How to Edit The Order Form Updated Nov freely Online
Start on editing, signing and sharing your Order Form Updated Nov online under the guide of these easy steps:
- Push the Get Form or Get Form Now button on the current page to access the PDF editor.
- Wait for a moment before the Order Form Updated Nov is loaded
- Use the tools in the top toolbar to edit the file, and the edited content will be saved automatically
- Download your completed file.
The best-rated Tool to Edit and Sign the Order Form Updated Nov
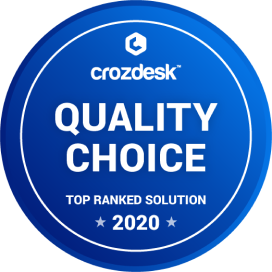
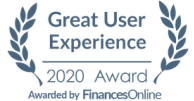
A quick guide on editing Order Form Updated Nov Online
It has become very simple just recently to edit your PDF files online, and CocoDoc is the best free tool you would like to use to make some editing to your file and save it. Follow our simple tutorial to start!
- Click the Get Form or Get Form Now button on the current page to start modifying your PDF
- Add, change or delete your content using the editing tools on the tool pane on the top.
- Affter altering your content, put the date on and add a signature to finalize it.
- Go over it agian your form before you save and download it
How to add a signature on your Order Form Updated Nov
Though most people are adapted to signing paper documents by writing, electronic signatures are becoming more usual, follow these steps to sign documents online free!
- Click the Get Form or Get Form Now button to begin editing on Order Form Updated Nov in CocoDoc PDF editor.
- Click on the Sign tool in the tool menu on the top
- A window will pop up, click Add new signature button and you'll have three choices—Type, Draw, and Upload. Once you're done, click the Save button.
- Drag, resize and settle the signature inside your PDF file
How to add a textbox on your Order Form Updated Nov
If you have the need to add a text box on your PDF in order to customize your special content, follow the guide to finish it.
- Open the PDF file in CocoDoc PDF editor.
- Click Text Box on the top toolbar and move your mouse to position it wherever you want to put it.
- Write in the text you need to insert. After you’ve input the text, you can take full use of the text editing tools to resize, color or bold the text.
- When you're done, click OK to save it. If you’re not happy with the text, click on the trash can icon to delete it and start again.
A quick guide to Edit Your Order Form Updated Nov on G Suite
If you are looking about for a solution for PDF editing on G suite, CocoDoc PDF editor is a suggested tool that can be used directly from Google Drive to create or edit files.
- Find CocoDoc PDF editor and set up the add-on for google drive.
- Right-click on a PDF document in your Google Drive and choose Open With.
- Select CocoDoc PDF on the popup list to open your file with and give CocoDoc access to your google account.
- Modify PDF documents, adding text, images, editing existing text, mark with highlight, trim up the text in CocoDoc PDF editor before saving and downloading it.
PDF Editor FAQ
Are you alloted AIIMS Bhubaneswar. When are you joining.?
Yes, I took admission todayYou have to report at lecture theater-1 between 9:30am –5:00 pm , you'll be given 3 forms to fill up.1- A document checklist. (arrange your documents in the order given in it)(candidate id mentioned in the checklist is your neet registration number so keep it with you.)2- self declaration.3- student information .Carry a demand draft in favour of Aiims bbsr Rs-5856.After document verification , they'll give you admission letter.The tentative joining date on website is 18th nov but we were told joining would be around 3rd dec, final dates will be updated on the site soon.The staff is really helpful they'll help you out with any confusions you may have.Best of luck!
How does the AI in Tesla distinguish between a large hole in the road or a dark stain on the road?
