How to Edit Your 200 W Online With Efficiency
Follow the step-by-step guide to get your 200 W edited with accuracy and agility:
- Select the Get Form button on this page.
- You will enter into our PDF editor.
- Edit your file with our easy-to-use features, like highlighting, blackout, and other tools in the top toolbar.
- Hit the Download button and download your all-set document for reference in the future.
We Are Proud of Letting You Edit 200 W Like Using Magics
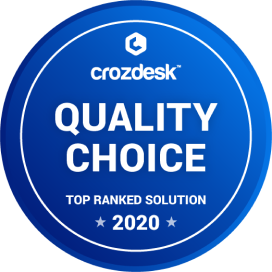
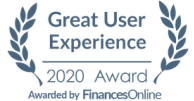
How to Edit Your 200 W Online
When you edit your document, you may need to add text, fill in the date, and do other editing. CocoDoc makes it very easy to edit your form with just a few clicks. Let's see how can you do this.
- Select the Get Form button on this page.
- You will enter into CocoDoc PDF editor web app.
- Once you enter into our editor, click the tool icon in the top toolbar to edit your form, like checking and highlighting.
- To add date, click the Date icon, hold and drag the generated date to the field you need to fill in.
- Change the default date by deleting the default and inserting a desired date in the box.
- Click OK to verify your added date and click the Download button for the different purpose.
How to Edit Text for Your 200 W with Adobe DC on Windows
Adobe DC on Windows is a popular tool to edit your file on a PC. This is especially useful when you finish the job about file edit offline. So, let'get started.
- Find and open the Adobe DC app on Windows.
- Find and click the Edit PDF tool.
- Click the Select a File button and upload a file for editing.
- Click a text box to optimize the text font, size, and other formats.
- Select File > Save or File > Save As to verify your change to 200 W.
How to Edit Your 200 W With Adobe Dc on Mac
- Find the intended file to be edited and Open it with the Adobe DC for Mac.
- Navigate to and click Edit PDF from the right position.
- Edit your form as needed by selecting the tool from the top toolbar.
- Click the Fill & Sign tool and select the Sign icon in the top toolbar to make you own signature.
- Select File > Save save all editing.
How to Edit your 200 W from G Suite with CocoDoc
Like using G Suite for your work to sign a form? You can make changes to you form in Google Drive with CocoDoc, so you can fill out your PDF without Leaving The Platform.
- Add CocoDoc for Google Drive add-on.
- In the Drive, browse through a form to be filed and right click it and select Open With.
- Select the CocoDoc PDF option, and allow your Google account to integrate into CocoDoc in the popup windows.
- Choose the PDF Editor option to begin your filling process.
- Click the tool in the top toolbar to edit your 200 W on the needed position, like signing and adding text.
- Click the Download button in the case you may lost the change.
PDF Editor FAQ
How do you tell the difference between different aircraft just by their names?
I’m going to be straight up: aviation is filled with inconsistencies. You’ll see what I mean.Let’s have a look at the B737–822NGWsc to start with.‘B’ means Boeing. This is a Boeing aircraft.“737” is a small commercial jet, made by Boeing.“-8//” means that this is a Boeing 737–800. This is the 8th version of the 737.“-/22” means that this is a United Airlines aircraft. Any Boeing plane that has “-/22” is United Airlines (747–422, 727–122, 767–322, etc.)“NG” is just the type of 737. The 737NG series includes the -600, -700, -800, and -900. ‘NG’ stands for Next Generation.“W” means that this aircraft has winglets, and…“sc” means that this aircraft has scimitar winglets.So put it together and we get…A United Airlines Next Generation 737–800, with split scimitar winglets.And now for our first issue: the prefix ‘B’. If the name of a commercial aircraft starts with ‘B’, then it’s most likely Boeing. But a military aircraft will usually be referring to a Bomber.In fact, military aircraft have a whole different system for naming planes. I’ll discuss them later.Next example: an A321–211WL.‘A’ is another manufacturer: Airbus. And they run their aircraft slightly different to how Boeing does.“32/” means that this is an aircraft belonging to the A320 family.“321” means that this is an Airbus A321. It’s in the A320 family but is a little longer.“-2//” is an A321–200. It’s the second variant of the A321 and is basically the only one you’ll actually see flying (only about 3% of A321s produced were A321–100s).“-/11” is a bit tricky. Airbus doesn’t base their idents off customers, they base it off the engine type. So an A321–211 will have CFM56–5B3, CFM56–5B3/P, or CFM56–5B3/2P engines. Don't even try to understand engine idents, it’ll just hurt your brain. Anyway,“WL” is an Airbus aircraft with large ‘Sharklet’ winglets. These are relatively new and are applied to both A320CEO and A320NEO aircraft. So now we have:An Airbus A321–200, with CFM56–5B3/P engines (for this certain plane) and advanced ‘sharklets.’You ready for a challenge? Try a B747–467BCF.So yes, we know what ‘B’ is, we know what a 747 is, we know that this is a 747–400, and we know that ‘67’ is the customer code (for Cathay Pacific btw), but what on earth is that last part? Well, not all commercial aircraft carry passengers.The suffix ‘BCF’ stands for Boeing Converted Freighter. This is an old passenger aircraft that has been converted to carry cargo. There are a few more types of freighters out there:‘F’ is a cargo aircraft that was bought, built, sold, and flown as a cargo aircraft it’s whole life. Examples are the 767–300F, the A330–200F, or the MD-11F. Various manufacturers may have various phrases, for example instead of the RJ-100F, it’s an RJ-100QT (Quiet Transport), but usually it’s ‘F’.‘C’ is a bit weird. A 737–400C is a Combi aircraft. This is a plane with passengers at the back, and cargo at the front. However, a 737–700C is a Cargo aircraft. Usually, ‘C’ stands for Cargo, however on some 727s and 737s, it can also mean Combi.‘CF’ is a Converted Freighter. This is the same as a BCF except it’s either converted by a different company, or it’s not a Boeing plane.‘QC’ is a Quick Change aircraft. This is for if an airline can’t really decide whether they like cargo or passengers, so it can be quickly converted into either one. The difference between a ‘CF’ and a ‘QC’ is that a Quick Change aircraft can transition smoothly from either one, however a Converted Freighter has no intentions of ever flying passengers again.‘M’, as far as I know, has only been used on one aircraft: the 747–400M. ‘M’ stands for ‘Mix’, and it is basically a 747 Combi (which I’ve previously discussed).Anyway, this is the B747–467BCF. “-/67”, as I briefly mentioned, is a Cathay Pacific aircraft.One last thing: range. Sometimes, an aircraft is made with more (or even less) range, and so a suffix is added to the aircraft to demonstrate this.