 individuals and need 12 subjects. He first picks his interval as 8 (k = 100/12), then choose his starting number at random form 1 to 8, lets say 5 . The members of his sample will be individuals 5, 13, 21, 29, 37, 45, 53, 61, 69, 77, 85, 93.Multistage samplingWith this method, we select a sample by using combinations of different sampling methods. For example, in Stage 1, we might use cluster sampling to choose clusters from a population. Then, in Stage 2, we might use simple random sampling to select a subset of elements from each chosen cluster for the final sample.Here’s a full explanation of sampling: Sampling (statistics) - Wikipedia
How does word2vec work? Can someone walk through a specific example?
There are already detailed answers here on how word2vec works from a model description perspective; focussing, in this answer, on what word2vec source code actually does (for those like me who are not endowed with the mathematical prowess to gain intuition from just reading the paper or the model description; on first glance, the relationship of word2vec model [as described in Mikholov’s paper of learning word representations that can predict surrounding context words] to the source code implementation, is not apparent).Regardless of the model (skip gram/continuous bag of word) or sampling (hierarchical/negative sampling) code path, one chunk of code pattern is largely similar for all the four code paths in ~700 lines of word2vec C source code (the snippet below is skipgram-negative sampling case).In those few lines above (largely similar for all four code paths) lies the essence of word2vec unsupervised training.If we take any two vectors, both of say, 300 dimensions, that are randomly initialized with some values, and if we add just a tiny bit of one vector to the other, the vectors get “closer” to each other (the cosine similarity increases), simply by virtue of vector addition. Figure below shows this for 2 dimensions.If we subtract a tiny bit of one vector from the other - the vectors will move “apart” (cosine similarity decreases) by a tiny bit (In the boundary case of both vectors being exact opposite direction of each other, one vector will be nudged to eventually flip from opposite to same direction, as its magnitude decreases/increases with each positive nudge).So during word2vec training , in each step, every word is either (1) “nudged” (as Stephan mentions in his response) or pulled closer to words that it co-occurs with, within a specified window or (2) nudged/pushed away from all the other words that it does not appear with. Since typical neighborhood window is small (e.g. 5–15) pulling operations when a word is tugged by its neighbors is bounded by this window size; however for a large corpus, pushing away non-neighborhood words is an expensive operation to do. So word2vec has two schemes (hierarchical and negative sampling), where nudging away of all other words not in neighborhood of a word is avoided; instead just a sample of words are nudged.(Pushing and pulling effectively alter the probability of occurrence of a word, given its neighborhood words; the reason to both pull vectors closer[ increase probability] and push vectors apart[ decrease probability] is tantamount to computing the denominator in the softmax function - equation 2 in Mikholov’s paper - the probability of occurrence of a word given its neighborhood. The probabilities themselves are not explicitly calculated in source code since the objective is only to find the vectors that maximizes the probabilities as given in equation 1 in the paper mentioned above).In the negative sampling method, every time a word is brought closer to its neighbors, a small number of other words (randomly chosen from a unigram distribution of all words in the corpus) are nudged/pushed away.In the hierarchical sampling method, every neighboring word is pulled closer or further apart from a subset of words (log2(corpus size)), where the word subset for each word is chosen from a tree structure (binary Huffman tree is used in word2vec where short binary codes are assigned to frequent words).In the source code snippet above two words indexed by l1 and l2 are either brought together or pushed apart depending on whether they co-occur or not in corpus (label = 1 is co-occurring case; label = 0 is negative sampling case). When they co-occur (label = 1 case in lines 522–524 above) the nudge is positive. In the negative sampling case (label = 0 case in lines 522–524 above), the nudge is negative.So the training process is essentially a tug of war of vectors (as Xin Rong mentions) that are being pulled closer or pushed further apart. The end of the training (sufficient iterations) produces as output, the magic, of words that are not only co-occurring in corpus to come closer, but also instances of words coming closer to each other even if they don’t co-occur (an example illustrated below) in corpus. The other non-intuitive consequence of this training is similar orientation of vectors between analogous words enabling simple vector algebra to discover analogous words, popularly illustrated everywhere (king - man + woman yielding queen).Walking through each line of source