How to Edit The Application For Government Candidates conviniently Online
Start on editing, signing and sharing your Application For Government Candidates online refering to these easy steps:
- click the Get Form or Get Form Now button on the current page to access the PDF editor.
- hold on a second before the Application For Government Candidates is loaded
- Use the tools in the top toolbar to edit the file, and the added content will be saved automatically
- Download your modified file.
A top-rated Tool to Edit and Sign the Application For Government Candidates
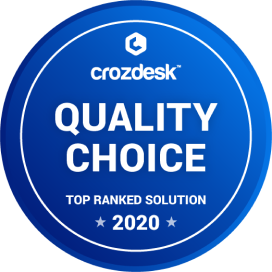
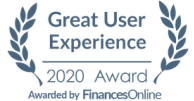
A clear direction on editing Application For Government Candidates Online
It has become really easy presently to edit your PDF files online, and CocoDoc is the best free web app you have ever used to make a lot of changes to your file and save it. Follow our simple tutorial to start!
- Click the Get Form or Get Form Now button on the current page to start modifying your PDF
- Add, modify or erase your text using the editing tools on the toolbar on the top.
- Affter editing your content, add the date and make a signature to finalize it.
- Go over it agian your form before you save and download it
How to add a signature on your Application For Government Candidates
Though most people are in the habit of signing paper documents by handwriting, electronic signatures are becoming more accepted, follow these steps to sign PDF online!
- Click the Get Form or Get Form Now button to begin editing on Application For Government Candidates in CocoDoc PDF editor.
- Click on the Sign icon in the tool box on the top
- A box will pop up, click Add new signature button and you'll have three choices—Type, Draw, and Upload. Once you're done, click the Save button.
- Move and settle the signature inside your PDF file
How to add a textbox on your Application For Government Candidates
If you have the need to add a text box on your PDF for making your special content, follow the guide to finish it.
- Open the PDF file in CocoDoc PDF editor.
- Click Text Box on the top toolbar and move your mouse to carry it wherever you want to put it.
- Fill in the content you need to insert. After you’ve typed in the text, you can use the text editing tools to resize, color or bold the text.
- When you're done, click OK to save it. If you’re not settle for the text, click on the trash can icon to delete it and start over.
An easy guide to Edit Your Application For Government Candidates on G Suite
If you are seeking a solution for PDF editing on G suite, CocoDoc PDF editor is a recommended tool that can be used directly from Google Drive to create or edit files.
- Find CocoDoc PDF editor and establish the add-on for google drive.
- Right-click on a chosen file in your Google Drive and click Open With.
- Select CocoDoc PDF on the popup list to open your file with and allow access to your google account for CocoDoc.
- Make changes to PDF files, adding text, images, editing existing text, mark with highlight, fullly polish the texts in CocoDoc PDF editor before saving and downloading it.
PDF Editor FAQ
Which are the top 20 papers related to AI (both machine learning & symbolic), so that I can cover the basics and choose a niche for my research?
Ah, the sort of challenging question that I like to ponder about on an otherwise lazy Saturday morning in the San Francisco Bay Area! I began my career in AI as a young Master’s student in Indian Institute of Technology, Kanpur, actually enrolled as a EE major, but got enchanted by Hofstadter’s Godel, Escher and Bach book into studying AI. That was 1982, so I’ve been working in AI and ML for the past 36 years. Along the way, I’ve read, oh, easily about 10,000 papers or so, give or take a few hundred. So, among these thousands of papers, now, I have to pick the “top 20 papers”, so that you, the interested Quora reader, can get a glimpse of what attracts someone like me to give up everything in pursuit of this possibly idealized quest to make machines as smart as humans and other animals. Now, there’s a challenge I can't resist.OK, any list like this is going to be 1) hopelessly biased by my personal choices 2) not entirely representative of modern AI. 3) a VERY long read! Remember that a lot of us who got into AI in the late 1970s or early 80s did so far before there was any commercial hope that AI would pay off. We were drawn to the scientific quest underlying AI: how to build a theory that explain how the brain works, how the mind was the result of the brain etc. None of us had any clue, it is safe to say, that in the early 21st century, AI would become a hugely profitable venture.But, I’m going to argue that now more than ever, it is vitally important for those entering the AI field to understand 1) where did the ideas for AI come from 2) that insights into the brain come from many fields, from neuroscience and biology to psychology and economics and from mathematics as well, so my choice of papers reflects that, and I’ve chosen papers from multiple academic fields. I’ve also not shied away from papers that are critical of things that you might believe in deeply (e.g., the power of statistical machine learning to solve potentially any AI problem).I’ll try to pepper the list with my chatty commentary as well, so it’s not going to be one of those all too boring “here’s 20 things you should know about blah”, which is all too often what you see on the web. But, with my commentary, this is going to be a really long reply. What I want to give you a glimpse of is the panoply of fascinating characters who made up this interdisciplinary quest to understand brains from a scientific and computational point of view, how diverse their backgrounds were, and what an amazingly accomplished set of minds they were. It is to their credit that AI has come along as quickly as it has, barely 60 years since it began. Without such a dazzling collection of minds working on the problem, we would have probably taken much longer to make any real progress.The list is somewhat historical and arranged chronologically as far as it is possible. I’ve also tried to keep in mind that the point of this list is that it should be comprehensible to a newbie entering the field of AI, so much as I like to include some heavy hitting math papers (of which I’ve selected a couple), I’ve included a few very sophisticated highly technical papers, since you need to get a sense of what AI is in the 21st century. So, the readability of this top 20 list varies widely: some papers are easy to get through in a Sunday afternoon. Others — well, let’s say that you’ll need several weeks of concentrated reading to make headway, assuming you have the math background. But, there are not many of the latter, so don't worry about not having the right background (yet).Let’s begin, as they say, at the beginning…..A logical calculus of the ideas immanent in nervous activity, by Warren McCullough and Walter Pitts (Univ. of Chicago), vol. 5, pp. 115–133, 1943. (http://www.cs.cmu.edu/~./epxing/Class/10715/reading/McCulloch.and.Pitts.pdf). This is the first great paper of modern computational neuroscience, written by two brilliant researchers, one senior and distinguished (McCullough), the other, Pitts, a dazzling prodigy who had had no education of any sort, but talked his way into a position with McCullough. Pitts grew up in inner city Detroit, and because he was mercilessly beaten up by gang members who were older than him, he took refuge in the Detroit Public Library. It is rumored he devoured all 1000+ pages of Bertrand Russell and Alfred North Whitehead’s Principia Mathematica in one marathon reading session of several days and nights. This is not easy reading — it is a dense logical summary of much of modern math. Pitts was brave enough, even through he was barely in high school and had had no education, to audaciously write to Bertrand Russell in England, then a famous literary figure would go on to win the Nobel Prize in literature, as well as a great mathematician, pointing out a few errors and typos in the magnum opus. This young boy so impressed Russell that later he wrote him a glowing recommendation to work with Warren McCullough. Thus was born a great collaboration, and both moved shortly to MIT, where they came under the influence of none other than Norbert Wiener, wunderkind mathematician who invented the term “cybernetics” (the study of AI in man and machines). McCullough was a larger than life character who worked all night, and seemingly subsisted on diet of “Irish whiskey and ice cream”. Pitts wrote a dazzlingly beautiful PhD thesis on “three-dimensional neural nets”, and then, as a tragic Italian opera would have it, everything fell apart. Wiener and McCullough had a falling out (so petty was the reason that I will not repeat it here), and thus, McCullough stopped being actively working with Pitt, and Pitt sort of just faded away, but sadly, not before burning the only copy of his unpublished PhD dissertation before he defended it. No copy has yet been found of this work. Read the tragic story here — warning: keep a box of Kleenex handy for at the end, you will cry! — The Man Who Tried to Redeem the World with Logic - Issue 21: Information - Nautilus (read also the classic paper “What the Frog’s Eye tells the Frog’s Brain” https://hearingbrain.org/docs/letvin_ieee_1959.pdf by the same duo. A great modern paper is the recent breakthrough in biology at Caltech where the facial code used in primate brains to identify faces has finally been cracked — The Code for Facial Identity in the Primate Brain. — showing that in one narrow area, we may know what the human eye is telling the human brain, almost 60 years after McCullough and Pitts asked the question).Steps towards Artificial Intelligence, Marvin