How to Edit The Standard Of Care quickly and easily Online
Start on editing, signing and sharing your Standard Of Care online following these easy steps:
- click the Get Form or Get Form Now button on the current page to make your way to the PDF editor.
- hold on a second before the Standard Of Care is loaded
- Use the tools in the top toolbar to edit the file, and the edits will be saved automatically
- Download your modified file.
A top-rated Tool to Edit and Sign the Standard Of Care
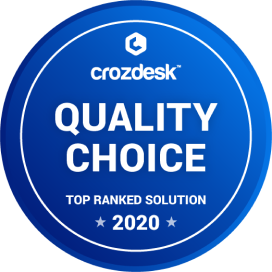
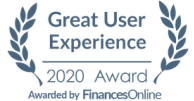
A clear guide on editing Standard Of Care Online
It has become very simple these days to edit your PDF files online, and CocoDoc is the best PDF editor you have ever seen to do some editing to your file and save it. Follow our simple tutorial to start!
- Click the Get Form or Get Form Now button on the current page to start modifying your PDF
- Add, modify or erase your text using the editing tools on the tool pane on the top.
- Affter editing your content, put the date on and create a signature to complete it.
- Go over it agian your form before you click the download button
How to add a signature on your Standard Of Care
Though most people are in the habit of signing paper documents using a pen, electronic signatures are becoming more accepted, follow these steps to finish the PDF sign!
- Click the Get Form or Get Form Now button to begin editing on Standard Of Care in CocoDoc PDF editor.
- Click on the Sign icon in the toolbar on the top
- A box will pop up, click Add new signature button and you'll be given three options—Type, Draw, and Upload. Once you're done, click the Save button.
- Move and settle the signature inside your PDF file
How to add a textbox on your Standard Of Care
If you have the need to add a text box on your PDF and customize your own content, do the following steps to accomplish it.
- Open the PDF file in CocoDoc PDF editor.
- Click Text Box on the top toolbar and move your mouse to carry it wherever you want to put it.
- Fill in the content you need to insert. After you’ve writed down the text, you can take full use of the text editing tools to resize, color or bold the text.
- When you're done, click OK to save it. If you’re not settle for the text, click on the trash can icon to delete it and take up again.
An easy guide to Edit Your Standard Of Care on G Suite
If you are seeking a solution for PDF editing on G suite, CocoDoc PDF editor is a recommendable tool that can be used directly from Google Drive to create or edit files.
- Find CocoDoc PDF editor and set up the add-on for google drive.
- Right-click on a chosen file in your Google Drive and choose Open With.
- Select CocoDoc PDF on the popup list to open your file with and give CocoDoc access to your google account.
- Make changes to PDF files, adding text, images, editing existing text, annotate with highlight, trim up the text in CocoDoc PDF editor before saving and downloading it.
PDF Editor FAQ
Is it possible to diagnose infections by analyzing the cytokine spectrum?