above.Lines 521–524 compute the tiny scale factor for nudging vectors closer/further apart. In the case of nudging vectors closer, the scale factor iszero when the vectors are already pointing in the same direction,or a tiny positive value (a scale factor is stored in a pre-computed table of sigmoid function 1/(1 + e^-x) for the region -6 to 6 where slope is non-zero. This scale factor is multiplied with a learning rate to yield a small positive value).Line 525 computes the scaled version of word l2 (ignoring the description of line 526 for now)Line 529 adds the tiny scaled version of l2 computed in line 525 to l1. In the negative sampling case, the added value is the negative of l2, which is effectively a subtraction. So, in essence, l1 gets a little closer to words l2 that co-occur in corpus and further apart from those words that it does not co-occur with (since the words to be pushed away are chosen from a unigram distribution of all words in corpus, there is a small possibility of a neighbor being picked too and nudged away during training).Walking through a specific example for the toy corpus below trained with each word being represented by 300(should have been a sixth of it or lesser - 300 is an overkill for this toy corpus; results are same for a dimension of 10 for this corpus) dimensions, skipgram model , and negative sampling (1 sample):The top 10 closest neighbors words to the word “dogs” are “wolves”, “variety”, “large”, “mammals”, “humans”, “largely”, “are”, “genetic”, “roots”, “in”. The top 10 closest to the word “dogs” for skipgram, hierarchical sampling are “mammals”, ”large”, ”humans”, ”wolves”,”largely”,”variety”,”are”,”genetic”,”days”, “tigers”.Word2vec training only brings together words that are in the same line of a word and within the specified window context. So “dogs” will not be trained with “humans”, with a context window of size 5, even though that window size straddles across to include “humans” as within context for “dogs”. In other words,the code stub above will never be invoked with “human” and “dogs” being l1/l2 and label=1( however the code stub, above may be invoked, in negative sampling case, for “humans” and “dogs”, i.e. with label=0). So, in essence, “humans” and “dogs”, that do not co-occur, are never explicitly nudged closer together ( but may be pushed apart in negative sampling case). One can only observe words like “cats” and “dogs” nudged closer by the above code stub during training.The top 3 neighbors to the word “dogs” - “wolves”, “variety”and “large” are apparent from the toy corpus - those words co-occur and get closer by the simple training process described above.The word “mammals” and “humans” don’t co-occur with “dogs” and yet they are closer to “dogs” than other words like “largely”,”are”, that actually co-occur with “dogs”. The intuition for this is that, “cats” occur with “mammals”; so “mammals” are pulled closer to “dogs”. Similarly, “pets” co-occur with “humans” and bring “humans” close to “dogs”. It is interesting to note, that even though “cats” bring “mammals” closer to “dogs”, “cats” itself does not occur in the top 10 neighbors of “dogs” - it falls down beyond the top 10 list in the “tug of war” with other words. Similarly for “pets” - “pets” doesn’t make it into the top 10 words close to “dogs” though it brings “humans” closer to “dogs” (one can see how “pets” brings “humans” close to “dogs” by just replacing “pets” in the first line with “animals” and retraining on modified corpus - “humans” will fall off the top 10 words close to “dogs”).The net effect of the tug of war of word vectors pulling/pushing each other closer/apart is:Entities (e.g. dogs, cats, humans) that appear together get pulled closer to each other, with all the frequently occurring glue words like “the, are, in” removed (explained below).Entities that do not even appear together in sentences, but are transitively linked by neighbor entities, by their occurrences in training corpus, get pulled closer (e.g. dogs and mammals in above toy corpus linked by “pets”).Glue words (or stop words) like “the”, “are”,”in” pull each other closer and away from the entities they occur with. This fact is not evident from the toy corpus given its small size - it is evident in larger corpus. For example, in a corpus of 6.4 billion words with 20 million unique words, the top neighbors of the word “the” are: “of”, ”in”, ”and”, ”which”, ”a”. Conversely, one will not find frequently occurring glue words like “in” appear in the top 10 nearest neighbors list of an entity like “dogs” ( “in” appears in the “dogs” neighbor list above because it is a very small corpus) - they will all be other entities (e.g. cats etc).The “tug of war” on words has an impact on the magnitude on word vectorsThe vector magnitude of words that occur the most or the least in the corpus have lower magnitudes than those in the middle. For instance, in a 20 million unique word corpus, a word like “the” that occurs ~780,000 times has a vector magnitude of 2.39. A word that occurs just once in the corpus has a magnitude of .606; a word that occurs 64,00 times has a magnitude of 4.7. Words in the middle of the occurrence frequency scale, 