‘ER’ is the most common suffix. It stands for Extended Range and can be seen on the 737–900ER, the 747–400ER, or the DC-10–30ER.‘LR’ is also a popular range suffix. It stands for Long Range and oddly enough, a Long Range aircraft usually has more range than an Extended Range aircraft. Examples are the A321LR and the 777–200LR.‘ULR’ and ‘XLR’ stand for Ultra Long Range and Extra Long Range respectively.‘SR’ was used by Boeing in the 1970’s, on the 747–100SR. It stands for Short Range and was used by JAL and ANA.‘D’ stands for ‘Domestic’ and is used on the 747–400D. It’s pretty much the same apart from the name.‘R’ was used by Airbus on the A300–600R and simply stands for Range.So have a try for yourself: Can you guess what a B737–490C is? What about a B777–323ER? Even an A350–941ULR?Here's the codes if you need them:Airbus - WikipediaList of Boeing customer codes - WikipediaNow disregard everything I just said: it’s time for military aircraft.Usually, when a passenger aircraft has a suffix (such as ‘ER’ or ‘BCF’), it means that it has a different role or specification. However, when a military aircraft has a suffix, it means something different.Here's the difference between an A330–200 and an A330–200F:And here’s the difference between a B-52G and a B-52H:As you can see, a suffix doesn’t really do much for a military aircraft. There’s only a few minor differences between the B-52G and the B-52H models, but the A330 was practically redesigned into the A330F.However, a prefix does a lot in the military.This is an F-5:And this is a C-5:As you can tell, there’s a huge difference between these two aircraft: the wheels ;)But seriously, the reason an F-5 and a C-5 look so different is because they are made for different roles. Like I mentioned earlier, the prefix ‘B’ means Bomber. Here’s some more:‘C’ is for Cargo. Examples are the C-17 and the C-130.‘F’ is for Fighter. More specifically, an aircraft that has the ability to shoot down another aircraft. Examples are the F-22 and the F-16.‘A’ is for Afighter. Lol just kidding, it stands for Attack. These are like fighters except they’re more suited to blowing stuff up, on the ground. I think currently the only strictly attack aircraft is the A-10.‘T’ is for Trainer. Usually these look exactly like a fighter except they don’t have any weapons. Examples are the T-X, and the T-38 (which is an unarmed variant of the F-5).‘X’ is for eXperiemental. This is an aircraft that is being tested, and usually the ‘X’ is then dropped when the aircraft enters service. For example, the X-35 was redesignated the F-35 after entering production. Other aircraft, like the XB-70, never got past the testing stage. If it had, it would have been redesignated as the B-70.‘Y’ also means experimental. This is an aircraft that has not yet been tested. An example is the YF-23, which was a rather interesting design by Lockheed that was cancelled.‘V’ is for Vertical, or in full: Vertical Take-Off and Landing. These are aircraft that can take off straight up, without the need for a runway. They are different to helicopters!!! An example is the V-22.‘H’ is for helicopters. Yeah.There's a few more but these are the main prefixes used by the military. They also combine them quite often, such as the F/A-18 (Fighter/Attack), AV-8 (Attack/VTOL), or a KC-30 (Refuelling/Cargo).Another reason the military is weird: the numbers.On a commercial aircraft, the numbers usually go 100, 200, 300, 400, etc. (or if you’re French, 300, 310, 320, 340, 330, 380, 350 lol).On a military aircraft, the numbers usually mean the year it came out: B-52 from 1952, SR-71 from 1971, etc.But once again, the military is weird. Obviously, the F-100 didn’t come out in 2000, and the F/A-18 didn’t come out in 1918.There’s no real order to how these numbers come about, you just have to guess. Usually, the numbers get bigger, such as F-15<F/A-18<F-22<F-35.Okay note: I just realised that the F-111 came before any of those. Damn it, so close to having something normal.Fun fact: did you know that the reason there’s no F-13 because even the military gets a little sceptical at times. Since 13 is an unlucky number, it goes F-11, YF-12, F-14, F-15, etc.So in summary, planes are weird. It can be difficult to ident an aircraft based just on it’s name, but it is possible in some cases.Follow me, Lachie Smith, for more awesome answers about aviation!Thanks, hope this helps!
Is transforming Mars even possible? Could it even hold an atmosphere?
Air pressure on Mars is 610 pascal.Mars surface gravity is 3.27 m/s2.Mars atmosphere by volume is composed 95.32% CO2, 2.7% Nitrogen, 1.6% Argon, 0.174% Oxygen. The gas has a molecular weight of 43.482 grams/mole.0.9532 * 610 = 581.452 Pascal Carbon Dioxide0.0270 * 610 = 16.47 Pascal Nitrogen0.0160 * 610 = 9.76 Pascal Argon0.00174 * 610 = 1.0614 Pascal OxygenSo, by weight Mars atmosphere is95.32%*44/43.482 = 96.456% Carbon Dioxide2.7%*28/43.482 = 1.7387% Nitrogen1.6%*40/43.482 = 1.4719% Argon0.174%*32/43.482 = 0.0012805% Oxygen610 Pascal / 3.27m/s2 = 164.4 kg/m2 - mass of gas per square meter0.96456 * 164.4 = 158.573664 kg/m2 Carbon Dioxide0.017387 * 164.4 = 2.8584228 kg/m2 Nitrogen0.014719 * 164.4 = 2.4198036 kg/m2 Argon0.0012805 * 164.4 = 0.2105142 kg/m2 Oxygen2.4198036/(2.8584228+2.4198036)Early Settlers & Pioneers - step 1.So, early settlers will use molecular seive to produce an Earth normal atmosphere, 78% Nitrogen 21% Oxygen, 0.4% Carbon Dioxide, 0.6% Argon at 101,325 Pascal.Molecular sieve - Wikipedia101325 * 0.78 = 79,033.5 Nitrogen → 79033.5/16.47 = 4798.6 x101325 * 0.21 = 21,278.25 Oxygen → 21278.25/1.0614 = 20047.3 x101325 * 0.006 = 607.95 Argon → 607.95/9.76 = 62.29 x101325 * 0.004 = 405.3 Carbon Dioxide → 405.3/581.452 = 0.697 xSo, you compress Mars atmosphere 20% and throw away 29,000 of 30,000 parts CO2, along with the Argon Nitrogen and Oxygen.You then compress 51.9x and 320.8 of 321.8 parts of Argon is thrown away, and 1 part in 321.8 of Argon is kept.You then compress the remaining Nitrogen, Oxygen and other gases 77x. You throw away 3.1 of 4.1 times the nitrogen, and keep 1/4.1 times the nitrogen. You then compress 4.1x - a total compression of 20,047.3 over the Mars atmosphere.At STP1000 liters * 0.78* 28 grams / 22.4 liters = 0.975 kg/m3 Nitrogen1000 liters * 0.21 * 32 grams/ 22.4 liters = 0.300 kg/m3 Oxygen1000 liters * 0.006 * 40 grams/ 22.4 liters = 0.0107 kg/m3 Argon1000 liters * 0.004 * 44 grams/ 22.4 liters = 0.0008 kg/m3 Carbon DioxideR = 8.314462618T= 293.15 K (20 C)n→ 975 / 28 = 34.82 moles Nitrogenn→ 300/ 42 = 9.375 moles Oxygenn→ 10.7 / 40 = 0.2675 moles Argonn→ 0.8 / 44 = 0.0182 moles Carbon DioxideW=293.15*8.314462618*34.82*ln(4798.6)W = 293.15*8.314462618*34.82*ln(4798.6)= 