How to Edit The Activity 1 How To Design Your Presentation - Technology Learning quickly and easily Online
Start on editing, signing and sharing your Activity 1 How To Design Your Presentation - Technology Learning online following these easy steps:
- click the Get Form or Get Form Now button on the current page to jump to the PDF editor.
- hold on a second before the Activity 1 How To Design Your Presentation - Technology Learning is loaded
- Use the tools in the top toolbar to edit the file, and the edits will be saved automatically
- Download your modified file.
A top-rated Tool to Edit and Sign the Activity 1 How To Design Your Presentation - Technology Learning
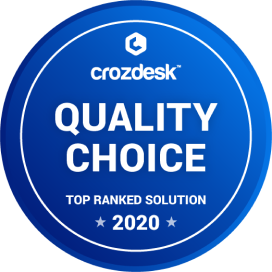
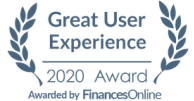
Start editing a Activity 1 How To Design Your Presentation - Technology Learning in a minute
Get FormA clear guide on editing Activity 1 How To Design Your Presentation - Technology Learning Online
It has become much easier nowadays to edit your PDF files online, and CocoDoc is the best online tool you have ever seen to make a series of changes to your file and save it. Follow our simple tutorial to start!
- Click the Get Form or Get Form Now button on the current page to start modifying your PDF
- Add, modify or erase your text using the editing tools on the tool pane on the top.
- Affter editing your content, put the date on and create a signature to make a perfect completion.
- Go over it agian your form before you click on the button to download it
How to add a signature on your Activity 1 How To Design Your Presentation - Technology Learning
Though most people are in the habit of signing paper documents using a pen, electronic signatures are becoming more usual, follow these steps to sign PDF online for free!
- Click the Get Form or Get Form Now button to begin editing on Activity 1 How To Design Your Presentation - Technology Learning in CocoDoc PDF editor.
- Click on the Sign icon in the toolbar on the top
- A box will pop up, click Add new signature button and you'll have three ways—Type, Draw, and Upload. Once you're done, click the Save button.
- Move and settle the signature inside your PDF file
How to add a textbox on your Activity 1 How To Design Your Presentation - Technology Learning
If you have the need to add a text box on your PDF and customize your own content, take a few easy steps to complete it.
- Open the PDF file in CocoDoc PDF editor.
- Click Text Box on the top toolbar and move your mouse to carry it wherever you want to put it.
- Fill in the content you need to insert. After you’ve filled in the text, you can take full use of the text editing tools to resize, color or bold the text.
- When you're done, click OK to save it. If you’re not settle for the text, click on the trash can icon to delete it and take up again.
An easy guide to Edit Your Activity 1 How To Design Your Presentation - Technology Learning on G Suite
If you are seeking a solution for PDF editing on G suite, CocoDoc PDF editor is a recommendable tool that can be used directly from Google Drive to create or edit files.
- Find CocoDoc PDF editor and set up the add-on for google drive.
- Right-click on a chosen file in your Google Drive and choose Open With.
- Select CocoDoc PDF on the popup list to open your file with and give CocoDoc access to your google account.
- Make changes to PDF files, adding text, images, editing existing text, mark up in highlight, trim up the text in CocoDoc PDF editor before saving and downloading it.
PDF Editor FAQ
What makes Rails a framework worth learning in 2017?