Minsky, Proceedings of the IRE, January 1960 (http://worrydream.com/refs/Minsky%20-%20Steps%20Toward%20Artificial%20Intelligence.pdf). Many date the beginning of AI formally to this article, which really outlined the division of AI into different subfields, many of which are still around, so this paper really can be said to have been the first to lay out the modern field of AI in its current guise. Minksy was a prodigy who did a PhD in math at Princeton (like many others in AI currently and in the past), and after a dazzling postdoc at Harvard as a Fellow (where he did early work in robotics), started the highly influential MIT AI Lab, which he presided over for a number of decades. He was a larger than life character, and those who knew him well had a large stock of stories about him. Among the best I’ve heard is one where he was interviewing a faculty candidate — a rather nervous young PhD who was excitedly explaining his work on the blackboard — when the student turned around, he discovered he was alone in his office. Minksy had disappeared during his explanation. The student was mortified, but Minksy later explained that what the student had told him sounded so interesting that Minksy had to step outside and take a walk to think the ideas over. Minsky was a polymath, at home in theoretical computer science where wrote some influential papers and a book, in psychology where he was an avid disciple of Freud and wrote a paper on AI and jokes and what it meant about the subconscious, in education where he pioneered new educational learning technology, and many other fields.Programs with Common Sense, John McCarthy, in Minsky, Semantic Information Processing, pp. 403–418, 1968. (http://www-formal.stanford.edu/jmc/mcc59.ps) McCarthy was the other principal founder of AI, who after a short period of working at MIT, left to found the Stanford AI Lab, which in due course proved to be just as influential as its East coast cousin. McCarthy above all was a strong believer in the power of knowledge, and in the need for formal representations of knowledge. In this influential paper, he articulates his ideas for a software system called “an Advice Taker”, which can be instructed to do a task using hints. The Advice Taker is also endowed with common sense, and can deduce obvious conclusions from the advice given to it. For example, a self-driving car can be given the official rules of the road, as well as some advice about how humans drive (such as “in general, humans do not follow the speed limit on most highways, but tend to drive 5–10 miles above the speed limit”). Critical to McCarthy’s conceptualization, it would not be sufficient to have a neural net learn the driving task. Knowledge had to represented explicitly so it could be reasoned about. He says something profound in the paper, which may shock most modern ML researchers. He says in page 4 in italics (emphasis) that “In order for a program to be capable of learning something it must first be capable of being told it”! By this definition, McCarthy would not view most deep learning systems as really doing “learning” (for none of the deep learning systems can be told what they learn). McCarthy was also famous for his work on lambda calculus, inventing the programming language LISP, using which much of AI research was then carried out. Most of my early research in AI was done using LISP, including my first (and most highly cited paper) work on using reinforcement learning to teach robots in the early 1990s at IBM.Why should Machines Learn, by Herbert Simon, in Michalski, Carbonell, and Mitchell (editors), Machine Learning, 1983 (http://digitalcollections.library.cmu.edu/awweb/awarchive?type=file&item=33928). Herb Simon was a Nobel laureate in Economics, who spent his entire academic career at Carnegie Institute of Technology (later Carnegie Mellon University), doing much to build the luster and prestige of this now world-class university. He was one of the true polymaths, at home in half a dozen departments, from computer science to economics to business administration and psychology, in all of which he made foundational contributions. He was a gifted speaker, and I was particularly fortunate to be able to attend several presentations by Simon during the mid 1980s when I spent several years at CMU. In this article, Simon asks the question that very few AI researchers today bother asking: why should machines learn? According to Simon, why should machines, which can be programmed, bother with this slow and tedious form of knowledge acquisition, when something far quicker and more reliable is available. You’ll have to read the article to find his answers, but this article is valuable for giving perhaps the first scientific definition of the field of machine learning, a definition that is still valid today. Simon made many other contributions to AI, including his decades long collaboration with Allan Newell, another AI genius at CMU, whose singular ability in asking the right questions, made him a truly gifted researcher. It is rumored that computer chess came to life when Allan Newell mentioned casually in a conversation in the CMU CS common room about how the branching factor of chess is not all difficult to emulate in hardware, a comment that Hans Berliner followed up on in bringing the first modern chess player, Deep Thought, to fruition (the same CMU team went to IBM, built Deep Blue which of course beat Kasparov).Non-cooperative games, PhD thesis, John Nash, Princeton. (Non-Cooperative Games) John Nash came to Princeton as a 20 year old mathematician from Carnegie Institute of Technology in 1948 with a one line recommendation letter: “This man is a genius”. His PhD thesis would fully affirm his alma mater’s assessment of his capabilities. Nash took the work of von Neumann and Morgenstern’s zero-sum games into a whole new level with his dazzling generalization, which would earn him a Nobel prize decades later. Most of Nash’s history has been recounted in Sylvia Nasar’s wonderful biography A Beautiful Mind (later made into a movie starring Russell Crowe as John Nash). Legend had it that von Neumann himself did not think much of Nash’s work, calling it “another fixed point theorem”. Nash finished his ground breaking thesis in less than a year from start to finish. He arrived in Princeton in September 1948, and in November 1949, Solomon Lefschetz, a distinguished mathematician communicated the results of Nash’s thesis to the National Academy of Sciences meeting. Today, billions of dollars of product (from wireless cellular bandwidths to oil prospects) are traded using Nash’s ideas of game theory. The most influential model in deep learning today is the Generative Adversarial Network (GAN), and the key question being studied for GANs is whether and when do they converge to a Nash equilibrium. So, 70 years after Nash defended his short but Nobel prize winning thesis at Princeton, his work is still having a huge impact in ML and AI. Nash’s work also become a widely used framework to study evolutionary dynamics, giving rise to a new field called evolutionary game theory, pioneer by John Maynard Smith. Game theory is a crucial area for not only AI but also for CS. It has been said that the “Internet is just a game. We have to find what the equilibrium solution is”. Algorithmic game theory is a burgeoning area of research, studying things like “The Price of Anarchy”, or how solutions to hard optimization problems can be solved by letting millions of agents make locally selfish decisions. Nash’s PhD advisor at Princeton was Tucker, who Nash called “The Machine”. His second reader of his PhD thesis was Turkey, who can be called one of the fathers of modern machine learning, since he invented exploratory data analysis at Princeton (and later also invented the Fast Fourier Transform).Maximum likelihood from Incomplete Data using the EM Algorithm, Dempster, Laird, and Rubin (Journal of the Royal Statistical Society, Series B, 1977) (Maximum Likelihood from Incomplete Data via the EM Algorithm). In the mid 1980s, ML took a dramatic turn, along with AI, towards the widespread use of probabilistic and statistical methods. One of the most influential models of machine learning during the 1990s was based on Fisher’s notion of maximum likelihood estimation. Since most interesting probabilistic models in AI had latent (unobserved) variables, maximum likelihood could not be directly applied. The EM algorithm, popularized by three Harvard statisticians, came the rescue. It is probably the most widely used statistical method in ML in the past 25 years, and well worth knowing. This paper, which is cited over 50,000 times on Google Scholar, requires a certain level of mathematical sophistication, but it is representative of modern ML, and much of the edifice of modern ML is based on ideas like EM. A very simple way to think of EM is in terms of “data hallucination”. Let’s say you want to compute the mean of 20 numbers, but forgot to measure the last 5 numbers. Well, you could compute the mean over the 15 numbers only, or you could do something clever, namely put in an initial guess of the mean for each of the missing 5 numbers. This leads to an easy recurrence relation that lets you find the true mean. In the one dimensional case, this happens to be the same as ignoring the last 5 numbers, but in the two dimensional case, where one or the other dimension may be different, EM finds a different solution.A Theory of the Learnable, by Les Valiant, Communications of the ACM, 1984. (https://people.mpi-inf.mpg.de/~mehlhorn/SeminarEvolvability/ValiantLearnable.pdf). George Orwell wrote a brilliant novel about the rise of the all powerful all knowing Government, which spies on everyone. Well, in the same year of the novel, Les Valiant, a brilliant computer scientist at