719362.63 Joules NitrogenW=293.15*8.314462618*9.375*ln(20047.3) = 226353.44 Joules Oxygen (300 grams)- 7.3 watts is needed to extract oxygen at a rate needed for an adult male to breathe.W=293.15*8.314462618*0.2675*ln(62.29) = 2693.93 Joules ArgonW=293.15*8.314462618*0.0182*ln(0.697) = (-16.01) Joules Carbon Dioxide719362.63+226353.44+2693.93–16.01 = 948,393.99 Joules/m3A Spacesuit contains 1 m3 of gas and a 10 m2 cabin that’s 2.2 m tall (22 m3). So it takes a 7.3 watt compressor 36 hours to recharge a spacesuit and 33 days to fill a cabin. If a 146 Watt solar panel powers a 146 Watt gas exchange system described here, could charge up a 10 m2 cabin in 40 hours and 1.8 hours to charge a spacesuit. With a 20 watt solar panel built into the helmet of the spacesuit, that charges a 175.2 Watt-hour (500 gram) lithium ion battery to run a limitless oxygen supply.Cities - Step 2A 7500 ft (2287 meters) diameter sphere filled with Oxygen Nitrogen atmosphere with oxygen extracted from CO2 using Sabatier Process. First there is hydrogen and oxygen extracted from water.Then hydrogen is used to make methane and water from Carbon DioxideThen methane is pyrolysed to form elemental carbon and hydrogenThe process water and the process hydrogen is recycled to produce the following net reactionThis is 8.943 MegaJoules per kilogram of carbon dioxide, which produces 272.7 grams of carbon and 727.3 grams of oxygen.Now this is more complex, and requires more energy per kg of oxygen. That’s 10.3 MJ per day to support the breathing of an adult male. That’s 118.9 Watts per person.Now, 6.107 million metric tons of nitrogen that takes 4.506 PJ.1.879 million metric tons of oxygen that takes 23.081 PJ to MAKE oxygen from the CO2.This also produces 704,528 metric tons of carbon. This is 200,435 cubic meters of diamond graphene or carbon nanotube materials. This is sufficient to produce a 12.2 mm thick diamond shell thats 2287 m diameter.= 0.515 mmSo, 1.36 tonnes of carbon is surplus to the needs of making the shell.A 15,000 ft (4573 meter) diameter disc of high efficiency solar panels that produce a continuous 1.63 GW of power and 8.6 GW peak, takes 28 weeks to produce this shell and the oxygen and nitrogen to fill it.The 7500 ft diameter sphere is set in a 1110 ft depression which is back filled with 1110 ft martian regolith that is converted to earth soil simulant, and a 5280 ft diameter ring mall 200 feet wide and 50 feet tall, contains 8 large air locks and offices, shops and warehouses. There’s a 5280 foot diameter ‘central park. Atop the mall is a 5280 foot tall cylindr that is 5280 ft in diameter that spins at 195 mph.At the base of the cylinder is 78 rings each 3.28 ft wide. A 22 degree lip at the base of the cylinder meets up with rings that are 2.5 mph slower than the base, and the next is 2.5 mph slower than the next ring. Each tilts 0.8 degrees more. So, there is a final ring only 2.5 mph and 0.8 degrees and people and machines move freely between the 2011 acre low gravity park and the 2011 acre high gravity hill. The hill has a one gravity at 22 degree tilt when the centripetal force is combined vectorially with the axial force - Mars’ gravity. The hill is less steep than San Francisco hillsides. There is a ceiling at 80 feet above the outer cylinder wall, and that ceiling has a LED imaging sunlamp that reproduces Earth sky images inside. A 120 foot wide spiral that wraps 176 times around the 5280 foot interior cylinder forming 30 foot ceiling heights covering 8042 acres of spiral. This is where vertical farming is done.Mars Ecumenopolis - Step 3Connctions between cities separated by 200 miles (362 km) Areas of control for which the city is the center of is a hexagon with a 100 mile (161 km) edge length.Spacecraft atmospheres have been tested consisting of 50% oxygen and 22.2% Argon along with 26.2% Nitrogen at 42,556.5 Pascal pressure. This atmosphere is breathable, and supports plant growth.43556.5/101325*1000/22.4*0.5 * 32 grams → 307 grams/m3 Oxygen43556.5/101325*1000/33.4*0.262*28 grams → 94.42 grams/m3 Nitrogen43556.5/101325*1000/33.4*0.222*40 grams→ 114.30 grams/m3 Argon43556.5/101325*1000/33.4*0.016*44 grams → 9.06 grams/m3 CO2So, there is per square meter…0.96456 * 164.4 = 158.573664 kg/m2 Carbon Dioxide158.573664*12/44 = 43.247 kg/m2 → 12.30 mm thickness diamond film158.573664*32/44 = 43.247 kg/m2 → 140.9 meters thickness0.017387 * 164.4 = 2.8584228 kg/m2 Nitrogen → 30.27 meters thickness.0.014719 * 164.4 = 2.4198036 kg/m2 Argon → 21.17 meters thickness0.96456 * 164.4 = 158.573664 kg/m2 Carbon Dioxide → 17,505 metersSo, Argon limits our depth to 21.17 meters, and we have21.17/140.9 = 0.15= 15% of the oxygen is used.21.17/30.27 = 0.70= 70% of the nitrogen is used.21.17/17505 = 0.0012 = 0.12% of the carbon dioxide.70 feet average depth of cylinder arcs connected by tenion lines to a gas barrier at the base. A 15 meter radius with separation of 42.4 meters. 10.6 meters at the low side and 25 meters at the peak, and the base is connected via the tensioning poles resisting the compressed air.So you can have up to a 6 storey building. anywhere on the planet.2146 of the early stage cities separated by 200 iles (322 km) at the center of a 100 mile edged hexagon, which tesselates the planet. This is of the type described above are completed as a self replicating city with a doubling time of 28 weeks. This takes 6 years from the beginning of the self replicating machine.This is 452.8 million people with 211,000 people per city.6.107 million metric tons per city of Nitrogen means 13.1 billion tons of nitrogen for all cities. There is 412.7 billion tons nitrogen available. 3.1% of the total.1.879 million metric tons per city of Oxygen means 4.5 billion tons of oxygen is needed for all cities. 6244.1 billion tons oxygen available from CO2. 0.072% of the total.There are other sources of nitrogen in the Martian soil. This can increase the utilisation of oxygen several times. This increases the number of cities that can be supported 6 times. 12,876 cities of 211,000 people each, 2.716 billion people. Separated by 81.65 miles (131.4 km) with 40.825 mile edge length (65.7 km).A torus around each of the cities that have an outer diameter of 25,000 feet and an inner diameter of 15,000 feet. These consist of 100 foot tall warehouses filled with spaceship air and spacecraft land on the roof, with 100 foot wide disc that lowers down 180 feet inside a 100 foot wide cylinder that acts like an airlock, when the lid closes over the lowered ship. 48,369 landing spots of this type exist for each city, separated by 200 feet with observation domes between, and smaller airlocks serving smaller ships between the major airlocks.The remaining air is left wild, the native Mars haibtat, which most Martians don’t mind keeping.