'Is it possible to diagnose infections by analyzing the cytokine spectrum?'Rather than cytokine spectrum alone, blood gene signatures of not just cytokines but other genes as well are being mooted as diagnostic possibilities for infections. For this approach to become a practical reality, initial promising reports from some basic research labs need to be replicated by others using other, larger patient data-sets, and one or more such approach needs to be spun out to commercial entities having the resources necessary to accelerate their translation to clinical medicine. Currently, this type of research is still in the earliest stages of discovery work.Irony is posting this answer on the very day the US SEC charged Theranos, Elizabeth Holmes and Sunny Balwani with fraud (1, 2) only serves to emphasize just how important and lucrative it's becoming to rapidly and accurately diagnose not just infections but also other diseases using just a drop of blood.Potential moneymaking scope isn't the only reason though. Increasingly irresponsible antibiotic usage over recent decades has made responsible antibiotic stewardship an urgent medical priority, where rapidly diagnosing an infection as viral rather than bacterial as early as possible could help minimize unnecessary antibiotic use (3, 4, 5).As illustrative examples of proof of principle, this answer focuses on the work being done in the lab of Purvesh Khatri, a computational immunologist at Stanford University who has in recent years published a few high-profile studies on this topic (6). The approach is something we're going to see more of in the coming years, scouring publicly available large genetic data-sets to discern clinically important patterns.Khatri’s group examined such data-sets obtained from either whole blood or circulating blood cells (PBMC) from both healthy individuals as well as those with bacterial or viral infections or with other types of inflammatory conditions. Specifically, his group examines public Microarray - Wikipedia gene expression databases such as National Center for Biotechnology Information - Wikipedia (NCBI) GEO (Gene Expression Omnibus) looking to see if gene expression patterns map to specific infections.Microarray analysis attempts to find differentially expressed genes between different sets of samples while examining thousands and even tens of thousands of genes simultaneously.In their 2015 study, Khatri's group analyzed 205 samples in 3 data-sets that included healthy controls as well as patients with respiratory viral or bacterial infections (7),They identified 396 genes as being differentially expressed in bacterial versus viral respiratory infections.They identified a unique signature for flu that set it apart from other viral infections.They could even separately identify asymptomatic flu patients who were shedding the flu virus as well as those with flu-like symptoms but who weren't infected with it.In their 2016 study (8),They identified 7 genes they claimed could differentiate bacterial or viral infections.Validated these gene signatures in a group of 96 critically ill children.They identified a 3-gene signature they claim could distinguish patients with active or latent tuberculosis, another infection or no infection. Specifically, they claim this test is far better at identifying those without TB unlike standard TB tests which often miss it in those patients who are unable to spit up sufficient amount of Sputum - Wikipedia needed for diagnosis.While such basic research data appears promising at first blush, using microarray data for clinical diagnosis comes with its own set of limitations that require mitigation in order to make such an approach practical.Need a robust approach to distinguish true from false positives.Data reproducibility between data-sets. Since it can and often is the case that results range all the way from a given gene being found significantly differentially expressed to borderline significance to not significant at all, effect sizes are of greater importance. All the more reason for biologists to wean themselves away from the unfortunate tendency to focus on significance (p value) to the exclusion of more biologically meaningful measures.Meta-analysis - Wikipedia of some publicly available human microarray data-sets suggest there's greater value not in single large studies for a given disease but rather in larger numbers of smaller studies that are moderately powered (Statistical power - Wikipedia) (9).Not all human microarray data studies make all their data publicly available. This is a science policy, not research matter, issue that can only be remedied by funders and regulators.Researchers tend to focus their attention on better annotated genes, which are usually simply those that started being studied earlier, rather than on those that appear to have the strongest evidence supporting their role in a given disease. Such stereotypical 'looking under the lamp-post' attitude stymies discovery of most