How to Edit The Fire Science Professional Track quickly and easily Online
Start on editing, signing and sharing your Fire Science Professional Track online with the help of these easy steps:
- Push the Get Form or Get Form Now button on the current page to make access to the PDF editor.
- Wait for a moment before the Fire Science Professional Track is loaded
- Use the tools in the top toolbar to edit the file, and the added content will be saved automatically
- Download your completed file.
The best-rated Tool to Edit and Sign the Fire Science Professional Track
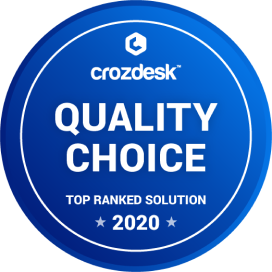
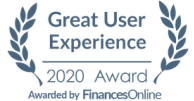
A quick guide on editing Fire Science Professional Track Online
It has become really simple nowadays to edit your PDF files online, and CocoDoc is the best free PDF editor for you to make some changes to your file and save it. Follow our simple tutorial to start!
- Click the Get Form or Get Form Now button on the current page to start modifying your PDF
- Add, change or delete your text using the editing tools on the toolbar on the top.
- Affter altering your content, put the date on and draw a signature to finish it.
- Go over it agian your form before you click to download it
How to add a signature on your Fire Science Professional Track
Though most people are adapted to signing paper documents by handwriting, electronic signatures are becoming more regular, follow these steps to sign documents online for free!
- Click the Get Form or Get Form Now button to begin editing on Fire Science Professional Track in CocoDoc PDF editor.
- Click on the Sign tool in the tool box on the top
- A window will pop up, click Add new signature button and you'll have three options—Type, Draw, and Upload. Once you're done, click the Save button.
- Drag, resize and settle the signature inside your PDF file
How to add a textbox on your Fire Science Professional Track
If you have the need to add a text box on your PDF and customize your own content, do the following steps to carry it throuth.
- Open the PDF file in CocoDoc PDF editor.
- Click Text Box on the top toolbar and move your mouse to position it wherever you want to put it.
- Write in the text you need to insert. After you’ve filled in the text, you can use the text editing tools to resize, color or bold the text.
- When you're done, click OK to save it. If you’re not happy with the text, click on the trash can icon to delete it and take up again.
A quick guide to Edit Your Fire Science Professional Track on G Suite
If you are looking about for a solution for PDF editing on G suite, CocoDoc PDF editor is a recommended tool that can be used directly from Google Drive to create or edit files.
- Find CocoDoc PDF editor and set up the add-on for google drive.
- Right-click on a PDF document in your Google Drive and choose Open With.
- Select CocoDoc PDF on the popup list to open your file with and give CocoDoc access to your google account.
- Modify PDF documents, adding text, images, editing existing text, annotate in highlight, fullly polish the texts in CocoDoc PDF editor before saving and downloading it.
PDF Editor FAQ
How can I become a data scientist?
Here are some amazing and completely free resources online that you can use to teach yourself data science.Besides this page, I would highly recommend following the Quora Data Science topic if you haven't already to get updates on new questions and answers!Step 1. Fulfill your prerequisitesBefore you begin, you need Multivariable Calculus, Linear Algebra, and Python. If your math background is up to multivariable calculus and linear algebra, you'll have enough background to understand almost all of the probability / statistics / machine learning for the job.Multivariate Calculus: What are the best resources for mastering multivariable calculus?Numerical Linear Algebra / Computational Linear Algebra / Matrix Algebra: Linear Algebra, Introduction to Linear Models and Matrix Algebra. Avoid linear algebra classes that are too theoretical, you need a linear algebra class that works with real matrices.Multivariate calculus is useful for some parts of machine learning and a lot of probability. Linear / Matrix algebra is absolutely necessary for a lot of concepts in machine learning.You also need some programming background to begin, preferably in Python. Most other things on this guide can be learned on the job (like random forests, pandas, A/B testing), but you can't get away without knowing how to program!Python is the most important language for a data scientist to learn. To learn to code, more about Python, and why Python is so important, check outHow do I learn to code?How do I learn Python?Why is Python a language of choice for data scientists?Is Python the most important programming language to learn for aspiring data scientists and data miners?R is the second most important language for a data scientist to learn. I’m saying this as someone with a statistics background and who went through undergrad mainly only using R. While R is powerful for dedicated statistical tasks, Python is more versatile as it will connect you more to production-level work.If you're currently in school, take statistics and computer science classes. Check out What classes should I take if I want to become a data scientist?Step 2. Plug Yourself Into the CommunityCheck out Meetup to find some that interest you! Attend an interesting talk, learn about data science live, and meet data scientists and other aspirational data scientists. Start reading data science blogs and following influential data scientists:What are the best, insightful blogs about data, including how businesses are using data?What is your source of machine learning and data science news? Why?What are some best data science accounts to follow on Twitter, Facebook, G+, and LinkedIn?What are the best Twitter accounts about data?Step 3. Setup and