Question from Chris Gunson—Thanks for asking the question, Chris!^ How does a car AI system tell these apart? The third one is potentially dangerous. The others… not so much.What about when you add shadows into the equation—what then?^ Dark shadows and stains may appear like…or obscure…potholes. They complicate 2D “outline” pattern recognition.~~~~Q. ORIGINAL QUESTION: How does the AI in Tesla distinguish between a large hole in the road or a dark stain on the road?A. Tesla Dojo AI Training program is going 4D.This means that the new Tesla AI will be able to learn to work more like human eyes and mind—to distinguish potholes from stains and shadows.The Tesla NN (Neural Net) system is just learning now—in Fall 2020—how to tell these apart. This is often a difficult job for humans, so it is not surprising that it is also hard for a Neural Net.DETAILSHow did the Tesla AI work before 4D and Dojo?Basically, Tesla’s AI started out using 2D pattern matching or pattern recognition with just a little information from shading or coloring of the inside of the pattern, and little fine edge detail. This means that the AI looked mainly for gross OUTLINES of objects, or other large patterns, and classified those.This, Tesla learned, is a person:^ Architectionary~~~~How does the addition of Dojo change things—what is 4D anyway?The problem with 2D “outlines”—like the one above—is that a shape like this one below—made by a shadow of a tree—could be a two-dimensional shadow, or a wet spot, or a filled pothole that is now level, or a 10 inch deep hole.From a distance, shadow shapes like this one cannot be recognized by their outline alone. Possible outlines of the 4 types above are infinite… because a hole, shadow or stain could be any shape. So black spots shaped like this are randomly correlated with the types of objects we need to classify them into.The black spot’s outline on the road ahead could indicate a shadow, or a pothole, or a stain—the rough outline alone does not tell us.Once you get close enough, it is not too hard for the human eye/mind to recognize this one above as a shadow in what Elon calls 2.5D—when you add some fine details of edge shaping, contrast, color, and shading information.Humans know from experience that trees cast shadows. A human who lives in this area would be familiar with the typical leaf and branching characteristics of this kind of tree. We would immediately recognize the shadow as a projection of a common type tree outline cast by the sun. Humans in this area would see hundreds or thousands of trees like this one—and their shadows—per day, so their outlines are easily recognized.But other shadows can be much harder.It is especially difficult to ID a dark spot on the road…while approaching from a distance …at high speed and a shallow viewing angle…with slowly changing perspective until we are nearly “upon” the object.Let’s dig a little deeper…^ It is not necessary for a human to see the entire shadow above—nor the entire tree that cast it—for recognition. Just a sufficient sample of the fine edge patterning is enough. Humans seeing a small part of the shadow above will recognize the typical leaf and branching structure on the edge of the shadow. We can then infer that it is a shadow from a particular tree type we are used to seeing. Then, the rest of the shadow, as it is scanned, will be recognized as just an extension—more parts—of the same phenomenon.^ Look at the leaf group in the lower right of the picture. Humans know that this is a tree because of context—they know where they are at the moment. If this is their own driveway, they would know exactly WHICH tree cast this shadow, and about what time of day it is. They could even know a little bit about the weather from the clarity and contrast of the shadow boundary, and the apparent color warmth of the concrete—it is a bright sunny day!What if the human seeing this view were SCUBA diving in the South China Sea at 40 feet depth?THEN, the human would realize that this could not be a tree shadow.Maybe it is a grouping of sea urchins viewed through murky water. Maybe this is some seaweed or coral formation. Maybe something with sharp spines and venom that should be avoided, or watched until it is ID-ed better.Note: it may be hard for you, right now, to imagine that the picture above could be sea urchins—because you saw the whole picture first—and “locked on” to the context and ID of a driveway with tree shadow. But if I showed you the smaller picture above FIRST and told you this was a photo from a low-res black and white underwater camera at depth…with maybe some dirt on the lens…you would not have imagined a driveway or a tree at all. You probably would have complained to me that the picture lacks definition and looks “flat” and “grainy.” But you would likely not be thinking “driveway?” “tree?”But I digress. Back to the roads:How about this dark spot below?