How to Edit Your Instructions 1099 R 2011 Online Free of Hassle
Follow these steps to get your Instructions 1099 R 2011 edited in no time:
- Click the Get Form button on this page.
- You will be forwarded to our PDF editor.
- Try to edit your document, like adding date, adding new images, and other tools in the top toolbar.
- Hit the Download button and download your all-set document for the signing purpose.
We Are Proud of Letting You Edit Instructions 1099 R 2011 super easily and quickly
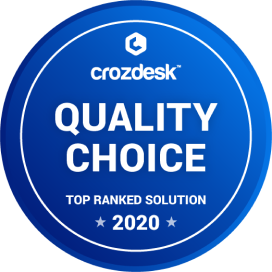
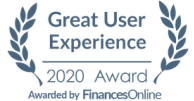
How to Edit Your Instructions 1099 R 2011 Online
When dealing with a form, you may need to add text, put on the date, and do other editing. CocoDoc makes it very easy to edit your form in a few steps. Let's see the simple steps to go.
- Click the Get Form button on this page.
- You will be forwarded to this PDF file editor webpage.
- In the the editor window, click the tool icon in the top toolbar to edit your form, like adding text box and crossing.
- To add date, click the Date icon, hold and drag the generated date to the field to fill out.
- Change the default date by modifying the date as needed in the box.
- Click OK to ensure you successfully add a date and click the Download button for sending a copy.
How to Edit Text for Your Instructions 1099 R 2011 with Adobe DC on Windows
Adobe DC on Windows is a must-have tool to edit your file on a PC. This is especially useful when you do the task about file edit on a computer. So, let'get started.
- Click and open the Adobe DC app on Windows.
- Find and click the Edit PDF tool.
- Click the Select a File button and select a file to be edited.
- Click a text box to give a slight change the text font, size, and other formats.
- Select File > Save or File > Save As to keep your change updated for Instructions 1099 R 2011.
How to Edit Your Instructions 1099 R 2011 With Adobe Dc on Mac
- Browser through a form and Open it with the Adobe DC for Mac.
- Navigate to and click Edit PDF from the right position.
- Edit your form as needed by selecting the tool from the top toolbar.
- Click the Fill & Sign tool and select the Sign icon in the top toolbar to make a signature for the signing purpose.
- Select File > Save to save all the changes.
How to Edit your Instructions 1099 R 2011 from G Suite with CocoDoc
Like using G Suite for your work to finish a form? You can do PDF editing in Google Drive with CocoDoc, so you can fill out your PDF in your familiar work platform.
- Integrate CocoDoc for Google Drive add-on.
- Find the file needed to edit in your Drive and right click it and select Open With.
- Select the CocoDoc PDF option, and allow your Google account to integrate into CocoDoc in the popup windows.
- Choose the PDF Editor option to move forward with next step.
- Click the tool in the top toolbar to edit your Instructions 1099 R 2011 on the applicable location, like signing and adding text.
- Click the Download button to keep the updated copy of the form.
PDF Editor FAQ
Why hasn't a mathematical model to beat the stock market been found?
Short answer: It has been done already. I did it.Long answer: Below is how I did it, in detail. It is way too long for most readers so you can skip the entire section labeled “Software R&D” and skip down to the “Final Design” and “Update” section.<< I make no claims about this answer. You will have to judge for yourself if it has any value to you. To me, it is old news and would take a long time to replicate but I offer it only to point out that it is possible to create a math model that can beat the market, however, as I learned, the final model relies heavily on subjective data.>>BackgroundOne of the most interesting applications of computer technology is in the field of investing. It is interesting that with all the sophisticated systems and all the monetary rewards possible, that there has not been a successful program that can guide a broker to make foolproof investment predictions…..until now.It is a fact that out of all the investors and resources on Wall Street, that none of them do much better than just slightly above random selection in picking the optimum investment portfolio. Numerous studies have been done on this subject that show that the very best investment advisers have perhaps a 10% or 15% improvement over random selection and that even the best analysts cannot sustain their success for very long.There are lots of people that are able to see very near term trends (on the order of a few days or a week or two, at most) and invest accordingly but no one has figured out how to consistently predict stock rises and falls over the long term (more than 3 or 4 weeks out). That was the task I attempted to solve – not because I want to be rich but because it seemed like an interesting challenge. It combines the math of finance and the psychology of sociology with computer logic.I did a lot of research and determined that there is, in fact, no one that knows how to do it but there is a lot of math research that says that it should be able to be predictable using complex math functions, like chaos theory. That means that I would have to create the math and I am not that good at math. However, I do know how to design analytical software programs so I decided to take a different approach and create a tool that will create the math for me. That I could do.Let me explain the difference. In college, I took programming and one assignment was to write a program that would solve a six by six numeric matrix multiplication problem but we had to do it in 2,000 bytes of computer core memory. This uses machine code and teaches optimum and efficient coding. It is actually very difficult to get all the operations needed in just 2k of memory and most of my classmates either did not complete the assignment or worked hundreds of hours on it. I took a different approach. I determined that