Harvard proved that Orwell’s fears could not be completely realized due to intrinsic limitations on what can be learned from data in polynomial time. That is, even if the Government could spy on individuals, it is possible to construct functions whose identity may be hidden because it would require intractable computation to discover them. Valiant’s work lead to his winning the Turing award several decades later, computer science’s version of the Nobel Prize. What Valiant did in this landmark paper was articulate a theory of machine learning that is analogous to complexity theory for computation. He defined PAC learning, or probably approximately correct learning, as a model of knowledge acquisition from data, and showed examples where a class of functions was PAC learnable, and also speculated about non-learnable functions. Valiant’s work in the past three decades has been hugely influential. For example, the most widely used ensemble method in ML is called boosting, and came out as a direct result of PAC learning. Also to be noted is that support vector machines or SVMs were justified using the tools of PAC learning. This is a short but beautifully written paper, and while it is not an easy read, your ability to understand and grasp this paper will make the difference between whether you are a ML scientist or an ML programmer (not to make any value judgements of either, the world needs plenty of both types of people!).Intelligence without representation, Rodney Brooks, IJCAI 1987 Computers and Thought Award lecture (http://www.fc.uaem.mx/~bruno/material/brooks_87_representation.pdf). Brooks based his ideas for building “behavior-based robots” on ethology, the study of insect behavior. What ethologists found was that ants, bees, and lots of other insects were incredibly sophisticated in their behaviors, building large complex societies (ant colonies, bee hives), and yet their decision making capacity seemed to be based on fairly simple rules. Brooks took this type of idea to heart, and launched a major critique at the then representation heavy apparatus of modern knowledge-based AI. He argued that robots built using knowledge-based AI would never function well enough in the real world to survive. A robot crossing the road that sees a truck and begins to reason about what it should do would get flattened by the truck before its reasoning engine came up with a decision. According to Brooks, this failure was due to a misunderstanding of how brains are designed to produce behavior. In animals, he argued, behaviors are hard wired in a layered highly modularized form, so that complexity emerges from the interleaving of many simple behaviors. One of his early PhD students, Jonathan Connell, showed that you can design a complex robot, called Herbert (after Herb Simon), that could do a complex task of searching an indoor building for soda cans and picking them and throwing them into trash, all the while having no explicit representation anywhere of the task. Later, after Jon Connell graduated, he came to work for IBM Research, where he and I collaborated on applying RL to teach behavior-based robots new behaviors. Brooks was a true pioneer of robotics, and inserted a real-world emphasis in his work that was till then sorely lacking. He had a common-sense wisdom about how to apply the right sort of engineering design to a problem, and was not enamored of using fancy math to solve problems that had far simpler solutions. Much of the success of modern autonomous driving systems owes something to Brooks’ ideas. It is possible that the tragic accident in Arizona involving an Uber vehicle might have been averted had that particular vehicle been outfitted with a behavior-based design (which countermands bad decisions, like the one the Uber vehicle allegedly made, of labeling he pedestrian as a false positive).Natural Gradient Works Efficiently in Learning, Amari, Neural Computation, 1989 (http://citeseerx.ist.psu.edu/viewdoc/download?doi=10.1.1.452.7280&rep=rep1&type=pdf). One of the living legends of statistics is the Indian scientist C.R. Rao, now in his 90s, who basically has done the most since Fisher in building up the edifice of modern statistics. C. R. Rao invented much of modern multivariate statistics as a young researcher at Univ. of Cambridge, England, due to his study of fossils of human bones from Ethiopia. In a classic paper written in his 20s, C. R. Rao showed that the space of probability distributions is curved, like Einstein’s space-time, and has a Riemannian inner product defined on the space of tangents at each point on its surface. He later showed how the Fisher information metric could be used to define this inner product. Amari, a brain science researcher in Japan, used this insight to define natural gradient methods, a widely used class of methods to train neural networks, where the direction pursued to modify the weights at any given point is not the Euclidean direction, but the direction that is based on analyzing the curved structure of the underlying probability manifold. Amari showed natural gradient often works better, and later wrote a highly sophisticated treatise on information geometry, expanding on his work on natural gradients. Many years later, in 2013, a group of PhD students and I showed that natural gradient methods could actually be viewed as special cases of a powerful class of dual space gradient methods called mirror descent, invented by Russian optimization researchers Nemirovksy and Yudin. Mirror descent has now become a basis for one of the most widely used gradient methods in deep learning called ADAGRAD by Duchi (now at Stanford), Hazan (now at Princeton) and Singer (now at Google). It is very important to understand these various formulations of gradient descent methods, which requires exploring some beautiful connections between geometry and statistics.Learning to Predict by the Methods of Temporal Differences, by Richard Sutton, Machine Learning journal, pp 9–44, 1988 (https://pdfs.semanticscholar.org/9c06/865e912788a6a51470724e087853d7269195.pdf). TD learning remains the most widely used reinforcement learning method, 34 years after they were invented by UMass PhD student Richard Sutton, working in collaboration with his former PhD advisor, Andrew Barto, both of whom can be said to have laid the foundations of the modern field of RL (on whose work the company Deep Mind was originally formed, and then acquired by Google). It is worth noting that Arthur Samuel in 1950s experimented with a simple form of TD learning, and used it to teach an IBM 701 to play checkers, which can be said to be the first implementation of both RL and ML in the modern era. But Rich Sutton brought TD -learning to life, and if you read the above paper, you’ll see the mathematical sophistication he brought to its study was far beyond Samuel. TD learning is now far beyond this paper, and if you want to see how mathematically sophisticated its modern variants are, I will point you to the following paper (which builds on the work of one of my former PhD students, Bo Liu, who brought the study of gradient TD methods to a new level with his work on dual space analysis). Janet Yu has written a very long (80+ pages) dense mathematical treatise on the modern version of gradient TD, which you have to be very strong in math to understand fully ([1712.09652] On Convergence of some Gradient-based Temporal-Differences Algorithms for Off-Policy Learning). TD remains one of the few ML methods for which there is some evidence that it is biologically plausible. The brain seems to encode TD error using dopamine neurotransmitters. The study of TD in the brain is a very active area of research (see http://www.gatsby.ucl.ac.uk/~dayan/papers/sdm97.pdf).Human learning in Atari, Tsividis et al., AAAI 2017 (http://gershmanlab.webfactional.com/pubs/Tsividis17.pdf). Deep reinforcement learning was popularized in a sensational paper in Nature (Human-level control through deep reinforcement learning) by a large group of Deep Mind researchers, and it is by now so well known and cited that I resisted the temptation to include it in my top 20 list (where most people would put it). It has led to large numbers of follow on papers, but many of these seem to miss the fairly obvious fact that there is a huge gulf between the speed at which humans learn Atari games and TD Q-learning with convolutional neural nets does so. This beautiful paper by cognitive scientists at MIT and Harvard shows that humans learn many of the Atari games in a matter of minutes in real time play, whereas deep RL methods require tens of millions of steps (which would be many months of human time, perhaps even years!). So, deep RL cannot be the ultimate solution to the Atari problem, even if it is currently perhaps the best we can do. There is a huge performance gap between humans and machines here, and if you are a young ML researcher, this is where I would go to make the next breakthrough. Humans seem to do much more than deep RL when learning to play Atari.Distributed Optimization and Statistical Learning via the Alternating Direction Method of Multipliers, Boyd et al., Foundations and Trends in Machine Learning, 2011 (Distributed Optimization and Statistical Learning via the Alternating Direction Method of Multipliers, which has MATLAB code as well). The 21st century has arrived, and with it, the dawn of cloud computing, and machine learning is poised to exploit these large numbers of cloud based computational structures. This very long and beautifully written paper by Stanford optimization guru Stephen Boyd and colleagues shows how to design cloud based ML algorithms using a broad and powerful framework called Alternating Direction Method of Multipliers (or ADMMs). As the saying goes in the Wizard of Oz, “we are no longer in