relevant genetic signatures associated with a particular disease (see below from 10).'Collectively, our results provide an evidence of a strong research bias in literature that focuses on well-annotated genes instead of those with the most significant disease relationship in terms of both expression and genetic variation. We show that the inequality follows a “rich-getting-richer” pattern, where annotation growth is biased towards genes that were richly annotated in the initial versions of GO [Gene Ontology]. We believe this stems from the typical experimental design. To illustrate this, consider an omics experiment that generates a list of hundreds or thousands of interesting genes. To interpret these genes, researchers use GO and pathway analysis tools. The researchers then generate targeted hypotheses for validation by interpreting the list of significant GO terms, focusing the genes or proteins annotated with that GO term. The researchers learn more about those targeted genes, leading to additional GO annotations for the already annotated genes. In this process, the list of unannotated genes is simply ignored because pathway analysis tools cannot map them to any GO terms. Hence, the self-perpetuating cycle of inequality continues.While focusing research on the best characterized genes may be natural because it is easy to formulate a mechanistic hypothesis of the gene’s function in disease, we propose that the researchers in the era of omics should instead allow data to drive their hypotheses. We have repeatedly shown that expanding research outside of the streetlight of well characterized genes identifies novel disease-gene relationships35–37, identifies FDA-approved drugs that can be repurposed for other diseases27, and identifies clinically translatable diagnostic and prognostic disease signatures27,30–34,39. For example, we have previously identified PTK7 as causally involved in non-small cell lung cancer37. At the time of publication, PTK7 was labelled as an orphan tyrosine kinase receptor. In a very short span, this finding was transformed into an antibody-drug conjugate targeting PTK7 that induced sustained tumor regression, outperformed standard-of-care chemotherapy, and reduced frequency of tumor-initiating cells in a preclinical study45. A Phase 1 clinical trial (NCT02222922) of PTK7 antibody drug conjugate, PF-06647020, has already completed with acceptable and manageable safety profile, and is now being considered for further clinical development. To enable researchers to pursue data-driven hypotheses, we have made our rigorously validated gene expression multicohort analysis data publicly available (MetaSignature) where it may be explored based on either diseases or genes of interest29,46. Focusing on genes with the strongest molecular evidence instead of the most annotations would enable researchers to break the self-perpetuating annotation inequality cycle that results in research bias.'Bibliography1. Elizabeth Holmes, Theranos C.E.O. and Silicon Valley Star, Accused of Fraud2. https://www.sec.gov/litigation/complaints/2018/comp-pr2018-41-theranos-holmes.pdf3. Commitments to Responsible Use of Antimicrobials in Humans4. MacDougall, Conan, and Ron E. Polk. "Antimicrobial stewardship programs in health care systems." Clinical microbiology reviews 18.4 (2005): 638-656. Antimicrobial Stewardship Programs in Health Care Systems5. Morency-Potvin, Philippe, David N. Schwartz, and Robert A. Weinstein. "Antimicrobial stewardship: how the microbiology laboratory can right the ship." Clinical microbiology reviews 30.1 (2017): 381-407. How the Microbiology Laboratory Can Right the Ship6. SOHN, EMILY. "Frontiers in blood testing." https://www.nature.com/articles/549S16a.pdf7. Andres-Terre, Marta, et al. "Integrated, multi-cohort analysis identifies conserved transcriptional signatures across multiple respiratory viruses." Immunity 43.6 (2015): 1199-1211. https://ac.els-cdn.com/S1074761315004550/1-s2.0-S1074761315004550-main.pdf?_tid=a93d5cd6-e42b-4eff-9491-6de212501044&acdnat=1520988578_76170b60d7036712945c5c512c72bdbe8. Sweeney, Timothy E., Hector R. Wong, and Purvesh Khatri. "Robust classification of bacterial and viral infections via integrated host gene expression diagnostics." Science translational medicine 8.346 (2016): 346ra91-346ra91. Robust classification of bacterial and viral infections via integrated host gene expression diagnostics9. Sweeney, Timothy E., et al. "Methods to increase reproducibility in differential gene expression via meta-analysis." Nucleic acids research 45.1 (2016): e1-e1. https://pdfs.semanticscholar.org/e718/5ca72f190593b05246f80fdbbc3e0c40422a.pdf10. Haynes, Winston A., Aurelie Tomczak, and Purvesh Khatri. "Gene annotation bias impedes biomedical research." Scientific Reports 8.1 (2018): 1362. https://www.nature.com/articles/s41598-018-19333-x.pdfThanks for the R2A, Huang ZheYu.
What was Brett Kavanaugh's GPA at Yale College? How did it compare to the average GPA at Yale at the time?
