How to Edit Your What Is Dbn Number Online With Efficiency
Follow these steps to get your What Is Dbn Number edited in no time:
- Click the Get Form button on this page.
- You will be forwarded to our PDF editor.
- Try to edit your document, like adding checkmark, erasing, and other tools in the top toolbar.
- Hit the Download button and download your all-set document for the signing purpose.
We Are Proud of Letting You Edit What Is Dbn Number Like Using Magics
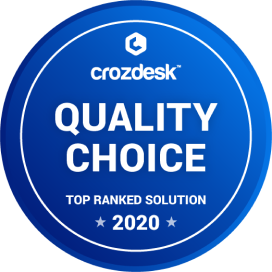
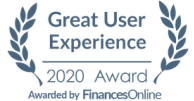
How to Edit Your What Is Dbn Number Online
When dealing with a form, you may need to add text, fill in the date, and do other editing. CocoDoc makes it very easy to edit your form in a few steps. Let's see how can you do this.
- Click the Get Form button on this page.
- You will be forwarded to our free PDF editor webpage.
- In the the editor window, click the tool icon in the top toolbar to edit your form, like highlighting and erasing.
- To add date, click the Date icon, hold and drag the generated date to the field to fill out.
- Change the default date by modifying the date as needed in the box.
- Click OK to ensure you successfully add a date and click the Download button when you finish editing.
How to Edit Text for Your What Is Dbn Number with Adobe DC on Windows
Adobe DC on Windows is a must-have tool to edit your file on a PC. This is especially useful when you like doing work about file edit on a computer. So, let'get started.
- Click and open the Adobe DC app on Windows.
- Find and click the Edit PDF tool.
- Click the Select a File button and select a file to be edited.
- Click a text box to make some changes the text font, size, and other formats.
- Select File > Save or File > Save As to keep your change updated for What Is Dbn Number.
How to Edit Your What Is Dbn Number With Adobe Dc on Mac
- Browser through a form and Open it with the Adobe DC for Mac.
- Navigate to and click Edit PDF from the right position.
- Edit your form as needed by selecting the tool from the top toolbar.
- Click the Fill & Sign tool and select the Sign icon in the top toolbar to make a signature for the signing purpose.
- Select File > Save to save all the changes.
How to Edit your What Is Dbn Number from G Suite with CocoDoc
Like using G Suite for your work to finish a form? You can make changes to you form in Google Drive with CocoDoc, so you can fill out your PDF in your familiar work platform.
- Integrate CocoDoc for Google Drive add-on.
- Find the file needed to edit in your Drive and right click it and select Open With.
- Select the CocoDoc PDF option, and allow your Google account to integrate into CocoDoc in the popup windows.
- Choose the PDF Editor option to move forward with next step.
- Click the tool in the top toolbar to edit your What Is Dbn Number on the field to be filled, like signing and adding text.
- Click the Download button to keep the updated copy of the form.
PDF Editor FAQ
What is the correct syllabus of machine learning?