the answer was going to be whole positive numbers so I wrote a program that asked if “1” was the answer and checked to see if that solved the problem. When it didn’t, I added “1” to the answer and checked again. I repeated this until I got to the answer. My code was the most accurate and by far the fastest that the instructor had ever seen.I got the answer correct and fast but I didn’t really “solve” the problem. I simply exploited the strengths of computers. That is how I decided to approach this investment problem. I created a program that would take an educated guess at an algorithm that would predict future stock values. If it was wrong, then I altered the algorithm slightly and tried again. The initial guessed algorithm needed to be workable and the method of making the incremental changes had to be well thought out.It should be noted that although I did this some years ago, this technique has now been codified into a new kind of programming called “deep learning” which is a subset of machine learning. My design was essentially a very clumsy artificial intelligence with a crude and simplistic self-learning process before those buzz words were poplar. In today’s software tools and faster processors, this task would be almost trivial and certainly take much less time and effort.About this time, the 2008 crash happened. I had been watching the market for some time and got out in late 2007 because I knew something had to give. I ended up make a fair amount of money when I reinvested at the bottom and rode it to new highs but it was mostly on gut feelings. I wanted to make use of some kind of automation or calculated indicator that was foolproof. I felt it was possible and tried to find a way to do itSoftware R&DWay back in early 2009, as I saw it, the answer was to use something called forward chaining neural nets with an internal learning or evolving capability that is related to the idea of was called then a unification algorithm. I could get real technical but the gist of it is this –I first created a placeholder program (N0. 1) that starts with an initial algorithm for hundreds of possible variables but has many of them set to 1 or zero. This basic algorithm is made up of micro and macroeconomic formulas as well as having an allowance for a wide variety of projected factors in the form of “a*f(x). It then selects inputs from available data and assigns that data to the variable placeholders for that data. It then refines a possible formula that might predict the movements of the stock market using various inference rules to extract more data. Following an interim evaluation process, it uses the inferred data in a feedback and then repeats the process. The evaluation process also looks to see if it is moving toward an end goal. If it is, then it continues. If t is not, then it terminates the chain and resets back to a new set of input data.This program has the option to add additional input parameters, constants, variables, input data and computations to the placeholder formula. It seeks out data to insert into the formula. I also allowed it to vary the operator between factors so that if it added a factor, it could add it or multiply it, or divide by it, etc. I also allowed boolean factors so that it could use “and”, “or” and “if” operators between factors.These variables were initially randomized using the Monte Carlo iteration method but would settle in on those choices that worked better. In a sense, it allows the formula to evolve into totally new algorithms that might include content that has never been considered before.Then I created a program (No. 2) that executes that formula created by program No. 1, using all the available input data and the selected parameters or constants and generates specific stock predictions. This program applied a Monte Carlo kind of interruption in which all the parameters, factors and operators are varied over a range in various combinations and then the calculations are repeated. It also can place any given set of available data into various or multiple positions in the formula. This can take hundreds of thousands (up to millions) of repetitions of executing the formula to examine all the possible combinations of all of the possible variations of all the possible variables in all the possible locations in the formula. Each iteration of the execution is recorded in a database along with its final answer and fed into a third program.Then I created a program (No. 3) that evaluates the results against known historical data. If the calculations of program No. 2 are not accurate, then this third program notifies the first program and it changes its inputs and/or its formula and then the process repeats. This third program can keep track of trends that might indicate that the calculations are getting more accurate and makes appropriate edits in the previous programs. This allows the process to begin to focus toward any algorithm that begins to show promise of leading to an accurate prediction capability.I should point out that this model evolved over time. As I thought of new factors or read about new analysis methods, I tried to add them into the appropriate program. For instance, I invented a number of factors that I thought might have some value. For instance, I wrote code that would take words and phrases from an investment and economics dictionary and then search newspaper and online text databases and count the number of times that word or phrase was used over a given period of time. I, later on, added a social media text processing and semantic analysis that was just beginning to be used by sociologists to examine attitudes on Twitter and Facebook. This later proved to be a valuable aspect of the final formula and ended up being associated with multiple factors and operators within the final algorithm.I initially also had numerous steps that required manual setup or inputs to