TLDR: I estimate Brett Kavanaugh’s GPA at Yale to be between [math]\textbf{3.39}[/math] and [math]\textbf{3.77}[/math]. If I had to take a stab at a single value, it would be [math]\textbf{3.58}[/math] (an average of the bounds). Based on this estimation, Kavanaugh’s GPA was higher than the average Yale GPA in 1987 ([math]3.30[/math]). He was also at least as good as the median student in his class.Step 1: Playing D̶e̶t̶e̶c̶t̶i̶v̶e̶ FBITo estimate Brett Kavanaugh’s GPA at Yale College and how it compares with the average at the time, let’s start off with what we know. First, Kavanaugh graduated cum laude from Yale College in 1987.Next, according to these charts from The Economist, we can gather that the average GPA when Kavanaugh graduated was roughly [math]3.30[/math].Source: What the Ivies can learn from WellesleySource: The Ivy League's crazy grade inflation, in one chart (although this is a Vox article, the article states that the chart is from The Economist).Now comes the fun detective legwork! According to a 1989 opinion article fished out of the Yale Daily News archives, headlined “Diploma Honors Changes Are Too Arbitrary,” the requirement for graduating with cum laude honors in 1987 was to achieve “A” grades in between [math]45\%[/math] and [math]65\%[/math] of classes (achieving “A” grades in more than [math]65\%[/math] of classes would confer magna or summa cum laude status).The opinion piece also states that, “For the last ten graduating classes, anywhere from [math]45[/math] percent to [math]50[/math] percent of students graduated with honors.”Furthermore, this 1988 Letter to the Editors clarifies that the “A” grades referred to in the above opinion piece are actually either “As or A-minuses”.Armed with this information, we can now take a stab at estimating Brett Kavanaugh’s GPA at Yale.Step 2: Making an Ivy-League Educated GuessFor the purposes of this estimation, I will use the following letter-grade-to-GPA mapping:A: 4.00 A-: 3.67 B+: 3.33 B: 3.00 B-: 2.67 C+: 2.33 C: 2.00 C-: 1.67 **A C-minus is the cutoff for “passing” a course at Yale. Therefore, I am not listing the GPA spectrum beyond a C-minus.In the best case, Kavanaugh could have achieved [math]65\%[/math] straight-A grades and [math]35\%[/math] B-plus grades. In this case, his GPA would be [math]0.65 \times 4.0 + 0.35 \times 3.33 = \textbf{3.7655}[/math].In the worst case, Kavanaugh could have achieved [math]45\%[/math] A-minus grades and [math]55\%[/math] C-minus grades. In this case, his GPA would be [math]0.45 \times 3.67 + 0.55 \times 1.67 = \textbf{2.57}[/math]. Granted, this worst-case is extreme. And I can’t be certain that there weren’t any other stipulations in the Yale Blue Book about receiving honors (e.g., requiring a minimum GPA or limiting the amount of grades below a B-minus).A more likely lower bound would be if Kavanaugh had achieved [math]45\%[/math] A-minus grades and an average B/B-plus GPA in his remaining [math]55\%[/math] of classes. This would yield a GPA of [math]0.45 \times 3.67 + 0.55 \times 3.165 = \textbf{3.39225}[/math].From these calculations, we can estimate Brett Kavanaugh’s GPA at somewhere between [math]\textbf{3.39}[/math] and [math]\textbf{3.77}[/math]. If I really had to take a stab a single value, I would just take the average of the lower and upper bounds and guess [math]\textbf{3.58}[/math].Step 3: Confirming… Your Biases, ProbablyAs the average GPA at Yale was [math]3.30[/math], we can likely say that Kavanaugh’s GPA was higher than average. In addition, we know that [math]45–50\%[/math] of students received Latin honors while graduating in 1987, so we can also say that Kavanaugh was at least as good as the median student.To put this into perspective for my more-technologically-oriented peers on Quora: as of 2018, the average GPA in UC Berkeley’s CS Department was a 3.30 as well.So, besides the desperate and unyielding desire to know everyone’s GPA intrinsic to all humans (wait, it’s just me?), why should we care? Well, in his September 27 Senate hearing, Kavanaugh implied that he could not have been a “sloppy drunk” because of how hard he devoted himself to his studies and basketball:HIRONO: [Senator Coons noted] that James Roche said, your roommate, “Although Brett was normally reserved, he was a notably heavy drinker, even by the standards of the time. And he became aggressive and belligerent when he was drunk.” So is your former college roommate lying?KAVANAUGH: I would refer you to what I said in the sealed or redacted portion about his relationship with the other two roommates, and I’m going to leave it at that. I will say – Senator, you were asking about college.I got into Yale Law School. That’s the number one law school in the country. I had no connections there. I got there by busting my tail in college.