Learn to use your toolsPythonInstall Python, iPython, and related libraries (guide)How do I learn Python?RInstall R and RStudio (It's good to know both Python and R)Learn R with swirlSublime TextInstall Sublime TextWhat's the best way to learn to use Sublime Text?SQLHow do I learn SQL? What are some good online resources, like websites, blogs, or videos? (You can practice it using the sqlite package in Python)Step 4. Learn Probability and StatisticsBe sure to go through a course that involves heavy application in R or Python. Knowing probability and statistics will only really be helpful if you can implement what you learn.Python Application: Think Stats (free pdf) (Python focus)R Applications: An Introduction to Statistical Learning (free pdf)(MOOC) (R focus)Print out a copy of Probability CheatsheetStep 5. Complete Harvard's Data Science CourseAs of Fall 2015, the course is currently in its third year and strives to be as applicable and helpful as possible for students who are interested in becoming data scientists. An example of how is this happening is the introduction of Spark and SQL starting this year.I'd recommend doing the labs and lectures from 2015 and the homeworks from 2013 (2015 homeworks are not available to the public, and the 2014 homeworks are written under a different instructor than the original instructors).This course is developed in part by a fellow Quora user, Professor Joe Blitzstein. Here are all of the materials!Intro to the classWhat is it like to design a data science class? In particular, what was it like to design Harvard's new data science class, taught by professors Joe Blitzstein and Hanspeter Pfister?What is it like to take CS 109/Statistics 121 (Data Science) at Harvard?Course MaterialsClass main page: CS109 Data ScienceLectures, Slides, and Labs: Class MaterialAssignmentsIntro to Python, Numpy, Matplotlib (Homework 0) (Solutions)Poll Aggregation, Web Scraping, Plotting, Model Evaluation, and Forecasting (Homework 1) (Solutions)Data Prediction, Manipulation, and Evaluation (Homework 2) (Solutions)Predictive Modeling, Model Calibration, Sentiment Analysis (Homework 3) (Solutions)Recommendation Engines, Using Mapreduce (Homework 4) (Solutions)Network Visualization and Analysis (Homework 5) (Solutions)Labs(these are the 2013 labs. For the 2015 labs, check out Class Material)Lab 2: Web ScrapingLab 3: EDA, Pandas, MatplotlibLab 4: Scikit-Learn, Regression, PCALab 5: Bias, Variance, Cross-ValidationLab 6: Bayes, Linear Regression, and Metropolis SamplingLab 7: Gibbs SamplingLab 8: MapReduceLab 9: NetworksLab 10: Support Vector MachinesStep 6. Do all of Kaggle's Getting Started and Playground CompetitionsI would NOT recommend doing any of the prize-money competitions. They usually have datasets that are too large, complicated, or annoying, and are not good for learning. The competitions are available at Competitions | KaggleStart by learning scikit-learn, playing around, reading through tutorials and forums on the competitions that you’re doing. Next, play around some more and check out the tutorials for Titanic: Machine Learning from Disaster for a binary classification task (with categorical variables, missing values, etc.)Afterwards, try some multi-class classification with Forest Cover Type Prediction. Now, try a regression task House Prices: Advanced Regression Techniques. Try out some natural language processing with Quora Question Pairs | Kaggle. Finally, try out any of the other knowledge-based competitions that interest you!Step 7. Learn Some Data Science ElectivesData science is an incredibly large and interdisciplinary field, and different jobs will require different skillsets. Here are some of the more common ones:Product Metrics will teach you about what companies track, what metrics they find important, and how companies measure their success: The 27 Metrics in Pinterest’s Internal Growth DashboardMachine Learning How do I learn machine learning? This is an extremely rich area with massive amounts of potential, and likely the “sexiest” area of data science today. Andrew Ng's Machine Learning course on Coursera is one of the most popular MOOCs, and a great way to start! Andrew Ng's Machine Learning MOOCA/B Testing is incredibly important to help inform product decisions for consumer applications. Learn more about A/B testing here: How do I learn about A/B testing?Visualization - I would recommend picking up ggplot2 in R to make simple yet beautiful graphics and just browsing DataIsBeautiful • /r/dataisbeautiful and FlowingData for ideas and inspiration.User Behavior - This set of blogs posts looks useful and interesting - This Explains Everything " User BehaviorFeature Engineering - Check out What are some best practices in Feature Engineering? and this great example: http://nbviewer.ipython.org/github/aguschin/kaggle/blob/master/forestCoverType_featuresEngineering.ipynbBig Data Technologies - These are tools and frameworks developed specifically to deal with massive amounts of data. How do I learn big data technologies?Optimization will help you with understanding statistics and machine learning: Convex Optimization - Boyd and VandenbergheNatural Language Processing - This is the practice of turning text data into numerical data whilst still preserving the "meaning". Learning this will let you analyze new, exciting forms of data. How do I learn Natural Language Processing (NLP)?Time Series Analysis - How do I learn about time series analysis?Step 8. Do a Capstone Product / Side ProjectUse your new data science and software engineering skills to build something that will make other people say wow! This can be a website, new way of looking at a dataset, cool visualization, or anything!What are some good toy problems (can be done over a weekend by a single coder) in data science? I'm studying machine learning and statistics, and looking for something socially relevant