—What is it? How deep is it? Would it be easy to tell when approaching at 80 MPH—130 kph?The answer the human perceptual system has evolved is to look at things for a few fractions of a second while moving. You will even see humans and animals moving their heads from side to side or walking around—in order to create movement—and get more perspectives on something they do not immediately recognize.If the thing is a shadow, it may move as you move, and will show no 3D vertical development or depth different from the surface it is projected upon (it requires 4D to tell that, though).If the thing is 10 inches deep, the 3D view will change a lot as you move… and light and shadows change—revealing the depth aspect (again, 4D required).If it is a water puddle, specular (image) reflections will move across the surface as you approach (4D required).If it is a “dry” oil stain with no depth, the view and shading will not change in the same way—it will usually be more “flat” without specular (image) surface reflections nor much “new” 3D information being revealed as we get closer (4D required).Christopher Albertson, in his comment below, adds some sophisticated ways the Tesla systems could work. Maybe check out the comments…..Elon Musk has explained that Tesla has basically moved from ~2.5D to 4D with the changes that started propagating through the Tesla Neural Net system in summer 2020—see first two separate links at end of answer, below.Tesla Dojo will now allow the Tesla to do the same thing as humans instinctively do—to look at streams of pictures over time. Simultaneously with the jump from ~2.5D to 4D, the machine system continues to learn from the billions of miles accumulating and benefits from other system architecture improvements—such as many more HW3 (the latest version of in-car computer) coming online. So the algorithm improves in several ways. And the results?We add more sophisticated 3D per frame—depth perception, which the Tesla does with stereoscopic vision and sensor fusion of camera, radar and ultrasonic sensors. At the high resolutions now possible with HW3, I imagine that the accumulation of miles alone improves 3D.(EDIT/UPDATE Nov 4, 2020—maybe too obvious to mention, but the NN—Neural Net—memory can also estimate the size and distance of vehicles by knowing their identity and the model history. For example—a 2019 Ford F-150 has a particular size, and having seen hundreds of them, when we recognize another, we can infer its distance from what we know from the past of the pixels covered—the “size” of its silhouette at various distances.)We add more ability to look at what is inside an outline, its shading and coloring.AND, we add 4D—perception of change in view over time and change in spatial perspective.Importantly, The Tesla AI can see and “focus on” 360 degrees all at once, and can analyze many snapshots or “frames” per second while approaching. The human driver focusing on the puddle above…might miss something more important. Important objects may be relegated to the humans’ peripheral vision and subconscious processing, versus focal, attention. The puddle may attract our full visual and mental focus. But the Tesla can watch this puddle as it approaches—and also track and analyze the other cars all around, the road edge, the signs and lines, etc.—all at once.The Tesla (HW 3.0) FSD autonomous driving chip sees/thinks at a time division rate of 2 GHz. That is 2,000,000,000 Hz or cycles per second. MIT scientists have measured human thinking processes at around 60 bits per second. Here:New Measure of Human Brain Processing SpeedThe Tesla GPU operates at 1 GHz, capable of up to 600 GFLOPS (GFLOPS is 10^9 or Billions of Floating Point Operations Per Second).Dojo is reportedly designed to operate in the exaFLOP range—that is 10^18 FLOPS or 1,000,000,000,000,000,000 FLOPS.HW3 includes a custom Tesla-designed system on a chip. Tesla claimed that the new system would process 2,300 frames per second (fps), which is a 21x improvement in image processing compared to HW2.5, which is capable of 110 fps.Tesla Autopilot - Wikipedia.So the computer can see a lot at once, and divide the visual streams into many short moments that can be compared—to yield quicker identification of what it’s looking at. And that is how it will become possible to differentiate a stain or shadow from a hole.Tesla's Elon Musk details Dojo, Autopilot's 4D training programTesla Autopilot's 4D upgrade could lead to more FSD featuresElon Musk hints at Tesla's not-so-secret Dojo AI-training supercomputer capacity - ElectrekFSD Chip - Tesla - WikiChipDisclaimers — I am not employed by Tesla and do not have first hand knowledge of the design and operation of Tesla AI systems—other than the Model 3 I drive. I am reporting information from the press and from statements made in Tesla Shareholder meetings. There is some inference here, and I have simplified things in ways I think may be useful.As Gregor Kikelj has pointed out below, the Dojo system does not yet appear to be complete. For that matter, the FSD system is also obviously not complete with final software—whatever that may mean—and not all Tesla cars have the FSD computer.We are talking, here, about technology development in progress. It is happening as I write this, and there will be delays and also new developments that I may not immediately know about…as is the nature of new technology development happening in many places at once. My verbs tenses above may be wrong—allows, allowed, will allow…..Development is happening quickly at Tesla, and rather than continually update the content in this answer to reflect new Tesla FSD software changes, I intend to leave it in its current form from November, 2020—as historical data…from a point in time that is now in the past. I will add amplifying information as needed—to clarify points. Otherwise, this answer is about something that, by the time you read it—is history.o
What are some examples of extremely clever problem solving in the software development world?