What is logistic regression?
Hi:I wrote an article sometime back, hope this is useful.Introduction to Logistic RegressionOverview-Logistic regression is generally used where the dependent variable is Binary or Dichotomous. That means the dependent variable can take only two possible values such as “Yes or No”, “Default or No Default”, “Living or Dead”, “Responder or Non Responder”, “Yes or No” etc. Independent factors or variables can be categorical or numerical variables.Please note that even though logistic (logit) regression is frequently used for binary variables (2 classes), it can be used for categorical dependent variables with more than 2 classes. In this case it’s called Multinomial Logistic Regression.Here we will focus on Logistic Regression with binary dependent variables as it is most commonly used.Applications of Logistic Regression-Logistic regression is used for prediction of output which is binary, as stated above. For example, if a credit card company is going to build a model to decide whether to issue a credit card to a customer or not, it will model for whether the customer is going to “Default” or “Not Default” on this credit card. This is called “Default Propensity Modeling” in banking lingo.Similarly an ecommerce company that is sending out costly advertisement / promotional offer mails to customers, will like to know whether a particular customer is likely to respond to the offer or not. In Other words, whether a customer will be “Responder” or “Non Responder”. This is called “Propensity to Respond Modeling”Using insights generated from the logistic regression output, companies may optimize their business strategies to achieve their business goals such as minimize expenses or losses, maximize return on investment (ROI) in marketing campaigns etc.Underlying Algorithm and AssumptionsThe underlying algorithm of Maximum Likelihood Estimation (MLE) determines the regression coefficient for the model that accurately predicts the probability of the binary dependent variable. The algorithm stops when the convergence criterion is met or maximum number of iterations are reached. Since the probability of any event lies between 0 and 1 (or 0% to 100%), when we plot the probability of dependent variable by independent factors, it will demonstrate an ‘S’ shape curve.Let’s take an example- here we are predicting the probability of a given candidate to get admission in a school of his or her choice by the score candidates receives in the admission test. Since the dependent variable is binary/dichotomous- “Admission “or “No Admission”, we can use a logistic regression model to predict the probability of getting the “Admission”. Let’s first plot the data and analyse the shape to confirm that this is following an ‘S’ shape.Since the relationship between the Score and Probability of Selection is not linear but shows an ‘S’ shape, we can’t use a linear model to predict probability of selection by a score. We need to do Logit transformation of the dependent variable to make the correlation between the predictor and dependent variable linear.Logit Transformation is defined as follows-Logit = Log (p/1-p) = log (probability of event happening/ probability of event not happening) = log (Odds)Now we can model using regression to predict the probability of a certain outcome of the dependent variable. The regression equation that the model will try to come out is-Log (p/1-p) = b0+ b1*x1+b2*x2+ eWhere b0 is the Y intercept, e is the error in the model, b1 is the coefficient (slope) for independent factor x1, and b2 is the coefficient (slope) for independent factor x2 and so on…In the above example, the regression equation will look like this-Log (p/1-p) = b0 + b1*Score+ eThe model will generate the coefficients b0 and b1 that gives us the best model in terms of key metrics that we will be discussing later.Tools to Build Logistic RegressionR- Function glm() with the family = “ Logit” is frequently usedSAS- PROC Logistic is a dedicated procedure for running logistic regression with several differKey Metrics and Interpretation-Key metrics that enable comparison among different models and provide indicators of the model performance.Lorenz Curve and Gini Index- Captures the discriminatory power of the model in separating “Good” from “Bad” vs. random selection. Gini Index is the ratio of areas marked below [A/ (A+B)]. This measures how much better the model is performing compared to random selection. The Gini can range between 0% and 100%. Higher the Gini Index, better the model (higher separation between good and bad). Gini value of 0% would indicate a model which is no better than random or in other words no prediction power. On the other hand a Gini value of 100% will indicate a perfect model- it will accurately predict good and Bad 100%.Kolmogorov-Smirnov statistics (KS) - Similar to Gini, KS also captures the discriminatory power of the model in separating “Good” from “Bad”. It is the highest separation between the Cumulative Good Rate and Cumulative Bad Rate. Higher the KS, better is the model (higher separation between good and bad). KS values can range between 0 -100%, KS values greater than 20% are considered acceptable for a model.Receiver Operating Characteristic Curve (ROC) / Area Under Curve (AUC)- Gauges model’s performance in identifying “True Positive” as opposed to “False Positive”. It plots TPR vs FPR. The area under the ROC chart is an indication of this metric. AUC can range from 50% to 