How to Edit The S List Tracking And Distribution Of Any Potential quickly and easily Online
Start on editing, signing and sharing your S List Tracking And Distribution Of Any Potential online refering to these easy steps:
- click the Get Form or Get Form Now button on the current page to jump to the PDF editor.
- hold on a second before the S List Tracking And Distribution Of Any Potential is loaded
- Use the tools in the top toolbar to edit the file, and the added content will be saved automatically
- Download your modified file.
A top-rated Tool to Edit and Sign the S List Tracking And Distribution Of Any Potential
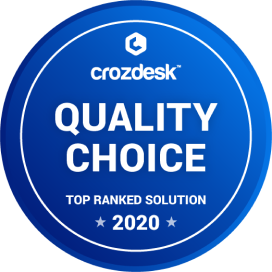
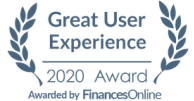
Start editing a S List Tracking And Distribution Of Any Potential right now
Get FormA clear guide on editing S List Tracking And Distribution Of Any Potential Online
It has become quite easy just recently to edit your PDF files online, and CocoDoc is the best free app you have ever used to make a lot of changes to your file and save it. Follow our simple tutorial to try it!
- Click the Get Form or Get Form Now button on the current page to start modifying your PDF
- Add, modify or erase your text using the editing tools on the toolbar on the top.
- Affter editing your content, put the date on and make a signature to complete it.
- Go over it agian your form before you click on the button to download it
How to add a signature on your S List Tracking And Distribution Of Any Potential
Though most people are in the habit of signing paper documents by handwriting, electronic signatures are becoming more normal, follow these steps to sign documents online for free!
- Click the Get Form or Get Form Now button to begin editing on S List Tracking And Distribution Of Any Potential in CocoDoc PDF editor.
- Click on the Sign icon in the tool box on the top
- A box will pop up, click Add new signature button and you'll have three ways—Type, Draw, and Upload. Once you're done, click the Save button.
- Move and settle the signature inside your PDF file
How to add a textbox on your S List Tracking And Distribution Of Any Potential
If you have the need to add a text box on your PDF and customize your own content, take a few easy steps to accomplish it.
- Open the PDF file in CocoDoc PDF editor.
- Click Text Box on the top toolbar and move your mouse to carry it wherever you want to put it.
- Fill in the content you need to insert. After you’ve input the text, you can use the text editing tools to resize, color or bold the text.
- When you're done, click OK to save it. If you’re not settle for the text, click on the trash can icon to delete it and take up again.
An easy guide to Edit Your S List Tracking And Distribution Of Any Potential on G Suite
If you are seeking a solution for PDF editing on G suite, CocoDoc PDF editor is a recommended tool that can be used directly from Google Drive to create or edit files.
- Find CocoDoc PDF editor and set up the add-on for google drive.
- Right-click on a chosen file in your Google Drive and choose Open With.
- Select CocoDoc PDF on the popup list to open your file with and give CocoDoc access to your google account.
- Make changes to PDF files, adding text, images, editing existing text, mark up in highlight, fullly polish the texts in CocoDoc PDF editor before saving and downloading it.
PDF Editor FAQ
A potential investor just asked to see my SWOT analysis. Is this normal?
It's certainly a legitimate question as part of due diligence. If you don't have one, take the time to think it through and develop one. It's a good thing for you to consider.(By the way, to put things in perspective, here's a typical diligence checklist that might be requested from a venture fund, super angel or an organized angel investor group):Company OverviewArticulate your company’s “equity story” (i.e. why you are on to something and why your stock will appreciate greatly).Discuss company history as well as key milestones achieved since inception.Provide your internal business planning document(s).Provide and discuss any internally generated reports used to monitor and measure operating and fiscal performance.Identify the 3—5 most important challenges going forward.Management TeamPlease provide résumés and a list of business references (with contact information) for each key member of the management team.Summarize the key responsibilities of each member of the management team.Are there any key management positions open? If so, how will you fill them (headhunter, personal contacts, etc.)?How many total employees do you have now and how do you forecast this to grow over the next five years?Have any members of your management team left in the last 2 years and, if so, why? Please provide contact information for these individuals.List the Board of Directors with board positions held, terms of office and compensation. Please provide contact information and short bios for each board member.Market Size and Other MarketingPlease describe your target market and quantify its current size and projected growth.Please describe the characteristics of companies/individuals in your target market?Provide your market research, if any.Please explain your marketing strategy and describe the assumptions underlying it. Compare your approach to that of your principal competitors.What market share do you anticipate and when will you capture it?How do you see your market evolving over the next 12 – 18 months? What trends are most important?Describe your long-term competitive advantage that competitors cannot easily replicate. How long will this advantage last?What are the major drivers of growth