The same reasons why it was a framework worth learning in 2004. The more things change, the more they stay the same. While we’ve seen a lot a progress in the JavaScript world, we’ve also seen a regression to the complexity-laden world that Rails offered refuge from in the early days.Back then the complexity merchant of choice was J2EE, but the complaints are uncannily similar to those leveled against JavaScript today. That people spent hours, if not days, just setting up the skeletons. The basic build configurations. Assembling and cherry-picking from lots of little libraries and frameworks to put together their own snowflake house variety.The core premise of Rails remains in many ways as controversial today as it was when it premiered. That by formalizing conventions, eliminating valueless choices, and offering a full-stack framework that provides great defaults for anyone who wants to create a complete application, we can make dramatic strides of productivity.It’s somewhat surprising to me that despite the astounding success of Rails, that there haven’t been more competition for this proposition. The vast majority of activity today is for yet another option on the a la carte menu. Yet another build system, yet another view library, yet another ORM. Very little activity in integrated solutions.I guess the answer is that the foundational proposition of Rails continues to cut against the psychological grain of most programmers. That by reducing choices and accepting community conventions and answers to most of the basic questions in web development, you end up better off. Less unique, less tailored, but in ways that just don’t matter anyway.Anyway, that’s the big ideological appeal of Rails. I’ve elaborated further on convention over configuration, the a la carte/omakase conflict, the appeal of integrated systems, and other core values of the Rails community in The Rails Doctrine.After reading that, you’ll probably have a pretty good idea as whether Rails is something for you or not. If you can’t recognize any of the struggles outlined in that document, or you just don’t like the solutions presented to those struggles, the particulars of Rails technology probably doesn’t matter much. If that document resonates, or at least piques your interest, read on.On top of these ideological choices, we’ve built an incredibly pragmatic and multi-paradigm web framework. When people hear “web framework”, they sometimes thinks that “oh, that’s just some stuff to generate HTML, right?”. And in that definition, some might see it as though Rails competes against something like React. And I suppose it does, but in a very remote way that isn’t very useful to thinking about whether Rails is right for you or not.As I talked about above, Rails has an incredibly ambitious mission. In the full-stack goal lies a pursuit to deal with just about every piece of code needed to connect databases and no-sql stores to a business domain model written in Ruby to a set of controllers that expose that model via REST and then, yes, finally to HTML. But that last step is a small minority of the code and focus of Rails.So if you think that client-side MVC, React, Angular, or whatever is The Future, then you’re still squarely in the target audience for using Rails. Because the bits you use to design your HTML/JavaScript-based UI still needs to connect to a back-end domain model that saves stuff to the databases, computes things, enqueues jobs for later processing, sends out emails, triggers push notifications, and all the other stuff that real apps need to do.And that’s where the meat of Rails sits. In what happens once that POST or PUT or GET is triggered. Now, as I said, Rails is full-stack by default. So of course we also include answers for how to generate and update HTML. We have some phenomenally productive answers in Turbolinks and SJR, but even if that path doesn’t appeal, everything that leads up to generating that JSON is still stuff we’ll have in common.Anyway. That’s a very long pitch for two basic tenets of Rails appeal in 2017: 1) We have a unique ideological foundation that’s still controversial today and offers the same benefits against the mainstream choices as it did 13 years ago, 2) We have a pragmatic, full-stack answer that could be formulated based on that ideology that still offers amazing productivity from the second you run the rails new command.Oh, and on top of all that, I’ve saved the cherry for last. You get to use Ruby, which, even in a world that has rediscovered the benefits of functional programming and immutability, remains the most extraordinarily beautiful and luxurious language I’ve yet to encounter. Just look at some code. I dare you not to fall in love.
What should I study or learn if I want to be a data analyst for a software company like Quora, Zynga, Airbnb, etc.?
Updated Aug 2018The following sections will outline five skills that will help you further a career as a Data Analyst:Data Exploration via Excel/Google SheetsData Extraction with SQLData Visualization via TableauData Automation via PythonData Analysis/Science with Python + Stat librariesWho this is for - College students, new graduates, career changers, and new analysts will probably benefit most from this article. It assumes you have minimal analytics, programming, or work experience. This article should help you build a foundation so you can begin or further a career in data analytics.Who I am - I’m a self-taught analyst who has worked at various companies (Netflix, CNET, Zynga) in a variety of analytical roles (Marketing, Finance, Social, Growth) for over a decade.Two notes before proceeding:This article will not outline how to become a data scientist or data engineer (read more about the differences), which generally require degree(s) in statistics or computer science respectfully.While you can learn these in any order, you’ll probably progress most seamlessly by starting with #1 and #2 before #3–51. Data Exploration via Excel / Google SheetsAt most organizations, Microsoft Excel and/or Google Sheets are the most broadly used data applications. While many tools perform