get it to work - like downloading a database, reformatting it into a usable form, writing an extract and sort routine and then feeding it into the No. 1 program. This got very tedious so I automated these processes over time as much as I could.This tweaking process continued for years.I then created sort of a super command override program that first replicates this entire three-program process and then manages the results of the outputs of dozens of copies of the number 2 and 3 program and treats them as if they were one big processor. This master executive program can override the other three by injecting changes that have been learned in other sets of the three programs. This allowed me to set up multiple parallel versions of the three-program analysis across multiple networked computers and speed the overall analysis many times over.Each iteration of the analysis that showed it to be a positive improvement over the last version was saved using what I called its “key”. This was a coded alpha-numeric sequence that I could decypher back to being able to replicate the formula that was derived in the analysis. I kept a record of the past 25 keys that showed progressive improvements. Initially, the key had 36 separate factors in it with 19 of them being dummy placeholder values of 1’s or 0’s. I kept track of the growth of this key and the use of those placeholder values.If the time stamp between these keys was close together, that meant that I was quickly closing in on a winning overall algorithm. If they were farther apart, that meant I was making slow progress. I called this my “key time”. I plotted the key time every day to see how I was doing.As you might image this is a very computer intensive program. The initial three programs were relatively small but as the system developed, they expanded into first dozens and then hundreds of parallel copies. All of these copies reading from data sets placed in a bank of DBMS’s or accessible online that represented hundreds of gigabytes of historical data. As the size of the calculations and data grew, I began to divide the data and processing among multiple computers.With my prior and ongoing computer modeling consultation services, I knew people at several large data processing centers that allowed me to use some of their excess and off-demand processing power on mini and mainframe computers and on one super computer. In some circumstances, I was able to replicate as many as 50 copies of my three programs on a single large computer. I used a VPN with encrypted data exchange over the internet for most of the data coordination. To be honest, In some uses, I asked and in others I just sort of snuck in under the radar and used their processing power during low demand periods without asking. To be sure, these were massive processing centers that allowed external access by sharing research facilities and universities so it wasn’t without some precedence.I began with input of financial performance data that was known during the period from 1970 through 2000. This 30 years of data includes the full details of billions of data points and technical indicators about tens of thousands of stocks as well as huge databases of social-economic data about the general economy, politics, international news, and research papers and surveys of the psychology of consumers, the general population and of world leaders. I was surprised to find that a lot of this data had been accumulated for use in dozens of other previous studies. In fact, most of the input data I used was from previous research studies and I was able to use it in its original database form.Program No. 1 used data that was readily available from various sources from these historical research records. Program No.3 uses slightly more recent historical stock performance data and technicals. In this way, I can look at possible predictive calculations and then check them against real world performance. For instance, I input historical 1980 data and see if it predicts what actually happened in 1981. Then I advance the input and the predictions by a year.Since I have all this data, I can see if the 1980-based calculations accurately predicts what happened in 1981? Then I looked at 1980 thru 1982 to see if it could be used to predict 1983. By expanding and repeating this for the entire 30 years of available data, I can try out millions of variations of the analysis algorithms in a Monte Carlo analysis with easy graphic visualizations. Once I find something that works on this historical data. I can advance it forward to input current data to predict future stock performance. If that works then I can try using it to guide actual investments.For each key and key time, I also recorded the percentage of accuracy of the predictions being made against the historical data. This gave me a daily indicator of not only progress but of predictive accuracy being achieved.This has actually been done before. Back in 1991, a professor of logic and math at MIT created a neural net to do just what I have described above. It was partially successful but the software, the input data and the computer hardware back then were far less powerful than what I used. However, I was using so much more data than he used, I found that even my very powerful home computer systems were much too slow to process the massive volumes of data I needed. Even the borrowed and loaned systems I gathered from former co-workers and clients proved to be too slow.To get past this problem, I created a distributive-processing version of my programs that allowed me to split up the calculations among a large number of computers. I then wrote a sort of computer virus that installed these various computational fragments on dozens of college and university computers around the country. Such programs are not uncommon on campus computers and I was only using 2% or 3% of the total system assets but collectively, it was like using 200 high end PC’s or about 1/8th of one super computer.Even with all that processing power, it was more than 18 months and more than 9,700 hours of total processing time on 67 different computers before I began to see a steady improvement in the key time improve and the predictive percentages of the programs to begin evolving. By then, the formula and data inputs had evolved into a very complex algorithm that I would never have imagined but it was closing in on a more and more accurate version. By early 2011, the key had expanded to 63 separate factors with all of them being live variables that were being used in every iteration of the algorithm.