How to Edit Your Binary System Computer Definition Of Salutation In A Letter Online Easily Than Ever
Follow the step-by-step guide to get your Binary System Computer Definition Of Salutation In A Letter edited with accuracy and agility:
- Hit the Get Form button on this page.
- You will go to our PDF editor.
- Make some changes to your document, like signing, highlighting, and other tools in the top toolbar.
- Hit the Download button and download your all-set document into you local computer.
We Are Proud of Letting You Edit Binary System Computer Definition Of Salutation In A Letter With a Streamlined Workflow
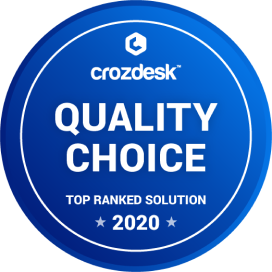
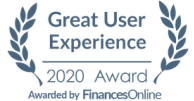
Explore More Features Of Our Best PDF Editor for Binary System Computer Definition Of Salutation In A Letter
Get FormHow to Edit Your Binary System Computer Definition Of Salutation In A Letter Online
If you need to sign a document, you may need to add text, fill out the date, and do other editing. CocoDoc makes it very easy to edit your form with just a few clicks. Let's see the easy steps.
- Hit the Get Form button on this page.
- You will go to this PDF file editor web app.
- When the editor appears, click the tool icon in the top toolbar to edit your form, like highlighting and erasing.
- To add date, click the Date icon, hold and drag the generated date to the target place.
- Change the default date by changing the default to another date in the box.
- Click OK to save your edits and click the Download button when you finish editing.
How to Edit Text for Your Binary System Computer Definition Of Salutation In A Letter with Adobe DC on Windows
Adobe DC on Windows is a useful tool to edit your file on a PC. This is especially useful when you prefer to do work about file edit offline. So, let'get started.
- Click the Adobe DC app on Windows.
- Find and click the Edit PDF tool.
- Click the Select a File button and select a file from you computer.
- Click a text box to change the text font, size, and other formats.
- Select File > Save or File > Save As to confirm the edit to your Binary System Computer Definition Of Salutation In A Letter.
How to Edit Your Binary System Computer Definition Of Salutation In A Letter With Adobe Dc on Mac
- Select a file on you computer and Open it with the Adobe DC for Mac.
- Navigate to and click Edit PDF from the right position.
- Edit your form as needed by selecting the tool from the top toolbar.
- Click the Fill & Sign tool and select the Sign icon in the top toolbar to customize your signature in different ways.
- Select File > Save to save the changed file.
How to Edit your Binary System Computer Definition Of Salutation In A Letter from G Suite with CocoDoc
Like using G Suite for your work to complete a form? You can integrate your PDF editing work in Google Drive with CocoDoc, so you can fill out your PDF without Leaving The Platform.
- Go to Google Workspace Marketplace, search and install CocoDoc for Google Drive add-on.
- Go to the Drive, find and right click the form and select Open With.
- Select the CocoDoc PDF option, and allow your Google account to integrate into CocoDoc in the popup windows.
- Choose the PDF Editor option to open the CocoDoc PDF editor.
- Click the tool in the top toolbar to edit your Binary System Computer Definition Of Salutation In A Letter on the specified place, like signing and adding text.
- Click the Download button to save your form.
PDF Editor FAQ
Which kind of data does machine learning predict accurately?
Machine learning model do not always work as expected. Nonetheless, they produce satisfactory results for many problems. I will classify statistical learning models as:ClassificationRegressionClusteringI. Applications of Classificationa) Determine whether a person has cancerThis is an example of a binary classification problem. The patients are classified as either “has cancer” or “no cancer”. The “number of Tumors” could be a feature of determining this. The number of Features p = 1. The patient is only concerned with determining whether he/she has cancer, not the relationship between the predictor variable (number of tumors) and the response variable (the classification). Hence, the goal of this cancer classification problem is a “prediction”.b) Gender Classification from NameGiven a person’s name, the objective is to determine whether the person is male or female. This is a binary classification problem with 2 response variables (“male” or “female”). Some features of the name include:Frequency of character “A”Last Letter of NameFirst Letter of NameLength of NameDepending on the region, the type of features chosen may be different. Consider a bank of Indian names both male and female. Female names usually end with an “A” and tend to be longer than male names. Thus they are good features to check. The list of 4 features mentioned above is not exhaustive. Since the problem involves only determining the gender, it is a problem of “prediction”.c) Spam ClassificationThis binary classification problem determines whether a given email is either spam/not spam. This is a well known problem in the field of Natural Language Processing. Features may include:Presence of the word “FREE”Presence of the word “Congratulations”Length of the Email (Spam Emails could be longer)Salutation (An email that does not address you by name is potential spam)The goal is to determine if a given email is either spam or not spam. There is no concern about the relationship between constituent predictor variables and the response variable (the classification as spam/not spam). Hence, this is also a problem of “prediction”.d) Prediction of Visual PerceptionThe goal is to determine the object a person sees. Consider a subset of say 6 objects (bottle, cat, chair, table, shoe, TV). Our model should take the fMRI scan of a person viewing one of those 6 objects at a given instant of time and predict the object category viewed. The features would be the brain activation all the voxels (3D Pixels) of the brain. This could be around 100,000 features, which can be reduced with feature selection techniques and dimensionality reduction. The response variable would be one of 6 classes (bottle, cat, chair, table, shoe, TV). Since there are p = 100,000 features and we may not have many training samples, it is important to modify the training dataset by analyzing the relationship between every feature (voxel) and group of