Kansas, Toto”. Namely, with this paper, we are now squarely in modern machine learning land, where the going gets tough (but, then as the saying goes, “the tough get going”). This is a mathematically deep and intense paper, of more than 100 pages, so it is not an easy read (unless, that is, your are someone like Walter Pitts!). But, the several weeks or months you spend reading it will greatly improve your ability to see how to exploit modern optimization knowledge to speed up many machine learning methods. What is provided here is a generic tool box, and you can design many specialized variants (including Hadoop based variants, as shown in the paper). To understand this paper, you need to understand duality theory, and Boyd himself has written a nice book on convex optimization to help you bridge that chasm. The paper is highly cited, for good reason, as it is a model of clarity.Learning Deep Architectures for AI, by Bengio, TR 1312, Univ. of Montreal (https://www.iro.umontreal.ca/~lisa/pointeurs/TR1312.pdf) (also a paper published in the journal Foundations and Trends in Machine Learning). Bengio has done more than almost anyone else in popularizing deep learning, and is also one of its primary originators and innovators. In this paper, he lays out a compelling vision for why AI and ML systems need to incorporate ideas from deep learning, and while many of the specifics he says have changed due to the rapid progress in deep learning in the last few years, this paper is a classic that bears well. This paper was written as counter point to the then popular approach of shallow architectures in machine learning, such as kernel methods. Bengio is giving another of his popular tutorials on deep learning at the forthcoming IJCAI conference in July in Sweden, in case you are interested in attending the conference or the tutorial. I don't have to say much more about deep learning, as it is the subject of a barrage of publicity these days. Suffice it to say that today AI is very much in the paradigm of deep learning (meaning a framework in which every problem is posed as a problem of deep learning, whether it is the right approach or not!). Time will tell how well deep learning survives in its current form. There are beginning to be worries about the robustness of deep learning solutions (the Imagenet architectures seem very vulnerable to random noise, which humans can’t even see, let along respond to), and the sample complexity seems formidable still. Scalability remains an open question, but deep learning has shown remarkable performance in many areas, including computer vision (if you download the latest version of MATLAB R2018a, you can run the demo image recognition program with a web cam with objects in your own house, and decide for yourself how well you think deep learning works in the real world).Theoretical Impediments to Machine Learning, with Seven Sparks from the Causal Revolution, by Judea Pearl, Arxiv 2018. ([1801.04016] Theoretical Impediments to Machine Learning With Seven Sparks from the Causal Revolution). Pearl is in my view the Isaac Newton of AI. He developed the broad probabilistic framework of graphical models, which dominated AI in the 1990s-2010s. He subsequently went into a different direction with his work on causal models, and now argues that probabilities are “an epiphenomenon” (or a surface property, of a much deeper causal truth). Pearl’s work on causal models has yet to gain the same traction in AI as his earlier work on graphical models (which is a major subfield in both AI and ML). Largely, the reasons have to do with the sort of applications that causal models fit well with. Pearl is focusing on domains like healthcare, education, climate change, societal models etc. where interventions are needed to change the status quo. In these hugely important practical applications, he argues that descriptive statistics is not the end goal, but causal models are. His 2009 2nd edition of Causality is still the most definitive modern treatment of the topic, and well worth acquiring.Prospect Theory: An Analysis of Decisions under Risk, by Daniel Kahneman and Amos Tversky, Econometrica, pp. 263–291, 1979. Daniel Kahneman received the Nobel prize in Economics for this work, with his collaborator Amos Tversky (who sadly died, and could not share in the prize). In this pathbreaking work, they asked themselves the simple question: how do humans make decisions under uncertainty? Do they follow the standard economic model of maximizing expected utility? If I gave you the choice between two outcomes: choose Door 1, and with 50% probability, you get no cash prize, or you get $300; alternatively if you choose Door 2, you get a guaranteed prize of $100. It perhaps won't surprise you that many humans choose Door 2, even through expected utility theory shows you should choose Door 1( since the expected utility is $150, much higher than Door 2). What’s going on? Well, humans tend to be risk averse. We would rather have the $100 for sure, than risk getting nothing with Door 1. This beautiful paper, which has been cited over 50,000 times, explores such questions in a number of beautiful simple experiments that been repeated all over the world with similar results. Well, here’s the rub. Much of the theory of modern probabilistic decision making and reinforcement learning in AI is based on maximizing expected utility (Markov decision processes, Q-learning, etc.). If KT is right, then much of modern AI is barking up the wrong tree! If you care about how humans actually make decisions, should you continue to chose an incorrect approach? Your choice. Read this paper and decide.Towards an Architecture for Never-ending Language Learning, Carlson et al., AAAI 2010. Humans learn over a period of decades, but most machine learning systems learn over a much shorter period of time, often just a single task. This CMU effort led by my former PhD advisor, Thomas Mitchell, explores how a machine learning system can learn over a very long period of time, by exploring the web, and learning millions of useful facts. You can interact with the actual NELL system online at Carnegie Mellon University. NELL is a fascinating example of how the tools of modern computer technology, namely the world wide web, makes it possible to design ML systems that can run forever. NELL could potentially live longer than any of us, and constantly acquire facts. One issue, at the heart of recent controversies, is “fake news”, of course. How does NELL know what it has learned is true? The web is full of fake assertions. NELL currently uses a human vetting approach of deciding which facts it learns are really to be trusted. Similar systems can be designed for image labeling, language interactions, and many others.Topology and Data, by Gunnar Carlson, Bulletin of the American Mathematical Society, April 2009 (http://www.ams.org/images/carlsson-notes.pdf). The question that many researchers are interested in knowing the answer to is: where is ML going in the next decade? This well known Stanford mathematician is arguing in favor of the use of more sophisticated methods from topology, a well developed area of math that studies the abstract properties of shape. Topology is what mathematicians use to decide that a coffee cup (with a handle) and a doughnut are essentially the same, since one can be smoothly deformed into the other without cutting. Topology has one great strength: it can be used to analyze data even when standard smoothness assumptions in ML are not possible to make. It goes without saying that the mathematical sophistication needed here is quite high, but Carlson refrains from getting very deep into the technical subject matter, giving for the most part, high level examples of what structure can be inferred using the tools of computational topology.2001: A Space Odyssey, book by Arthur C. Clarke, and movie by Stanley Kubrick. My next and last choice of reading — this has gone on long enough, and both you and I are getting a bit tired by now — is not an AI paper, but a movie and the associated book. The computer HAL in Kubrick’s movie 2001 is to my mind the best exemplar of an AI based intelligent system, one that is hopefully realizable soon. 2001 was released in 1968, exactly 50 years from now, and its 50th anniversary was marked recently. Many of my students and colleagues, I find, have not seen 2001. That is indeed a sacrilege. If you are all all interested in AI or ML, you owe it to yourself to see this movie, or read the book, and preferably do both. It is in my mind the most intelligent science fiction movie ever made, and it puts all later movies to shame (no, there is no silly laser sword fights or fake explosions or Darth Vaders here!). Instead, Stanley Kubrick designed the movie to be as realistic as the technology from 1960s would allow, and it is surprisingly modern even today. HAL is of course legendary from his voice (“I’m sorry Dave” is now available as a ring tone on many cellphones). But, HAL is also a great example of how modern AI will work with humans, and help assist many functions. Many long voyages into space, such as Mars or beyond, cannot be done with a HAL, as humans will have to sleep or be in hibernation to save on storage for food etc. There is a nice book by Stork on doing a scene by scene analysis of HAL in the movie, with where AI is in the 21st century. This book is also worth acquiring.OK, I’m ending this uber long reply two papers short of the required 20, but I’m sure I’ve given you plenty of material to read and digest. I did also cheat a bit here and there, and gave you multiple papers to read per bullet. Happy reading. Hope your journey into the fascinating world of AI is every bit as rewarding and fun as mine has been over the past 30+ years.
What are some instances where Trump was racist?