Self Notes on ML and Stats.ContentsBasicsSupervised,unsupervised,reinforcementBias-variance trade-offOverfitting, underfittingGradient descent:-batch,stochasticResampling methodsBootstrappingCross-ValidationLinear discriminant analysis (LDA)Principal Component Analysis(PCA)Learning Vector Quantization (LVQ)Regularization methods:- Ridge,LASSOKernel smoothing methodsEnsemble learning:-Bagging(bootstrap aggregation),boosting,stacking,blendingOrdinary least squaresPartial Least squaresKernel density EstimationRadial basis functionsMulti co-linearity :Detection(Tolerance,VIF,correlation matrix) and treatmentAIC,BICK-fold cross validationGeneralized Additive Models (GAMs)Multivariate Adaptive Regression Splines(MARS)Gradient boostingNLPWord sense disambiguationPronoun resolutionMachine translationTokenizationRegular expressionsStemmingLemmatizationEvaluation metricsAUCPrecisionRecallSpecificityMean absolute percentage errorRoot mean square errorAlgorithmsLinear regression: Usually performed through OLSLogistic regressionNaive BayesK-Nearest NeighborsK means clusteringClassification and regression trees(CARTs)Support vector machinesAdaBoostRandom forestARIMADecision TreesID3CHAIDC4.5, C5.0Hierarchical ClusteringMiscellaneousCurse of dimensionalityNo free lunch theoremOccams RazorDeep LearningNeural NetworksBayesian neural netsDeep Boltzmann Machine(DBM)Deep Belief Networks(DBN)Convolutional Neural NetworksStatsHypothesis testingConfidence intervalsP-valuedifferent types of testsTypes of SamplingScedasticity2) Gradient descentGradient descent is an optimization algorithm used to find the values of parameters (coefficients) of a function (f) that minimizes a cost function (cost)Batch Gradient DescentOne iteration of the algorithm is called one batch and this form of gradient descent is referred to as batch gradient descent. Batch gradient descent is the most common form of gradient descent described in machine learningStochastic gradient descentIn this the gradient descent variation the update to the coefficients is performed for each training instance, rather than at the end of the batch of instances3 a)BootstrappingIn the context of machine learning with bootstrapping, we’re drawing random samples from another sample to generate a new sample that has a balance between the number of samples per class. This is useful when we’d like to model against a dataset with highly unbalanced classes.3 b) Cross ValidationCross-validation (also called rotation estimation) is a method to estimate how well a model generalizes on a training dataset. In cross-validation we split the training dataset into N number of splits and then separate the splits into training and test groups. We train on the training group of splits and then test the model on the test group of splits. We rotate the splits between the two groups many times until we’ve exhausted all the variations4 ) LDAParametric, linear, Classification algorithm used to classify more than two categoriesIf you have more than two classes, then the Linear Discriminant Analysis is the preferred linear classification technique.22 a) Linear RegressionIt is a linear, parametric, regression algorithm.Different techniques can be used to prepare or train the linear regression equation from data, the most common of which is called Ordinary Least Squares.OLS:When we have more than one input we can use Ordinary Least Squares to estimate the values of the coefficients. The Ordinary Least Squares procedure seeks to minimize the sum of the squared residuals.Gradient Descent:When there are one or more inputs you can use a process of optimizing the values of the coefficients by iteratively minimizing the error of the model on your training data. This operation is called Gradient DescentRidge and LASSO:There are extensions of the training of the linear model called regularization methods. These seek to both minimize the sum of the squared error of the model on the training data (using Ordinary Least Squares) but also to reduce the complexity of the model (like the number or absolute size of the sum of all coefficients in the model).Two popular examples of regularization procedures for linear regression are:Lasso Regression: where Ordinary Least Squares is modified to also minimize the absolute sum of the coefficients (called L1 regularization). ˆRidge Regression: where Ordinary Least Squares is modified to also minimize the squared absolute sum of the coefficients (called L2 regularization)Assumptions for linear regression:Linear Assumption Linear regression assumes that the relationship between your input and output is linear.Remove NoiseRemove CollinearityGaussian Distributions. Linear regression will make more reliable predictions if your input and output variables have a Gaussian distribution.Rescale Inputs: Linear regression will often make more reliable predictions if you rescale input variables using standardization or normalization22 b) Logistic regressionIt is a linear, parametric classification algorithm. One vs Rest logistic regression can be used to classify multiple categoriesLogistic regression is named for the function used at the core of the method, the logistic function. The logistic function, also called the sigmoid functionLogistic regression models the probability of the default classLogistic regression is a linear method, but the predictions are transformed using the logistic function22 c) Naive bayesClassification algorithmIt is called naive Bayes or idiot Bayes because the calculation of the probabilities for each hypothesis are simplified to make their calculation tractable. Rather than attempting to calculate the values of each attribute value P(d1, d2, d3|h), they are assumed to be conditionally independent given the target value and calculated as P(d1|h) × P(d2|h) and so on. This is a very strong assumption that is most unlikely in real data22 d )KNNk-Nearest Neighbors (KNN) algorithm is a non-parametric for classification and regressionKNN is used for regression problems the prediction is based on the mean or the median of the K-most similar instances.22 