features. However, the goal is still determining the object that the person visually perceives. Hence, the nature of the problem is that of “prediction”.II. Applications of Regressiona) Prediction of House PriceThe goal is to determine the price of a house, given certain features. This Regression Problem can be solved with the following features:Number of BedroomsArea in Square FeetDoes the house have a Basement (categorical field)Cost of living indexThis predictor list is not exhaustive. The price predicted is the response variable. Since we are only concerned with predicting house price over relationship with response variables, we are dealing with a “prediction” type problem.b) Determine Credit ScoreThe FICO scale is usually used to measure credit score. The goal is to compute credit score that ranges from 300 to 850. The major factors that can impact credit score:Payment History: This could be represented as rating from say 0 to 100, where 0 means one has not paid any bills on time while 100 means all payments have been made. Higher this value, higher the credit score.Amount Owed: As a predictor variable, it can be represented by the fraction of total available credit used. Lower the amount spent means one may have enough to pay off a future debt. This positively influences credit score.Length of Account History: The longer a person has kept a credit card (and payed bills), their credit score is likely to increase.The credit score is the response variable. This computation can be used by banks and money lenders to give an individual car/house/educational loans.c) YouTube AnalyticsThe goal is to determine the factors affecting revenue for a YouTube content creator. Some These factors include features such as:Demographic viewership: Who is watching your videos? This encompasses age groups, gender distribution and location. Content creators are paid more for views from the United States than the same from African countries.Subscriber count based on region: Greater the number of subscribers to the channel, larger is your audience and ability to expand viewership. This is turn leads to more revenue.Runtime of ads on every video: Creators are paid for every ad on their videos.Duration of every video: Longer videos tend to increase watch time.A content creator is interested in earning money, but also constantly trying to improve the channel. They analyze how say, the number of ads are affecting their revenue. If they see a noticeable (positive) impact on increasing ads, they keep the change and look for other ways to improve. The goal is not to only estimate the revenue (the response variable) , but find ways to increase it based on its relationship with a number of features – the basic definition of an “inference” problem. ( I have a youtube channel where I talk about data sciences. Check out CodeEmporium after reading this answer!III. Applications of clustering analysis:a) Recommender SystemsSay you run an online stores that sells movies. Once a user logs in, the objective is to recommend movies that you think the user may find interesting. This can be done by monitoring activities of the user such as:Social Media: Using Natural Language Processing, you can analyse a user’s Tweet/ Facebook Post on a Movie to see how much they liked/hated a movie.Observe Star Ratings: This is explicit feedback from the userPurchase History: Recognize the type of movies a user has watched/purchased in the past.Preferred GenreOnce this information is obtained, we can group users. When plotted graphically, movie watchers closer to each other visually have similar tastes. So if Mark, John and Ross fall in the same cluster and Ross and Mark loved the movie “Imitation Game”, we would recommend John to watch the same.b) Document categorizationConsider the situation where there are 10,000 documents that need to be grouped such that similar topics are grouped together. Some topics include:SciencesTechnologyWildlifeMovies (and more.)Unlike classification, there is no predefined set of categories. In order to categorize documents, we could define them based on properties like:TitleKeywords (The type of words used can give us an idea about the document)LengthWhen 10,000 data points plotted against a set of N Features X , we notice that similar documents are close together. A clustering technique (like K means clustering) is used to group such related data points in order to form K Clusters. When new document is required to be categorized, it is plotted using the N features. The cluster in which the point lies is the predicted cluster the document now belongs.c) Customer Segmentation (Amazon, Flip Kart)The goal is to understand consumer interests, keep track of site interaction, and categorize them to offer better products/services. Say we own an online store similar to Amazon. There is a large amount of data we can get from the consumer without explicit feedback:Cursor & Screen Position: Users are interested in different aspects of a product. Some look at the specifications, while others may pay more attention to product reviews. Knowing what a user is looking for in a product makes it easier recommend better products.Products Visited: Users may visit different products, but have not added anything to their cart. We can find the similarities between the products and guide users in their search.Products added to Cart: Certain customers on their first visit add an item to the cart, but let it go at the last minute. The next time they visit, they put the same item in their cart, but decide to change their mind (again) and not make the transaction.Based on this data collected from every consumer, we group similar customers together. This is how “People who bought products X & Y also bought product Z”, and package offers like “Buy X+Y+Z and save $5” can be used as further incentives for users to purchase products.The list goes on. Clearly there are limitless possibilities for machine learning. Phew! That took a while to write. If you liked this answer, don’t forget to follow me for more answers on Data Sciences, Machine Learning, Deep Learning. Oh, and check out my youtube channel where I talk about the same. Here is a sample video on the introduction to ML (subscribe for more):
- Home >
- Catalog >
- Finance >
- Financial Statement Form >
- Personal Financial Statement Form >
- how to fill out a personal financial statement >
- Binary System Computer Definition Of Salutation In A Letter