Here’s some lists and excerpts of articles, assembled from sources that I cite below:1973: The US Department of Justice — under the Nixon administration, out of all administrations — sued the Trump Management Corporation for violating the Fair Housing Act. Federal officials found evidence that Trump had refused to rent to black tenants and lied to black applicants about whether apartments were available, among other accusations. Trump said the federal government was trying to get him to rent to welfare recipients. In the aftermath, he signed an agreement in 1975 agreeing not to discriminate to renters of color without admitting to discriminating before.1980s: Kip Brown, a former employee at Trump’s Castle, accused another one of Trump’s businesses of discrimination. “When Donald and Ivana came to the casino, the bosses would order all the black people off the floor,” Brown said. “It was the eighties, I was a teenager, but I remember it: They put us all in the back.”1988: In a commencement speech at Lehigh University, Trump spent much of his speech accusing countries like Japan of “stripping the United States of economic dignity.” This matches much of his current rhetoric on China.1989: In a controversial case that’s been characterized as a modern-day lynching, four black teenagers and one Latino teenager — the “Central Park Five” — were accused of attacking and raping a jogger in New York City. Trump immediately took charge in the case, running an ad in local papers demanding, “BRING BACK THE DEATH PENALTY. BRING BACK OUR POLICE!” The teens’ convictions were later vacated after they spent seven to 13 years in prison, and the city paid $41 million in a settlement to the teens. But Trump in October 2016 said he still believes they’re guilty, despite the DNA evidence to the contrary.1991: A book by John O’Donnell, former president of Trump Plaza Hotel and Casino in Atlantic City, quoted Trump’s criticism of a black accountant: “Black guys counting my money! I hate it. The only kind of people I want counting my money are short guys that wear yarmulkes every day. … I think that the guy is lazy. And it’s probably not his fault, because laziness is a trait in blacks. It really is, I believe that. It’s not anything they can control.” Trump at first denied the remarks, but later said in a 1997 Playboy interview that “the stuff O’Donnell wrote about me is probably true.”1992: The Trump Plaza Hotel and Casino had to pay a $200,000 fine because it transferred black and women dealers off tables to accommodate a big-time gambler’s prejudices.1993: In congressional testimony, Trump said that some Native American reservations operating casinos shouldn’t be allowed because “they don’t look like Indians to me.”2000: In opposition to a casino proposed by the St. Regis Mohawk tribe, which he saw as a financial threat to his casinos in Atlantic City, Trump secretly ran a series of ads suggesting the tribe had a “record of criminal activity [that] is well documented.”2004: In season two of The Apprentice, Trump fired Kevin Allen, a black contestant, for being overeducated. “You’re an unbelievably talented guy in terms of education, and you haven’t done anything,” Trump said on the show. “At some point you have to say, ‘That’s enough.’”2005: Trump publicly pitched what was essentially The Apprentice: White People vs. Black People. He said he “wasn’t particularly happy” with the most recent season of his show, so he was considering “an idea that is fairly controversial — creating a team of successful African Americans versus a team of successful whites. Whether people like that idea or not, it is somewhat reflective of our very vicious world.”2010: In 2010, there was a huge national controversy over the “Ground Zero Mosque” — a proposal to build a Muslim community center in Lower Manhattan, near the site of the 9/11 attacks. Trump opposed the project, calling it “insensitive,” and offered to buy out one of the investors in the project. On The Late Show With David Letterman, Trump argued, referring to Muslims, “Well, somebody’s blowing us up. Somebody’s blowing up buildings, and somebody’s doing lots of bad stuff.”2011: Trump played a big role in pushing false rumors that Obama — the country’s first black president — was not born in the US. He even sent investigators to Hawaii to look into Obama’s birth certificate. Obama later released his birth certificate, calling Trump a ”carnival barker.” (The research has found a strong correlation between “birtherism,” as this conspiracy theory is called, and racism.) Trump has reportedly continued pushing this conspiracy theory in private.2011: While Trump suggested that Obama wasn’t born in the US, he also argued that maybe Obama wasn’t a good enough student to have gotten into Columbia or Harvard Law School, and demanded Obama release his university transcripts. Trump claimed, “I heard he was a terrible student. Terrible. How does a bad student go to Columbia and then to Harvard?”Trump launched his campaign in 2015 by calling Mexican immigrants “rapists” who are “bringing crime” and “bringing drugs” to the US. His campaign was largely built on building a wall to keep these immigrants out of the US.As a candidate in 2015, Trump called for a ban on all Muslims coming into the US. His administration eventually implemented a significantly watered-down version of the policy.When asked at a 2016 Republican debate whether all 1.6 billion Muslims hate the US, Trump said, “I mean a lot of them. I mean a lot of them.”He argued in 2016 that Judge Gonzalo Curiel — who was overseeing the Trump University lawsuit — should recuse himself from the case because of his Mexican heritage and membership in a Latino lawyers association. House Speaker Paul Ryan, who endorsed Trump, later called such comments “the textbook definition of a racist comment.”Trump has been repeatedly slow to condemn white supremacists who endorse him, and he regularly retweeted messages from white supremacists and neo-Nazis during his presidential campaign.He tweeted and later deleted an image that showed Hillary Clinton in front of a pile of money and by a Jewish Star of David that said, “Most Corrupt Candidate Ever!” The tweet had some very obvious anti-Semitic imagery, but Trump insisted that the star was a sheriff’s badge, and said his campaign shouldn’t have deleted it.Trump has repeatedly referred to Sen. Elizabeth Warren (D-MA) as “Pocahontas,” using her controversial — and later walked-back — claims to Native American heritage as a punchline.At the 2016 Republican convention, Trump officially seized the mantle of the “law and order” candidate — an obvious dog whistle playing to white fears of black crime, even though crime in the US is historically low. His speeches, comments, and executive actions after he took office have continued this line of messaging.In a pitch to black voters in 2016, Trump said, “You’re living in poverty, your schools are no good, you have no jobs, 58 percent of your youth is unemployed. What the hell do you have to lose?”Trump stereotyped a black reporter at a press conference in February 2017. When April Ryan asked him if he plans to meet and work with the Congressional Black Caucus, he repeatedly asked her to set up the meeting — even as she insisted that she’s “just a reporter.”In the week after white supremacist protests in Charlottesville, Virginia, in August 2017, Trump repeatedly said that “many sides” and “both sides” were to blame for the violence and chaos that ensued — suggesting that the white supremacist protesters were morally equivalent to counterprotesters that stood against racism. He also said that there were “some very fine people” among the white supremacists. All of this seemed like a dog whistle to white supremacists — and many of them took it as one, with white nationalist Richard Spencer praising Trump for “defending the truth.”Throughout 2017, Trump repeatedly attacked NFL players who, by kneeling or otherwise silently protesting during the national anthem, demonstrated against systemic racism in America.Trump reportedly said in 2017 that people who came to the US from Haiti “all have AIDS,” and he lamented that people who came to the US from Nigeria would never “go back to their huts” once they saw America. The White House denied that Trump ever made these comments.Speaking about immigration in a bipartisan meeting in January 2018, Trump reportedly asked, in reference to Haiti and African countries, “Why are we having all these people from shithole countries come here?” He then reportedly suggested that the US should take more people from countries like Norway. The implication: Immigrants from predominantly white countries are good, while immigrants from predominantly black countries are bad.Trump denied making the “shithole” comments, although some senators present at the meeting said they happened. The White House, meanwhile, suggested that the comments, like Trump’s remarks about the NFL protests, will play well to his base. The only connection between Trump’s remarks about the NFL protests and his “shithole” comments is race.Trump mocked Elizabeth Warren’s presidential campaign, again calling her “Pocahontas” in a tweet before adding, “See you on the campaign TRAIL, Liz!” The capitalized “TRAIL” is seemingly a reference to the Trail of Tears — a horrific act of ethnic cleansing in the 19th century in which Native Americans were forcibly relocated, causing thousands of deaths.Trump tweeted that several black and brown members of Congress — Reps. Alexandria Ocasio-Cortez (D-NY), Ayanna Pressley (D-MA), Ilhan Omar (D-MN), and Rashida Tlaib (D-MI) — are “from countries whose governments are a complete and total catastrophe” and that they should “go back” to those countries. It’s a common racist trope to say that black and brown people, particularly immigrants, should go back to their countries of origin. Three of four of the members of Congress whom Trump targeted were born in the US.Source: Donald Trump’s long history of racism, from the 1970s to 2019A similar timeline can be found here: Trump’s history of racial controversies - AxiosAnother analysis:In 1989, he told Bryant Gumbel in an interview, “A well-educated black has a tremendous advantage over a well-educated white in terms of the job market…if I was starting off today, I would love to be a well-educated black, because I really do believe they have the actual advantage today. “ In fact, all the serious studies refuted that. However his statement did serve as a kind of shout-out to those who were ignorant about the racial dynamics in the U.S. economy.Earlier in that same year Trump helped fan the flames of racial resentment when black and Latino teens were arrested in the infamous “Central Park jogger” attack. Trump alone chose to pay for $85,000 worth of full-page newspaper ads trumpeting, in capital letters, “BRING BACK THE DEATH PENALTY. BRING BACK OUR POLICE!” In the text Trump objected to then-Mayor Ed Koch’s plea for peace: Mayor Koch stated that “hate and rancor should be removed from our hearts. I do not think so.”A telling moment arose during a 1993 Congressional committee hearing on gambling casino operated by Indian tribes. Trump, who considered the tribes competitors, offered a flourish of insensitivity during his testimony when he said, “They don’t look like Indians to me and they don’t look like Indians to Indians.”Trump also said that tribal gaming operators were somehow tied to organized crime and a scandal was about to erupt. “In the 19 years I have been on this committee, I have never seen such irresponsible remarks,” Rep. George Miller (D., Calif.) shouted back to Trump. (Decades later, the industry is still waiting for the scandal that Trump predicted.)Source: Is Donald Trump Racist? Here's what the record showsThe following is from the Brookings Institute:Donald Trump’s support in the 2016 campaign was clearly driven by racism, sexism, and xenophobia. While some observers have explained Trump’s success as a result of economic anxiety, the data demonstrate that anti-immigrant sentiment, racism, and sexism are much more strongly related to support for Trump. Trump’s much-discussed vote advantage with non-college-educated whites is misleading; when accounting for racism and sexism, the education gap among whites in the 2016 election returns to the typical levels of previous elections since 2000. Trump did not do especially well with non-college-educated whites, compared to other Republicans. He did especially well with white people who express sexist views about women and who deny racism exists.Even more alarmingly, there is a clear correlation between Trump campaign events and incidents of prejudiced violence. FBI data show that since Trump’s election there has been an anomalous spike in hate crimes concentrated in counties where Trump won by larger margins. It was the second-largest uptick in hate crimes in the 25 years for which data are available, second only to the spike after September 11, 2001. Though hate crimes are typically most frequent in the summer, in 2016 they peaked in the fourth quarter (October-December). This new, higher rate of hate crimes continued throughout 2017.The association between Trump and hate crimes is not limited to the election itself. Another study, based on data collected by the Anti-Defamation League, shows that counties that hosted a Trump campaign rally in 2016 saw hate crime rates more than double compared to similar counties that did not host a rally.The data analysis discussed above has centered on correlations; they are suggestive of a link between Trump and racist attitudes and behavior, but do not actually demonstrate that one leads to the other. However, there is also causal evidence to point to. In experiments, being exposed to Trump’s rhetoric actually increases expressions of prejudice.In a 2017 survey, researchers randomly exposed some respondents to racist comments by the president, such as: “When Mexico sends its people, they’re not sending their best. They’re sending people that have lots of problems… They’re bringing drugs. They’re bringing crime. They’re rapists. And some, I assume, are good people.”Other respondents were exposed to a statement by Hillary Clinton condemning prejudiced Trump supporters. Later in the study, the respondents were asked their opinion of various groups, including Mexican people, black people, and young people. Those who had read Trump’s words were more likely to write derogatory things not only about Mexican people, but also about other groups as well. By contrast, those who were exposed to Clinton’s words were less likely to express offensive views towards Muslims. Words do matter, and data prove it.Source: Trump and racism: What do the data say?Also consider incidents cited here: PolitiFact - Allegations of racism against Trump didn't start when he challenged the DemocratsReview the above and draw your own conclusions. I suggest the conclusion is rather clear.