…KAVANAUGH: And you know, in college – two things. A, I studied. I was in cross-campus library every night, and B, I played basketball for the junior varsity. I tried out for the varsity. The first day I arrived on campus, we had captain’s workouts.I played basketball everyday, all throughout – and then as soon as the season was over, in late February, captain’s workouts started again. I was obsessed with being …HIRONO: So you were not …KAVANAUGH: … the best basketball player.HIRONO: I only have 23 seconds. So you were not a “sloppy drunk,” and so, your roommate was lying.KAVANAUGH: I will refer you – I will refer you, again, to the redacted portion. I’ll say, look at my academic record, and I don’t usually to talk about myself this way, but in response to your – you know, I – I – I worked very hard in college, in my studies, and I also played basketball, I did sports and I also did socialize.Given Kavanaugh’s estimated moderately-above-average GPA, evaluating the validity of this argument is left as an exercise to the reader.AppendixSource: Diploma Honors Changes Are Too ArbitraryFun fact - the author of this piece, Nathaniel Persily, is now the James B. McClatchy Professor of Law at Stanford Law School.Source: New honors system creates unnecessary pressurePhew, well that was fun! And I thought my days of obsessing over GPAs were over when I graduated from college.
What are some of the technologies used in the Google self-driving car?
The Google Self-Driving Car is one of the most popular self-driving vehicles, often synonymous with self-driving car, and a great example of the some of the technologies required for autonomous navigation. To understand the technologies, we need to first understand the problems.Problem #1: What is the world? This is called mapping and refers to understanding the structures of the world around you. Imagine waking up in a strange, perfectly dark room where you can turn on lights gradually to see what's inside the room.Problem #2: Where am I? This is called localization and refers to understanding the relative location you are in the world. Imagine being blindfolded while inside your own house. You know where everything is, but you only get a better, rough approximation through slowly feeling your way around.Robotic navigation is like being in a strange, perfectly dark room with a blindfold on. That's a tough problem to have. And to make it even harder...Problem #3: Did I just run over someone? This is an absolute no-no for self-driving cars for hopefully obvious reasons. Strangely enough, humans tend to seemingly ignore this problem all the time.As we look at the technologies, think about them in terms of how they solve the above problems. Okay, got it? Let's drive right in!1. The car itself(modified Toyota Prius)(the current Lexus fleet)Self-driving cars are still cars, and Google's fleet has historically included Toyota Prius, Audi TT, and Lexus RX450h. Its current fleet is about two dozen Lexus SUVs outfitted with an array of hardware and software.(the latest fully driverless model)Edit: Adding in the excellent point from User-12685428141664529627, the modified cars in this fleet are not fully autonomous and do require human operations (more on that below). The latest experiment -- a built-from-scratch, truly driverless, electric prototype -- exclusively ferries Googlers around their campus (a.k.a a very well defined environment) at a top speed of 25 MPH: http://www.theguardian.com/technology/2014/may/28/google-self-driving-car-how-does-it-work2. Lasers!(raw output from the HDL-64E LIDAR)Velodyne's HDL-64E is the car's famous LIDAR system, a.k.a. the whirling laser range finder mounted on top of the car. This system works as you might think, via measuring the distance of its surroundings by bouncing its 64 lasers off of objects as it spins 360 degrees. The HDL-64E reads up to 1.3 million points per second, accurate to 2cm within a radius of ~100-120 meters. This ability comes with a hefty pricetag ($70,000), but the price of the eventual consumer model will be reduced in the next handful of years.3. Position tracking: good ol' GPS and othersJust by pinging GPS satellites and triangulation of the received radio signals, we can find out where we are in the world. One example found in Google cars is the Applanix POS LV