and profitability in your industry? What metrics do you watch to track them?Please describe your pricing strategy and your overall business model. What was your logic in structuring your business model the way you did?SalesPlease provide your top customers (with references), your sales backlog (firm contracts) and pipeline (sales prospects).Describe your direct and indirect sales channels and explain key factors in customer acquisition and customer retention.How do you motivate your sales force?Describe your sales cycle from sourcing a lead to closing a sale. What is your average cost to acquire a customer, average revenue per sale, and potential lifetime revenue per customer (for recurring revenue customers)?What is your value proposition to your customer and how do you communicate it? Please provide a copy of your sales pitch.If you sell to businesses, to whom do you sell in an organization (i.e. what titled position such as CTO, CMO, Sales Manager, etc.)?List your revenue targets for the next two years and describe the milestones or hurdles you will have to achieve to meet these targets.CompetitionPlease analyze your key direct and indirect competitors, including the products and services provided, comparative prices and quality, market share data and perceived competitive advantages.What is your IP position, relative to your competitors?Product DevelopmentProvide a detailed product description.Detail the history of the company’s technology and product development. How much has been spent on development? What has been licensed from third parties?How has market research guided your product development?What mechanisms (both formal and informal) do you have to collect feedback from customers? Provide examples of how customer feedback has influenced product design.Have there been any delays in developing your technologies and, if so, what have the causes of these delays been?Intellectual PropertyPlease provide U.S. and European patents, patent applications and CIPs.Provide U.S. and European trademarks and trademark applications.Have any employees signed NDAs? If so, please list them and provide a complete list of employees/consultants who have not signed non-disclosure agreements while performing work for the company.Has your company ever been accused of infringing on the intellectual property of other companies, or have you ever accused others of infringing on your IP? If so, please provide details.Production / OperationsPlease describe your compensation system, including salary ranges, bonuses, commissions and benefits.What stock option plans or other employee equity participation strategies do you have? What percentage of the company are you reserving for management?Provide summary of the vesting schedules of any stock or options, subject to vesting.Do you employ consultants or contract labor? If so, in what capacity?Describe how you handle post-sales customer support?What systems exist for customer support?What support options are available to customers?How do you handle fulfillment and logistics of distribution?What are your return policies and how you process returns?How do you track and manage inventory? Please describe how your inventory system interfaces with your cash management system.Please detail your data redundency and disaster recovery strategy.What accounting systems/procedures do you employ?How do you handle HR administration? Please provide an overview of your employee benefits package.Have you fully or partially implemented Sarbanes-Oxley compliance?Financing StrategyProvide a full capitalization table showing fully-diluted shares, notes, warrants, option pools (issued and available).List of all investors, contact information, number of shares issued, issuance date and price.Explain the company’s use of funds from this round in detail, including assumptions behind line item estimates.What are your future financing needs (amount and timing) for both debt and equity? When do you become EBITDA and cash flow positive?FinancialsProvide any historical audited financial statements (balance sheet, income statement, cash flow statement).Provide a full financial model (balance sheet, income statement, cash flow statement) and detailed discussion of the key assumptions therein. Include trailing 12-month results by month, projections for the next 12 months (including gross and net burn rates) and annual projections for the next five years.
What are probabilistic graphical models, and why are they useful?
These are Probabilistic Graphical Models. They are arguably our most complete and promising toolkit for inferring truth from complexity. They're born from a single set of principles that endow our machines to dominate chess, diagnose disease, translate language, decipher sound, recognize images and drive cars. 'Neural Networks' and 'Probabilistic Programming' are famous signatures of the Machine Learning community simply because they are effective tool sets for applying these devices.My aim here is to reveal the machinery behind this magic. I intend to show what they are, why we use them and how we actually use them. To do that, I’ve answered seven questions on this subject:What are Probabilistic Graphical Models and why are they useful?What is 'exact inference' in the context of Probabilistic Graphical Models?What is Variance Inference in the context of Probabilistic Graphical Models?How are Monte Carlo methods used to perform inference in Probabilistic Graphical Models?How are the parameters of a Bayesian Network learned?How are the parameters of a Markov Network learned?How is the graph structure of Probabilistic Graphical Models learned?I realize this is