2000–70,000 occurrences, range in magnitude from 4 to 12.This may be just a consequence of the fact that a frequently occurring word like “the” gets pulled closer in all directions, leading to the overall reduction in its magnitude - since its context spans nearly the whole corpus, for every neighbor word that nudges it in a certain direction, there is likely to be another neighbor word vector that nudges it closer to itself, in the exact opposite direction cancelling the effect of the previous nudge. However, despite this, “the” along with its high frequency cohorts like “in”, manage to huddle together, given their large numbers (“in” would pull “the” many more times than any entity like “dogs”,”cats” etc. in a large corpus). For words that occur very rarely, the nudges are few relative to the other words anyway (e.g. for 5 full iterations over the corpus, the vector for the word “the” will be pulled, or updated in 780,000*5 iterations, whereas the vector for a word that occurs just once in corpus will be updated a meagre 5 iterations) - hence their magnitudes are also low. (The hyperparameter for subsampling is assumed to be zero in the discussion above).The vector magnitudes are a relevance measure for the “important words” in a corpus. High frequency and low frequency words have low magnitudes, while those in the middle have high magnitudes - like a “Goldilocks zone”. For instance, in a 11 billion word, 66 million unique word corpus, just 2% of terms have magnitudes above 4 - all high frequency stop words and low frequency words have magnitudes less than 4. While low frequency words may show up like “needle in a haystack” in the neighborhood of some terms , and may prove insightful in some applications (unlike their high frequency word counterparts who seem to have no utility at all in most cases - they can even be burdensome; for instance when trying to generate phrases - they blow up phrase combinations when using word2phrase to generate phrases ; section 4 in Mikholov’s paper ), they are often not reliable since they are nudged so few times, as mentioned above (though according to Mikholov’s paper subsampling improves vector representations of low frequency words).In the “tug of war” on words, even though pulling a word in different directions may seem tantamount to “pushing” apart, the explicit operation of pushing apart (in both sampling schemes) has a direct impact on vector neighborhood quality (this is of course ignoring the fact that softmax calculation requires all words to be considered in predicting their occurrence with a word). For instance, even in the small toy corpus, (where negative sampling value was as low as 1 in the neighborhood examples shown earlier) the neighborhood for “dogs” in skipgram, with negative sampling set explicitly to zero (needs simple code change in word2vec C source) - the quality deterioration is apparent - the top 10 neighbors of “dogs” become “and”, “of”, “humans”, “breeding”, “large”, ”largely”, ”performing”, ”the”.In the implementation, two words that co-occur are not directly trained to get closer to each other - instead they are brought together by a “level of indirection”. For instance, in the negative sampling case, each word vector(in syn0), say “dogs” has an image(or context) vector (captured in syn1neg in line 526 above) that captures the context of words around that word - it is that context vector of dogs that is trained to get closer to the words “dogs” co-occurs with (e.g. cats, and, are, pets). The context vector of “dogs” brings together all words adjacent to “dogs”, and by doing so, indirectly bring the “dogs” word vector close to them - i.e. word vectors for cats, pets etc., get close to dogs through their context vectors (in code stub, syn0 contains the word vectors, and syn1neg contains the context vectors).The image above shows the training for the sentence “cats and dogs are pets”. The word vectors (in syn0 array in code) are trained to get closer to the image(context) vectors (syn1neg) of words in neighborhood. So the “cats” word vector influences, and is influenced by, the image vectors of its neighboring words, bringing them closer to each other. The word “humans” (not shown in above figure) gets close to “dogs” because the context vector for “pets” that co-occurs with “humans” in second line in toy corpus, brings “humans” close to “dogs”.As an aside, this working of word2vec - starting with randomly pointing vectors, and generating clusters of related words by pulling and pushing vectors apart, which in essence is just vector addition and subtraction, is reminiscent, even if perhaps only superficially, of the path integral formalism for explaining light (QED: The Strange Theory of Light and Matter Richard P. Feynman). For example, add amplitude vectors of all possible virtual paths light can travel - the virtual paths all neatly add and cancel, and magically, one gets the path that light will indeed travel through a lens, or the path light will traverse across regions of different types of matter ( land to water - refraction) or same matter type but with differing densities ( hot/cold air - mirages)…
What is the meaning of stratified cluster sampling?