GPS navigation system. Add in standard vehicle navigation instruments like tachometers and gyroscopes (rotational measurements), altimeters (altitude), and odometers (distance traveled), and we now have a solid package. Many modern day consumer vehicles are outfitted with these gadgets already -- and that's great news. Google's self-driving car also includes an additional nifty gadget called the wheel encoder to measure lateral movement from the left rear wheel.GPS and the other components are designed to be only accurate within a few meters, unfortunately. A few meters is a big difference. We are going to have to do much, much better.4. Google MapsThe laser mapping, its onboard GPS, and lateral movement sensors all feed into a data bucket that gets poured into the high resolution maps Google has from its extensive mapping initiative. By high resolution, I mean detailed down to the height of the curbs and the dimensions of the lane the car is currently traveling in.Google Maps work pretty well on your phone. Google Maps for self-driving cars works even better. We're adding another layer of mapping and map alignment, so imagine the entire map rotating to fit the raw data of your surrounding. If you can't solve mapping via just lasers, just throw one of the best map systems in the world at it.5. Video camerasPairs of digital video cameras are mounted, slightly separated by a known distance, around the exterior of the car. The high performance stereo cameras typically have 50 degrees FOV and accuracy up to 30 meters.The neat part here are the algorithms rather than the specific camera technologies -- which can vary, but Google does favor Point Grey (http://www.edmundoptics.com/cameras/usb-cameras/point-grey-grasshopper-3-high-performance-usb-3-0-cameras/3626/). A single image can only reliably offer 2D information, but two images of the same scene offset by a known distance allows depth information (distance away) to be derived. This concept is called stereo vision and aids in the mapping process.Notably, one more additional camera is mounted near the rear-view mirror specifically for traffic lights, signs, and pedestrians in front of the car.(bit outdated prototype, but shows the position of some of the hardware)6. Echo technology: radar and sonarWhile LIDAR and stereo camera help solve mapping, a set of four radar sensors attached to the front and back bumpers are used for accurately estimating distance and speed of the obstacles in real-time. Radar is a well established technology that bounces high frequency radio waves to do its object tracking, and this particular radar system is good for about 200 meters. Google likes Bosch Driver assistance systems - Adaptive cruise control (ACC). For some car prototypes, a smaller sonar setup that echoes sound waves out 6 meters have been tested. These systems automatically apply the brakes, pre-tension seat belts for impact, or swerve to avoid obstacles.At this point the minimalist in you may be thinking: Wow, this is a lot of stuff. Some of these systems sound redundant -- radar, sonar, GPS, laser range finders, and cameras? Do we really need everything? The answer is definitely yes. Redundancy addresses unusual driving conditions like fog or snow -- when visibility for some sensors including the LIDAR or GPS/network setups are essentially blind, and other feeds like the video cameras will spam confused inputs.7. Humans(what human drivers need to know)To the likely chagrin of the vehicle itself, Google Self-Driving Cars still need human babysitters. Humans are overwhelmingly the highest source of accidents for Google's self-driving cars (100% as of June 2015, in over 1.8 million miles). Humans are running with some highly advanced technologies like eyes that can capture 180 degrees horizontal FOV with dynamic range and movement, biological neural networks that are some of the most complex systems in the known universe, and even handheld smart phones. Yet despite of their decades of individual training data and millions of years of evolution, humans still make silly mistakes.Thankfully, driverless mode is activated for most of the time that humans are in the car. A pair of humans are tasked with monitoring the road and normal car operations when necessary. One of the earliest modifications: Grabbing that wheel works as an "OH SHI- oh whew, we're okay".8. Software algorithms(what the car sees via its probabilistic maps)Finally the car needs to understand all of its knowledge from the gathered data sources. This is where fun algorithms come in!We're