a specific function very well (such as Tableau for visualization), few can enable most lightweight data tasks as easily as a spreadsheet. Not only are Gsheets/Excel the Swiss Army knives of data exploration, they also have a relatively shallow learning curve, which make either a great tool to learn first. If you’re dead-set on other analysts skills, don’t spend too much time here--but don’t make the mistake of not becoming familiar with a spreadsheet program either. Many data questions can be answered and communicated with a spreadsheet faster than with other technologies.Start by learning the following:FormulasGeneral Formulas. Once you’ve downloaded the data, see if you can enhance it with some formulas. The IF statement, boolean logic (AND, OR), and VLOOKUP functions are the most common formulas used across spreadsheets. Afterward, graduate to learning text-based formulas like MID, LEFT/RIGHT, SUBSTITUTE, TRIM. Experiment with the date formulas--such as converting a date (in any format) to the components of a date (year, month, day).Formula References. You should know the difference between an absolute and a relative reference as well as how to input either via editing a formula using the keyboard (F2) as well as toggling either (F4) via the keyboard.Aggregation Formulas. These formulas help you find conditional summary level statistics: SUMIF(s) , COUNTIF(s), and SUMPRODUCT, which are good to learn for reporting purposesInterested in learning more formulas? See this article.Data Filter. The data filter is a key feature which helps end users, sort, filter, and understand a sample from a large data set. Memorize the keyboard shortcut for creating one--you’ll use this often.Pivot Tables. Pivot tables allow an end users to easily get summary level statistics for a given dataset. Learn how to create a pivot table, and scenarios in which to place fields or metrics in the row, column, filter, or value section. Learn how to create formulas at the pivot table level, and understand how creating them on a pivot table is different than at the data table level. Finally, learn the GETPIVOTDATA function, which is especially useful when creating dashboardsCharting and Pivot Charting. Lean how to create bar, line, scatter, and other charts in Excel. Formatting charts is relatively easy--when you want to change something click on it (or right click), and in general the Excel Ribbon or the right click menu will allow you to modify the look and feel of a chart within the ribbon or or menu.Keyboard Shortcuts. As you begin to get more comfortable, begin mastering the keyboard shortcuts rather than use the mouse. Start by learning the basic shortcuts for tactics like find and replace and paste special. Then move onto to navigating using the keypad. Experiment with selecting rows and columns by using a combination of shift and control. You should eventually learn how to add rows/columns, hide rows/columns, delete rows/columns--all by using the keyboard.Excel Dashboard Design. Learn the Data → Pivot → Presentation pattern, in which one separates the source data from summarized data, and summarized data from the viewable dashboard. This pattern will allow you to easily update a report as more data comes in as well as hide complexity from those who just want to see the most important learnings. How? The first tab contains your data, which you should ideally not change. The second tab contains one or many pivot tables that calculate summary statistics needed for the report. The third tab is a dashboard with one or many visuals or data tables that source data primarily from the second tab (and not from first tab). You’ll present just the third tab to end users, but hide the first and second tabs. When displaying summary level statistics, you’ll likely leverage GETPIVOTDATA—instead of using other summary formulas—will has a faster runtime than the summary formulas. This article explains how to create a dashboard using GETPIVOTDATA such that an end user can select various input options and see a visualization change---Some notes:Excel or Google Sheets? Google Sheets performs best with smaller datasets (<10k rows). It’s also free. Out of the box, Gsheets is also more collaborative, and a good solution if your dataset will be viewed or modified by multiple stakeholders. For larger datasets, spreadsheets with lots of formulas, or the use of esoteric features, Excel is usually the preferred optionDon’t learn Excel VBA. If you’re interested in programming, skip to the Data Programming section and consider Python instead.2. Data Extraction with SQLExcel allows you to slice and dice data, but it assumes you have the data readily available. As you become a more seasoned analyst, you'll find that a better way to get at data is to pull it directly from the source, which often means authoring SQL.The great news about SQL is that unlike a procedural based programming language like Python, SQL is a declarative language. In most cases, instead of writing step-by-step syntax to perform an operation, you describe what you want. As a result, you should be able to learn SQL faster than learning most programming languages.I’m not going to outline all of the flavors of data storage solutions (to start, learn about relational vs non-relational databases) but instead focus on what you’re most likely to encounter--a relational database which supports some flavor of SQL.Start by learning the big six reserved keywords:SELECTFROMWHEREGROUP BYHAVINGORDER BYNext, you’ll want to learn common sql functions, such as the CASE statement, boolean operators (AND, OR, NOT), and IFNULL/COALESCE. Next, learn string functions such as INSTR, SUBSTR, and REPLACE.As you begin to write summary level queries which use the GROUP BY keyword, experiment with the aggregate functions such as SUM, COUNT, MIN, and MAX. Following that, learn how to join to other tables. Know the difference between an inner and outer join.Next, take a break from writing SQL and invest in learning more about how relational databases are structured. Know the difference between a fact and dimension table, understand why database indexes (or partitions) are leveraged, and read about why traditional database adhere to 1st, 2nd, and 3rd normal forms. If someone says they have a high cardinality dataset, a snowflaked schema, or slowly changing dimension--you should know what they mean.As you work with larger datasets, you’ll discover that more involved SQL queries require issuing several SQL queries in sequence. For example, the first query may create a table; the second one will insert data into that table; and the third will extract such data. To get started here, read more about temporary tables. Then you’ll want to learn about column data types as well as how to create traditional database tables and indexes/partitions to support more performant querying.