a ton to digest, especially for internet browsing, but allow me to sell you. This information is typically delivered with a worthwhile 1000+ page textbook to graduate computer scientists. We can 80/20 these ideas with just a few answers! It'll take discipline, but you'll gain a surprisingly good understanding of an absolutely foundational theory of Machine Learning.As a compromise, I've structured things such that you need only read a subset of these answers to get a full picture. Here's a map of that structure:For example, if you read [math]1 \rightarrow 2 \rightarrow 6 \rightarrow 7[/math], you'll get a complete taste. Also, I'll include refreshers at the beginning of each answer - this should make things more self contained. (If you read these answers in sequence, I'd skip those refreshers, as they will sound redundant.)If this sounds like a good deal to you, please follow those questions!Now, let's start walking.Notation GuideAs a first stop, we'll review notation, an admittedly boring place. But, it's my unconventional belief that most confusion is due to notation. So if we wish to survive, we'll need a few tips:An upper case non-bold letter indicates a single random variable ('RV'). The same letter lower cased with a super script indicates a specific value that RV may take. For example, [math]X=x^1[/math] is the event the RV [math]X[/math] took on the value [math]x^1[/math]. We call this event an assignment. The set of unique values an RV may take is [math]Val(X)[/math]. So we might have [math]Val(X)=\{x^0,x^1\}[/math] in this case.A bold upper case letter indicates a set of RVs (like [math]\mathbf{X}[/math]) and a bold lower case letter indicates a set of values they may take. For example, we may have [math]\mathbf{X}=\{A,B\}[/math] and [math]\mathbf{x}=\{a^3,b^1\}[/math]. Then the event [math]\mathbf{X}=\mathbf{x}[/math] is the event that [math]A=a^3[/math] happens and [math]B=b^1[/math] happens. Naturally, [math]Val(\mathbf{X})[/math] is the set of all possible unique joint assignments to the RVs in [math]\mathbf{X}[/math].If you see [math]\mathbf{x}[/math] (or [math]\mathbf{y}[/math] or [math]\mathbf{z}[/math] etc...) within a probability expression, like [math]P(\mathbf{x}|\cdots)[/math] or [math]P(\cdots|\mathbf{x})[/math], that's always an abbreviation of the event '[math]\mathbf{X}=\mathbf{x}[/math]'.Perhaps confusingly, we also abbreviate the event '[math]\mathbf{X}=\mathbf{x}[/math]' as '[math]\mathbf{X}[/math]', though this isn't a clean abbreviation. Omission of [math]\mathbf{x}[/math] means one of two things: either we mean this for any given [math]\mathbf{x}[/math] or for all possible [math]\mathbf{x}[/math]'s. As an example for the latter case, 'calculate [math]P(\mathbf{X})[/math]' would mean calculate the set of probabilities [math]P(\mathbf{X}=\mathbf{x})[/math] for all [math]\mathbf{x}\in Val(\mathbf{X})[/math].[math]\sum_\mathbf{X}f(\mathbf{X})[/math] is shorthand for [math]\sum_{\mathbf{x}\in Val(\mathbf{X})}f(\mathbf{X}=\mathbf{x})[/math]. This is similarly true for [math]\prod_\mathbf{X}(\cdot)[/math] and [math]\textrm{argmin}_\mathbf{X}(\cdot)[/math]. Look out for this one - it can sneak in there and change things considerably.You may see equations like [math]f(A,B,C)=g(\mathbf{X})h(\mathbf{Y})[/math]. They look strange - the RVs on the left aren't on the right! Well, in such cases, you also have something like [math]\mathbf{X} = \{A,B\}[/math] and [math]\mathbf{Y} = \{B,C\}[/math]. So the equation really is [math]f(A,B,C)=g(A,B)h(B,C)[/math].Probability distributions are references with a [math]P[/math], [math]\textrm{Q}[/math], [math]q[/math] or [math]\pi[/math]. Keep in mind that distributions are a special kind of function. Remember that!Everything is in reference to the discrete case. Unfortunately, the continuous case is not a simple generalization from the discrete case. The minor exception is in the visuals. The discrete case is less friendly to graphs, so I might use some continuous distributions. As it relates to the discussion, pretend these are in fact discrete distributions with a fine granularity.Almost all of this notation comes from the text Probabilistic Graphical Models - one of those 1000 page monsters. That book is extremely thorough, and should be considered stop number 8.Still here? You must have discipline! Onto the fun stuff - we ask:What generic problem do PGMs address?Our goal is to understand a complex system. We assume the complex system manifests as [math]n[/math] RVs, which we may write as [math]\mathcal{X} = \{X_1,X_2,\cdots,X_n\}[/math] [1][2]. We take it that 'a good understanding' means we can answer two types of questions accurately and efficiently for these RVs. If we say [math]\mathbf{Y}[/math] and [math]\mathbf{E}[/math] are two given subsets of [math]\mathcal{X}[/math], then those questions are:Probability Queries: Compute the probabilities [math]P(\mathbf{Y}|\mathbf{E}=\mathbf{e})[/math]. That is, what is the distribution of the RV's of [math]\mathbf{Y}[/math] given we have some observation of the RVs of [math]\mathbf{E}[/math]?MAP Queries: Determine [math]\textrm{argmax}_\mathbf{Y}P(\mathbf{Y}|\mathbf{E}=\mathbf{e})[/math]. That is, determine the most likely assignments of RVs given an assignment of other RVs.Before continuing, we should point a few things out:Since [math]\mathbf{Y}[/math] and [math]\mathbf{E}[/math] are any two subsets of [math]\mathcal{X}[/math], there is potentially a remaining set (call it [math]\mathbf{Z}[/math]) that's in [math]\mathcal{X}[/math]. In other words, [math]\mathbf{Z} = \mathcal{X} - \{\mathbf{Y},\mathbf{E}\}[/math]. This set appears left out of our questions, but