100%. Higher the AUC value, better is the prediction power of model.Other Metrics-Lift, Confusion Matrix (Actual vs Predicted), Characteristic Stability Index, % Concordance etc.Here is the complete code and output from a logistic regression model built on the famous German Credit Card data. German Credit Risk | Kaggle##Step 1 - setwd setwd("C:/Users/rpandey/Desktop/Classes") ##Step 2 - Load Data logdata <- read.csv("Default_On_Payment.csv") ##Step 3 - Explore Data- where are the missing values? why some are NAs and others no values? dim(logdata) ## [1] 40121 22 names(logdata) ## [1] "Customer_ID" "Status_Checking_Acc" ## [3] "Duration_in_Months" "Credit_History" ## [5] "Purposre_Credit_Taken" "Credit_Amount" ## [7] "Savings_Acc" "Years_At_Present_Employment" ## [9] "Inst_Rt_Income" "Marital_Status_Gender" ## [11] "Other_Debtors_Guarantors" "Current_Address_Yrs" ## [13] "Property" "Age" ## [15] "Other_Inst_Plans" "Housing" ## [17] "Num_CC" "Job" ## [19] "Dependents" "Telephone" ## [21] "Foreign_Worker" "Default_On_Payment" head(logdata) ## Customer_ID Status_Checking_Acc Duration_in_Months Credit_History ## 1 100015 A14 27 A32 ## 2 100031 A11 12 A34 ## 3 100046 A12 13 A34 ## 4 100103 A14 24 A32 ## 5 100104 A11 24 A32 ## 6 100128 A11 10 A32 ## Purposre_Credit_Taken Credit_Amount Savings_Acc ## 1 A40 2570 A61 ## 2 A43 385 A61 ## 3 A43 882 A61 ## 4 A40 1393 A61 ## 5 A40 3123 A61 ## 6 A43 2315 A61 ## Years_At_Present_Employment Inst_Rt_Income Marital_Status_Gender ## 1 A73 3 A92 ## 2 A74 4 A92 ## 3 A72 4 A93 ## 4 A73 2 A93 ## 5 A72 4 A92 ## 6 A75 3 A93 ## Other_Debtors_Guarantors Current_Address_Yrs Property Age ## 1 A101 3 A121 21 ## 2 A101 3 A121 58 ## 3 A103 4 A121 23 ## 4 A103 2 A121 31 ## 5 A101 1 A122 27 ## 6 A101 4 A121 52 ## Other_Inst_Plans Housing Num_CC Job Dependents Telephone Foreign_Worker ## 1 A143 A151 1 A173 1 A191 A201 ## 2 A143 A152 4 A172 1 A192 A201 ## 3 A143 A152 2 A173 1 A191 A201 ## 4 A143 A152 1 A173 1 A192 A201 ## 5 A143 A152 1 A173 1 A191 A201 ## 6 A143 A152 1 A172 1 A191 A201 ## Default_On_Payment ## 1 1 ## 2 0 ## 3 0 ## 4 0 ## 5 1 ## 6 0 tail(logdata) ## Customer_ID Status_Checking_Acc Duration_in_Months Credit_History ## 40116 986907 A11 24 A32 ## 40117 986936 A12 24 A34 ## 40118 986939 A12 60 A32 ## 40119 986944 A12 36 A30 ## 40120 986974 A11 20 A34 ## 40121 987000 A14 36 A34 ## Purposre_Credit_Taken Credit_Amount Savings_Acc ## 40116 A40 1285 A65 ## 40117 A49 1935 A61 ## 40118 A40 14027 A61 ## 40119 A43 3804 A61 ## 40120 A40 2235 A61 ## 40121 ## Years_At_Present_Employment Inst_Rt_Income Marital_Status_Gender ## 40116 A74 4 A92 ## 40117 A75 4 A91 ## 40118 A74 4 A93 ## 40119 A73 4 A92 ## 40120 A73 4 A94 ## 40121 NA ## Other_Debtors_Guarantors Current_Address_Yrs Property Age ## 40116 A101 4 A124 32 ## 40117 A101 4 A121 31 ## 40118 A101 2 A124 27 ## 40119 A101 1 A123 42 ## 40120 A103 2 A122 33 ## 40121 NA NA ## Other_Inst_Plans Housing Num_CC Job Dependents Telephone ## 40116 A143 A151 1 A173 1 A191 ## 40117 A143 A152 2 A173 1 A192 ## 40118 A143 A152 1 A174 1 A192 ## 40119 A143 A152 1 A173 1 A192 ## 40120 A141 A151 2 A173 1 A191 ## 40121 NA NA ## Foreign_Worker Default_On_Payment ## 40116 A201 1 ## 40117 A201 1 ## 40118 A201 1 ## 40119 A201 1 ## 40120 A202 1 ## 40121 NA str(logdata) ## 'data.frame': 40121 obs. of 22 variables: ## $ Customer_ID : int 100015 100031 100046 100103 100104 100128 100148 100164 100182 100230 ... ## $ Status_Checking_Acc : Factor w/ 5 levels "2","A11","A12",..: 5 2 3 5 2 2 2 5 3 5 ... ## $ Duration_in_Months : Factor w/ 34 levels "10","11","12",..: 14 3 4 12 12 1 2 12 8 3 ... ## $ Credit_History : Factor w/ 6 levels "46","A30","A31",..: 4 6 6 4 4 4 6 6 4 4 ... ## $ Purposre_Credit_Taken : Factor w/ 12 levels "","A143","A40",..: 3 7 7 3 3 7 3 7 7 3 ... ## $ Credit_Amount : Factor w/ 923 levels "","1007","10127",..: 419 602 877 153 511 373 615 353 875 150 ... ## $ Savings_Acc : Factor w/ 7 levels "","2","A61","A62",..: 3 3 3 3 3 3 3 4 3 5 ... ## $ Years_At_Present_Employment: Factor w/ 7 levels "","A172","A71",..: 5 6 4 5 4 7 5 7 5 5 ... ## $ Inst_Rt_Income : int 3 4 4 2 4 3 1 4 4 2 ... ## $ Marital_Status_Gender : Factor w/ 6 levels "","A192","A91",..: 4 4 5 5 4 5 5 5 6 4 ... ## $ Other_Debtors_Guarantors : Factor w/ 5 levels "","A101","A102",..: 2 2 4 4 2 2 2 2 4 2 ... ## $ Current_Address_Yrs : int 3 3 4 2 1 4 2 4 2 2 ... ## $ Property : Factor w/ 5 levels "","A121","A122",..: 2 2 2 2 3 2 2 3 2 3 ... ## $ Age : int 21 58 23 31 27 52 40 52 25 26 ... ## $ Other_Inst_Plans : Factor w/ 4 levels "","A141","A142",..: 4 4 4 4 4 4 4 2 4 4 ... ## $ Housing : Factor w/ 4 levels "","A151","A152",..: 2 3 3 3 3 3 3 3 3 3 ... ## $ Num_CC : int 1 4 2 1 1 1 2 2 1 1 ... ## $ Job : Factor w/ 5 levels "","A171","A172",..: 4 3 4 4 4 3 3 4 3 4 ... ## $ Dependents : int 1 1 1 1 1 1 2 1 1 1 ... ## $ Telephone : Factor w/ 3 levels "","A191","A192": 2 3 2 3 2 2 2 2 2 2 ... ## $ Foreign_Worker : Factor w/ 3 levels "","A201","A202": 2 2 2 2 2 2 2 2 2 2 ... ## $ Default_On_Payment : int 1 0 0 0 1 0 0 0 0 1 ... summary(as.factor(logdata$Default_On_Payment)) ## 0 1 NA's ## 28118 12001 2 summary_logdata = summary(logdata) write.csv(summary_logdata,"summary_logdata.csv",row.names=F) ##Step 4 - Remove any rows with missing values and removed customer_id logdata <- logdata[complete.cases(logdata),] logdata <- logdata[,c(-1)] # Step 5- Bivariate Analyis library(gmodels) CrossTable(logdata$Status_Checking_Acc,logdata$Default_On_Payment) ## ## ## Cell Contents ## |-------------------------| ## | N | ## | Chi-square contribution | ## | N / Row Total | ## | N / Col Total | ## | N / Table Total | ## |-------------------------| ## ## ## Total Observations in Table: 40119 ## ## ## | logdata$Default_On_Payment ## logdata$Status_Checking_Acc | 0 | 1 | Row Total | ## ----------------------------|-----------|-----------|-----------| ## A11 | 5573 | 5417 | 10990 | ## | 588.743 | 1379.407 | | ## | 0.507 | 0.493 | 0.274 | ## | 0.198 | 0.451 | | ## | 0.139 | 0.135 | | ## ----------------------------|-----------|-----------|-----------| ## A12 | 6624 | 4175 | 10799 | ## | 117.900 | 276.237 | | ## | 0.613 | 0.387 | 0.269 | ## | 0.236 | 0.348 | | ## | 0.165 | 0.104 | | ## ----------------------------|-----------|-----------|-----------| ## A13 | 1967 | 564 | 2531 | ## | 21.023 | 49.255 | | ## | 0.777 | 0.223 | 0.063 | ## | 0.070 | 0.047 | | ## | 0.049 | 0.014 | | ## ----------------------------|-----------|-----------|-----------| ## A14 | 13954 | 1845 | 15799 | ## | 749.606 | 1756.306 | | ## | 0.883 | 0.117 | 0.394 | ## | 0.496 | 0.154 | | ## | 0.348 | 0.046 | | ## ----------------------------|-----------|-----------|-----------| ## Column Total | 28118 | 12001 | 40119 | ## | 0.701 | 0.299 | | ## ----------------------------|-----------|-----------|-----------| ## ## CrossTable(logdata$Status_Checking_Acc,logdata$Default_On_Payment,expected=FALSE,prop.r=FALSE, prop.c=FALSE,prop.t=FALSE, prop.chisq=FALSE,chisq=FALSE) ## ## ## Cell Contents ## |-------------------------| ## | N | ## |-------------------------| ## ## ## Total Observations in Table: 40119 ## ## ## | logdata$Default_On_Payment ## logdata$Status_Checking_Acc | 0 | 1 | Row Total | ## ----------------------------|-----------|-----------|-----------| ## A11 | 5573 | 5417 | 10990 | ## ----------------------------|-----------|-----------|-----------| ## A12 | 6624 | 4175 | 10799 | ## ----------------------------|-----------|-----------|-----------| ## A13 | 1967 | 564 | 2531 | ## ----------------------------|-----------|-----------|-----------| ## A14 | 13954 | 1845 | 15799 | ## ----------------------------|-----------|-----------|-----------| ## Column Total | 28118 | 12001 | 40119 | ## ----------------------------|-----------|-----------|-----------| ## ## CrossTable(logdata$Status_Checking_Acc,logdata$Default_On_Payment,expected=FALSE, prop.c=FALSE,prop.t=FALSE, prop.chisq=FALSE,chisq=FALSE) ## ## ## Cell Contents ## |-------------------------| ## | N | ## | N / Row Total | ## |-------------------------| ## ## ## Total Observations in Table: 40119 ## ## ## | logdata$Default_On_Payment ## logdata$Status_Checking_Acc | 0 | 1 | Row Total | ## ----------------------------|-----------|-----------|-----------| ## A11 | 5573 | 5417 | 10990 | ## | 0.507 | 0.493 | 0.274 | ## ----------------------------|-----------|-----------|-----------| ## A12 | 6624 | 4175 | 10799 | ## | 0.613 | 0.387 | 0.269 | ## ----------------------------|-----------|-----------|-----------| ## A13 | 1967 | 564 | 2531 | ## | 0.777 | 0.223 | 0.063 | ## ----------------------------|-----------|-----------|-----------| ## A14 | 13954 | 1845 | 15799 | ## | 0.883 | 0.117 | 0.394 | ## ----------------------------|-----------|-----------|-----------| ## Column Total | 28118 | 12001 | 40119 | ## ----------------------------|-----------|-----------|-----------| ## ## # Step 6- WOE & IV library(Information) library(riv) ## Loading required package: MASS ## Loading required package: rrcov ## Loading required package: robustbase ## Scalable Robust Estimators with High Breakdown Point (version 1.4-3) ## Loading required package: quantreg ## Loading required package: SparseM ## ## Attaching package: 'SparseM' ## The following object is masked from 'package:base': ## ## backsolve library(devtools) library(woe) library(gridExtra) # Step 6a Generate IV of each independent factor- what variables we want to select for building model? stat <- create_infotables(data=logdata, y = "Default_On_Payment") grid.table(stat$Summary, rows=NULL) write.csv(stat$Summary,"IV_summary.csv",row.names=F) # Step 6b- subset the data to select only significant variables newdata <- subset(logdata, select = c(Status_Checking_Acc, Duration_in_Months, Credit_History, Savings_Acc, Purposre_Credit_Taken, Age, Property, Years_At_Present_Employment, Housing, Other_Inst_Plans, Default_On_Payment)) # 6c Generate WOE table for each independed factor # stat <- create_infotables(data=newdata, y = "Default_On_Payment") # # grid.table(new$Tables$Status_Checking_Acc, rows=NULL) # # grid.table(stat$Tables$Duration_in_Months, rows=NULL) # Step 7 - Build Linear Reg model library(car) linreg = lm(Default_On_Payment~., data = newdata) summary(linreg) ## ## Call: ## lm(formula = Default_On_Payment ~ ., data = newdata) ## ## Residuals: ## Min 1Q Median 3Q Max ## -0.86382 -0.28676 -0.09219 0.32246 1.00918 ## ## Coefficients: ## Estimate Std. Error t value Pr(>|t|) ## (Intercept) 0.7697585 0.0218420 35.242 < 2e-16 *** ## Status_Checking_AccA12 -0.1002857 0.0056839 -17.644 < 2e-16 *** ## Status_Checking_AccA13 -0.1982932 0.0089160 -22.240 < 2e-16 *** ## Status_Checking_AccA14 -0.2719186 0.0053806 -50.537 < 2e-16 *** ## Duration_in_Months11 -0.0883551 0.0241493 -3.659 0.000254 *** ## Duration_in_Months12 0.1316079 0.0127738 10.303 < 2e-16 *** ## Duration_in_Months13 -0.1679345 0.0336398 -4.992 6.00e-07 *** ## Duration_in_Months14 -0.0310201 0.0334909 -0.926 0.354335 ## Duration_in_Months15 0.0651237 0.0142697 4.564 5.04e-06 *** ## Duration_in_Months16 0.1752283 0.0463289 3.782 0.000156 *** ## Duration_in_Months18 0.2004975 0.0134166 14.944 < 2e-16 *** ## Duration_in_Months20 -0.0221322 0.0254798 -0.869 0.385061 ## Duration_in_Months21 0.1846304 0.0165940 11.126 < 2e-16 *** ## Duration_in_Months22 0.0164428 0.0457009 0.360 0.719005 ## Duration_in_Months24 0.1777103 0.0128954 13.781 < 2e-16 *** ## Duration_in_Months26 -0.1471282 0.0637199 -2.309 0.020950 * ## Duration_in_Months27 0.2374538 0.0215329 11.028 < 2e-16 *** ## Duration_in_Months28 0.1920215 0.0379099 5.065 4.10e-07 *** ## Duration_in_Months30 0.2005126 0.0157673 12.717 < 2e-16 *** ## Duration_in_Months33 0.3675467 0.0381566 9.633 < 2e-16 *** ## Duration_in_Months36 0.2938116 0.0139703 21.031 < 2e-16 *** ## Duration_in_Months39 0.1831788 0.0306500 5.976 2.30e-09 *** ## Duration_in_Months4 0.0600658 0.0282814 2.124 0.033688 * ## Duration_in_Months40 0.4745183 0.0640407 7.410 1.29e-13 *** ## Duration_in_Months42 0.1325365 0.0226113 5.862 4.62e-09 *** ## Duration_in_Months45 0.5373842 0.0307741 17.462 < 2e-16 *** ## Duration_in_Months47 -0.4267245 0.0633595 -6.735 1.66e-11 *** ## Duration_in_Months48 0.3796413 0.0154894 24.510 < 2e-16 *** ## Duration_in_Months5 0.1641571 0.0641030 2.561 0.010446 * ## Duration_in_Months54 0.2297115 0.0470660 4.881 1.06e-06 *** ## Duration_in_Months6 -0.0284747 0.0138936 -2.049 0.040421 * ## Duration_in_Months60 0.2800291 0.0212741 13.163 < 2e-16 *** ## Duration_in_Months7 -0.0453094 0.0304958 -1.486 0.137351 ## Duration_in_Months72 0.8438571 0.0636178 13.264 < 2e-16 *** ## Duration_in_Months8 -0.0262472 0.0267354 -0.982 0.326235 ## Duration_in_Months9 0.1470090 0.0149335 9.844 < 2e-16 *** ## Credit_HistoryA31 -0.0168741 0.0139103 -1.213 0.225113 ## Credit_HistoryA32 -0.1712827 0.0109288 -15.673 < 2e-16 *** ## Credit_HistoryA33 -0.1722833 0.0123986 -13.895 < 2e-16 *** ## Credit_HistoryA34 -0.2559607 0.0113152 -22.621 < 2e-16 *** ## Savings_AccA62 -0.0331820 0.0069638 -4.765 1.90e-06 *** ## Savings_AccA63 -0.0727308 0.0085281 -8.528 < 2e-16 *** ## Savings_AccA64 -0.1124998 0.0096075 -11.710 < 2e-16 *** ## Savings_AccA65 -0.1105122 0.0055340 -19.970 < 2e-16 *** ## Purposre_Credit_TakenA41 -0.2385023 0.0078033 -30.564 < 2e-16 *** ## Purposre_Credit_TakenA410 -0.1952226 0.0191432 -10.198 < 2e-16 *** ## Purposre_Credit_TakenA42 -0.1275840 0.0063874 -19.974 < 2e-16 *** ## Purposre_Credit_TakenA43 -0.1372409 0.0057771 -23.756 < 2e-16 *** ## Purposre_Credit_TakenA44 -0.0593060 0.0185287 -3.201 0.001372 ** ## Purposre_Credit_TakenA45 -0.0251342 0.0140787 -1.785 0.074226 . ## Purposre_Credit_TakenA46 0.0183318 0.0100066 1.832 0.066963 . ## Purposre_Credit_TakenA48 -0.2157282 0.0213985 -10.081 < 2e-16 *** ## Purposre_Credit_TakenA49 -0.1231291 0.0080966 -15.208 < 2e-16 *** ## Age -0.0012711 0.0002051 -6.198 5.76e-10 *** ## PropertyA122 0.0611509 0.0058654 10.426 < 2e-16 *** ## PropertyA123 0.0528360 0.0054169 9.754 < 2e-16 *** ## PropertyA124 0.1489449 0.0097867 15.219 < 2e-16 *** ## Years_At_Present_EmploymentA72 0.0252472 0.0097476 2.590 0.009599 ** ## Years_At_Present_EmploymentA73 -0.0414445 0.0090543 -4.577 4.72e-06 *** ## Years_At_Present_EmploymentA74 -0.1210120 0.0096866 -12.493 < 2e-16 *** ## Years_At_Present_EmploymentA75 -0.0506770 0.0091716 -5.525 3.31e-08 *** ## HousingA152 -0.0798273 0.0055000 -14.514 < 2e-16 *** ## HousingA153 -0.1272980 0.0112635 -11.302 < 2e-16 *** ## Other_Inst_PlansA142 0.0045000 0.0107968 0.417 0.676837 ## Other_Inst_PlansA143 -0.0726811 0.0061262 -11.864 < 2e-16 *** ## --- ## Signif. codes: 0 '***' 0.001 '**' 0.01 '*' 0.05 '.' 0.1 ' ' 1 ## ## Residual standard error: 0.3908 on 40054 degrees of freedom ## Multiple R-squared: 0.2726, Adjusted R-squared: 0.2715 ## F-statistic: 234.6 on 64 and 40054 DF, p-value: < 2.2e-16 plot(predict(linreg)) #predicted values lie outside 0 to 1 range - hence improper model fit plot(linreg) # Step 8- Transformmation/Dummy coding of variables for getting the best model write.csv(stat$Tables$Duration_in_Months,"Duration_summary.csv",row.names=F) newdata$Duration_Category <- ifelse(newdata$Duration_in_Months %in% c("4","5","6", "7", "8", "9", "10", "11","12", "13", "14", "15", "16", "17", "18"),"lessthan20", ifelse(newdata$Duration_in_Months %in% c("20","21","22", "24", "26", "27", "28", "30","33", "36", "39"), "20to40", "morethan40")) table(newdata$Duration_in_Months, newdata$Duration_Category) ## ## 20to40 lessthan20 morethan40 ## 10 0 1123 0 ## 11 0 361 0 ## 12 0 7179 0 ## 13 0 161 0 ## 14 0 161 0 ## 15 0 2567 0 ## 16 0 80 0 ## 18 0 4536 0 ## 20 322 0 0 ## 21 1205 0 0 ## 22 80 0 0 ## 24 7386 0 0 ## 26 40 0 0 ## 27 521 0 0 ## 28 120 0 0 ## 30 1604 0 0 ## 33 121 0 0 ## 36 3326 0 0 ## 39 200 0 0 ## 4 0 240 0 ## 40 0 0 41 ## 42 0 0 440 ## 45 0 0 200 ## 47 0 0 40 ## 48 0 0 1924 ## 5 0 40 0 ## 54 0 0 80 ## 6 0 3009 0 ## 60 0 0 524 ## 7 0 200 0 ## 72 0 0 40 ## 8 0 280 0 ## 9 0 1968 0 ## A122 0 0 0 stat <- create_infotables(data=newdata, y = "Default_On_Payment") newdata$Duration_Dummy_20 <- ifelse(newdata$Duration_Category == "lessthan20", 1,0) newdata$Duration_Dummy_40 <- ifelse(newdata$Duration_Category == "20to40", 1,0) newdata$Status_Checking_Acc_A11 <- ifelse(newdata$Status_Checking_Acc == "A11", 1,0) newdata$Status_Checking_Acc_A12 <- ifelse(newdata$Status_Checking_Acc == "A12", 1,0) newdata$Status_Checking_Acc_A13 <- ifelse(newdata$Status_Checking_Acc == "A13", 1,0) newdata$Default_On_Payment1 <- as.factor(ifelse(newdata$Default_On_Payment == 1,"1","0")) #Step 9 - Build