---Some notes:SQL Bolt has a great interactive tutorial to help you learn SQL by doingToptal’s top SQL interview questions can help you get your next job that requires knowing SQLThis section only covered data extraction. As you become more senior, you’ll need to know how to build intermediary tables for analysis, or even construct source tables to store non-temporal data. Read more about SQL DML and DDLIf you’re interested in learning more about dimensional modeling, purchase Kimball’s The Data Warehouse Toolkit, which was originally published in 1996 but still relevant for traditional relational databases today.Try creating your own database locally by downloading and installing mysql or postrgres. Or do so via google cloud.This section only covered relational databases. See this article to learn more about non-relational databases3. Data Visualization via TableauIn the past decade, Tableau has become the leading enterprise tool for visualization. If you’re familiar with pivot tables, you’ll find that creating lightweight visualizations and dashboards with Tableau is relatively easy. To spreadsheet users, Tableau feels like working with an enterprise version of Pivot Tables and Pivot Charts. While keeping your analyses private requires a purchased Tableau Desktop license, Tableau public--which stores any saved analyses to the publicly accessible Tableau portal--is free and a great way to get started learning.Let’s start with Tableau Public--begin by creating an account and downloading the software, and then import a dataset into Tableau. Next, learn more about the panels within the tool. You’ll see the data you’ve added broken up into Dimensions and Measures. Try dragging a given dimension into the columns shelf, and a given measure into the Rows shelf. Tableau will analyze the structure of your data, and automatically generate a visualization (without you selecting one). You can easily change the visualization displayed by changing the type, or by shifting the data between Rows and Columns.After you’ve created a couple of different visualizations across multiple worksheets, create a dashboard. A dashboard can contain one or many views (worksheets) and also allow an end user to manipulate such a view via buttons, filters, and other controls. Start by adding one view to your new Dashboard. Then, add a Filter for a given measure or dimension. Once added, you can change the nature of each filter. For example, you can create a slider to change the range of dates included, or add a radio form to allow an end user to select a given measure. Once you have a functional dashboard, feel free to save it to Tableau Public so you can both view it as an external user would as well as modify it later. For inspiration, see some existing dashboards.From here, there’s a lot more you can do and learn. Tableau’s learning curve quickly steepens as you produce more advanced visualizations and deal with more complex datasets. If you want to continue learning, your best bet is to watch Tableau’s series of free training videos.---Some notes:While Tableau is the current Enterprise visualization market leader, it may not be five years from now. Tableau started as a desktop application and then grew to support web-based reporting, and now many upstarts are producing Tableau-like tools that are 100% browser based (See alternatives to Tableau), responsive by default, and built to work in the cloud as well as integrate with other sources.4. Data Programming via PythonNow you can source data from a database with SQL, manipulate it with a spreadsheet, and publish visualizations via a Tableau dashboard. A next natural step is to learn a programming language. Python is the most utilized programming language in the data community as well as the most common language taught at universities. With it you can achieve a number of data-related tasks such as extracting data from a website, loading said data into a database, and emailing the results of a SQL select statement to a set of stakeholders. If you’re interested in building web application, you could use Python and Flask to create an API as well as create a website leveraging the Flask HTML templating engine Jinja2. Or, you can leverage Python Notebooks for iterative development, the PANDAS library to see the results of a model you’re building as you develop it.The best way to build a strong programming foundation is to start by learning computer science fundamentals. For example, I was introduced to many computer programming concepts via the book Structure and Interpretation of Computer Programs (SICP) at university. Although originally authored in 1979, the book’s concepts are still relevant today and are still leverage today used at UC Berkeley to teach introductory computer science. Once you learn many of the fundamentals, you should be able to apply them to learn any computer programming language. However, learning the fundamentals can take a lot of time--and the content in SICP is academically dense (this review describes it well). Sometimes the better tactic to get started is to learn by doing.I learned python syntax years ago via Learn Python the Hard Way. The online course costs $30 now--and there are plenty of other free alternatives--but when I took the course (at the time it was free), I found it to be one of the better tutorials for learning the Python syntax. If you’re