going to throw these algorithms at the onboard processors; an example setup from the car would be two Xeon computers running Linux: a 12-core server for vision and laser algorithms while a 6-core server takes care of planning, control, and low-level communication. We're not going to go too deeply into any of the specific algorithms here, but we can mention some of the software challenges.Unsupervised multi-beam laser calibration - we need to understand optimal extrinsic parameters from each sensor (accounting for and to calculate things like distance, pose, orientation). Think of it as standardizing the input against all of the other input sources. Turns out, for dozens of input sources, this is pretty hard.Mapping and localization - remember the fundamental problems we considered back at the beginning? The data from LIDAR and other sensors go into algorithms like Particle filter and Kalman filter and Recursive Bayesian estimation, essentially iterative passes for positioning weighted based on Bayesian inference principles, to generate real-time probabilistic mappings of the car's surroundings. This may sound complicated. All you need to know is that the car definitely knows what it's doing -- probably.Object recognition - to know how to behave correctly, depending on whether the object is another vehicle, pedestrian, bicyclists, sign, traffic light, etc. Most of the objects are labeled through pre-trained, deep-learning, boosted classification models Boosting methods for object categorization -- one for shape of the object and one for motion of the object -- and classified objects can be then tracked using Kalman filtering.Trajectory planning - to deal with crazy human drivers and driving physics: merging into traffic flow, passing on-coming traffic, changing lanes, avoiding other vehicles, etc. For control/optimization theory, we start getting into the Bellman equation for decision-making and modeling. For the moving frame analysis, we can rely on the Frenet–Serret formulas, and create lateral and longitudinal cost functionals for different tasks as well as to mimic human-like driving behavior.Dynamic modeling and control - feeds from the previously mentioned trajectory output to control the system of the car itself. The Google car blends multiple strategies from Model predictive control, well established physically based car physics models, and PID controller for operating low-level actions like applying torque on the wheels. The planner and the control algorithms cycle to understand the motions of the world and what ought to be done next.All that plus several others major algorithmic challenges like interpreting traffic signals and signs. Pretty neat stuff to explore further, right? Here's a course on Udacity by Sebastian Thrun, one of the inventors of Google's Self-Driving Car along with his Stanford team: Artificial Intelligence for Robotics Course9. InformationLastly, the difference between Google's self-driving car project and all other similar projects is the scale of information: Google computing, its onboard processors as well as remote servers, processes up to 1GB/sec from these sensors. Remember all of the databases/architecture that folks like Jeff Dean and thousands of talented Googlers have developed over the years to handle petabytes of training data and maps, remote server farms dedicated to supporting some heavy processes that the car needs, optimized algorithms for data crunching, and innumerable other ideas? Good computer science is the true heart of the self-driving car.So if you're interested in learning more about self-driving cars: here is a fantastic paper (Page on cmu.edu), and CMU and Stanford are great universities for this research (consider applying: http://www.ri.cmu.edu, Stanford Robotics Lab). You may realize by now that much of the seemingly rocket science technologies that power the Google car isn't rocket science whatsoever; they are all just really fun computing/data/hardware challenges. If you're good with algorithms or computers or electronics, check out careers for the self-driving car project at Google: like (Robotics, Search - Google Careers) and (Systems, Search - Google Careers).Thanks for reading! And feel free to point out corrections. :)Image sources:Behind the wheel: a look inside Google's self-driving carsNHTSA Proposes Guidelines for Self-Driving Cars Photo Gallery - Motor TrendHow Google’s self-driving cars detect and avoid obstacles | ExtremeTech
- Home >
- Catalog >
- Business >
- Corporate Document >
- Sop Template >
- Standard Of Care