logistic regression library(ROCR) ## Loading required package: gplots ## ## Attaching package: 'gplots' ## The following object is masked from 'package:stats': ## ## lowess logreg <- glm(Default_On_Payment ~ Duration_Dummy_20+Duration_Dummy_40+Status_Checking_Acc_A11+Status_Checking_Acc_A12+Status_Checking_Acc_A13+Age, family = binomial("logit"),data = newdata) summary(logreg) ## ## Call: ## glm(formula = Default_On_Payment ~ Duration_Dummy_20 + Duration_Dummy_40 + ## Status_Checking_Acc_A11 + Status_Checking_Acc_A12 + Status_Checking_Acc_A13 + ## Age, family = binomial("logit"), data = newdata) ## ## Deviance Residuals: ## Min 1Q Median 3Q Max ## -1.6125 -0.8832 -0.4819 1.0449 2.3970 ## ## Coefficients: ## Estimate Std. Error z value Pr(>|z|) ## (Intercept) -0.595650 0.059126 -10.07 <2e-16 *** ## Duration_Dummy_20 -1.154614 0.041206 -28.02 <2e-16 *** ## Duration_Dummy_40 -0.677572 0.042070 -16.11 <2e-16 *** ## Status_Checking_Acc_A11 1.984595 0.031684 62.64 <2e-16 *** ## Status_Checking_Acc_A12 1.495144 0.032235 46.38 <2e-16 *** ## Status_Checking_Acc_A13 0.865074 0.054460 15.88 <2e-16 *** ## Age -0.015651 0.001076 -14.55 <2e-16 *** ## --- ## Signif. codes: 0 '***' 0.001 '**' 0.01 '*' 0.05 '.' 0.1 ' ' 1 ## ## (Dispersion parameter for binomial family taken to be 1) ## ## Null deviance: 48956 on 40118 degrees of freedom ## Residual deviance: 42581 on 40112 degrees of freedom ## AIC: 42595 ## ## Number of Fisher Scoring iterations: 4 plot(predict(logreg,type="response")) #note plot option has type to get inverse of log_odds newdata$predicted = predict(logreg,type="response") write.csv(newdata,"output_logreg.csv", row.names = F) capture.output(summary(logreg), file = "summary_logreg.csv") summary_residuals_model.csv<-residuals(logreg, type="deviance") write.csv(summary_residuals_model.csv, "summary_residuals_model.csv") #Step 10 - Model Diagnostics # ROCR newdata$predicted = predict(logreg,type="response") pred<-prediction(newdata$predicted,newdata$Default_On_Payment) perf <- performance(pred,"tpr","fpr") plot(perf) abline(a=0, b=1, col="Red") # AUC auc.perf = performance(pred, measure = "auc") [email protected] ## [[1]] ## [1] 0.7429535 # Create Decile by scorebands library(dplyr) ## ## Attaching package: 'dplyr' ## The following object is masked from 'package:car': ## ## recode ## The following object is masked from 'package:gridExtra': ## ## combine ## The following object is masked from 'package:MASS': ## ## select ## The following objects are masked from 'package:stats': ## ## filter, lag ## The following objects are masked from 'package:base': ## ## intersect, setdiff, setequal, union newdata$decile <- ntile(-newdata$predicted,10) write.csv(newdata, "newdata.csv") # KS max(attr(perf,'y.values')[[1]]-attr(perf,'x.values')[[1]]) ## [1] 0.3810604 # Lift lift.obj <- performance(pred, "lift", x.measure = "rpp") plot(lift.obj, main="Cross-Sell - Lift Chart", xlab="% Populations", ylab="Lift", col="blue") abline(1,0,col="grey") # Lorenz and Gini library(ineq) # Gini Index ineq(newdata$predicted,type="Gini") ## [1] 0.3388472 ## Lorenz Curve plot(Lc(newdata$predicted),col="darkred",lwd=2) ##Get Concordance/Pairs Stats # High-Low Ratio of bad rate by decile in Excel. # Confidence interval for ROC, KS, Gini # mydata[ which(mydata$gender=='F' & mydata$age > 65), ] Concordance = function(y,yhat) { outcome_and_fitted_col<-data.frame(y, yhat) colnames(outcome_and_fitted_col)<-c("Responder","fitted.values") # get a subset of outcomes where the event actually happened ones = outcome_and_fitted_col[outcome_and_fitted_col[,1] == 1,] # get a subset of outcomes where the event didn't actually happen zeros = outcome_and_fitted_col[outcome_and_fitted_col[,1] == 0,] # Equate the length of the event and non-event tables if (length(ones[,1])>length(zeros[,1])) {ones = ones[1:length(zeros[,1]),]} else {zeros = zeros[1:length(ones[,1]),]} # Following will be c(ones_outcome, ones_fitted, zeros_outcome, zeros_fitted) ones_and_zeros = data.frame(ones, zeros) # initiate columns to store concordant, discordant, and tie pair evaluations conc = rep(NA, length(ones_and_zeros[,1])) disc = rep(NA, length(ones_and_zeros[,1])) ties = rep(NA, length(ones_and_zeros[,1])) for (i in 1:length(ones_and_zeros[,1])) { # This tests for concordance if (ones_and_zeros[i,2] > ones_and_zeros[i,4]) {conc[i] = 1 disc[i] = 0 ties[i] = 0 } # This tests for a tie else if (ones_and_zeros[i,2] == ones_and_zeros[i,4]) { conc[i] = 0 disc[i] = 0 ties[i] = 1 } # This should catch discordant pairs. else if (ones_and_zeros[i,2] < ones_and_zeros[i,4]) { conc[i] = 0 disc[i] = 1 ties[i] = 0 } } # Here we save the various rates conc_rate = mean(conc, na.rm=TRUE) disc_rate = mean(disc, na.rm=TRUE) tie_rate = mean(ties, na.rm=TRUE) return(list(concordance=conc_rate, num_concordant=sum(conc), discordance=disc_rate, num_discordant=sum(disc), tie_rate=tie_rate,num_tied=sum(ties))) } Concordance_test<-Concordance(newdata$Default_On_Payment,newdata$predicted) Concordance_test ## $concordance ## [1] 0.7421882 ## ## $num_concordant ## [1] 8907 ## ## $discordance ## [1] 0.2553121 ## ## $num_discordant ## [1] 3064 ## ## $tie_rate ## [1] 0.002499792 ## ## $num_tied ## [1] 30 Hope this helps.Cheers!Thanks for your upvotes in advance. They Keep me going! Thank You!Disclaimer: The views expressed here are solely those of the writer in his private capacity.
- Home >
- Catalog >
- Finance >
- Deposit Form >
- Deposit Slip >
- Deposit Template >
- deposit receipt pdf >
- 200 W