is very much at play. We have to sum these RVs out, which can considerably complicate our calculations. For example, [math]P(\mathbf{y}|\mathbf{e})[/math] is actually [math]\sum_\mathbf{Z}P(\mathbf{y},\mathbf{Z}|\mathbf{e})[/math].We haven't mentioned any model yet. This set up is asking generically for probabilities and values that accurately track reality.To this end, we are assisted by the fact that we have some, at least partial, joint observations of [math]\mathcal{X}[/math]. However, some of our [math]n[/math] RVs may never be observed. These are called 'hidden' variables and they will complicate our lives later on.This set up is extremely general, and as such, this problem is extremely hard.The problem with joint distributions.Our starting point, perhaps surprisingly, will be to consider the joint distribution of our RVs [math]\mathcal{X}[/math], which we aren't given in real application (but we'll get there). We'll call that joint distribution [math]P[/math]. Conceptually, we can think of this as a table that lists out all possible joint assignments of [math]\mathcal{X}[/math] and their associated probabilities. So if [math]\mathcal{X}[/math] is made up of 10 RVs, each of which can take 1 of 100 values, this table has [math]100^{10}[/math] rows, each indicating a particular assignment of [math]\mathcal{X}[/math] and it's probability.The issue is, for a complex system, this table is too big. Even if we had the crystal ball luxury of having [math]P[/math], we can't handle it. So now what?The Conditional Independence statementWe need a compact representation of [math]P[/math] - something that gives us all the information of that table, but doesn’t involve writing it down. To this end, our saving grace is the Conditional Independence (CI) statement:Given subsets of RVs [math]\mathbf{X}[/math], [math]\mathbf{Y}[/math] and [math]\mathbf{Z}[/math] from [math]\mathcal{X}[/math], we say [math]\mathbf{X}[/math] is conditionally independent of [math]\mathbf{Y}[/math] given [math]\mathbf{Z}[/math] if[math]P(\mathbf{x},\mathbf{y}|\mathbf{z})=P(\mathbf{x}|\mathbf{z})P(\mathbf{y}|\mathbf{z})\tag*{}[/math]for all [math]\mathbf{x}\in Val(\mathbf{X})[/math], [math]\mathbf{y}\in Val(\mathbf{Y})[/math] and [math]\mathbf{z}\in Val(\mathbf{Z})[/math]. This is stated as '[math]P[/math] satisfies [math](\mathbf{X}\perp \mathbf{Y}|\mathbf{Z})[/math][3]'Now, if we had sufficient calculation abilities, we could calculate the left side and the right side for a distribution [math]P[/math]. If the equations hold for all values, then, by definition, the CI statement holds. Intuitively, though not obviously, this means that if you are given the assignment of [math]\mathbf{Z}[/math], then knowing the assignment of [math]\mathbf{X}[/math] will never help you guess [math]\mathbf{Y}[/math] (and vice versa). In other words, [math]\mathbf{X}[/math] provides no information for predicting [math]\mathbf{Y}[/math] beyond what [math]\mathbf{Z}[/math] has. Similarly, you can't predict [math]\mathbf{X}[/math] from [math]\mathbf{Y}[/math] any better.Knowing such statements turns out to be massively useful - they give us that compact representation we need. To see this, let's say [math](X_i \perp X_j)[/math] for all [math]i \in \{1,\cdots,10\}[/math] and [math]j \in \{1,\cdots,10\}[/math] where [math]i\neq j[/math]. This is to say, all RVs are independent of all other RVs. It turns out that with these statements, we only need to know the marginal probabilities of each value for each RV (which is a total of [math]10\cdot 100=1000[/math] values) and may reproduce all the probabilities of [math]P[/math]. So if we are considering the case where [math]\mathbf{X}=\mathcal{X}[/math] and would like to know the probability [math]P(\mathbf{X}=\mathbf{x})[/math], we simply return [math]\prod_{i=1}^{10}P(X_i=x_i)[/math], where [math]x_i[/math] is the [math]i[/math]-th element of [math]\mathbf{x}[/math].Though this isn't just a save on storage. This is a simplification on [math]P[/math] that will ease virtually any interaction with [math]P[/math], including summing over many assignments and finding the most likely assignment. So at this point, I'd like you to think that CI statements regarding [math]P[/math] are a requirement for wielding it.Now put a pin in this and let's switch gears.The Bayesian NetworkIt's time to introduce the first type of PGM - the Bayesian Network ('BN'). A BN refers to two things, both in relation to some [math]\mathcal{X}[/math]: a BN graph (called [math]\mathcal{G}[/math]) and an associated probability distribution [math]P_B[/math]. [math]\mathcal{G}[/math] is a set of nodes, one for each RV of [math]\mathcal{X}[/math], and a set of directed edges, such that there are no directed cycles. Said differently, it's a DAG. [math]P_B[/math] is a distribution with probabilities for assignments of [math]\mathcal{X}[/math] using a certain rule and Conditional Probability Tables ('CPTs' and 'CPDs'), which augment [math]\mathcal{G}[/math]. That rule, called the 'Chain Rule for BNs', for determining probabilities can be written:[math]P_B(X_1,\cdots,X_n)=\prod_{i=1}^n P_B(X_i|\textrm{Pa}_{X_i}^\mathcal{G})\tag*{}[/math]where [math]\textrm{Pa}_{X_i}^\mathcal{G}[/math] indicates the set of parent nodes/RVs of [math]X_i[/math] according to [math]\mathcal{G}[/math]. The CPDs tell us what the [math]P_B(X_i|\textrm{Pa}_{X_i}^\mathcal{G})[/math] probabilities are. That is, a CPD lists out the probabilities of all assignments of [math]X_i[/math] given any joint assignment of [math]\textrm{Pa}_{X_i}^\mathcal{G}[/math][4]. These CPDs are the parameters of our model. Their form is to list out actual conditional