looking for a free option, head to Learn Python or Code Academy.You will have covered python basics when you’re familiar with python variables, control-flow, data structures (lists, dictionaries), classes, inheritance, and encapsulation. A good way to solidify your knowledge is to think of a project you’d like to implement and begin developing—this site has a couple of datasets that you can use to get started.Now that you have the basics down, you’ll want to learn more about how to become a more productive programmer by improving your development environment. The next three sub-sections will cover how to save/share/iterate your work using Github, author Python scripts using Jupyter Notebooks, and make changes to projects using the command line.4a. Learn version control using GitHub/git.GitHub allows you to host, update, document, and share your projects easily online. You’ll soon discover that GitHub will likely be where you end up when you’re discovering new programming libraries. Start by creating a GitHub account (almost all developers have one). Then spend time iterating through the GitHub tutorials, which will outline all of the capabilities of git. Once complete, you should be familiar with how to git clone an existing repository, how to create a new repository, git add files to a commit, prepare a set of changes with git commit, and push changes to a branch via git push. As you invest time in any project, make a habit of committing it to github to ensure that you won’t lose your work. You’ll know that you’re progressing with git once you feel comfortable using the above commands for both managing your own projects as well as cloning other projects to augment your development efforts.4b. Author Python scripts using Jupyter Notebooks As you’re learning Python, you’ll discover that there are multiple ways to author python code. Some developers will use IDEs built specifically for programming such as PyCharm, others elect rich text editors with a focus specifically on coding such as Sublime, and a small minority will edit code exclusively through a shell using VIM. Increasingly, data professionals are gravitating toward using notebooks--specifically Jupyter Notebooks--to author scripts in a web browser for exploration purposes. A key feature within notebooks is the ability to execute code blocks within each notebook rather than all at once, allowing the developer to gradually tweak a data analaysis. Moreover, since the output is in the web browser versus a shell, notebooks can display rich outputs, such as an annotated datatable or timeseries graph beneath the code that generated it. This is incredibly helpful when you’re writing a script to perform a data task and want to see the progress of our script as it executes without leaving the browser.There are a variety of ways to get started with Notebooks. One way is to download Jupyter and run an instance on your local machine. Another option is to use Google’s free version of notebooks or Microsoft Azure Notebooks. I prefer to use notebooks hosted on pythonanywhere, which is the same service I use to host python-based web applications. The free service will let you create your own python apps but you can’t run notebooks--the most affordable tier is $5/month.A good way to learn some of the key value adds of developing with Notebooks is to explore a dataset using the Python Data Analyst library, PANDAS. This site has a great getting started tutorial. Start by importing a dataset and print it out. Learn more about the data-frame storage structure, and then apply functions to it just like you would with another dataset. Filter, sort, group by, and run regressions. Try leveraging seaborn, a statistical visualization library which leverages matplotlib to explore your datasets visually. You’ll quickly discover that the framework allows for repeatable data operations with option for data exploration against a moderate cardinality dataset. Notebooks are often the preferred prototyping interface for data scientists, and thus worth learning how to use if you’re interested in learning more about statistics.4c. The Command Line - using shells and editing with vimIf you’ve read this far, you’ve probably already used a shell, a command-line based user interface for interacting with a computer. You’ve likely used shells to execute python code, download code libraries, and commit changes to git. Knowing how to execute a file, navigate within a shell, and monitor an active process will help you become a stronger data analyst. A great place to learn more about shells is following this interactive tutorial. You know that you’re becoming more proficient with shells when you can easily navigate within a directory, create aliases, change file permissions, search for files and/or contents using grep, and view the head/tail of a file.VIM is a unix-originated command-line text editor which is run in a shell. It’s especially useful when you want to view or edit a file—such as a log or a data output—on a remote server. Initially, you’ll likely find that learning VIM is a bit cumbersome because you primarily interact with the application without a mouse. However, over time you’ll begin to develop the muscle memory needed to toggle between edit-mode, view-mode, and executing commands. A great place to get started with VIM is to go through this interactive tutorial. You’ll know that you’re becoming more comfortable with VIM once you can easily navigate between input and edit mode, go to a row by a number, add or delete a row or character, search and replace text, and easily exit and save files you’ve edited.5. Data Analysis/Science with Python + Stat librariesWhile the goal of this article is not to describe how to be a data scientist--that typically requires a undergraduate and/or graduate level education in statistics--having a solid foundation in statistics will help any analyst make statistically sound inferences from most data sets.One way to get started is to take an online course in descriptive statistics--such as this free one from Udacity--which will teach you how to communicate summarized observations