f) CARTIt is a nonlinear algorithmClassically, this algorithm is referred to as decision treesFor classification the Gini cost function is used which provides an indication of how pure the leaf nodes are (how mixed the training data assigned to each node is).The most common stopping procedure is to use a minimum count on the number of training instances assigned to each leaf node.You can use pruning after learning your tree to further lift performance. The complexity of a decision tree is defined as the number of splits in the tree. Simpler trees are preferred. They are easy to understand22 g) SVMNon parametricsupport vector machine is a generalization of a simple and intuitive classifier called the maximal margin classifiersupport vector machine, which is a further extension of the support vector classifier in order to accommodate non-linear class boundariesA hyperplane is a subspace whose dimension is one less than that of its ambient spacemaximal margin hyperplane (also known as the maximal margin hyperplane optimal separating hyperplane), which is the separating hyperplane that optimal separating hyperplane is farthest from the training observations. That is, we can compute the (perpendicular) distance from each training observation to a given separating hyperplane; the smallest such distance is the minimal distance from the observations to the hyperplane, and is known as the margin.22 k) Decision TreesIt is a Non-parametric, nonlinear algorithmThere are many good ways to decide the variable which should be used for splitting. Below are a few:Gini Index (used for the popular CART algorithm)A perfect separation results in a Gini score of 0Formula for Gini Indexwhere pi is the probability of an object being classified to a particular class.Chi Square (used for CHAID algorithm)CHAID (Chi-square Automatic Interaction Detector) analysis is an algorithm used for discovering relationships between a categorical response variable and other categorical predictor variables.Information Gain/Entropy (used for ID3 algorithm)Reduction in Variance25 a ii)P- ValueQ and A1)Is rotation necessary in PCA?Yes, the rotation is necessary because it maximizes the differences between the variance captured by the components2)What happens if the components are not rotated in PCA?It is a straight effect. If the components are not rotated, then it will diminish eventually, and one must use a lot of various components to explain the data set variance.3)Explain why Naive Bayes is so Naive?It assumes that all the features in the data set are important, equal and independent.4)What is the difference between stochastic gradient descent (SGD) and gradient descent (GD)?Both algorithms are methods for finding a set of parameters that minimize a loss function by evaluating parameters against data and then adjusting.In standard gradient descent, you'll evaluate all training samples for each set of parameters. This is akin to taking big, slow steps toward the solution.In stochastic gradient descent, you'll evaluate only 1 training sample for the set of parameters before updating them. This is akin to taking small, quick steps toward the solution.5)What is the Box-Cox transformation used for?The Box-Cox transformation is a generalized "power transformation" that transforms data to make the distribution more normal.6)What are 3 ways of reducing dimensionality?1. Removing collinear features.2. Performing PCA, ICA, or other forms of algorithmic dimensionality reduction.3. Combining features with feature engineering.7)How can you choose a classifier based on training set size?If the training set is small, high bias / low variance models (e.g. Naive Bayes) tend to perform better because they are less likely to be overfit.If training set is large, low bias / high variance models (e.g. Logistic Regression) tend to perform better because they can reflect more complex relationships8)Explain Principle Component Analysis (PCA).PCA is a method for transforming features in a dataset by combining them into uncorrelated linear combinations.These new features, or principal components, sequentially maximize the variance represented (i.e. the first principal component has the most variance, the second principal component has the second most, and so on).As a result, PCA is useful for dimensionality reduction because you can set an arbitrary variance cutoff.9)What is the advantage of performing dimensionality reduction before fitting an SVM?Support Vector Machine Learning Algorithm performs better in the reduced space. It is beneficial to perform dimensionality reduction before fitting an SVM if the number of features is large when compared to the number of observations.10)How will you find the correlation between a categorical variable and a continuous variable ?You can use the analysis of covariance technqiue to find the correlation between a categorical variable and a continuous variable.11)Difference between factor analysis and PCA?12)Explain p-value. Present it as if talking to a client.?13)Explain what a local optimum is and why it is important in a specific context, such as K-means clustering. What are specific ways of determining if you have a local optimum problem? What can be done to avoid local optima?14)What are feature vectors?15)What is: collaborative filtering, n-grams, cosine distance?16)Explain the difference between Gaussian Mixture Model and KMeans.?17)How would you deal with an imbalanced dataset?18)How do you deal with sparse data?19)Explain bootstrapping as if you’re talking to a non-technical person.20)What do you understand by statistical power and how do you calculate it?21)What’s the Central Limit Theorem and what are its practical implications?22)Tell me what heteroscedasticity is and how to solve it?If the variability of true values along the regression line is not constant, then this condition is known as heteroskedasticity.Heteroscedasticity means unequal scatter.23)Difference between Kmeans and KNN?Good resources:https://remicnrd.github.io./the-machine-learning-cheatsheet/
What is the typical deep learning architecture?