using publicly available datasets/APIs.How can I start building a recommendation engine? Where can I find an interesting data set? What tools/technologies/algorithms are best to build the engine with? How do I check the effectiveness of recommendations?What are some ideas for a quick weekend Python project? I am looking to gain some experience.What is a good measure of the influence of a Twitter user?Where can I find large datasets open to the public?What are some good algorithms for a prioritized inbox?What are some good data science projects?Create public github repositories, make a blog, and post your work, side projects, Kaggle solutions, insights, and thoughts! This helps you gain visibility, build a portfolio for your resume, and connect with other people working on the same tasks.Step 9. Get a Data Science Internship or JobHow do I prepare for a data scientist interview?How should I prepare for statistics questions for a data science interviewWhat kind of A/B testing questions should I expect in a data scientist interview and how should I prepare for such questions?What companies have data science internships for undergraduates?What are some tips to choose whether I want to apply for a Data Science or Software Engineering internship?When is the best time to apply for data science summer internships?Check out The Official Quora Data Science FAQ for more discussion on internships, jobs, and data science interview processes! The data science FAQ also links to more specific versions of this question, like How do I become a data scientist without a PhD? or the counterpart, How do I become a data scientist as a PhD student?Step 10. Share your Wisdom Back with the Data Science CommunityIf you’ve made it this far, congratulations on becoming a data scientist! I’d encourage you to share your knowledge and what you’ve learned back with the data science community. Data Science as a nascent field depends on knowledge-sharing!Think like a Data ScientistIn addition to the concrete steps I listed above to develop the skill set of a data scientist, I include seven challenges below so you can learn to think like a data scientist and develop the right attitude to become one.(1) Satiate your curiosity through dataAs a data scientist you write your own questions and answers. Data scientists are naturally curious about the data that they're looking at, and are creative with ways to approach and solve whatever problem needs to be solved.Much of data science is not the analysis itself, but discovering an interesting question and figuring out how to answer it.Here are two great examples:Hilary: the most poisoned baby name in US historyA Look at Fire Response DataChallenge: Think of a problem or topic you're interested in and answer it with data!(2) Read news with a skeptical eyeMuch of the contribution of a data scientist (and why it's really hard to replace a data scientist with a machine), is that a data scientist will tell you what's important and what's spurious. This persistent skepticism is healthy in all sciences, and is especially necessarily in a fast-paced environment where it's too easy to let a spurious result be misinterpreted.You can adopt this mindset yourself by reading news with a critical eye. Many news articles have inherently flawed main premises. Try these two articles. Sample answers are available in the comments.Easier: You Love Your iPhone. Literally.Harder: Who predicted Russia’s military intervention?Challenge: Do this every day when you encounter a news article. Comment on the article and point out the flaws.(3) See data as a tool to improve consumer productsVisit a consumer internet product (probably that you know doesn't do extensive A/B testing already), and then think about their main funnel. Do they have a checkout funnel? Do they have a signup funnel? Do they have a virility mechanism? Do they have an engagement funnel?Go through the funnel multiple times and hypothesize about different ways it could do better to increase a core metric (conversion rate, shares, signups, etc.). Design an experiment to verify if your suggested change can actually change the core metric.Challenge: Share it with the feedback email for the consumer internet site!(4) Think like a BayesianTo think like a Bayesian, avoid the Base rate fallacy. This means to form new beliefs you must incorporate both newly observed information AND prior information formed through intuition and experience.Checking your dashboard, user engagement numbers are significantly down today. Which of the following is most likely?1. Users are suddenly less engaged2. Feature of site broke3. Logging feature brokeEven though explanation #1 completely explains the drop, #2 and #3 should be more likely because they have a much higher prior probability.You're in senior management at Tesla, and five of Tesla's Model S's have caught fire in the last five months. Which is more likely?1. Manufacturing quality has decreased and Teslas should now be deemed unsafe.2. Safety has not changed and fires in Tesla Model S's are still much rarer than their counterparts in gasoline cars.While #1 is an easy explanation (and great for media coverage), your prior should be strong on #2 because of your regular quality testing. However, you should still be seeking information that can update your beliefs on #1 versus #2 (and still find ways to improve safety). Question for thought: what information should you seek?Challenge: Identify the last time you committed the Base Rate Fallacy. Avoid committing the fallacy from now on.