probabilities from [math]P_B[/math].To help, let's consider a well utilized example from that monstrous text: the 'Student Bayesian Network'. Here, we're concerned with a system of five RVs: a student's intelligence ([math]I[/math]), their class's difficulty ([math]D[/math]), their grade in that class ([math]G[/math]), their letter of recommendation ([math]L[/math]) and their SAT score ([math]S[/math][math][/math]). So [math]\mathcal{X}=\{I,D,G,L,S\}[/math]. The BN graph along with the CPDs can be represented as:According to our rule, we have that any joint assignment of [math]\mathcal{X}[/math] factors as:So we would calculate a given assignment as:Not too bad, right? All this is to show is that a BN along with CPDs gives us a way to calculate probabilities for assignments of [math]\mathcal{X}[/math].Now we're ready for:The big idea.It's so big, it gets its own quote block:The BN graph, just those nodes and edges, implies a set of CI statements regarding it's accompanying [math]P_B[/math].It's a consequence of the Chain Rule for calculating probabilities. As a not-at-all-obvious result, a BN graph represents all [math]P[/math]'s that satisfy these CI statements and each of those [math]P[/math]'s could be attained with an appropriate choice of CPDs.For a BN, one form of those CI statements are:[math](X_i \perp\textrm{NonDescendants}_{X_i}|\textrm{Pa}_{X_i}^\mathcal{G})[/math] for [math]X_i \in \mathcal{X}[/math]So in the student example, we'd have this set:The third statement tells us that if you already know the student's intelligence and their class's difficulty, then knowing their SAT score won't help you guess their grade. This is because the SAT score is correlated with their grade only via their intelligence, and you already know that.These are referred to as the local semantics of the BN graph. To complicate matters, there are almost always many other true CI statements associated with a BN graph outside of the local semantics. To determine those by inspecting the graph, we use a scary 'D-separation' algorithm that I will shamelessly not explain.There is a reason this is so important. Since a BN graph is a way of representing CI statements and such statements are a requirement for handling a complex system's joint distribution (if you had it), then this is a good reason to use a BN to represent such systems. If we can accurately represent a system with a BN, we will be able to calculate our probability and MAP queries. Therefore, BNs will solve our problems when we're dealing with a certain class of [math]P[/math]'s. This choice, unsurprisingly, is called our representation.But there's an issue - I said a 'class' of [math]P[/math]'s. It's not hard to invent [math]P[/math]'s that come with CI statements a BN cannot represent.So now what? Well, we have other tools, the biggest of which is...The Markov NetworkA Markov Network ('MN') is likewise composed of a graph ([math]\mathcal{H}[/math]) and a probability distribution ([math]P_M[/math]). Though this time, the graph's edges are undirected and it may have cycles. The consequence is that a MN can represent a different set of CI statements. But, the lack of directionality means we can no longer use CPDs. Instead, that information is delivered with a factor, which is a function (function! remember it) that maps from an assignment of some subset of [math]\mathcal{X}[/math] to some nonnegative number. These factors are used to calculate probabilities with the 'Gibbs Rule'[5].To understand the Gibbs Rule, we must define a complete subgraph. A ‘subgraph’ is exactly what it sounds like - we make a subgraph by picking a set of nodes from [math]\mathcal{H}[/math] and including all edges from [math]\mathcal{H}[/math] that are between nodes from this set. A 'complete' graph is one which has every edge it can - each node has an edge to every other node.Now, let's say [math]\mathcal{H}[/math] breaks up into a set of [math]m[/math] complete subgraphs. By 'break up', I mean that the union of all nodes and edges across these subgraphs gives us all the nodes and edges from [math]\mathcal{H}[/math]. Let's write the RVs associated with the nodes of these subgraphs as [math]\{\mathbf{D}_i\}_{i=1}^m[/math]. Let's also say we have one factor (call it [math]\phi_i(\cdot)[/math]) for each of these. We refer to these factors together with [math]\Phi[/math], so [math]\Phi=\{\phi_i(\cdot)\}_{i=1}^m[/math]. For terminology's sake, we say that the 'scope' of the factor [math]\phi_i(\cdot)[/math] is [math]\mathbf{D}_i[/math] because [math]\phi_i(\cdot)[/math] takes an assignment of [math]\mathbf{D}_i[/math] as input.Finally, the Gibbs Rule says we calculate a probability as:where(It's hidden from this notation, but we're assuming it's clear how to match up the assignment of [math]X_1,\cdots,X_n[/math] with the assignments of the [math]\mathbf{D}_i[/math]'s.)Wait - the MN was introduced because it represents a different set of CI statement. So, which ones? It's considerably simpler in the case of a MN. A MN implies the CI statement [math](\mathbf{X} \perp \mathbf{Y}|\mathbf{Z})[/math] if all paths between [math]\mathbf{X}[/math] and [math]\mathbf{Y}[/math] go through [math]\mathbf{Z}[/math]. Easy!Now let's get specific. Below is an MN for the system [math]\mathcal{X}=\{A,B,C,D\}[/math] and the CI statements it represents:As you may notice, it's not hard to write those CI statements by viewing the graph.While we're here, let's write out the Gibbs Rule. By looking at this, we could identify our complete subgraphs as: [math]\{\{A,B\},\{B,C\},\{C,D\},\{D,A\}\}[/math]. With that, we calculate a probability as:whereTo repeat, each [math]\phi_i(\cdot,\cdot)[/math] is just a function that maps from it's given joint assignment to some nonnegative. So if [math]A[/math] and [math]B[/math] could only take on two values each, [math]\phi_1(\cdot,\cdot)[/math] would relate the four possible assignments to four nonnegative numbers. These functions serve as our parameters just as the CPDs did. Determining these functions brings us from a class of [math]P[/math]'s to a specific [math]P[/math] within it, defined with probabilities.But, ahem, uhh… there's an issue. In the BN case, I said:As a not-at-all-obvious result, a BN graph represents all [math]P[/math]'s that satisfy its CI statements and each of those [math]P[/math]'s could be attained with an appropriate choice of CPDs.The analogous is not true in the case of MNs. There may exist a [math]P[/math] that satisfies the CI statements of a MN graph, but we can't calculate it's probabilities with the Gibbs rule. Damn!Fortunately, these squirrely [math]P[/math]'s falls into a simple, though large, category: those which assign a zero probability to at least one assignment. This leads us to the Hammersley-Clifford theorem:If [math]P[/math] is a positive distribution ([math]P(\mathbf{X}=\mathbf{x})>0[/math] for all [math]\mathbf{x} \in Val(\mathcal{X})[/math]) which satisfies the CI statements of [math]\mathcal{H}[/math], then we may use the Gibbs Rule, along with a choice of complete subgraphs and associated factors, to yield the probabilities of [math]P[/math]. [6]And that about does it for the basics of MNs. They are just another way of representing another class of [math]P[/math]'s.How do BNs and MNs compare?At this point, we're not evolved enough for a full comparison, so let's do a partial one.First, it's clearly easier to determine CI statements in a MN - no fancy D-separation algorithm required. This follows from their simple symmetric undirected edges, which make them a natural candidate for certain problems. Broadly, MNs do better when we have decidedly associative observations - like pixels on a screen or concurrent sounds. BNs are better suited when we suspect the RVs attests to distinct components of some causal structure. Timestamps and an outside expectation of what's producing the data are helpful for that.Also, there's a certain overlap between a MN and a BN that'll unify our discussion in later answers. That is, the probabilities produced by the Chain Rule of any given BN can be exactly reproduced by the Gibbs Rule of a specially defined MN. To see this, look at the Chain Rule - [math]P_B(X_i|\textrm{Pa}_{X_i}^\mathcal{G})[/math] is just the conditional probability of some (unspecified) [math]X_i[/math] value given some assignment of the parent RVs. Well, to translate this to the Gibbs Rule, let [math]\mathbf{D}_i=\{X_i\}\cup\textrm{Pa}_{X_i}^\mathcal{G}[/math]. Next, define [math]\phi_i(\mathbf{D}_i)[/math] to produce the same output you'd get from looking up the BN conditional probability in the CPD (which is [math]P_B(X_i|\textrm{Pa}_{X_i}^\mathcal{G})[/math]). Awesome - now the Gibbs Rule is the same expression as the Chain Rule. This is useful because we can speak solely in terms of the Gibbs Rule and whatever we discover, we know will also work for the Chain Rule (and hence BNs). What this doesn't mean is that MNs are a substitute for BNs. If you were to look at this invented MN, it would likely imply way more edges in its graph and therefore, fewer CI statements and therefore, a wider and more unwieldy class of [math]P[/math]'s. In other words, BNs are still useful representations.But there's more to learn.Let's say we determined our graphical model along with its parameters. How do we actually answer those queries? Well, I have three suggestions:2. What is 'exact inference' in the context of Probabilistic Graphical Models?3. What is Variance Inference in the context of Probabilistic Graphical Models?4. How are Monte Carlo methods used to perform inference in Probabilistic Graphical Models?Footnotes[1] This is the one exception where we don't refer to a set of RVs with a bold uppercase letter.[2] This actually isn't the fully general problem specification. In complete generality, the set of RVs should be allowed to grow/shrink over time. That, however, is outside what I expect to accomplish in these posts.[3] There is a subtlety of language here. Often we'll say '[math]P[/math] satisfies these CI statements'. That means those CI statements are true for [math]P[/math], but others may be true as well. So it means 'these CI statements' are a subset of all [math]P[/math]'s true CI statements. This technicality matters, so keep an eye out for it.[4] If [math]X_i[/math] doesn't have any parents, then the CPD is the unconditional probability distribution of [math]X_i[/math].[5] This isn't a real name I'm aware of, but the form of that distribution makes it a Gibbs distribution and I'd like to maintain an analogy to BNs, which had the Chain Rule.[6] The implication goes the other way as well: If the probabilities of [math]P[/math] can be calculated with the Gibbs Rule, then it's a positive distribution which satisfies CI statements implied by a graph which has complete subgraphs of RVs that correspond to the RVs of each factor. This direction, however, doesn't fit into the story I'm telling, so it sits as a lonely footnote.Sources[1] Koller, Daphne; Friedman, Nir. Probabilistic Graphical Models: Principles and Techniques (Adaptive Computation and Machine Learning series). The MIT Press. Kindle Edition. This is the source of the notation, the graphics in this answers (with permission) and my appreciation for this subject.
Scalability: How does Heroku work?