The number of architectures that can be used in a deep learning problem are many and they have many different varieties. Over the last 20 years or so, the deep learning architecture has many developments. The below figure the development of the architecture.These architectures can be applied on a variety of scenarios, to name a few.RNN - Speech recognition, handwriting recognition.LSTM/GRU - Natural language text compression, handwriting recognition, speech recognition, gesture recognition, image captioning.CNN - Image recognition, video analysis, natural language processing.DBN - Image recognition, information retrieval, natural language understanding, failure prediction.DSN - Information retrieval, continuous speech recognition.
Kevin Murphy: Given that deep learning has outperformed other approaches in numerous fields, what is the future of graphical models and kernel methods?
A key part of ML is understanding what types of models are appropriate for different problems. These properties of deep learning are relevant to determining their suitability for a given problem:The current successes of deep learning are all on complex problems and training data with massive numbers of training samples, only moderate numbers of relatively dense feature. These are commonly called $n >> p$ problems.DL models many hypeparameters: network type (simple feed forward, CNN, RNN, adverserial, DBN/RBM, …), activation function(s), number and sizes of layers, connection toplogy, learning rate, regularization type (dropout, lasso, …) and strength, optimization algorithm etc. This means that tuning and model selection are critical to success.DL models take a long time to train even an large GPU clusters and can cost in the many thousands of dollars of compute time to train a single model for some the state of the art models that have made the news recently.DL also does not have a natural way to include prior knowledge in the model.DL is a non-convex option problem, ie there may be local minima and plateaus. This is less important than used to be believed, but can still be an issue in specific circumstances. The problems that used to be attributed to local minima are now generally attributed to plateaus and can be mitigated by better optimization algorithms, activation & loss functions that lead to better behaved energy landscapes, and by massive compute power.DL models are black boxesThese properties point to some of the scenarios where DL may not be optimal or even appropriate:Simple problems. Don’t use cannons on gnats$n << p$ problems. Regularization methods like dropout help reduce the amount of training data needed but SVMs originally designed for this specific type of problem. Ensemble methods like random forests also perform well hereGraphical models are most appropriate when there is significant prior knowledge available and training data is limited. Kernel method can also be suitable here if prior knowledge can be encoded in the kernel.When agility of producing models is more important then accuracy or when computing resources are scarce.Unusual input feature distributions (sparse, long tailed, skewed, multimodal, …) may derail the optimization process.Tree based ensembles are immune to these. Feature transformations to make features better behaved may be necessary for DL to work.When we are more interested in the model itself than its predictionsAlso deep belief networks (ie multilayer restricted Boltzmann machines) are both DL and graphical models.Under and even more liberal interpretation of the term, you could call all NN models “graphical”.Coincidentally the video I just watched is also relevant:
- Home >
- Catalog >
- Life >
- Pregnancy Chart >
- Birth Plan Template >
- simple birth plan >
- What Is Dbn Number