(5) Know the limitations of your tools“Knowledge is knowing that a tomato is a fruit, wisdom is not putting it in a fruit salad.” - Miles KingtonKnowledge is knowing how to perform a ordinary linear regression, wisdom is realizing how rare it applies cleanly in practice.Knowledge is knowing five different variations of K-means clustering, wisdom is realizing how rarely actual data can be cleanly clustered, and how poorly K-means clustering can work with too many features.Knowledge is knowing a vast range of sophisticated techniques, but wisdom is being able to choose the one that will provide the most amount of impact for the company in a reasonable amount of time.You may develop a vast range of tools while you go through your Coursera or EdX courses, but your toolbox is not useful until you know which tools to use.Challenge: Apply several tools to a real dataset and discover the tradeoffs and limitations of each tools. Which tools worked best, and can you figure out why?(6) Teach a complicated conceptHow does Richard Feynman distinguish which concepts he understands and which concepts he doesn't?Feynman was a truly great teacher. He prided himself on being able to devise ways to explain even the most profound ideas to beginning students. Once, I said to him, "Dick, explain to me, so that I can understand it, why spin one-half particles obey Fermi-Dirac statistics." Sizing up his audience perfectly, Feynman said, "I'll prepare a freshman lecture on it." But he came back a few days later to say, "I couldn't do it. I couldn't reduce it to the freshman level. That means we don't really understand it." - David L. Goodstein, Feynman's Lost Lecture: The Motion of Planets Around the SunWhat distinguished Richard Feynman was his ability to distill complex concepts into comprehendible ideas. Similarly, what distinguishes top data scientists is their ability to cogently share their ideas and explain their analyses.Check out https://www.quora.com/Edwin-Chen-1/answers for examples of cogently-explained technical concepts.Challenge: Teach a technical concept to a friend or on a public forum, like Quora or YouTube.(7) Convince others about what's importantPerhaps even more important than a data scientist's ability to explain their analysis is their ability to communicate the value and potential impact of the actionable insights.Certain tasks of data science will be commoditized as data science tools become better and better. New tools will make obsolete certain tasks such as writing dashboards, unnecessary data wrangling, and even specific kinds of predictive modeling.However, the need for a data scientist to extract out and communicate what's important will never be made obsolete. With increasing amounts of data and potential insights, companies will always need data scientists (or people in data science-like roles), to triage all that can be done and prioritize tasks based on impact.The data scientist's role in the company is the serve as the ambassador between the data and the company. The success of a data scientist is measured by how well he/she can tell a story and make an impact. Every other skill is amplified by this ability.Challenge: Tell a story with statistics. Communicate the important findings in a dataset. Make a convincing presentation that your audience cares about.Good luck and best wishes on your journey to becoming a data scientist! For more resources check out Quora’s official Quora Data Science FAQ
In a total nuclear exchange where the entire worlds arsenals are used, how long would the nuclear winter last and would we survive?
Flashback to the 1980’s … Mutually Assured Destruction (MAD)Note: For an updated and more complete answer please go here Allen E Hall's answer to Who would win in a war between Russia and the US?A lot has changed…..In 2016 a nuclear winter isn't possible even in an all out nuclear war. This is because both the quantities and yield of the world's nuclear arsenals has dropped precipitously from the all time high in 1986. The arsenals today are only 20% the size they were in 1986 and the total megatons available is less than 10% of the peak.Surprisingly quietly, the USA and Russia have dismantled over 50,000 nuclear weapons over the past 30 years. The nuclear materials from these bombs and other stockpiles of weapons grade materials, was recycled and used in nuclear power generation over the past 20 years. A fact that few may be aware of, the situation actually crashed the uranium market in the early 2000’s. The glut of available fuel brought the open market trading value down from $20 dollars a pound to near $2 per pound at that time. So a lot has changed from the time when many of us can remember the very real threat of mutually assured destruction.Multi Megaton Weapons Now ObsoleteWhat has changed that the world no longer is building megaton weapons? The need for multi-megaton weapons was the result of low accuracy of warhead deliver on target…. we needed a sledgehammer approach to take out hardened targets and the way that was done was through very high yield bombs >=5 mt typically. The average nuclear weapon size today in 2016 is about 443kt at full yield but a large portion of those bombs can be adjusted in the field to a very small fraction of their potential yield.Today the accuracy of on target delivery has massively improved ..we hit what we aim for. This means we need less hammer to do the same job. In the 1980’s the development of earth penetrating rounds was another game changer. Not only were we on target but now we could penetrate hundreds of feet of earth and concrete before detonating the warhead. This allowed a 100 kt weapon to do the damage of a >1 mt surface detonation. This is the primary method now for targeting hardened targets and is the final driver for smaller yield bombs.The net effect of the use of EPW’s (Earth Penetrating Weapons) is a reduction in the number of casualties as compared with the number of casualties from a surface burst. This is primarily due to a 96% reduction in the weapon yield needed using an EPW. The greater coupling of the released energy to the ground shock for a buried detonation is the same as a surface burst with 25 times the explosive energy. For rural targets, the use