Is Narendra Modi fit to be the prime minister of India? Why or why not?
Modi is the worst PM of India.1.Ticket to Criminal Candidates :A report in Hindu says that 57 members of Gujarat cabinet have criminal cases on them. Well, I seriously don’t understand how my India will shine under criminal candidates. Can BJP stop giving tickets to criminals ? if yes,then I can think to vote for them.2 . Right to Information Act (RTI) –RTI activists in Gujarat are suffering , their RTI Applications are not responded and thousands of RTI applications are pending. Gujarat RTI Activists Association, which has around 5,000 members, says that there are around 14,000 RTI applications pending at different stages in various departments of the state government. According to them, these RTIs can expose Corruption on larger scale. So stopping an RTI and Modi ji wants India to be like USA, no that is not possible if you stop the Right To Information for your citizens.Apart from this , why Modi has to keep tainted minister in his Cabinet? Many RTI activists have received threats to life , RTI Activist Amit Jethwa was murdered and BJP MP Dinu Bogha Solanki has been held . Freedom of knowledge and Free Speech which can threaten the govt is silenced . BJP MP arrested for RTI Activists Murder3. Weak Lokayukta :In April2012, Modi ji passed a new bill, The Gujarat Lokayukta Aayog which allows him to1. Choose the Lokayukta of his own choice.2. Block Lokayukta from investigating any cases against himself or his cabinet ministers.3. Remove the primacy of high court chief justice and the governor in the appointment of Lokayukta. ReadSupreme Court Upholds Gujarat’s Lokayukta Appointment And Mr Modi was slammedIs this what you call a strong Lokpal, when you criticize central government for bringing strong Lokpal, Mr Modi? If this is what you call a strong Lokpal, then all corrupt politicians would have backed Anna Hazare for this bill.4. No Riots after 2002: Narendra Modi ji has claimed Many times that there are no riots in Gujarat after 2002, any intelligent person would try to do a re-search on this claim . No Riots in Gujarat in Last 12 years – ModiFacts : Large scale riots happened within Vadodara in 2006 (no coincidence that next Gujarat elections were in 2007), Apart from that, regular stream of incidences involving arson, stone-pelting, stabbing, lynching etc kept on happening regularly all over Gujarat – Ahmedabad, Rajkot, Dohad, Veraval etc.4. False Promotions and fudging of facts , there are many examples of the same. i will list out some.a) Claim: Modi Claims that Gujarat has generated 25 lakh jobs generated in 2009 link and also said that jobs generated will be 52 lakh in 2011 . 52 lakh jobs in 2011.Truth: Planning Commission Employment Report, page no 126, suggests that, number of people employed in major sectors has come down from 25.3 million in 2004-05 to 24.65 million in 2009-10. Check Page number 126, Planning Commission Employment ReportSo, these high figures are for what when the jobs have gone down from 2004 to 2009 ?b) Education : let’s look at the Gujarat’s education Sector .Gujarat is the worst state in the country, when we look at the reading, writing and math skills of the rural students. Planning Commission reports Education Report. Only 7% of Gujarat’s class 5 kids can read English sentences, way below all India average and it’s better not to compare with other states.ASER survey taken in Jan 2013, shows clearly this fact. ASER surveyA major part of survey was reported by DNA which can be read here. Gujarat’s Class 8 literates can’t read.Its clear that Mr Modi is too busy in infrastructure and building roads that he doesn’t have time for future generations, he is aware that they can’t vote.b) TOURISM : Under Modi, Gujarat has turned out to be the visitors favourite destination in India. this is a quote from Modi’s website : Potential of Gujarat TourismHere is a quote from Modi’s website : ‘In the last year Gujarat got over 22 million tourists and witnessed a tourism growth rate of over 16%, which is double of the national tourism growth rate. From Rs 30 crore in 2005-2006, the tourism budget today is Rs 500 crore.’As recorded in Gujarat’s official tourism website Gujarat Tourism.Number of tourists during 2010-11 = 19,811,936 (19.8 million)Number of tourists during 2011-12 = 22,354,665 (22.3 million)Anyone who can do simple calculation would find out that, increase is around 13% (12.8% to be exact) and not 16% as Modi tells the nation.c) FALSE CLAIMS : Automobile majors are flocking to Gujarat because of great industrial climate Modi has created.Fact: Plain lie. Tata was given sops estimated to be worth Rs 30,000 crores over a period of time for investing only a fraction (Rs 2,000 crore) in Sanand, Gujarat. That’s what brought Nano to Gujarat. Modi Team confirms Mega Loan For TataModi Gives Rs 30,000 crore loan to Tata : Free public money in form of Rs 9,570 crore loan as the interest is 0.1% and payment time is 20 years (23% of Gujarat’s budget for that year). Plus, usual sops of tax and duty exemption, infrastructure development and 100 acres of land near Ahmedabad for township. Which businessman will refuse such a deal? link –> linkd) Claim: Gujarat is only state in the whole India to provide 24*7 and 365 days electricity to almost all of its 18000 villages. Modi to Mulayam: Modi to Mulayam: Making Gujarat means providing 24/7 electricityFact : Plain Lie . This is a two part claim1. 100% village electrification2. Gujarat’s leadership in electricity availability to its populaceGujarat’s villages were fully electrified by 1996 (or even before), Modi has no role in 100% village electrification. Electrification of villages, Page 23, Annual Report 1996-97, Ministry of PowerMoving on to availability of electricity, refer to a report of Census 2011, Availability of Electricity Census 2011Percentage of households using electricity as the source of lighting in Gujarat is 90.4%, ranked 14th behind big states like (TN 93.4, Andhra 92.2), many smaller states and UTs. Thus, Gujarat is not the leader in providing electricity to its populace. Interestingly, Gujarat rank fell from 12th in 2001 Census to 14th in 2011 Census.E) FALSE CLAIMS :Every Gujarati is in love with ModiFact: Less than 50% Gujaratis are voting for Modi and around 40% are voting for Congress. BJP’s high vote share pre-dates Modi.Vote per cent share Gujarat elections.In last General Elections (2009) in Gujarat, vote share difference between BJP and Congress was only 3.5%. Correspondingly, seats won BJP 15 and Congress 11.In India electoral systems, winners are based on seats won in which Modi/BJP is clear winner, but that does not mean everyone in Gujarat is voting for Modi/BJP. Within Gujarat Congress too have a healthy vote share in range of 40%.Source: Election Commission of IndiaF) False Claims: Gujarat’s agricultural growth is 10-11% , modi proudly says this fact and writes on his official website too Gujarat Agricultural growthFact : Plain lie. In last 6 years, Gujarat’s agriculture had -ve years of growth -1.08% (2007) & -8.71% (2009), and a zero growth year 0.3% (2010). Gujarat has had a very peculiar growth rate for agriculture. Although I have to say that the Sardar Sarovar Project has had a huge impact on agriculture in Gujarat.Page 61, GDP by Industry of Origin, Data for use of Deputy Chairman, Planning Commission.Furthermore, another data table of Ministry of Agriculture, mentions Gujarat’s agriculture from 1996-97 to 2010-2011 (not given whether it is at constant/current price or gross/net DP), to have frequently years of high negative growth and high positive growth, the variance is so high, by selecting a year, the corresponding growth can significantly change over a period.Page 53, GDP by Industry of Origin, Data for use of Deputy Chairman, Planning CommissionBy no stretch of Imagination Gujarat has 10-11% Agriculture growth, if that be would the farmers do suicides in Gujarat? Yet another lie exposed from RTI. What drove 135 Gujarat farmers to suicide?One more interesting read on suicides of Farmers in Gujarat . -> Sudden rise in farmer suicides in Gujarat.g) FALSE CLAIMS:Gujarat is the only state in country to have “high speed wireless broadband” service in its all 18000 villages.Fact: BSNL brought that to Gujarat. Ironically this is one of deals for which former Telecom minister A Raja is under investigation.BSNL, US’ Soma Networks in tie-up for largest WiMAX deal.SNL Launches India’s First High-speed Wireless Broadband Network in GujaratNow,they say its the only state in the country ,is it so ? No. Wimax comes to GoaIncidentally, BSNL-SOMA WiMAX deal is under investigation in corruption saga of former Telecom Minister A Raja. A Raja may be booked in BSNL’s WiMax deal.In other words, its A Raja, who bought WiMax to Gujarat.h) FALSE CLAIMS :Modi made the Asia’s biggest solar plant.Intending: Solar energy revolution was started by Modi.Fact: Solar energy revolution in India was started by UPA govt in 2010. JLNSM was launched on the 11th January, 2010 by the Prime Minister. The mission has set the ambitious target of deploying 20,000 MW of grid connected solar power by 2022. Jawaharlal Nehru National Solar