from a sample dataset. While you may be tempted to jump to other hotter industry topics such as machine learning, start with the basics. A solid foundation in descriptive statistics is a prerequisite for machine learning as well as many other statistics applications. After going through Udacity or other tutorials, you should be able to describe various types of distributions, identify skews, and how to describe central tendency, variance, and standard deviation.Next up, graduate to learning inferential statistics (such as Udacity’s free course), which will enable you to draw conclusions by making inferences from a sample (or samples) of a population. Regardless with the learning path you take, you should learn how to develop hypothesis as well as become familiar with tactics for validating such hypothesis using t-tests, understand when to leverage different types of experiments, as well as compute a basic linear regression with one more more dependent variables.The two most popular languages for applying statistics are R and Python. If you’re just getting started, I’d recommend using Python over R. Python is generally considered an easier language to learn. Moreover, Python is typically understood by most teams who build data products. There are more libraries available in Python that can be applied to a wider set of data applications--such as deploying a website or creating an api. This means you can often start an exploratory analysis in Python and easily append a few more libraries to deploy a tool / product leveraging such data, which can reduce the time to release. Finally, data applications continue to gravitate to Python over R as the preferred applied statistics language, so by learning the statistical libraries on Python you’ll be riding this latest adoption trend.Regardless of which language you choose, both Python and R can be executed via Jupyter Notebooks, which allow for more easy visualization and communication as you’re getting started.Next, try learning more about machine learning (Udacity’s free ML course is here). Following any course you should be more familiar with how to differentiate a supervised vs unsupervised learning, understand bayes theorem and how it’s used in ML applications, and outline when decision trees are leveraged. Once you’ve learned the concepts, try cementing your understanding by implementing one of these 8 machine learning projects.Finally, Python has a wealth of free libraries commonly leveraged by data scientists. One way to become more familiar with data scientist tactics are to try experimenting with data science libraries. For example, scikit-learn provides standard algorithms for machine learning applications, and NLTK is a library which can help you process and analysis text using NLP.Wrap UpNow you can write a python script to extract data (#4), store it in a database with SQL (#2), build a model to predict future observations with a python data science library (#5), and share what you learn via a spreadsheet (#1) or a Tableau Dashboard (#5). During that process, you may have committed your code to git, authored in a Jupyter Notebook, and published it on your python-hosted server. Congratulations! You’re well on your way to becoming a data analyst.
What is the purpose of getting a PhD if you do not want to have a research career?
I will give very practical answer. The purpose of getting a PhD if you do not want to have a research career would be to get following skills. I can say for myself that I would not have developed these skills if I had not done PhD.You learn how to do research for anything. Even if you did PhD in some particular subject or field, you actually learn how to do research of almost anything. In your own PhD you would have mastered skills and topics from disparate areas in which you never had any inkling about before (For example, it is quite common to read and assimilate works of physicists or mathematicians even if you trained only as an engineer). You understand the basic process of doing research and you can easily apply this to anything else in life. This helps you immensely for rest of your life.You learn how to manage your project. You learn to design, organize and implement the project. You will learn to compete against ever expanding goalposts (any research discovery shifts the goalpost even further). This will develop seriously good skills in managing other things in your life.You learn oral and written communication skills. Summarizing your research and presenting it in technical language develops oral and written communication skills as you get opportunities to write journal papers and present at conferences. This helps in presenting anything else in simple language to others.You learn patience and hardwork. You learn to be open minded, not assuming things, not to have bias and learn to interact with others.You learn to wonder at greatness of inventions and the amazing impact science and technology has created. You can learn about innovations and creative ability and can imbibe these skills in yourself, this will help you greatly in improving your personality.You go through rigor of achieving success through struggle and hardships during PhD. It will definitely give you learning for lifetime.If you are able to get only teaching job somewhere, then you may get more free time to pursue your other activities of interest. This is a practical advantage and not a skill.You will be definitely a better and more skilled person once you finish PhD than you were before. However, this was the answer to what could be the other purposes to do a PhD, one should look at all practical aspects before deciding to do a PhD. I would not advice anyone to do a PhD if he/she does not have inclination to have a research career. But yes, there are some high level jobs like consultant or as editor or as adviser in organizations where you can find a career with PhD.
- Home >
- Catalog >
- Miscellaneous >
- Powerpoint Templates >
- Simple Powerpoint Templates >
- simple ppt templates for project presentation >
- Activity 1 How To Design Your Presentation - Technology Learning