There are so many… it’s part of what makes the field so much fun.Here are two “clever tricks” that come to mind…Counting OnesConsider the binary representation of a number:[math]b_{N-1}b_{N-2}...b_1b_0[/math]Now, suppose the [math]Z[/math] least significant digits are 0, and the next is a 1:[math]x = b_{N-1}b_{N-2}...b_{Z+1}10...0[/math]If you subtract 1 from that number, you get:[math]x-1 = b_{N-1}b_{N-2}...b_{Z+1}01...1[/math]In other words, we’ve inverted the last [math]Z+1[/math] binary digits without changing any others. So, if I perform bitwise “and” operation on [math]x[/math] and [math]x-1[/math], I effectively cleared the least significant “one” bit:[math]x \& (x-1) = b_{N-1}b_{N-2}...b_{Z+1}00...0[/math]That leads to a clever little loop that counts “one” bits in C or C++:int count_ones(unsigned long long x) { int r = 0; while (x != 0) { x = x & (x-1); // Clear the least significant bit. r = r + 1; // Count it. } return r; } (Some modern compilers recognize this loop and optimize it to a single “popcount” instruction on CPUs that support the corresponding operation.)Corrolary: Checking that [math]x[/math] is a power of two amounts to checking that x != 0 && (x & (x-1)) == 0A Fast Fourier TransformYou might have heard of the “Fourier transformation”:[math]F(\nu) = \displaystyle\int_{-\infty}^{+\infty}f(t)e^{-i2\pi t\nu}dt[/math]which provides a way to decompose signals into the “frequencies” they’re made up of. In practice, we often compute a discrete counterpart, known as the “discrete Fourier transform” (DFT):[math]\forall n \in \{ 0...N-1 \}: F_n = \displaystyle\sum_{k=0}^{N-1}f_ke^{-i{{2\pi}\over{N}}kn}[/math]Here is a straightforward C++ implementation of that sum:#include <cassert> #include <complex> #include <vector> using Scalar = double; using CScalar = std::complex<Scalar>; using Vec = std::vector<CScalar>; Vec straight_dft(Vec input) { int const N = (int)input.size(); assert(N != 0 && (N & (N-1)) == 0); Vec output(N, 0.0); Scalar minus_2pi_over_N = -(2*M_PI)/N; for (int n = 0; n<N; ++n) { for (int k = 0; k<N; ++k) { CScalar c = exp(CScalar(0, k*n*minus_2pi_over_N)); output[n] += input[k]*c; } } return output; } There are [math]N[/math] complex values to compute as sums of [math]N[/math] terms, so this is an [math]O(N^2)[/math] process: The two nested [math]O(N)[/math] loops make that obvious. Here is a bit of code to exercise that function:#include <algorithm> #include <iostream> int main() { int const N = 1024; Vec v(N, 0.0); // Build a "signal" with two frequencies: "7" and "13". Scalar unit_freq = 2*M_PI/N; for (int k = 0; k<N; ++k) { v[k] = 3*sin(7*unit_freq*k) + 2*cos(13*unit_freq*k); } // Compute the DFT: Vec w1 = straight_dft(v); // Retrieve the "peak" frequency in the first half. // The expected result is "7" since that component has // the highest amplitude. auto cmp = [](CScalar x, CScalar y) { return abs(x) < abs(y); }; auto peak1 = std::max_element(w1.begin(), w1.begin()+N/2, cmp); std::cout << "DFT: peak @" << peak1-w1.begin() << ", amplitude = " << abs(*peak1)/(N/2) << ", phase = " << arg(*peak1)/M_PI*180 << " degrees\n"; return 0; } Here main() builds up an input vector sampling a combination of two purely sinusoidal “signals”. The first uses the sine function and the second the cosine function, so there is a [math]90{^\circ}[/math] phase difference between the two components. The remainder then computes the DFT of this signal and outputs its highest component (in the first half of the result; when the input is real, the second half is essentially the mirror of the first half). Compiling and running this, gives the following result on my laptop:$ g++ -std=c++11 dft.cpp && ./a.out DFT: peak @7, amplitude = 3, phase = -90 degrees which is what I expected since the strongest component has amplitude 3.