of a nuclear earth-penetrator weapon is estimated to reduce casualties by a factor of 10 to 100 relative to a nuclear surface burst of equivalent probability of damage.. [1]The average warhead size in the USA arsenal is 330 kt. The Russian average is higher, but not enough to change this outcome. To cause a nuclear winter the debris clouds and smoke have to be elevated above the troposphere into the high stratosphere. Any debris or smoke that is released into the troposphere (below 70,000 feet) quickly rains out in the weather within a few days to a week or so max. Nuclear weapons yields do not affect the environment on a linear scale , that is to say that a 1 megaton bomb, even though it is 10 x more energy than 100 kt bomb, doesn't mean it produces 10 x more destruction. Thermal radiation decays as the inverse square while blast decays as the inverse cube of distance from the detonation point. Much of that extra heat and energy goes straight up and drops off quickly as distance is increased from the point of detonation. With smaller yields the energy isn't enough to breach the stratosphere, and for bombs that size the earth has its own protection mechanism for particles released in the troposphere called the weather, and it is extremely efficient.The only way to get particles to stay aloft longer is to blast them considerably higher than 70,000 feet. **The reason this won’t happen today is that the world has eliminated megaton-size bombs almost completely, and shortly it will be complete as the last ones are dismantled. Russia and the US both have eliminated megaton size weapons from the high-alert strategic forces (ICBM’s & SLBM’s).To get anything above 70,000 feet you need yields substantially above 1 megaton. The bombs deployed today will throw debris up 50,000 - 60,000 feet into the atmosphere and all of that will rain back down to the earth in hours and days later near the point of detonation.(** B-83 variable yield ≈ 20 kt - 1.2 mt slated for retirement in 2025. This is a gravity bomb and is also being considered as a reserve against an asteroid impact. Marshall Space Flight Center have developed designs for an array of asteroid interceptors wielding 1.2-megaton B83 nuclear warheads. 650 units in reserve but not alert status)As we have all heard in the past that there were enough nuclear weapons to kill everyone several times over, let me put that myth to rest. Hypothetical scenario for maximum damage: Starting in an arbitrary corner of the USA (or if you prefer … Canada) take the entire world's inventory of nuclear weapons (10,000 active and stockpiled) and place each one in its own circle covering 100 square miles. Using a world average yield size of 500 kt, this sets up the scenario for maximum destruction. If all the warheads are then elevated to 6000 feet, the height for maximum destruction and fatalities, and then detonated. Each bomb would make a 10 km radius of destruction from its center with 3rd & 2nd degree burns on the outskirts of this radius. The fallout would be minimal with only air bursts, most dangers would be gone within hours or days after the blasts. Using every bomb in existence today as laid out in this hypothetical scenario, the area of assured destruction would only amount to 1/3 of the USA’s total land mass. If it was Canada, many might not even notice. That’s it. On a global scale that isn’t hardly a scratch at 1/42 of the world's total land mass.Firestorms and other bad science that led to the wrong conclusions.A lot of new knowledge on pyrocumulonimbus cloud formation and soot into the lower stratosphere is still being interpreted. Until the early 2000’s it was thought the boundary layer between the troposphere and stratosphere presented a greater barrier for smoke, however, smoke columns rising into the lower stratosphere have been observed. This indicates that there is a long term lasting effect, but to what extent is still unanswered.A 2010 study by the American Meteorological Society is the first modern attempt to quantify these effects. In their report, they tracked the effects of 17 stratospheric smoke plumes in 2002. What they found is that the average time that the smoke plumes’ presence in the stratosphere was detectable, was only about 2 months. The report indicates that particles of carbon soot start to clump together at some point after interacting with sunlight and then drop out of the stratosphere quickly.[2] This happens in weeks not in years, a major contradiction to the premise of nuclear winter theories. What isn't known is there a tipping point of equilibrium that would keep the soot aloft if there was enough of it. So like many things, there is a certain element of the unknown in this.What is known is that the TTAPS study, made famous by Carl Sagan and his team, used exaggerated volumes of soot and smoke in their model. Their assumptions for a nuclear winter were significantly off in their calculations. Key government studies since then have shown that the available combustible materials used in the models in TTAPS were significantly overstated and this has flawed all the studies since that have used the TTAPS study as the basis of their work.The nuclear winter theory relies heavily on the worst case scenario of many of the events that would unfold during a nuclear exchange and as such exaggerates the effect dramatically. [3] A contemporary example of prediction not accurately modeling reality is the forecast effects of the Iraqis setting 600 oil rigs ablaze in 1991.Following Iraq's invasion of Kuwait and Iraqi threats of igniting the country's 800 or so oil wells were made, speculation on the cumulative climatic effect of this, presented at the World Climate Conference in Geneva that November in 1990, ranged from a nuclear winter type scenario, to heavy acid rain and even short term immediate global warming.As threatened, the wells were set ablaze by the retreating Iraqis in March of 1991 and the 600 or so successfully set Kuwaiti oil wells were not fully extinguished until November 6, 1991, eight months after the end of the war During this time they consumed an estimated six million barrels of oil daily at their peak intensity.In articles printed in the Wilmington morning star and the Baltimore Sun newspapers of January 1991, prominent authors of nuclear winter papers — Richard P. Turco, John W. Birks, Carl Sagan, Alan Robock and Paul Crutzen —together collectively stated that they expected catastrophic nuclear winter like effects with continental-sized effects of "sub-freezing" temperatures as a result of the Iraqis going through with their threats of igniting 300 to 500 pressurized oil wells that could subsequently burn for several months.