MissionSince launch of the JLNSM, the capacity of solar power projects has grown from 8 MW in January 2010 to over 979 MW in the country. Progress under Jawaharlal Nehru National Solar MissionClearly Rajasthan and Gujarat, being the biggest desert states in India, solar program was likely to have the largest impact there. Due credit to Gujarat though that it beat Rajasthan to first placeH) Also Modi PR people have claimed that “The entire length of SSNL canal network in Gujarat is around 19,000 kilometres and if even 10 per cent of it is used for this type of projects it could generate 2,400 MW of clean energy annually.” People have touted him as if he was the inventor of the idea of putting solar panels over the canals.Fact: Again Plain Lie. First and foremost, the World’s first over the water solar power project is in California, United States. It’s functioning over a pond since April 2011 (picture above and below). Thus, there is nothing original about the idea of solar panel over water nor Gujarat is first to implement that.Forget 2,400 MW, not even 2MW is a reality.In last one & half year, after 1MW launched in April 2012, not a even one MW more is added. At best there are few “announcements” of Gujarat government to fund 10-20 MW. No news of any further construction as of now. Perhaps Gujarat’s bureaucracy knows; the whole thing was just a publicity stunt, why waste more money on this one.The 0.75 km is the total length of the existing canal top solar panels; somehow fan boys are convinced that 1,900 km (2,500 times) is being covered on war footing and try to force this lie with full rhetoric over anyone skeptical.Just to add, the original purpose of Narmada canals, to bring water to parched lands of Gujarat (a high priority task for any Gujarat CM), as of now remains way behind schedule. Only 35% of the total 75,000 km canal work is complete and in Modi’s 10 years as CM, 10,000 km is dug. Modi’s announced deadline of year 2010 for the task expired 3 years back.I ) FALSE MODELS: Gujarat model of governance – Clean and corruption free.Comptroller and Auditor General (CAG) of India has come up with many reports on How Modi government gave undue benefits to corporates resulting in several hundred crores loss to exchequer. CAG reportApart from this, there are many allegations of him providing land at Rs 1 sq/ft to Adani , 17 scams of Gujarat govt can be read here – > 17 Scams that Narendra Modi doesn’t want to talk.j) Gujarat has lost zero man days due to labour unrest (strikes, lockouts etc.), Modi ji in his videos and his speeches many times claimed the same.Plain lie : Check the annual report of Union finance ministry – The economic survey for the year 2010/11 (page no. 235 – 236), It clearly shows the “maximum incidences” of strikes and lockouts were recorded in Gujarat. Wage and allowance, bonus, personnel, indiscipline and violence were the major reasons.k): Somethings that are not spoken about.a. POVERTYThere are some things which Modi doesn’t tell you. In social sector, Gujarat is one of the worst performing states in the country. Source: Planning Commission Report on state Wise Population Below Poverty lineAnd this was not enough that. Now facts coming out that a person who earns Rs 11/ Day is not below Poverty line in Gujarat and so the person who earns below Rs 11/day are 24 lakhs in number. Read Rs 11/day and you are rich in Gujarat.These were my reasons, apart from that there are many reasons why I wouldn’t choose Modi as my PM. There are 24 hour trolling of BJP trolls on Twitter, Facebook. Their abusive nature and calling other parties with a bad name. Let’s know that showing disregard and abusing are two different things. Many of the journalists are being threatened if they say against Modi and they have shown disregard on Twitter and some of them even have been fired because they write against Mr Modi. Must read -> Why Sagarika Ghose can no longer criticize Modi.Apart from that, the silence of BJP on section 377, their links with LTTE funded Viako (please Google for that), Baba Ramdev’s and Subharamaniam Swamy’s dual nature forces me to not vote for Modi. Silence of BJP big wigs in Asaram Bapu case too is worrying . Apart from it, Wikileaks never said Modi as fully uncorrupt and the whole cable tells Modi to be communal and a ruler by few means and corruption in Oil refinery is evident.His all major schemes get failed.1 The Namami Gange schemeThe Modi government set up a separate ministry headed by Uma Bharti to oversee the work to clean and rejuvenate the Ganga. The Union Cabinet approved a budget outlay of Rs 20,000 crore for the mission - to be spent over the next five years under the Namami Gange Mission.This was almost ten times the amount allocated in the previous cleaning programmes - namely, the Ganga Action Plan phase I and II. The government also declared a 100% tax exemption for those contributing to the clean project in 2015-2016.However, a number of promises made under the ambitious project are yet to materialize. Take a look:Calling the 'Clean Ganga' scheme a failure, the National Green Tribunal slammed the Centre and Uttar Pradesh government for their failure to clean River Ganga in Varanasi.A major roadblock that the scheme is yet to overcome is the issue of sewage discharge into the river. Nothing concrete has been done so far to manage the massive waste disposal (2700 million litres a day (MLD) from all the cities located alongside the banks of the river.A major hindrance is the tussle between the Centre and the states. Most of the states through which the Ganga flows are governed by opposition parties. The scheme is now 100% centrally funded, but is still implemented by local authorities.Corporate houses in the country have shown no interest in funding the project under CSR. Data from the corporate affairs ministry has shown that among all projects of the Narendra Modi government, the Swachh Bharat and Namami Gange have received least amount of funding from private companies in the year 2014-15.The government plans to implement river-interlinking. However, environmentalists claim this could obstruct the natural ecology of rivers.India can’t afford another failure of gigantic proportions because 7,300 million litres of sewage is flowing directly or indirectly into the river every day and effluents from 764 polluting industries on the banks of the river are continuing to add to the problem.2 DemonetizationPrime objectives of Demonetization:Abolish the cash component of Black money. This was the major goal (over 99% weightage), estimated to bring in around 3.5–7 L Cr of total cash in circulation. But (as on 13th Dec 2016) with over 12.4L Cr (80%) currency already deposited out of 15.4L Cr (in 500, 1000 Rs notes) it’s clear that this goal has gone for a toss. Govt. (as on 4th Jan 2017) has not declared the numbers after 13 Dec 2016, it clearly points at the dire situation.However there is an advantage to it, much of this cash, was lying idle, has now entered the banking system and can now be put to use for development (if utilized properly).But since it happened suddenly, there is a huge disadvantage. Deposit rates have come down, so you won’t get less interest than you were getting earlier, but lending rates have not come down (As per latest RBI meeting), so housing or other industry activity will not get its advantage.Abolish Fake currency: The total fake currency estimated (by RBI) was around 400 Cr. which was 0.025% of total currency in circulation, making this a minor goal with <1% weightage. Surprisingly apart from mentioning it in initial speeches, Govt. did not released much data about this. And finally RBI confirmed that it has no confirmed data on fake notes.And within 3 months of Narendra Modi’s demonetisation move, fake Rs 2000 notes from Pakistan enter India.Fake currency will always keep getting generated and govt. has to keep reducing it. Advertising that such drastic change will end fake currency is just a stunt.All other objectives like terrorism etc. were dependent on these 2 prime objectives.B. Impact on Economy:In India, 94% transactions use to happen in cash. With 86% of currency notes suddenly out of circulation, most trade activities came to grinding halt for many weeks. Standing in ques for hours also caused sever loss of productivity. This caused hide loss to economy.As per CMIE 25 Nov. 2016 estimates there will be loss of 1.68 L Cr to the economy.Most conservative figures calculate the loss at 1% of GDP or 1.25L Cr. and some even count it at 2% of economy at 2.5L Cr.C. Social Impact:Job Loss: Demonetization has resulted in severe job losses. It’s hard to put exact number, but it runs in Millions. Here are few examples for it:Demonetisation-induced unemployment is pushing some in Bundelkhand to the brink of starvationMGNREGA hires plummet by 23% in NovemberConstruction workers return home as cash crunch heightensDeaths: Dozens of people have died due to one of the following reasons:a. Not getting treatment due to not having currency notes.b. Death while waiting in lines for Cash.c. Suicide due to conditions created by cash crunch.Though it is not an alarmingly high number, but for a family which lost even 1 member