http://www.sgim.org/File Library/JGIM/Web Only/BMJ..Explanation for Stratified Cluster Sampling“The aim of the study was to assess whether the famine scale proposed by Howe and Devereux provided a suitable definition of famine to guide future humanitarian response, funding, and accountability. A cross sectional study design was applied. The scale and severity of the humanitarian crisis in Niger in 2005 would probably have varied across the country. It was therefore imperative that any sample was representative of the population of Niger.Simple random sampling across the country could have been used to recruit households. However, simple random sampling would have produced a representative sample only if enough households were recruited. The population of Niger was geographically diverse. Therefore, random sampling of households across the country would have been impractical and too expensive.A stratified two stage cluster sampling approach was therefore used to ensure the resulting sample was representative of the country, while concentrating resources in fewer areas (a is true). The stratified cluster sampling approach incorporated a combination of stratified and cluster sampling methods. Firstly, Niger was stratified by region.The country consists of eight regions—seven rural ones plus the capital, Niamey. Within each region a simple random sample could have been taken to ensure that each region was adequately represented. However, the population of each region was geographically diverse. Therefore, simple random sampling within each region or stratum would have been impractical and expensive. To concentrate resources in fewer places, a two stage cluster sampling process was performed within each stratum. A cluster is a natural grouping of people—for example, towns, villages, schools, streets, and households. The sampling of clusters in the above study was a two stage process. The first stage of cluster sampling involved a random sample of 26 villages within each stratum or region.The probability of selection was proportional to the population size of the region—that is, larger villages had a greater probability of being selected than smaller ones. Within each chosen village, a fixed number of 20 households were selected using systematic random sampling. The household was the unit of analysis, with a census of each household achieved through a questionnaire. The two stage cluster sampling process described above is referred to as a multistage cluster sampling approach, or simply multistage sampling. In multistage sampling, the resulting sample is obtained in two or more stages, with the nested or hierarchical structure of the members within the population being taken into account.Population members are arranged in clusters. The method is based on the random sampling of clusters at each stage, with the sampled clusters nested within the clusters sampled at the previous stage. In the example above, a two stage multistage sampling approach was used. The first stage involved random sampling of 26 villages within each region. The second stage involved the systematic random sampling of 20 households in each chosen village. The division of the country into regions was seen as stratification and not the first stage of a multistage sampling process (b is false). This is because all regions in the country were included and no random sampling of the regions took place.The cluster sampling of villages within each stratum involved the construction of the sampling frame—that is, a list of all villages within each region. However, presumably it was not possible to list all the households in each chosen village. Therefore, households in a village were selected using systematic random sampling, which does not depend on a sampling frame (c is false). This involved selecting a single household in a village at random, with households then chosen at regular intervals thereafter—for example, every fifth household. Systematic sampling is typically considered to be a random sampling method, as long as the starting point is random and the periodic interval of selecting participants is determined before sampling takes place.There are two types of sampling methods—probability sampling (also known as random sampling) and non-probability sampling (also known as non-random sampling). By definition, probability sampling methods involve some form of random selection of the population members, with each population member having a known and typically equal probability of being selected. For a non-probability sampling method, the probability of selection for each population member is not known. Although it is debatable, the method of stratified cluster sampling used above is probably best described as a non-probability sampling method.The villages in each region, and the households in each village, were chosen at random. It was possible to count the number of households in each chosen village. However, the number of households in each of the clusters that were not selected was not known. Hence the probability of selection of a household in the population could not be determined. Samples resulting from non-probability sampling methods are generally considered not to be representative of the population.However, there is no reason to think that the sample in the study above was not representative of the population—the sampling approach ensured that the resulting sample was representative of each region. If a sampling approach involves only a single stage of sampling of clusters it is referred to as cluster sampling. A random sample of clusters from the population is obtained and all members of the selected clusters are included in the resulting sample.After the selection of clusters, no further sampling takes place. Cluster sampling is often used to select participants for a trial—so called, cluster trials. Cluster trials have been described in a previous question. However, in such trials the clusters are typically not selected at random from the population but by using convenience sampling—that is, by selecting conveniently located clusters. Convenience sampling has been described in a previous question.1 Reza A, Tomczyk B, Aguayo VM, Zagré NM, Goumbi K, Blanton C, et al. Retrospective determination of whether famine existed in Niger, 2005: two stage cluster survey. BMJ 2008;337:a1622.2 Howe P, Devereux S. Famine intensity and magnitude scales: a proposal for an instrumental definition of famine. Disasters 2004;28:353-72.3 Sedgwick P. Cluster randomised controlled trials. BMJ 2012;345:e4654.4 Sedgwick P. Convenience sampling. BMJ 2013;347:f6304. BMJ 2013;347:f7016.”
- Home >
- Catalog >
- Miscellaneous >
- Manual Sample >
- Programming Instructions Manual Sample >
- Combining Probability And Non Probability Sampling Methods