[4]Carl Sagan later conceded in his book The Demon-Haunted World that his predictions obviously did not turn out to be correct: "it was pitch black at noon and temperatures dropped 4–6 °C over the Persian Gulf, but not much smoke reached stratospheric altitudes and Asia was spared.”The problems with the models that started the nuclear winter debate, the models used by Sagan and other teams of scientists at that time, is obvious when you look at the detail. The analysis was done at extremely low resolution and with no feedback loops. It was a 2D model, not a 3D model, so the volume and altitude of particles, heat flux, and fuel "mass loading" (the amount of fuel per square meter) were never actually calculated. The numbers were made uniform and plugged in as a single result for the entire world. So the heat flux, fuel “mass loading”, soot, smoke and debris was uniform no mater if the city was Fargo North Dakota or Los Angeles. It was inherently wrong and fatally flawed. [5][6]The atmospheric scientist tasked with studying the atmospheric effect of the Kuwaiti fires by the National Science Foundation, Peter Hobbs, stated that the fires' modest impact suggested that "some numbers (used to support the Nuclear Winter hypothesis)... were probably a little overblown.”[7]In a paper by the United States Department of Homeland Security finalized in 2010, fire experts stated that due to the nature of modern city design and construction, with the US serving as an example, a firestorm is unlikely after a nuclear detonation in a modern city. This is not to say that fires won't occur over a large area after a detonation, but that the fires would not coalesce and form the all-important stratosphere punching firestorm plume that the nuclear winter papers require as a prerequisite assumption in their climate computer models. Additional recent studies on smoke columns indicate that nearly every possible fire scenario results in little to no stratospheric injection of smoke..[8]The nuclear bombing of Nagasaki for example, did not produce a firestorm. This was similarly noted as early as 1986-88, when the assumed quantity of fuel "mass loading" in cities underpinning the winter models was found to be too high and intentionally creates heat fluxes that loft smoke into the lower stratosphere, yet assessments "more characteristic of conditions" to be found in real-world modern cities, had found that the fuel loading, and hence the heat flux that results from burning, would rarely loft smoke much higher than 4 km.[9]The scenarios contributing to a firestorm are also dependent on the size of bombs being used. Only bombs in the 1-megaton range and higher would ignite a sufficiently large area for firestorms to coalesce crossing over from sparsely located high fuel-load areas into these lower fuel-loaded areas in a mixed city model, such as Nashville.[10] [11]Russell Seitz, Associate of the Harvard University Center for International Affairs, argues that the winter model's assumptions give results which the researchers want to achieve and is a case of "worst-case analysis run amok". Seitz criticized the theory for being based on successive worst-case events.[12]Notes from “Disaster Preparedness, An International Perspective”: “If the amount of smoke assumed in the “nuclear winter” report (Science, v222, 1983, pp1283-92) were decreased by a factor of 2.5, the climatic effect would probably be trivial. In considering the actual terrain that surrounds most likely targets, the probable type of explosions (ground bursts against hardened military facilities), the overlapping of targets, and conditions that could reduce the incendiary potential of the thermal pulse, critics of the report believe that the quantity of smoke from non-urban fires has probably been overestimated by at least a factor of ten (Cresson Kearny, Fire Emissions and Some of Their Uncertainties, Presented at the Fourth International Seminar on Nuclear War, Erice, Sicily, August 19-24, 1984). Rathjens and Siegel (Issues in Science and Technology, v1, 1985, pp123-8) believe there would likely be four times less smoke and eight times less soot from cities than estimated in the National Research Council study.”[13]Putting the fires of a nuclear war in another perspective. Every year on earth, wildfires consume 350,000,000 - 450,000,000 hectares of forests, grasslands and structures and results in an average of 339,000 deaths worldwide. [14] This is equal to 1,700,000 square miles burned every year worldwide, nearly half the size of the entire Unites States. Earlier in this document, I laid out a hypothetical scenario where every nuclear bomb in existence, excluding ones listed as retired, are spread out equally at a density of 1 bomb every 100 square miles (10,000 bombs x 100 square miles = 1,000,000 square miles). Under that scenario, the bomb coverage only extends over 1/3 of the land mass of the USA (the USA is 3,800,000 square miles). The world burns more already every year without sending the climate into a nuclear winter. This also is equal to half the CO2 released from burning fossil fuels annually.