it’s devastating. And these death’s could have been averted with proper planning.Industrial output, tax numbers show note ban impactIt’s disheartening to hear that even as dozens of people lost their lives and millions got unemployed, some people still call it “minor inconvenience”?There are some social advantages to this too. Many people entered banking system for the fist time. This will increase bank savings in India, which is much required. But govt. Need to make people aware of this for long term results.Also some people became aware of digital transactions. This will increase the amount of accounted transactions. Which will marginally reduce corrupt practices and increase taxes.D: Digital Economy:Demonetization is not prerequisite for Digitization. It’s a separate activity and could (rather should) have been done earlier. But yes, not getting good result of demonstration compelled the government to look for alternatives and thats why it started talking about Digitization after 2 weeks of Demonetization.Lack of cash does gives a push to people to go digital but for the amount economy lost due to demonetization (1.25–2.5L Cr.), every Indian could have been incentivized by Rs 1000–2000 (very crude form) for going digital. And that would have much higher penetration than Demonetization.On other hand, if Digitization was planned properly, India had huge potential to start manufacturing the components to build digital infrastructure. One example is POS machines for cards are being imported in huge numbers mainly from China, could have been otherwise 'Made in India'. But ill planing gave such opportunities to foreign countries.If govt. is so serious about going cashless, why don't BJP declares that now they will take all donations only in cashless form (by cheque, DD, Online transfer etc.)? Why not practice what you preach?However there is a silver lining to this. Govt. was quick to realize the failure of demonetization, so it changed the focus and started pushing Digital money. This could have been done earlier also, but there was a lack of political will. Looming failure of demonetization, provided enough political will to push digital currency, even though the required infrastructure or awareness is not created by government. Penetration is still very low. But cash scarcity has Pushed some people to opt for digital currency. Which is good.E: Raids:Many raids were conducted during the exercise and it was widely covered on media. It felt like every corrupt person is raided and all black money will be recovered. But if we put the numbers in to prospective the total cash in old currency seized is 452 Cr, which around 0.1% of the estimated goal of over 3.5L Cr. So the catch was not as big as it was being portrayed.For undeclared asset, govt. could have conducted these raids any time. But yes, not getting desired results of demonstration “pushed the government” to conduct these raids, so there is some positive outcome.But again if we look at the flip side, new currency worth 110 Cr is also found in these raids, which points toward new avenues of black money being created. This is counter productive to the whole excise.The old black money is getting converted via various channels to new black money. And in the process it’s getting redistributed as black money to various people involved. On a lighter note this ‘redistribution’ is like “socialism of black money”.F. Corruption:Demonetization can only kill existing black money, that too only to some extent, it does not have any feature to end new black money from getting generated and as the raids suggest, that cycle has already begun. Creation of new black money while trying to convert old black money is a testimony to this.G. Long term Impact:Demonetization is not a structural policy change, hence it’s impacts (both +ve and -ve) are temporary and limited only to this event. Though the 2% loss of economy will have compounding ill effect in long term, but its very hard to calculate. While the advantages are mostly soft in nature, those fade off exponentially after the impetus ends.I don’t know why demonetization supporters are expecting long term changes without any policy change?Conclusion:Even if we over look the fact that only 3–7% of black money was in cash form (which is very less as compared to other forms). Isn't it a failure to achieve the main objective of demonetization?To make things worse it created a GDP loss of over 1.25L Cr. and a slew of other social losses?There are some benefits due to side effects, but those are either quite small as compared to the evident losses or could have been gained more efficiently by other less loss making methods. Overall it's a net loss to economy.3 Clean India CampaignThe specific objectives of Swachh Bharat mission areElimination of open defecationConversion of unsanitary toilets to pour flush toilets (a type of pit latrine, usually connected to two pits)Eradication of manual scavenging100% collection and processing/disposal/reuse/recycling of municipal solid wasteA behavioural change in people regarding healthy sanitationpracticesGeneration of awareness among citizens about sanitation and its linkages with public healthSupporting urban local bodies in designing, executing and operating waste disposal systemsFacilitating private-sector participation in capital expenditure and operation and maintenance costs for sanitary facilities.Source: Swachh Bharat AbhiyanNone of the objectives get fulfilled.4.Failure in creating new jobsThe India Exclusion Report 2016, released by the New Delhi-based Centre for Equity Studies (CES) on Friday, says that fewer jobs were created under the Modi government in 2015 compared to the previous government led by the Congress Party.Citing Labour Ministry Data, the report says that employment creation in 2015 plummeted to 135,000 jobs compared to 930,000 in 2011. "Yet more than half-way through his (Modi's) tenure, there are almost no jobs available. Job creation has fallen to levels even below those that the preceding UPA governments plunged to," the reportsays.The report also says that Dalits, Adivasis, Muslims and women, groups that have been traditionally oppressed, continue to be the most excluded from accessing public goods.Job Creation Under Modi Government Plunges To Levels Even Below The UPA Regime: Report5.Let us take Modi’s PET Smart City, the GIFT city and see it’s progress !! GIFT city is LIVING example of Modi’s LIES !!I guess 3 of 110 buildings have been completed in 9 years…another 107 buildings in 3 years is next to IMPOSSIBLE.2.7 percent work completed in 75% timeline ; do you expect remaining 97.3 percent will be completed in 25% time ?Coming to occupancy rate of 3 buildings, it is 82,12 & 6% ,Avg. occupancy = 33.33 % occupancy in 3 buildings (in spite of Modi forcing many PSUs and private companies to occupy these buildings) !!He is PhD in lying.2007: We will re-negotiate nuclear deal: BJP2014: Ahead of Modi’s US trip, IAEA pact ratifiedIAEA to get more access to India’s nuclear programme.Then: Narendra Modi opposes GSTBJP blocks GST because of Gujarat minister (Amit Shah): PMNow: Modi’s GST U-turn set to make India single market for first timeThen: 1962 Indo-China war secret report 'blames' Nehru; BJP demands the document be made publicNow: In U-turn, Modi govt rules out release of Henderson Brooks reportJaitley Wanted China War Report Declassified, Changes OpinionJaitley Deletes blog post demanding release of Henderson BrooksThen: No talks till Dawood and Saeed are handed over, demands BJPNow: Pakistan PM to attend inauguration of India's Narendra ModiThen: BJP warns against removal of AFSPA - The Times of IndiaNow: After Article 370, BJP does a U-turn on AFSPA in J&KThen: BJP wanted files on Netaji Subhas Chandra Bose made publicNow: U-turn: BJP govt won't make Netaji files publicBJP following Congress path in suppressing facts on NetajiThen: We'll bring back black money in 150 days: BJP presidentWill bring back black money in 100 days: BJP chief Rajnath SinghNow: Never talked about bringing black money in 100 days: GovtBlack money: Back to square one, list same as last June, says SITBJP falling into black money ditch: SIT report will embarrass ModiBlack money case: Most accounts cleared or closedHave BJP's white lies on black money been nailed?Then: UPA failed to deliver on promisesNow: Congress accuses BJP of U-turns on poll promises180 days, 25 U-turns by Modi government: CongressThen: Irony: Rape accused PJ Kurien heads for global meet on womenNow: Sexual assault: No question of minister's resignation, BJP saysThen: BJP to oppose land boundary agreement with BangladeshNow: PM Modi endorses UPA’s land swap deal with BangladeshThen: BJP attacks Robert Vadra over ‘inappropriate’ behaviourNow: Robert Vadra continue to enjoy security check exemptionsThen: BJP looking for clean candidatesBJP wants Chidambaram, Sibal droppedNow: Half of Modi's new ministers face criminal cases, majority crorepatis Government’s FCRA tweak plan to benefit BJP, Congress
- Home >
- Catalog >
- Business >
- Chart Template >
- Attendance Sheet >
- Attendance Record Template >
- attendance sheet in excel for office >
- Application For Government Candidates