(Note: this answer was written in 2011 and I’m guessing a lot has changed since then)Well, since no one else answered, I decided to break out The Google and dig a little deeper. Here's my high-level interpretation of how Heroku works. It's based on public information, but I don't think I've seen it all collected in one place before.Parts of it may be incorrect or imprecise, in which case please correct me. I've tried to note the places where I've made assumptions/inferences/guesses. Obviously there is a lot more to Heroku than what's publicly known.Request LifecycleHeroku runs DNS servers that point "appname.heroku.com" domains to a subset of their front-end reverse proxies, or you can setup a custom subdomain with a CNAME that points to proxy.heroku.com (which in turn resolves to the reverse proxy IPs), or a root domain with A records pointing directly to Heroku's reverse proxies. Multiple IPs (currently 3) will be returned to provide failover in case one or more of the reverse proxy servers is down.Your browser sends an HTTP request to ones of the reverse proxy servers (Nginx (web server)) pointed to by the DNS (if it fails to connect it should try another one of the IPs). The request is forwarded to the HTTP cache layer. When the response eventually comes back gzip encoding might be applied depending the the Content-Type header. The nginx servers also terminate SSL (i.e. HTTPS) connections from the browser. If you want a custom domain to work with SSL (not relying on SNI) Heroku must run one of these nginx server instances, with it's own IP, specifically for your application.The HTTP cache layer (Varnish) accepts requests forwarded from the reverse proxies. It will return a cached page immediately if it's in the cache, or forward the request to the "routing mesh" if not cached. Responses returned from the routing mesh / app servers are cached if the appropriate HTTP headers are set.[Information available on this piece is sparse, it's part of their secret sauce] The custom routing mesh (written in Erlang) looks for an app server (Heroku calls it a "dyno") with capacity to serve your request. If none is running it spawns one. If the load is high and the app pays for additional dynos it might spawn another dyno. The request is held until a dyno has been started or is idle, at which point it's forwarded to the new/idle dyno.Each app's dynos are spread across the "dyno grid", which consists of many servers (Heroku calls these "railgun" servers) running many applications' dynos. Dynos are spawned when necessary, which usually only takes a couple seconds. Non-responsive and excess capacity dynos are eventually killed / garbage collected, freeing up capacity for other apps' dynos. It looks like Heroku runs up to at least 60 dynos/workers per railgun instance, which appears to be an EC2 "High-Memory Extra Large Instance" with 17.1GB of RAM and two 2.67GHz CPU cores (see http://aspen-versions.heroku.com/evil)Upon deployment ("git push" to Heroku's git server) application code and dependencies are compiled into "slugs" (railgun fires off the slugs... get it?), a read-only compressed filesystem (SquashFS) that can quickly be downloaded to a railgun, mounted, and executed in a chroot sandbox with the app's configuration environment variables set. Each dyno has it's own Unix user, it can only see the files in it's own chroot jail, and cannot write to the filesystem. Security does not rely on VM sandboxing.The application server process is MRI Ruby (or Node.js) and the Ruby webserver used is Thin (based on Mongrel). The Ruby stack exposes the Rack web interface.DeploymentHeroku's git server accepts git pushes to each application's repository from permitted users. A pre-receive hook kicks off the rest of the deployment process.A git checkout of the HEAD of master is done.For Ruby apps, dependencies listed in the gem manifest (.gem) are downloaded, and native extensions are compiled if necessary.Extra files like .git, .gem, tmp, and logs are stripped.The application is compiled into the previously mentioned SquashFS "slug" with it's dependencies and configuration environment variables.The slug is tested by launching the app. If the app fails to start the deployment (including the git push) is rejected.[I'm making assumptions here.] The routing mesh stops sending requests to dynos running old slugs. Old dynos finish responding to their current request and are killed while new dynos with the new slug are started, and the routing mesh begins forwarding requests to the new dynos.Applications are also restarted after certain operations like setting configuration variables and changing add-ons so that the application has the latest configuration data.DNSIt appears Heroku runs approximately 6 nginx reverse proxies (plus the customers who pay $100/month for a dedicated IP/proxy so they can use SSL on a custom domain name).Of the domains I checked (a few hundred found through the "Find Subdomains" tool here: http://www.magic-net.info/), appname.heroku.com (including proxy.heroku.com) will return 3 of these:50.16.215.19650.16.232.13050.16.233.10275.101.145.87174.129.212.2It's possible Heroku's DNS is returning different IPs based the load of the reverse proxies, but when querying heroku.com's 4 nameservers directly I got different subsets of those 5 IPs. A random distribution of IPs probably gives good enough load distribution.And Heroku's documentation says to point A records to these three:75.101.163.4475.101.145.87174.129.212.2It's also interesting to note that apps aren't tied to specific proxy servers. If you set the "Host" header to your app's subdomain, a request to any one of those IPs will work.SQL DatabaseA PostgreSQL database is automatically provisioned for each application.Databases are run on either shared or dedicated EC2 instances with EBS persistance.Database connection configuration details are passed to dynos via environment variables.Database backups were previously