[math]O(N^2)[/math] isn’t great, but it turns out that rearranging these computations allows it to be done with [math]O(N log N)[/math] operations. The resulting algorithms are known as FFT algorithms (“Fast Fourier Transform”). They are most easily derived and implemented when [math]N[/math] is an integer power of 2 (e.g., [math]N = 4096[/math]): I’ll assume that in the following derivation and implementation of such an algorithm. Hopefully, you’ll find it “extremely clever” ;-)First separate the even and odd terms in the DFT formula:[math]\displaystyle F_n = \sum_{k=0}^{N/2-1}f_{2k}e^{-i{{2\pi}\over{N}}2kn} + \sum_{k=0}^{N/2-1}f_{2k+1}e^{-i{{2\pi}\over{N}}(2k+1)n}[/math][math] = \displaystyle\sum_{k=0}^{N/2-1}f_{2k}e^{-i{{2\pi}\over{N/2}}kn} + e^{-i{{2\pi}\over{N}}n}\sum_{k=0}^{N/2-1}f_{2k+1}e^{-i{{2\pi}\over{N/2}}kn}[/math]In that last form, the first sum is the DFT of the “even-numbered” [math]f_k[/math] and the second is the DFT of the “odd-numbered” [math]f_k[/math]. Let’s call those sums [math]F^{0:2}_n[/math] and [math]F^{1:2}_n[/math], respectively; i.e., the superscript denotes the index of the first element and the “stride” (2 in this case), separated by a colon (that’s going to be useful going forward):[math]F^{0:2}_n = \displaystyle\sum_{k=0}^{N/2-1}f_{2k}e^{-i{{2\pi}\over{N/2}}kn}[/math][math]F^{1:2}_n = \displaystyle\sum_{k=0}^{N/2-1}f_{2k+1}e^{-i{{2\pi}\over{N/2}}kn}[/math]In this notation, the original DFT could be denoted [math]F^{0:1}_n[/math] (i.e., every element starting from zero). So we can write:[math]F^{0:1}_n = F^{0:2}_n + e^{-i{{2\pi}\over{N}}n} F^{1:2}_n[/math]What’s more, we can use the fact that [math]e^{-i\pi} = -1[/math] and [math]e^{-i{2\pi}}[/math] = 1 to compute the [math]F_{N/2} … F_{N-1}[/math] using the same even/odd DFTs:[math]\displaystyle F^{0:1}_{n+N/2} = \sum_{k=0}^{N/2-1}f_{2k}e^{-i{{2\pi}\over{N/2}}k(n+N/2)} + e^{-i{{2\pi}\over{N}}(n+N/2)}\sum_{k=0}^{N/2-1}f_{2k+1}e^{-i{{2\pi}\over{N/2}}k(n+N/2)} [/math][math]= \displaystyle\sum_{k=0}^{N/2-1}f_{2k}e^{-i{{2\pi}\over{N/2}}kn}e^{-i{{2\pi}}k} + e^{-i{{2\pi}\over{N}}(n)}e^{-i{\pi}}\sum_{k=0}^{N/2-1}f_{2k+1}e^{-i{{2\pi}\over{N/2}}kn}e^{-i{{2\pi}}k}[/math][math] = F^{0:2}_n - e^{-i{{2\pi}\over{N}}n} F^{1:2}_n[/math]In other words, the “second half” of [math]F^{0:1}_n[/math] can be computed along with the first half by just replacing an addition by a subtraction. If we let [math]c_n(N) = e^{-i{{2\pi}\over{N}}n}[/math], this is summarized as:[math]F^{0:1}_n = F^{0:2}_n + c_n(N) F^{1:2}_n[/math][math]F^{0:1}_{n+N/2} = F^{0:2}_n - c_n(N) F^{1:2}_n[/math]Let’s call this our “elementary step”. Now that’s a typical “divide-and-conquer” situation: I can create a [math]N[/math]-input computation ([math]F^{0:1}_n[/math]) from two [math]N/2[/math]-input computations ([math]F^{0:2}_n[/math] and [math]F^{1:2}_n[/math]) with just [math]O(N)[/math] steps (each [math]F_n[/math] takes a fixed number of operations if I know [math]F^{0:2}_n[/math] and [math]F^{1:2}_n[/math]). And I can apply that reasoning recursively to [math]F^{0:2}_n[/math] and [math]F^{1:2}_n[/math], until I get to problems of “length one” (after [math]log^2 N[/math] steps). Adding it all up leads to [math]O(N log N)[/math] operations.One slightly tricky part is the arrangement of our intermediate results in memory. Many introductions to FFTs make a bit of a mess of this, introducing permutations that remove some of the elegance of the algorithm. I’ll try to avoid that here.Let’s reconsider our “elementary step”:[math]F^{0:1}_n = F^{0:2}_n + c_n(N) F^{1:2}_n[/math][math]F^{0:1}_{n+N/2} = F^{0:2}_n - c_n(N) F^{1:2}_n[/math]Again, this transforms the even and odd parts of an input vector ([math]F^{0:2}_n[/math] and [math]F^{1:2}_n[/math] — we’ll store them in that order) into the “upper” and “lower” parts of an output vector ([math]F^{0:1}_n[/math] and [math]F^{0:1}_{n+N/2}[/math]). How do we find the “input” vector? By reapplying the “elementary step”:[math]F^{0:2}_n = F^{0:4}_n + c_n(N/2) F^{2:4}_n[/math][math]F^{0:2}_{n+N/2} = F^{0:4}_n - c_n(N/2) F^{2:4}_n[/math][math]F^{1:2}_n = F^{1:4}_n + c_n(N/2) F^{3:4}_n[/math][math]F^{1:2}_{n+N/2} = F^{1:4}_n - c_n(N/2) F^{3:4}_n[/math]Note how the “even” entries of, e.g., the sequence of entries numbered [math]1, 3, 5, …[/math] (i.e., [math]1:2[/math]) are the entries [math]1, 5, 9, …[/math] or [math]1:4[/math] (i.e., “stride 4”). As you can see, the input vector of the previous step is the output of this step, but this time broken up in 4 sub-vectors of length [math]N/4[/math]: [math]F^{0:2}_n[/math], [math]F^{0:2}_{n+N/2}[/math], [math]F^{1:2}_n[/math], and [math]F^{1:2}_{n+N/2}[/math]. The input to this step is [math]F^{0:4}_n[/math], [math]F^{1:4}_n[/math], [math]F^{2:4}_n[/math], and [math]F^{3:4}_n[/math], once more in that order, which is the order of the leading entry number. Again, we can re-apply the “elementary” step to find out how to produce this input from subvectors of length [math]N/8[/math] but “stride 8”, and keep on going with subvectors of length [math]N/16[/math], [math]N/32[/math], etc. (doubling the stride as we go) until we reach subvectors of length 1 (and stride N, but the stride no longer matters for a single-element vector). Conveniently, by then the input vector will be [math]F^{0:N}_n[/math], [math]F^{1:N}_n[/math], [math]F^{2:N}_n[/math], [math]F^{3:N}_n[/math], … but since the DFT of a single element is that element itself, that last vector is in fact the input to the DFT computation.There is one more detail to notice… look at the general form of each update in a “elementary step” whose input vectors have length [math]p[/math]:[math]… = F^{x:N/p}_n \pm c_N(2p) F^{x+N/(2p):N/p}[/math]Note how the [math]F^{x+N/(2p):N/p}[/math] is [math]N/(2p)[/math] subvectors down from [math]F^{x:N/p}_n[/math], but since in that step each vector is p entries long, the subvectors are exactly [math]N/2[/math] scalar entries away from each other.Putting it all together, the implementation thus just has to compute the “elementary steps” backwards, which gives:#include <cassert> #include <complex> #include <vector> using Scalar = double; using CScalar = std::complex<Scalar>; using Vec = std::vector<CScalar>; Vec fft(Vec input) { int const N = (int)input.size(); assert(N != 0 && (N & (N-1)) == 0); Vec output(N, 0.0); for (int p = 1; p < N; p *= 2) { // p: length of the input subvectors for (int k0 = 0, k1 = 0; k0<N; k0 += 2*p, k1 += p) { // k0: start of the LHS subvector // k1: start of the first RHS subvector // k1+N/2: start of the second RHS subvector for (int k = 0; k<p; ++k) { CScalar c = exp(CScalar{ 0, k*-M_PI/p }); output[k0+k] = input[k1+k] + c*input[k1+N/2+k]; output[k0+k+p] = input[k1+k] - c*input[k1+N/2+k]; } } // Use the output vector as the input vector for the // next step: std::swap(input, output); } // Since we end on a "swap" above, the input vector // has the final result: return std::move(input); } Notice how the outer loop iterates [math]log_2 N[/math] times, the middle loop iterates [math]N/(2p)[/math] times, and the inner loop iterates [math]p[/math] times: Multiplying it out results in [math]O(N log N)[/math] iterations, each with a constant amount of work.Replace the straight_dft algorithm by this fft algorithm in the main() program above and you get:$ g++ -std=c++11 fft.cpp && ./a.out DFT: peak @7, amplitude = 3, phase = -90 degrees I.e., the same result at a fraction of the cost.Note that the implementation above is not tuned. For example, the computation of [math]c[/math] (the [math]c_n(N/2)[/math] in our derivation) is more expensive than it needs to be. (UPDATE (Nov. 27, 2019): See comments for some possibilities.)
- Home >
- Catalog >
- Business >
- Job Application Form >
- Walmart Job Application Form >
- Walmart Application Form >
- how much does walmart pay >
- Order Form Updated Nov