[15] Wild fires release massive amounts of energy on a scale equivalent to nuclear weapons. The Chisholm Fire, a man-caused forest fire in Edmonton, Alberta, Canada in 2001 released the equivalent energy of 1200 Hiroshima atomic detonations.[16] The firestorm after the bombing of Hiroshima released 200 times the energy of the atomic bomb itself.Taking all that into consideration and taking the available megatonnage in today's arsenals and and adjusting the implied atmospheric load of carbon black soot you might end up with 5 teragrams aloft in the lower stratosphere resulting in a 2–3 °C drop for several months to, worst case, several years. Not quite a nuclear winter, barely a nuclear fall… and even that is debatable since evidence suggests a much shorter time of smoke suspension in the stratosphere and that the premise on uncontrolled fire storms is unfounded based upon actual observations of the bombs dropped in 1945. While Hiroshima did experience a firestorm Nagasaki did not. Nagasaki was a city with much more combustible material than most modern day cities. The great flaw with the original nuclear winter models is that it assumed the same high loading of fuel for all cities and that firestorms would occur at all those locations. A firestorm isn’t assured and is considered unlikely in modern cities, and thus the theory is flawed from top to bottom.An interesting note about several major recent reports to the contrary of my conclusion, and even ones going back 10 years. None of these reports question the fuel loading and levels of atmospheric smoke generated. They all seem to use the original basis as put forth by Carl Sagan’s team, even though Sagan himself admitted his model did not work. The footnote here will take you to an example of the poor quality models still being pushed as real science. A Rutgers 2010 report that references the work by Sagan offers no explanation for the mechanism of smoke and soot transport into the stratosphere. Quality work is not guaranteed just because the sources are listed as a professionals in this field. Healthy skepticism is your friend, use it.[17]So nuclear winter was always a stretch because the science was unfounded and we never had enough high-yield bombs in reality to cause it ever, but for sure in 2016 because we don’t have any in the high-yield range required within the active arsenals of the nuclear nations at all (other than a small quantity of bombs held by China, around 50 and not enough to change these outcomes).Final ThoughtsI have always been intrigued by the specter of nuclear weapons and the power of the atom. I have a not insignificant set of reference books I have collected over the years. The ones from the late 1940’s and early 1950’s are quite amusing; we did not know what we were really dealing with back then.We have come a long way since the era of Dr. Strangelove.I have come full circle in my understanding and no longer buy into the popular myths because the clear science is there that tells you otherwise. However, having this knowledge may not be a blessing. Knowing that nuclear weapons are not the end of mankind in 2016 isn’t necessarily a great truth to latch onto. The pain and suffering that would transpire from the use of these weapons should always remain a strong deterrent from their use.Making the unthinkable thinkable, was there some sanity in the insanity of MAD?In dismissing the notions of nuclear winter and MAD (mutually assured destruction), could we be making the use of these weapons more palatable as a tool of political and ideological foreign policy enforcement? A quick fix to the next ISIS where collateral damage is deemed acceptable? Is that something we can manage as a civilization? Are our values within society as a whole, strong enough to kill any temptation in the future if using these weapons seems like a quick fix for an immediate problem? Is a limited exchange something to be seriously considered? Or are we better off letting our imagination embrace a nightmare, a dark vision of reality, a nuclear winter, with complete conviction without regard to the truth?Note: I make no claim that I am right… I only offer an analysis with considerations for details and data overlooked by others … sometimes intentionally. Please do your own due diligence and make an educated determination for yourself.Additional Notes and Recommended Follow-on ReadingObama committed to a major nuclear triad upgrade in order to get the Senate support of the New Start Treaty in 2010. This article does a good job questioning the reasons why we are planning to spend $1 trillion on nuclear weapons systems upgrades over the next couple of decades. It is a worthwhile read, and the access is free with registration. https://www.foreignaffairs.com/articles/americas/2016-08-01/rethinking-nuclear-policyAlso: Allen E Hall's answer to Are we in less danger or more danger today from a 1st strike?A Nuclear Conflict with Russia is Likelier Than You Thinkand with unplanned yet uncanny timing …on 60 minutes tonight 9/25/2016 Risk of nuclear attack risesA well thought out and compelling Harvard report “The end of MAD” argues that America’s technological edge and the reduced nuclear arsenals are actually compelling the USA towards a first strikeThis article makes three empirical claims. First, the strategic nuclear balance has shifted dramatically since the end of the Cold War, and the United States now stands on the cusp of nuclear primacy. Second, the shift in the balance of power has two primary sources: the decline of the Russian nuclear arsenal and the steady growth in U.S. nuclear capabilities. Third, the trajectory of nuclear developments suggests that the nuclear balance will shift further in favor of the United States in the coming years. Russia and China will face tremendous incentives to reestablish mutual assured destruction, but doing