<<If you have experience in this sort of thing, you will realize that beginning back in 2009, I was essentially building a machine learning or deep learning program with a little bit of AI thrown in. I was also making use of Big Data and used the analysis and performance of the neural nets using backpropagation. Today, some of the methods I used would be called rule induction or tree induction but back then, it was all part of stochastic analysis and pattern recognition using mostly Bayesian estimation and learning techniques. The descriptive words have changed but the methods and math have been around for a long time. My implementation of these methods was crude, clumsy and slow. Today, even using off-the-shelf AI, Big Data, deep learning and machine language tools, this could all be done must more elegantly and efficiently.>>By this time, mid-2011, I was getting up to 85% accurate predictions of both short term and long term fluctuations in the S&P and Fortune 500 index as well as several other mutual fund indexes.Short term predictions were upward to 95% accurate but that was out only 24 to 96 hours. The long term accuracy dropped off from 81% for 1 week out to just under 60% for 1 year out….but, it was slowly getting better and better.By early 2012, I was up to 96 factors in the key. My key time was averaging about 31 clock hours and the accuracy for 96 hours out had improved to 97% just over 93% for one week.I decided to put some money into the plan. I invested $5,000 in a day-trader account and then allowed my software to instruct my trades. I limited the trades to one every 72 hours and a maximum target of one week out. This was causing the commissions to eat up a lot of the profits from such a small investment but over a period of 6 months, I had pushed that $5,000 to just over $9,000, however there were times when I came close to a wipeout. I tweaked the selection process to reduce my risks on single stocks and began looking only at mutual funds and ETFs. This greatly slowed the growth of my ROI but it also improved my accuracy and reduced my risks. I then settled into an investment routine for more than a year.This partially validated the predictive quality of the formulas but it is just 2.5% of what it should have been if my formula's were exactly accurate. I have since done mock investments of much higher sums and a longer investment interval and had some very good success. I have to be careful because if I show too much profit, I’ll attract a lot of attention and get investigated or hounded by news people. Both of which I don’t want.The entire system was steadily improving in its accuracy but I was also getting more and more of my distributive programs on the college systems being caught and erased. These were simply duplicate parallel systems but it began to slow the overall advance of the key development and the key time processing. I was at a point that I was making relatively minor refinements to a formula that had evolved to 116 factors in the key from all of this analysis.Actually, it was not a single formula any more. To my surprise, what evolved was sort of a process of sequential interactive formulas that used a feedback loop of calculated data that was then used to analyze the next step in the process. This feedback loop was a surprise because I had not specifically design Pgm No. 1 to create feedbacks. The algorithm implemented this by making use of intermediate values created in the early half of the algorithm (in factors 1 to 75 or so) as variable inputs into factors in later key elements.I tried once to reverse-engineer the whole algorithm but it got very complex and there were steps that were totally baffling. I was able to figure out that it looked at the fundamentals of the stock, then it looked at the state of the economy (technicals) which was applied to the stock performance. All that seems quite logical but then it processed dozens of “if-then” statements that related to micro, macro and global economics in a sort of logical scoring process that was then used to modify parameters of the stock performance. This was a mashup of qualified iterative feedback loops that confused me.What was surprising was that these social and economic factors also included a number of semantic searches of multiple social media and news networks. I had added these semantic and emotional social network analysis parameters after reading about it in a magazine about Facebook. I had duplicated some analysis that was done by a university using social media data that gave plus and minus values to specific words that were deemed positive and negative with respect to financials and stocks. This looping and scoring repeated several times and seemed to be the area that was being refined in the final stages of my analysis.I could not follow all of the analysis but I could do a “sensitivity analysis” which is where you try to determine what are the MOST influential and least influential parameters in the calculation. I attempted this several times during the development process and much to my surprise, I found that there was a gradual shift from the technical and stock fundamentals to slowly put more value on the semantic searches of multiple social