performed at periodic intervals for shared databased but they will soon roll out a continuous backup scheme for all databases.TAPS can be used to import/export your databases.MemcachedUses Membase provided by Couchbase (formerly Membase formerly NorthScale) as an add-on.WorkersWorkers (formerly Delayed Job) are also run on the railgun servers similar to app server dynos.Almost half of the dynos on Heroku's dyno grid are workers.LoggingLogs from apps servers' and workers' stdout, and even some of Heroku's internal infrastructure components (nginx, router, api, slugc) and add-ons are sent to a syslog router they developed called Logplex.For Rails applications they inject rails_log_stdout to get logging on stdout.Users can access logs via the command line tool, and even setup their own syslog endpoint.Add-onsThird-party add-ons (and some of Heroku's own services) implement implement a REST API to automate provisioningWhen a customer adds an add-on Heroku makes requests to the add-on's API.The provider receives the API request and provisions the add-on for the application.Thee provider returns a response containing configuration data (locations and credentials) that the application can use to connect to the add-on's services.The application slug is recompiled and restarted. This data is exposed to the application via environment variables like other configuration data.Misc TechDoozer, "a new, consistent, highly-available data store written in Go" for distributed coordination between their internal services. Doozer is similar to Apache ZooKeeper.Redis for "a redundant cache of shared state data, a means of tracking dynamic clusters of running instances, a container for realtime statistics data and a transient data store for high volumes of log messages".RabbitMQ plus a Ruby DSL called Minion.Splunk (product) (http://www.splunk.com/view/SP-CAAAFP4) for managing, monitoring, and troubleshooting their infrastructure.Librato's Silverline/Load Manager (https://silverline.librato.com/press_releases/20100316) for fine-grained load management.References(Sorry these aren't cited inline, but I think they're all there)http://www.heroku.com/how/architecturehttp://devcenter.heroku.com/articles/custom-domainshttp://devcenter.heroku.com/articles/http-cachinghttp://devcenter.heroku.com/articles/slug-compilerhttp://devcenter.heroku.com/articles/why-does-ip-based-ssl-cost-100-monthhttp://blog.heroku.com/archives/2010/12/13/logging/http://blog.heroku.com/archives/2010/3/16/memcached_public_beta/http://status.heroku.com/incident/151http://adam.heroku.com/http://adam.heroku.com/past/2009/9/28/background_jobs_with_rabbitmq_and_minion/http://adam.heroku.com/past/2011/4/1/logs_are_streams_not_files/http://orion.heroku.com/past/2009/7/29/io_performance_on_ebs/http://groups.google.com/group/herokuhttps://addons.heroku.com/provider/resources/technical/how/overviewhttp://blog.golang.org/2011/04/go-at-heroku.htmlhttps://github.com/heroku and https://github.com/hahttp://aspen-versions.heroku.com/evil (inspect processes and other system information on a railgun server)http://highscalability.com/heroku-simultaneously-develop-and-deploy-automatically-scalable-rails-applications-cloudhttp://pivotallabs.com/talks/30Update 5/31/2011Heroku released a major update to the platform with the "Celadon Cedar" stack: http://news.heroku.com/news_releases/heroku-announces-major-new-version-celadon-cedar-includes-new-process-model-full-nodejs-Heroku.com also got a slick update: http://www.heroku.com/howList of new devcenter documents: https://gist.github.com/1000964Procfile (http://adam.heroku.com/past/2011/5/9/applying_the_unix_process_model_to_web_apps/) is used for defining and managing processes. "web" and "worker" map to the existing dynos and worker, and you can define custom process types. "heroku scale" is used to adjust the number of each process type running on the platform.Cedar has first-class Node.js and Ruby 1.9. There appears to be unofficial support for other languages including Python (https://gist.github.com/866c79035a2d066a5850), Go, and Erlang, and potentially the ability to use custom processes/languages.All processes (web and workers) are now considered "dynos" and treated identically. (http://devcenter.heroku.com/articles/process-model)LXC (http://lxc.sourceforge.net/) is used for better process isolation in addition to chroot for filesystem isolation. http://devcenter.heroku.com/articles/dyno-isolationNew herokuapp.com HTTP stack has support for HTTP/1.1, long polling, chunked responses (http://devcenter.heroku.com/articles/http-routing). It also supports multiple concurrent requests per dyno. The routing mesh will send requests to the dyno immediately, or if you have multiple dynos it will select a random dyno. This is necessary to take advantage of asynchronous or multithreaded web servers such as Node.js, EventMachine, etc.The herokuapp.com stack does not include the Varnish cache layer because it is incompatible with long-polling/chunked responses (rack-cache or memcache is recommended). It also doesn't have the 30 second timeout present on the heroku.com stack, but it does have a 60 second inactivity timeout.The "dyno manifold" is apparently the new name for the "dyno grid" (http://devcenter.heroku.com/articles/dyno-manifold). Cedar gives you greater visibility into the platform through the previously discussed Logplex logging system (now with pretty colored logs!), process listings ("heroku ps": http://devcenter.heroku.com/articles/ps), easier ways for running arbitrary programs (e.x. try "heroku run bash")Processes are idled (shut down) after one hour of inactivity.Processes are terminated if they bind to incorrect ports (e.x. anything except $PORT on 0.0.0.0).Cedar apps are detected based on the presence of "Gemfile" for Ruby or "package.json" for Node.
- Home >
- Catalog >
- Business >
- Tracking Templates >
- excel task tracker template >
- S List Tracking And Distribution Of Any Potential