so will require substantial sums of money and years of sustained effort. If these states want to reestablish a robust strategic deterrent, they will have to overcome current U.S. capabilities, planned improvements to the U.S. arsenal, and future developments being considered by the United States. U.S. nuclear primacy may last a decade or more[18]If this becomes a trend, the nuclear winter bit might need another take: Architects design 'world's tallest' wooden skyscraperFootnotes[1] The National Academies Press[2] http://journals.ametsoc.org/doi/pdf/10.1175/2010BAMS3004.1[3] http://www.tandfonline.com/doi/pdf/10.1080/02786828908959219[4] Doomsday Scenarios[5] http://www.atmos.washington.edu/~ackerman/Articles/Turco_Nuclear_Winter_83.pdf[6] http://www.junkscience.com/wp-content/uploads/2016/04/Nuclear-winter_MetAtmPhys1988.pdf[7] It Happened Here[8] https://www.remm.nlm.gov/PlanningGuidanceNuclearDetonation.pdf[9] http://personalpages.manchester.ac.uk/staff/S.Utyuzhnikov/Papers/AMM_SU.pdf[10] http://www.dtic.mil/dtic/tr/fulltext/u2/a240444.pdf[11] Nuclear Disasters & The Built Environment[12] In from the cold: 'nuclear winter' melts down[13] http://www.physiciansforcivildefense.org/PDF/5.pdf[14] Wildfires kill 339,000 people per year: study[15] Global Wildfires, Carbon Emissions and the Changing Climate - Future Directions International[16] http://www.atmos-chem-phys.net/6/5247/2006/acp-6-5247-2006.pdf[17] http://climate.envsci.rutgers.edu/pdf/WiresClimateChangeNW.pdf[18] http://belfercenter.hks.harvard.edu/files/is3004_pp007-044_lieberpress.pdf
I'm in college and I don't learn anything at all. I only have 1 year left (out of 5), but I'm totally unhappy and I know that I'm wasting my life. Would you drop out if you were me?
Honestly, you would be a fool to drop out. You’re most of the way through, you’ve spent most of the money. There aren’t any refunds.One more year, and you have a degree. Drop out, and you’ve effectively set four years of tuition money on fire. You also get to write “high school diploma” when you fill out anything and everything that asks for your “highest completed level of education.” Like job applications. No one, other than marketers, cares if you have “some” or “most” of a degree. “Most” of a degree, equals no degree at all.And if you realize you screwed yourself over, and want to go back? You better hope you did it soon enough that your degree program still exists (mine didn’t!). You need to hope you did it soon enough that you don’t have to retake science or tech classes, even if they just count as core electives (I didn’t). You even need to hope that they don’t drastically CHANGE the requirements of the degree program, which could mean losing a lot of ground, and spending more time and money on classes than if you stayed. And, heaven help you if you move, even if the credits are from an accredited institution. Those are regional, and schools LOVE to soak students for extra tuition dollars, by callously refusing to accept perfectly good credits.I don’t disagree that, in many cases, college as a learning experience is a joke. It’s more like an expensive-as-hell, half-way house, to transition children into young adults. If you’re smart and lucky (and went to a great school), you also hopefully get some invaluable social and professional networking contacts.BUT. The MOST IMPORTANT THING about staying one more year, is that you get that stupid piece of paper. Trust me, unless you’re some rarefied genius, with a ton of raw talent AND pure dumb luck (like some of those startup millionaires and billionaires), you will regret dropping out at some point. That lack of paper will be held against you, often unfairly.Even if you develop a strong career, you will find companies use that lack of a degree as an excuse to pay you less, despite years of experience and accomplishments. It will hold you back for promotions. And when you’re ready to move to a new company, it will limit which companies you can apply to.Tons of companies have policies where they just plain don’t hire people without a degree, no matter how smart or accomplished they are. Even for jobs that traditionally offered a decent professional track for people with only a high school diploma - like store managers. Want to manage a Target? You need a college degree. Doesn’t matter if you have 30 years of sterling store management experience. No, you can’t work your way up from cashier over time, the way people did back in the days of department stores, like Caldor - a now defunct, discount department store once referred to as “the Bloomingdale’s of discounting.”Then, there are places that not only won’t hire you on sheer stupid principle, they CAN’T hire you if you don’t have a degree. Some universities, like U of I, have so-called “academic professional” positions, which means a degree is mandatory, for skilled non-teaching positions. The apparent purpose of creating the “academic professional” designation is to prevent unionization, from what I’ve been told. And these positions are often one-year, lower-than-industry-standard-paying, salaried contract positions. Hence the need to actively guard against unionization!I can even remember 30 years ago, looking at a job application for a receptionist position at a non-profit animal shelter (I love animals). No benefits, part-time work, pay was beyond crap. Like, you can make more flipping burgers kind of crap. They wouldn’t even accept an application without a degree. Did that make sense? No.TL/DR: I won’t try to argue whether the actual education is worth anything. That stupid piece of paper, however, is disproportionately important to too many people and companies. Just suck it up and finish.
- Home >
- Catalog >
- Miscellaneous >
- Organizational Chart Template >
- Fire Department Organizational Chart >
- fire department organizational chart template >
- Fire Science Professional Track