media and news networks - particularly Facebook and Twitter and WhatsApp. The volume of data and the time spent procession that data from social media sites certainly increased dramatically in the last 18 months of my study. What was baffling was that most of the Big Data text being searched was not particularly focused on economic issues and even less on the stock market.Final Design:By December of 2013, I was satisfied that I had accomplished my goal. I had a processing capability that was proving to be accurate in the 87% to 95% range out to about three to four weeks into the future and then it drops off as you go further out.One worry I had was the legality of what I was doing. In most Nevada casinos, it is illegal to “count cards” in blackjack. Technically, this is not cheating in the sense of looking at the dealer’s cards but nevertheless, it is not permitted. My investment analysis model is technically not cheating in the sense of using insider information but it does impart a distinct advantage that I wonder if it would be seen as illegal.I never intended to use this project to exploit the system. It was mostly just a personal challenge. I did, however, use the formula to earn enough to anonymously send some money to all the universities and colleges that I had stolen computer time from. I also used it to help pay for some of the equipment that I used for this project and then just as sort of a trophy for myself, I bought myself a car with my ROI.Just in case you were wondering, I did report the income on my taxes. actually, you can’t avoid it because all the investments send in 1099 forms on all your gains and losses.But then I felt very guilty about sorta cheating the system. Actually, as I mentioned, I was unclear if this was legal or not, but to be safe, I archived the entire project. It might be able to be resurrected at some point in the future but it would probably take all-new programming using the latest languages and communications protocols and then months to update itself with the latest data - that is, if it could be done at all. It took a long time (over 4 years) but it was fun and satisfying in the end.UPDATE Dec. 2019:If you have read all this so far, then thank you. I got a lot of feedback saying this is not possible or isn’t real. Most base their perspective on the limitations of precise algorithms and calculations and reference the difficulty in working with Chaos Theory. As a computer modeler, I, too, am curious why this worked versus the “technical investor” that uses precise statistics and indicators of stock activity. After some time, I think I have the answer.Crowdsourcing - WikipediaThe Wisdom of Crowds - WikipediaThese links give a lot of examples of how this works. There are also some others that are both classic and defy logical explanations. One I learned a long time ago was how the Navy submarine Scorpion was found after it sank. How to unleash the wisdom of crowds.This initially seems illogical but it has been proven over and over again. In the past, it has been more difficult to gather a large number of crowd inputs to make this work but with the advent of the internet it is easier and with the advent of Big Data resources like Facebook, Quora, Twitter, and similar social networks, it is possible to take in tens of millions of data points around a single issue.The ability to quantify this social media data comes from the evolving science of semantic analysis and more specifically, sentiment analysis. I used a database I found that assigned numbers to words based on their degree of depth of sentiment. Then the semantic analysis algorithm I copied took over and quantified the “crowd’s” consensus on the stock or related topics like the oil industry in general or the use of plastics, etc.As noted in my original answer, the accuracy of my investment model grew significantly after I began to tap into larger and larger public opinion and social networking databases. I’m not so sure that my model leveraged some kind of self-generated AI as it might have simply refined a crowdsourcing algorithm by finding dictionary and database words that were used in abundance within the social media databases that also had some correlation to stock industry movements.This is a form of sequentially assigned inductive logic. A correlation is found between a stock and an general industry or line of business. This is validated as a positive or direct relationship meaning that when the positive semantic/sentiment (higher numbers) go up, then the value of the stock goes up. This is all based on and validated by historical data.This process helps establish a set of words that are found to correspond to a positive semantic/sentiment assessment of the industry. This is validated as a positive or direct relationship that is supported by both the stock’s technical fundamentals and by the actual stock performance. As you can see, this is an iterative process that suits a forward chaining, Monte Carlo, Bayesian analysis very well.This analysis methodology has, in fact, been validated since I did this and is now used by a number of Wall Street investment firms. The only reason they do not dominate the market is that they still inject their own biases, politics and self-interests into the decisions and investments made. You have to also remember that Wall Street is in the “transaction business” and not really into the investment business. Their focus is to make as many trades as possible to gain on the commissions. Buying and holding a stock is not really what they do so the appeal of this kind of a tool is reduced in that environment.I have no doubt that the Wisdom of Crowds and semantic analysis will be an important tool for decision making and investments in the future.
- Home >
- Catalog >
- Miscellaneous >
- Individual Tax Form >
- Irs Forms >
- Irs Form 5498 >
- form 5498 instructions >
- Instructions 1099 R 2011